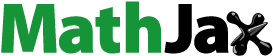
ABSTRACT
Leaf area index (LAI) is defined as one half of the total green leaf area per unit ground surface area. Its vertical profile is critical for understanding the remote sensing radiative transfer processes. LAI profile has been derived from airborne and spaceborne LiDAR data, such as the Global Ecosystem Dynamics Investigation (GEDI) installed on the International Space Station. However, the capability of various algorithms for the LAI profile estimation with airborne LiDAR is not clearly evaluated, and the estimated LAI profiles, including the GEDI LAI products, are not been fully validated. This study conducted a quantitative retrieval and validation of the LAI profiles using terrestrial and airborne laser scanning (TLS and ALS) and spaceborne GEDI data over a deciduous needleleaf forest site in northern China. The vertical LAI profile was estimated in the field using an upward digital hemispherical photography (DHP) attached to a portable measurement system in 2020 and 2021. A suite of new LiDAR indices combining both LiDAR return number and return intensity was explored for the LAI profile estimation. All LAI profiles obtained from the DHP, TLS, ALS, and GEDI during the leaf-on season and leaf-off season were compared. The DHP shows a good agreement with the TLS LAI profiles (R2 = 0.97). The LAI profile derived from the ALS data using the combined light penetration index (LPIRI) agrees well (R2 ≥0.86) with the DHP, TLS, and GEDI estimates. In general, the LPIRI is advantageous for regional LAI profile mapping from ALS. The GEDI cumulative LAI corresponds well with the DHP during the leaf-on season (R2 = 0.90, RMSE = 0.23), but underestimates during the leaf-off season (R2 = 0.70, RMSE = 0.14, bias=−0.13). The underestimation is attributed to the higher canopy and ground reflectance ratio (ρv/ρg) assigned in the algorithm and the height discrepancy between the GEDI and field measurements. For the GEDI LAI profile product, further validation and improvement are necessary for other biome types and landscape conditions, especially during the leaf-off season.
1. Introduction
The leaf area index (LAI) is an important vegetation biophysical parameter and a critical variable in photosynthesis and respiration processes (Alton Citation2016; Asner et al. Citation1998; Chen and Black Citation1992). Forests display a vertical stratification of foliage, which affects the light used in photosynthesis, and water interception (Goetz et al. Citation2007; Hopkinson et al. Citation2013). Knowledge regarding the forest LAI profile is significant for understanding remote sensing radiative transfer processes (Béland and Baldocchi Citation2021; Gastellu-Etchegorry, Martin, and Gascon Citation2004; Parker, Lefsky, and Harding Citation2001; Qi et al. Citation2022; Yang et al. Citation2020).
The LAI can be measured by direct sampling methods and indirect optical methods (Fang et al. Citation2019; Jonckheere et al. Citation2004; Weiss et al. Citation2004; Zheng and Moskal Citation2009). Both methods can be used to obtain the forest LAI profile by utilizing observation towers and mobile elevators (Clark et al. Citation2008; Wang et al. Citation1992). For example, Clark et al. (Citation2008) harvested foliage at 1.86 m height intervals via a modular tower in a tropical rain forest to obtain the landscape LAI profile. Ryu et al. (Citation2014) conducted a tower-based measurement using LAI-2200 Plant Canopy Analyzer (PCA) and digital hemispherical photography (DHP) to monitor the variation of forest vertical LAI. However, ground measurement of the LAI profile is labor-intensive and large area deployment is difficult.
On the other hand, remote sensing provides an optimal tool for LAI estimation on large scales (Fang et al. Citation2019). LAI has been derived from passive remote sensing data and active light detection and ranging (LiDAR) data (Baret et al. Citation2013; Zheng and Moskal Citation2009). However, passive remote sensing systems do not adequately capture vertical variation in LAI. In contrast, LiDAR provides unique advantages in capturing the vertical dimension information (Guo et al. Citation2021; Wang et al. Citation2019; Yan et al. Citation2019). The terrestrial laser scanning (TLS) provides a 3D point cloud from which LAI can be extracted at the individual tree or forest stand levels (Beland et al. Citation2011; Guo et al. Citation2021; Zheng et al. Citation2017; Zheng and Moskal Citation2012). However, TLS beams sampled in the upper canopy are easily obstructed by the middle and lower canopy components, which limits its broad application in forests.
The airborne laser scanning (ALS) can better characterize the upper layer and is commonly acquired at a large scale, which facilitates the LAI estimation at the regional level (Wang and Fang Citation2020). Various LiDAR indices, such as the light penetration index (LPI), canopy fractional cover index (FCI), and the above and below ratio index (ABRI), have been proposed to estimate the canopy LAI and LAI profile using various regression models (Hopkinson and Chasmer Citation2009; Sumnall et al. Citation2016, Citation2016, Citation2021; Zhao and Popescu Citation2009). However, the statistical regression models are specific to the area in which they are developed. The LiDAR indices have shown different performances in various forests (Alonzo et al. Citation2015; Sumnall et al. Citation2021; Zhao and Popescu Citation2009). Recently, Sumnall et al. (Citation2021) evaluated the capacity of eight LiDAR indices to estimate the forest overstory and understory LAIs. In their study, the forest was divided into overstory and understory with a threshold height corresponding to the height to live crown (HTLC). LiDAR indices derived from each layer were compared with field LAI, and the highest correlations are produced for overstory (R2 = 0.71) and understory (R2 = 0.49), respectively. However, the methods for the two-layer LAI may not be applicable for estimating the multi-layer LAI profiles. Moreover, it is still unclear which index is optimal for the LAI profile estimation. More extensive validation studies are necessary for the LiDAR LAI profile data estimated from various methods.
Compared to the TLS and ALS data, the spaceborne LiDAR is advantageous for forest measurement on a global scale. The Geoscience Laser Altimeter System (GLAS) was the first spaceborne full waveform LiDAR. GLAS provided the capability to map LAI and LAI profiles over large areas (Tang et al. Citation2014, Citation2016), but the process was affected by the slope and the mission was terminated in 2009. The Global Ecosystem Dynamics Investigation (GEDI) installed on the International Space Station is a spaceborne full waveform LiDAR with a 25 m footprint size and a 60 m along-track sampling interval (Dubayah et al. Citation2020; Roy, Kashongwe, and Armston Citation2021). GEDI provides high-quality measurements of vertical forest structure in temperate and tropical forests between 51.6° N and 51.6° S. A variety of footprint and gridded products (Level 1 to Level 4) were derived from GEDI observations, including canopy height, canopy cover and vertical profile, canopy LAI and LAI profile, topography, and biomass (Dubayah et al. Citation2020). GEDI Level 2B product provides the first global footprint level LAI profile data at a vertical resolution of 5 m since April 2019 (Dubayah et al. Citation2020). The LAI profile is derived from the modified Beer-Lambert method, which employs a global constant canopy and ground reflectance ratio (ρv/ρg = 1.5) at the footprint level (Dhargay et al. Citation2022; Tang and Armston Citation2020). However, whether this ratio is applicable for different biome types and temporal periods is questionable. Moreover, validation of the new GEDI LAI profile is lacking.
GEDI data are acquired throughout the year, and LAI data are available during both the leaf-on and leaf-off seasons. The LAI acquired during the leaf-off season is actually the woody area index (WAI) (Fang et al. Citation2019). WAI is an important vegetation biophysical parameter in canopy reflectance and land surface models (Bonan et al. Citation2002; Lawrence and Chase Citation2007). WAI accounts for an important component of light interception in forests (Sánchez-Azofeifa et al. Citation2009), and its presence significantly affects the snow surface albedo because of the absorption of nonphotosynthetic vegetation, the decrease of gaps in illumination, and the increase in shadows (Tian et al. Citation2004). However, optical remote sensing retrieval of WAI is difficult because of the mixed leaf and woody components in forest canopies. GEDI data products obtained during the leaf-off season provide a new data source for WAI. Therefore, it is necessary to assess the performance of the new GEDI LAI profile products during the leaf-off seasons.
As mentioned above, two questions are crucial: (1) What is the capability of various LiDAR indices and algorithms for the LAI profile estimation? (2) How does the new GEDI LAI profile product perform during both the leaf-on and leaf-off seasons? This study conducted a quantitative retrieval and validation of the LAI profiles derived from TLS, ALS, and GEDI. We aim to (1) develop a portable field measurement system for the LAI profile measurement, (2) obtain an optimal LiDAR index for the LAI profile estimation from ALS, and (3) validate the GEDI LAI profile product during both the leaf-on and leaf-off seasons. The study enhances the understanding of the capability of LAI profile estimation from different observation platforms and provides insight into the accuracy of the GEDI LAI profile product.
2. Materials and methods
The framework of this study is illustrated in , including field data collection, evaluation of LiDAR indices, and the performance of GEDI LAI profile. The vertical LAI profile was first estimated in the field using an upward DHP attached to a portable measurement system in 2020 and 2021. Then, a suite of new LiDAR indices combining both the return number and return intensity were explored for the estimation of the LAI profile from ALS. As an illustration, the optimal ALS model was used to map the LAI profile over the study area. Finally, the GEDI LAI profiles were validated by the DHP, TLS, and ALS during the leaf-on and leaf-off seasons.
Figure 1. The framework for retrieval and validation of the LAI profiles derived from DHP, TLS, ALS, and GEDI.

2.1. Study area
The location of the study area () is within the Saihanba National Forest Park (SNFP) in the Hebei Province, North China (42°24′N, 117°18′E). The dominant species of SNFP are larch (Larix principis-rupprechtii), birch (Betula platyphylla) and Mongolian pine (Pinus sylvestris var. mongolica) (Zeng and Wang Citation2015). The deciduous needleleaf forest site with larch (Larix principis-rupprechtii) was chosen as an area of interest (AOI) and for field data collection (). The terrain of the needleleaf forest site is generally flat (Figure S1).
Figure 2. The location of the study area. The left panel (a) shows the locations of all measurements and the GEDI footprints (Background Google Earth Image obtained by CNES/Airbus on June 29, 2018). The right panel shows two field pictures taken near a measurement tower (42°24′N, 117°18′E, yellow triangle in (a) during (b) leaf-on (September 6, 2020) and (c) leaf-off (April 24, 2021) seasons, respectively.

2.2. Field data collection
A total of 46 plots () were collected in September 2020 (leaf-on, 23 plots) and April 2021 (leaf-off, 23 plots), corresponding to the leaf-off GEDI footprints (gray circles in ). Twelve plots () were collected in September 2021 (leaf-on), corresponding to the leaf-on GEDI footprints (green circles in ) and the TLS site (orange rectangle in ). The plot is located at the center of the GEDI footprints and the TLS site, with a radius of 15 m (). The GEDI version 1 (V1) footprint locations were considered as the version 2 (V2) data were not available at the experiment design.
Figure 3. (a) The sampling strategy within a plot with five elementary vertical sampling points (VSPs) located at the center and four cardinal points, respectively; (b) a diagram of the portable measurement system at different heights (1.5–13.5 m) with 2 m interval; (c) field pictures were taken at 1.5–13.5 m with 2 m interval, respectively, on September 9, 2020. The upward DHP is within the red circle.

Table 1. DHP, TLS, ALS, and GEDI data used in the study. N is the number of plots for DHP and TLS and the number of footprints for GEDI, respectively.
An extensible lifting mast was used for field vertical measurement. A Nikon D5100 camera and a fisheye converter were attached to the top of the mast. The calibration of the camera is carried out following the CAN-EYE software manual (Weiss and Baret Citation2017). The field measurements were made in near dusk or cloudy conditions (Fang et al. Citation2014; Zhang et al. Citation2020).
Within each plot, five elementary vertical sampling points (VSPs) were selected. The VSPs are located at the center and four cardinal points, respectively, and a brick was used to mark the VSP position for consecutive measurements (). The measurement heights range from 1.5 m to 13.5 m in 2 m intervals (). Four upward-facing DHP images were taken at each height in VSP to ensure the measurement and environment stability. Sample upward DHP images acquired from a VSP during different seasons are shown in .
Figure 4. Upward DHP images acquired at different heights (1.5–13.5 m) with 2 m interval from the same vertical sampling point (VSP) in (a) leaf-on season (September 8, 2020) and (b) leaf-off season (April 25, 2021), respectively.

All DHP images at the same height of the five VSPs (i.e., 4 pictures/height × 5 VSPs = 20 pictures) were processed by the CAN-EYE software (version 6.49) to calculate the plot’s LAI. For the DHP images, only zenith angles varying from 0–60° were utilized to avoid the distortions of the edge. The zenith resolution and azimuth resolution were set as 2.5°, respectively. The LAI was estimated using a lookup-table technique in the CAN-EYE software (Weiss et al. Citation2000).
2.3. Derivation of LAI profile from TLS
A Riegl VZ-400 LiDAR system was used for TLS measurement at a 30 m × 30 m plot on 5 September 2020 (leaf-on, orange rectangle in ). The system employs a 1550 nm laser with a beam divergence of 0.35 mrad, and maximum range of 350 m (Zhu et al. Citation2018). The zenith angles ranged from 30° to 130°, and the azimuth angles ranged from 0° to 360°. A central scan position and four cardinal scan positions were used to minimize the occlusion effect. Eight cylindrical reflectance targets were marked as control points to register the TLS data acquired from multiple scans.
The LAI profile was derived from the TLS data using a voxel-based method which has been frequently used in similar studies (Beland et al. Citation2014; Beland, Kobayashi, and Goslee Citation2021; Hosoi and Omasa Citation2006; Zheng and Moskal Citation2012). The leaf angle distribution is assumed to be spherical and the voxel size is set to 0.05 m (Wei et al. Citation2020).
2.4. LAI profile estimation from ALS
2.4.1. ALS data and processing
A Riegl LMS-Q680i installed on the CAF-LiCHy platform was used to obtain the ALS data on September 5–17, 2018 (Pang et al. Citation2016). The detailed information about the LiDAR system is shown in .
Table 2. The parameters of airborne LiDAR system and flight.
The LiDAR return height was normalized by the digital elevation model (DEM) extracted from the ALS data. Returns from large off-nadir angles (>23°) were excluded to avoid potential uncertainties caused by the underestimated gap fraction (Liu et al. Citation2018). To reduce the impact of laser power, target area, atmosphere, and the range, the LiDAR intensity value was corrected to a user-defined standard range following (García et al. Citation2010; Gatziolis Citation2011; Hofle and Pfeifer Citation2007):
where and
are the corrected intensities and raw intensities, respectively.
is the range between the ALS instrument and the return, and
is the standard range, i.e. the average flying altitude ( = 1000 m in this study).
For the estimation of the LAI profile, the canopy and ground reflectance ratio (ρv/ρg) was first derived from the return energies of two adjacent footprints (Armston et al. Citation2013; Ni-Meister et al. Citation2010):
where ,
,
, and
are the canopy and ground return energies from two adjacent vegetation and ground footprints, respectively.
In this study, ten pairs of adjacent footprints (red adjacent forest and ground paired circles in ), each containing a forest and ground return, were randomly selected. The ALS data were clipped with a diameter size of 25 m, similar to the GEDI. and
are the mean intensity of the canopy and ground returns, respectively. To properly calculate
for the forest footprints (marked by a number in ), the 0.5 m height threshold was used to mask the ground grass contribution (Armston et al. Citation2013; Chen et al. Citation2014). The average canopy and ground reflectance ratio (ρv/ρg) at 1550 nm was calculated as 1.04 ± 0.12, which was used in the subsequent analysis. More details about the calculation of the canopy and ground reflectance ratio from ALS is shown in Appendix A.
2.4.2. LAI estimation from LPI with the Beer-Lambert model
The theoretical basis of indirect LAI estimation is the Beer-Lambert law (Nilson Citation1971):
where is the gap fraction and
is the leaf projection function.
The cumulative LAI is generally estimated from the LiDAR data following a modified Beer-Lambert method (Tang and Armston Citation2020; Tang et al. Citation2012):
where is the cumulative LAI,
is the gap fraction, and z0 is the height of the canopy bottom.
,
and
are the energy from the top of the canopy to the height z, from the canopy to bottom, and from the ground, respectively.
Based on Eqs. (4) and (5), the for layer
is calculated as:
where the light penetration index (LPI) for layer is calculated as the ratio of the LiDAR return number (LPIR) or intensity (LPIInt) going through a specific layer (dz) to those reaching the top of the layer (z + dz) (). The ground and canopy reflectance ratio
was derived during the ALS data processing procedure (see 2.4.1).
Table 3. Various LiDAR indices derived from the ALS return number and intensity*.
To harness the advantages of LiDAR return number and intensity, a suite of new LiDAR indices calculated as a weighted linear average of the original indices, was explored for the LAI profile estimation. The combined light penetration index (LPIRI) for layer is expressed as:
where the subscripts R and Int denote the ALS return and intensity, respectively, and RI indicates the new index combining both ALS return and intensity.
Accordingly, the for layer
was calculated as:
2.4.3. LAI estimation from linear regression model
LAI was also estimated from the FCI and ABRI using the linear regression method (Luo et al. Citation2015; Richardson, Moskal, and Kim Citation2009; Zhao and Popescu Citation2009). In this case, the for layer
was calculated as:
where ,
,
and
are the regression coefficients. The FCI and ABRI are derived from the ALS return number, intensity, and both ().
Likewise, the combined fractional cover index (FCIRI) for layer is expressed as:
and the combined above and below ratio index (ABRIRI) for layer is expressed as:
where R and Int denote the ALS return and intensity, respectively. The subscript all refer to all returns, and represents a height threshold. For example,
is the number of returns above the height
.
For the linear regression method, all leaf-on DHP data (34 plots in September 2020 and 2021, 238 heights) were divided into two parts: one half (119 heights) for training the ALS model to estimate LAI and the other half (119 heights) for the validation purpose.
2.4.4. Mapping the vertical LAI profile
Nine LiDAR indices () were investigated to estimate the LAI profile from 1.5 m to the maximum return height. All LiDAR returns were used to calculate the indices as previous studies have indicated that it would increase the point density throughout the canopy and may improve the estimation of LAI (Riano et al. Citation2004; Sumnall et al. Citation2016). Subsequently, the estimated ALS profile was validated using both the DHP and TLS estimates and compared with the GEDI product.
To illustrate the vertical LAI profile, the optimal ALS model was used to map the LAI profile for the 1 km × 1.5 km region (red box in ). The grid resolution and height intervals are 25 m and 5 m, respectively, similar to the GEDI footprint size and vertical resolution.
2.5. Validation of the GEDI LAI profile product
The cumulative vertical LAI profile was distributed in the GEDI Level 2 product (L2B) at 5 m vertical resolution (Dubayah et al. Citation2020). The GEDI L2B data product explored in the study were acquired on 21 May 2019 and 24 August 2020, respectively (gray and green circles in ). A total of 34 sample footprints were selected by the quality flag parameter (quality_flag = 1) for subsequent analyses (). The slopes of the GEDI footprints are less than 7° (Table S1). The GEDI V2 LAI profiles were explored to examine their performance (Dubayah et al. Citation2021). The GEDI V2 LAI profile was compared to those derived from the DHP, TLS, and ALS during the leaf-on season. During the leaf-off season, because of the lack of the TLS and ALS data, the GEDI V2 profile was compared to the DHP profile only.
2.6. Statistical analysis
All LAI profiles obtained from the DHP, TLS, ALS, and GEDI were inter-compared. The R2, RMSE, and bias metrics were used to evaluate the LAI estimation.
where and
are the reference LAI and estimated LAI, respectively, and
is the mean reference LAI value.
3. Results
3.1. Comparison of ALS indices for the estimation of LAI profile
This section first presents the relationships between the DHP LAI and various ALS indices based on the training data, and then compares the LAI estimated from ALS with the DHP data. Finally, the LAI derived from different ALS indices is compared with the TLS measurements.
shows the linear relationships constructed between the DHP LAI profile and the FCIs and ABRIs from the training data. For the layered LAI (), the FCIR shows a good correspondence with the DHP LAI (R2 = 0.88, RMSE = 0.10, ), while the ABRI indices show a weaker relationship (R2 <0.83, ). For the cumulative LAI (), all of the correlation values are similar to those for the layered LAI, with R2 and RMSE values ranging from 0.75–0.89 and 0.19–0.29, respectively. The FCIR and FCIRI are similar, and both show a significant correspondence with the DHP measurements (), whereas the ABRI indices show a slightly weaker relationship, especially for the ABRIInt ().
Figure 5. Relationships between the DHP layered and cumulative LAI (September 2020 and 2021) and various ALS (September 2018) indices from the training data. (a-c) and (g-i) the canopy fractional cover indices from return (FCIR), intensity (FCIInt), and both (FCIRI), respectively; (d-f) and (j-l) the above and below ratio indices from return (ABRIR), intensity (ABRIInt), and both (ABRIRI), respectively. The vertical color bar in (a) and (g) indicates the different heights for layered LAI and cumulative LAI, respectively. The index values are normalized to 0–1. The black line represents the regression line.

The FCI and ABRI models () were used to derive the vertical LAI profile, which was then compared with the layered DHP LAI. compares the layered LAI estimated from the ALS indices with those derived from DHP. The layered LAI from ALS is generally consistent with the DHP LAI (R2 >0.80, RMSE <0.15, ). The LPIRI model shows good correspondence with the DHP measurements (R2 = 0.86, RMSE = 0.13) with a slope close to the 1:1 line (), while the LPIR model slightly overestimates (bias = 0.06) and the LPIInt slightly underestimates (bias = −0.07). Similar results were obtained from the FCIR, FCIInt, and FCIRI models (R2 ≥0.87, ). The ABRIInt model shows the lowest correspondence (R2 = 0.81, RMSE = 0.14, ), and the results are more scatterly distributed than those of the other models, especially at >11.5 m. The ABRIR and ABRIRI models perform similarly and show better results than the ABRIInt ().
Figure 6. Comparison of the layered LAI estimated from ALS (September 2018) with the validation DHP data (September 2020 and 2021) during the leaf-on season. (a-c) 34 plots with the Beer-Lambert method; (d-i) 17 plots with the linear regression method.

Table 4. Comparison of statistics between the LAI profiles derived from various ALS indices () and those from DHP (34 plots, 238 heights) during the leaf-on season.
shows the cumulative LAI derived from various ALS indices compared with the DHP measurements. The ALS outputs generally show good consistency with the DHP measurements (R2 ≥0.84, RMSE ≤0.26, ). The LPIRI and FCI models show similar performance (R2 ≥0.89, ), whereas the ABRIInt model results in the lowest correspondence (). The LPIR shows a small overestimation, especially at >7.5 m (). The LPIInt systematically underestimates (bias = −0.28, ), while the FCI and ABRI underestimate for LAI >2.
Figure 7. Comparison of the cumulative LAI estimated from ALS (September 2018) with the validation DHP data (September 2020 and 2021) during the leaf-on season. (a-c) 34 plots for Beer-Lambert; (d-i) 17 plots for linear regression method.

The statistics for the different ALS indices are summarized in . For the layered LAI (), the FCIRI displays the highest agreement with DHP (R2 = 0.88), while the ABRIInt produces the lowest agreement. For the cumulative LAI (), the LPIRI and FCIs perform similarly and show the best correspondence with the DHP (R2 ≈0.90). The ABRIInt model shows the lowest correspondence. The performance of the return intensity models is similar to that of the return number models, especially for the LPI and FCI models, whereas the ABRIInt model performance is slightly inferior to the ABRIR and ABRIRI models.
compares the ALS LAI profiles derived from different LiDAR indices with the TLS measurements at 2 m height interval. In general, the LAI profiles estimated from ALS agree well with those from the TLS (R2 ≥0.78). The LAI profile from the LPIRI model shows the best correspondence with those from the TLS (R2 = 0.95, RMSE = 0.05, ), while the LPIR shows a slightly weaker performance (R2 = 0.88, RMSE = 0.07, ). The ABRIInt model shows the weakest correlation and slightly overestimates the TLS (R2 = 0.78, RMSE = 0.09, ). All the other models show moderate correlations (R2 >0.80, RMSE ≤0.08).
3.2. Vertical LAI distribution maps from ALS
The vertical LAI distribution maps of the study area were generated at 25 m resolution from ALS. The LAI profile maps derived from the LPIRI method are presented in . The LAI is close to 0 in the bare land and road areas, and the highest total LAI value reaches about 3.07. The LAI of each layer shows a geographic pattern generally similar to the total LAI map. However, different patterns exist for different layers. The highest LAI is distributed at 10–15 m (0.73 ± 0.35), while the lowest LAI is observed at 0–5 m (0.11 ± 0.11). The 5–10 m (0.38 ± 0.26) and >15 m (0.36 ± 0.31) layers show moderate LAI values. The vertical LAI maps were also generated using the other LiDAR indices (Figure S2). The results are generally similar to those of the LPIRI. However, the FCI and ABRI methods show a slight underestimation of the total LAI compared to those of the LPI models.
3.3. Comparison of LAI profiles derived from different systems
The LAI profiles derived from the GEDI V2, ALS, TLS, and DHP are compared in and . The LAI values derived from ALS (1.98 ± 0.42) and DHP (1.92 ± 0.35) are generally similar during the leaf-on season ( and ). The LAI values derived from DHP (1.92 ± 0.35, ) and GEDI (1.90 ± 0.29, ) during the leaf-on season are clearly larger than those from the leaf-off season (DHP: 1.12 ± 0.17, GEDI: 0.68 ± 0.16, ), especially for >5.5 m. At <5.5 m, the LAI profiles derived from all systems and seasons are nearly the same (~0.10–0.20, and ) because of the dominant tree trunks at this height. For TLS, the maximum LAI profile appears at 13.5–15.5 m (). As shown in Appendix B, the TLS and DHP LAI profiles are highly correlated (R2 = 0.97 and RMSE = 0.06, ).
Figure 10. The vertical LAI profiles derived from (a) DHP (September 2020 and 2021) and ALS (September 2018) during the leaf-on (34 plots) and leaf-off (23 plots in April 2021) seasons, and (b) TLS and GEDI V2 during the leaf-on (1 plot for TLS in September 2020, and 11 plots for GEDI in August 2020) and leaf-off (23 plots for GEDI in May 2019) seasons. The ALS LAIs were derived from LPIRI. The line and shades represent the mean and standard deviation values, respectively. Subscripts “on” and “off” indicate the leaf-on and leaf-off seasons, respectively.

Table 5. The mean LAI at different heights and the total LAI obtained from DHP, TLS, ALS, and GEDI V2 at different seasons, respectively. The ALS LAIs were derived from LPIRI. The values of the standard deviations are shown in the brackets. All LAI profile data are from .
3.4. The performance of the GEDI LAI profile data products
compares the LAI profiles derived from the GEDI V2 and DHP during the leaf-on season. The GEDI profile generally shows moderate correspondence with the DHP LAI (R2 = 0.49, RMSE = 0.21, ). At <15 m, the GEDI profile correlates well with the DHP value (R2 = 0.77, RMSE = 0.14, ). At >15 m, the GEDI values are generally lower than the DHP values (bias = −0.24, ). The cumulative LAI from the GEDI presents good correspondence with the DHP LAI (R2 = 0.90, RMSE = 0.23, ). The LAI profile derived from GEDI also agrees very well with the TLS LAI during the leaf-on season ().
Figure 11. Comparison of the layered LAI at (a) all heights, (b) 0–15 m, and (c) > 15 m and (d) the cumulative LAI derived from GEDI V2 (August 2020) with the DHP measurements (September 2021) during the leaf-on season (11 plots).

The LAI profiles derived from the GEDI V2 and DHP are also compared during the leaf-off season (). The GEDI profile shows poor correspondence with the DHP LAI (R2 = 0.04, RMSE = 0.10, ). At <15 m, the GEDI values correlate moderately with the DHP (R2 = 0.40, RMSE = 0.08, ). However, at >15 m, the GEDI LAI significantly underestimates (~0.43) the DHP LAI (). For the cumulative LAI values, the correlation coefficient is better than that for the layered LAI (R2 = 0.70), although the GEDI still shows a slight underestimation (bias = −0.13, ).
Figure 12. Comparison of the layered LAI at (a) all heights, (b) 0–15 m, and (c) > 15 m and (d) the cumulative LAI derived from GEDI V2 (May 2019) and DHP (April 2021) during the leaf-off season (23 plots).

compares the cumulative LAI of ALS and GEDI V2 during the leaf-on season. In general, the LAI profiles estimated from the various LiDAR indices agree well with those from the GEDI (R2 >0.85). The LPIRI model presents the best results among all models (R2 = 0.93, RMSE = 0.20, ). The LPIR model presents a systemic overestimation (bias = 0.22), whereas the LPIInt presents a systemic underestimation (bias = −0.25). Similar performance is observed for the other models (). Compared with the LPIRI model, the FCI and ABRI models show a slight underestimation of the cumulative LAI.
4. Discussion
4.1. LAI profile measurement with DHP
In this study, a portable field measurement system was developed to estimate the vertical LAI profile using upward-facing DHP images at different heights (). This system mitigates the challenges of tower-based measurements in representing large sampling areas and provides a promising method for validating the vertical LAI profile derived from ALS. The maximum height of the DHP system in this study is 13.5 m. A system utilizing a greater height would improve the LAI measurement in the upper canopy, but the system is prone to leaning at a higher level. Therefore, the maximum measurement height is a tradeoff between the canopy height and system stability.
4.2. Estimation of the LAI profile from LiDAR indices
Various LiDAR indices based on the return number and intensity were assessed in the LAI profile estimation. The LAI profiles estimated from ALS agree well with those from the DHP (R2 ≥0.81) (). The ALS performance is comparable with those reported in other similar studies (Sumnall et al. Citation2016, Citation2021; Zhao and Popescu Citation2009). In this study, a new LiDAR index combining both the return number and return intensity was proposed to estimate the LAI profile. Other researchers have used only return number or intensity in similar studies (Arnqvist, Freier, and Dellwik Citation2020; Hopkinson and Chasmer Citation2009; Solberg et al. Citation2009; Sumnall et al. Citation2021). Compared with the original indices based only on the return number (R2 = 0.86–0.88, RMSE = 0.11–0.15) or return intensity (R2 = 0.81–0.87, RMSE = 0.11–0.14), the new combined index improves the LAI estimation (R2 = 0.83–0.88, RMSE = 0.11–0.13, ). Among the combined indices, the performance of LPIRI is superior to those of FCIRI and ABRIRI, indicating that the LPIRI with the Beer-Lambert law may be more suitable for LAI estimation than the linear regression model with FCIRI and ABRIRI (). The LPIRI model is physically-based and less affected by site conditions and generally obtains the best results. The model may be useful for LAI mapping from ALS on a larger scale.
With the FCI models, the LAI values are slightly underestimated for LAI >2 (). This may possibly be attributed to the limited number of samples for LAI >2 in this study. Similar findings with FCI have been reported in other studies (Sumnall et al. Citation2016). The results from ABRI are similar to those from FCI, possibly because of FCI and ABRI are arithmetically exchangeable. Nevertheless, the FCI and ABRI regression models require sufficient amount of field data during the training process and are site-specific.
The LAI profiles estimated from ALS also show good agreement with TLS (). The LPIRI is superior to other indices (R2 = 0.95, RMSE = 0.05). The small differences between ALS and TLS are mainly attributed to the different observation systems. The ALS on fixed wing aircraft may better characterize the upper layer when looking downward, while the lower canopy is easily obstructed by the upper canopy. Using both TLS and ALS may help alleviate the occlusion effects and improve the LAI estimation (Hosoi, Nakai, and Omasa Citation2010). In this study, the TLS data were available in only one site, and more TLS data are necessary to evaluate the performance of different LiDAR indices in the future.
All of the ALS first, intermediate, and last returns were used to calculate the LiDAR indices. Using all returns not only provides increased point sampling density throughout the canopy to the ground, but also minimizes the LAI bias usually induced by using only the first or last return (Hopkinson and Chasmer Citation2009; Lovell et al. Citation2003). and show that slightly better results can be obtained using the return number than using the return intensity. In theory, the return intensity derived from radiometric information is more suitable for LAI estimation than the return number derived from point density (Yin, Cook, and Morton Citation2022; Yin et al. Citation2020). In practice, however, the intensity data are easily affected by atmospheric and aircraft conditions which greatly hampers the LAI estimation (García et al. Citation2010; Luo et al. Citation2019). Nonetheless, our results show that the LiDAR intensity data can be used to estimate the forest LAI profile satisfactorily (R2 >0.75).
4.3. Performance of the GEDI LAI profile
The GEDI LAI product was compared to the DHP LAI at different seasons. During the leaf-on season, the GEDI layered LAI generally showed moderate correspondence with the DHP LAI profile (R2 = 0.49, RMSE = 0.21, ). However, the GEDI layered LAI values were smaller than the DHP values at >15 m (bias = −0.24, ). On the other hand, the cumulative LAI from GEDI showed good correspondence with those of the DHP (R2 = 0.90, RMSE = 0.23, ). The main reason for the negative GEDI layered LAI (>15 m) discrepancy is attributed to the height mismatch between GEDI and DHP. The maximum height of the DHP system in this study is 13.5 m, and the layered LAI >15 m derived from DHP actually represents the LAI from 13.5 to the canopy top. Moreover, as shown in Appendix C, the layered LAI usually peaks at 13.5 − 15.5 m in the study area (0.39 ± 0.09, and ). Another reason may be because of the increasing LAI spatial variation at higher canopy. The LAI spatial variation generally increases with canopy height with the increasing of large gaps in tall trees (Wang et al. Citation1992).
During the leaf-off season, the GEDI products underestimated the layered and cumulative LAI values, especially at the upper canopy layers (>15 m) (). This underestimation is mainly attributed to the global static canopy and ground reflectance ratio (ρv/ρg = 1.5) adopted in the GEDI algorithm (Tang and Armston Citation2020). In the study area, the typical ρv/ρg value is around 1.04 ± 0.12 during the leaf-on season (). Other studies, such as the RAdiation transfer Model Intercomparison IV (RAMI-IV) project has indicated even smaller ρv/ρg values (0.71 ± 0.09, ) during the leaf-off season (Appendix D). These values are significantly smaller than the GEDI assignment. Like the leaf-on season, the underestimation is also caused by the height mismatch between GEDI and DHP. Furthermore, the stronger ground return in the absence of leaves and the weak return energy from the small twigs and branches in the upper canopy may also bring retrieval uncertainties during the leaf-off season.
4.4. Woody area measurement during the leaf-off season
In the study, the LAI profile was determined from DHP and LiDAR at different seasons. During the leaf-on season, the LAI is actually the plant area index (PAI) since it consists of both leaf and woody components, while the LAI obtained during the leaf-off season is the woody area index (WAI) (Fang et al. Citation2019). As shown in this study, the GEDI data obtained during the leaf-off season can be used to represent the WAI. However, estimation of the WAI from LiDAR data during the leaf-off season is usually difficult because of the relatively lower WAI value and weaker canopy return. The DHP system experimented in this study provides a promising way to determining the WAI profile in the leaf-off season (). For the study area, the WAI values obtained from DHP are around 1.12 ± 0.17 (). The value is slightly lower than the WAI value (1.4) reported for a similar temperate larch forest in Japan (Hirata et al. Citation2007). Indeed, more studies are necessary to obtain the WAI profile which is required in various canopy reflectance and land surface models.
4.5. Limitations
In this study, the ALS data were acquired in 2018, and the DHP data in 2020–2021. The two-year difference between the ALS and DHP data may introduce errors in the evaluation of the ALS indices (Shao et al. Citation2019; Sumnall et al. Citation2021). However, this impact is small in this study because of the short growing season (from May to September) and the slow forest growth rate in the SNFP (Li et al. Citation2020). Moreover, there is no deforestation in the protected study area during the study period. In this study, the TLS data were available in only one site. For a robust analysis, more TLS data are necessary to demonstrate the suitability of the DHP measurement system.
5. Conclusions
In this study, we introduced a quantitative retrieval and validation of the LAI profile estimation from TLS, ALS, and GEDI over a deciduous needleleaf forest site in northern China. The LAI profile data were obtained in the field with a portable and extensible DHP measurement system at different seasons. With enhanced heights and stability, similar measurements can be conducted in more areas to obtain field reference data for the validation of the emerging LAI profile product. The LAI profile estimation from ALS can be improved with the proposed LiDAR index, which combines both the return number and intensity. The Beer-Lambert model with the combined light penetration index (LPIRI) performs best when compared with the DHP, TLS, and GEDI data (R2 ≥0.86), and is recommended for the LAI profile mapping over larger areas.
The GEDI cumulative LAI product performs well with the field measurements during the leaf-on season, but shows a negative bias during the leaf-off season, especially for the upper canopy layer. The negative bias is attributed to the global constant canopy and ground reflectance ratio (ρv/ρg = 1.5) and the height discrepancies between the GEDI and field measurements. Further improvement of the GEDI LAI algorithm can be attained with locally adjusted ρv/ρg value and ancillary data. Further validation studies are necessary for the GEDI LAI product across various biome types and landscape conditions, especially during the leaf-off season.
Highlights
A portable field measurement system was developed to measure the LAI profile.
The combined light penetration index (LPIRI) is recommended for the LAI profile estimation from ALS.
GEDI cumulative LAI performs well during the leaf-on season.
GEDI cumulative LAI shows an underestimation during the leaf-off season.
Nomenclature
θ | = | Zenith angle |
z | = | Height in the canopy |
= | Leaf projection function | |
J0 | = | Transmitted laser pulse energy |
= | Gap fraction at zenith angle θ | |
= | Gap fraction at height z | |
Iraw | = | The raw return intensity |
Icorrected | = | The corrected return intensity |
R0 | = | The range between the ALS instrument and the return |
Rs | = | The standard range (the average flying altitude) |
ρg | = | The ground reflectance |
ρv | = | The canopy reflectance |
Rg | = | The laser energies from the ground return |
Rv(0) | = | The laser energies from the canopy top to bottom |
Rv(z) | = | The laser energies from the canopy top to height z |
Rv1 and Rv2 | = | The canopy return energies from two adjacent footprints |
Rg1 and Rg2 | = | The ground return energies from two adjacent footprints |
R2 | = | Coefficient of determination |
ALS | = | Airborne Laser Scanning |
DEM | = | Digital Elevation Model |
DHP | = | Digital Hemispherical Photography |
GEDI | = | Global Ecosystem Dynamics Investigation |
GLAS | = | Geoscience Laser Altimeter System |
LAI | = | Leaf Area Index |
LAIcum | = | Cumulative Leaf Area Index |
LAIi | = | Leaf Area Index for layer i |
LiDAR | = | Light Detection and Ranging |
PAI | = | Plant Area Index |
RMSE | = | Root Mean Square Error |
SNFP | = | Saihanba National Forest Park |
TLS | = | Terrestrial Laser Scanning |
VSP | = | Vertical Sampling Point |
WAI | = | Woody Area Index |
ABRI | = | Above and Below Ratio Index |
ABRIR | = | Above and Below Ratio Index from Returns |
ABRIInt | = | Above and Below Ratio Index from return Intensity |
ABRIRI | = | Above and Below Ratio Index combining Returns and Intensity |
FCI | = | canopy Fractional Cover Index |
FCIR | = | canopy Fractional Cover Index from Returns |
FCIInt | = | canopy Fractional Cover Index from return Intensity |
FCIRI | = | canopy Fractional Cover Index combining Returns and Intensity |
LPI | = | Light Penetration Index |
LPIR | = | Light Penetration Index from Returns |
LPIInt | = | Light Penetration Index from return Intensity |
LPIRI | = | Light Penetration Index combining Returns and Intensity |
Supplemental Material
Download (2.1 MB)Acknowledgments
This study was mainly supported by the National Natural Science Foundation of China (42171358) and the National Key Research and Development Program of China (2016YFA0600201) to H.F. The TLS data were provided by Dr. Jie Zou, Fuzhou University. The GEDI data are available from the NASA’s Land Processes Distributed Active Archive Center (LP DAAC). Drs. Lu Xu and Lixia Ma helped with the field data collection and the TLS data, respectively. The insightful comments provided by the anonymous reviewers helped improve the manuscript.
Disclosure statement
No potential conflict of interest was reported by the authors.
Data availability statement
The field measurement data in this study are available from the corresponding author, Y.W., upon reasonable request.
Supplementary Material
Supplemental data for this article can be accessed online at https://doi.org/10.1080/15481603.2023.2214987
Additional information
Funding
References
- Alonzo, M., B. Bookhagen, J. P. McFadden, A. Sun, and D. A. Roberts. 2015. “Mapping Urban Forest Leaf Area Index with Airborne Lidar Using Penetration Metrics and Allometry.” Remote Sensing of Environment 162: 141–29. doi:10.1016/j.rse.2015.02.025.
- Alton, P. B. 2016. “The Sensitivity of Models of Gross Primary Productivity to Meteorological and Leaf Area Forcing: A Comparison Between a Penman-Monteith Ecophysiological Approach and the MODIS Light-Use Efficiency Algorithm.” Agricultural and Forest Meteorology 218: 11–24. doi:10.1016/j.agrformet.2015.11.010.
- Armston, J., M. Disney, P. Lewis, P. Scarth, S. Phinn, R. Lucas, P. Bunting, and N. Goodwin. 2013. “Direct Retrieval of Canopy Gap Probability Using Airborne Waveform Lidar.” Remote Sensing of Environment 134: 24–38. doi:10.1016/j.rse.2013.02.021.
- Arnqvist, J., J. Freier, and E. Dellwik. 2020. “Robust Processing of Airborne Laser Scans to Plant Area Density Profiles.” Biogeosciences 17: 5939–5952. doi:10.5194/bg-17-5939-2020.
- Asner, G. P., B. H. Braswell, D. S. Schimel, and C. A. Wessman. 1998. “Ecological Research Needs from Multiangle Remote Sensing Data.” Remote Sensing of Environment 63: 155–165. doi:10.1016/S0034-4257(97)00139-9.
- Baret, F., M. Weiss, R. Lacaze, F. Camacho, H. Makhmara, P. Pacholcyzk, and B. Smets. 2013. “GEOV1: LAI and FAPAR Essential Climate Variables and FCOVER Global Time Series Capitalizing Over Existing Products. Part1: Principles of Development and Production.” Remote Sensing of Environment 137: 299–309. doi:10.1016/j.rse.2012.12.027.
- Béland, M., and D. D. Baldocchi. 2021. “Vertical Structure Heterogeneity in Broadleaf Forests: Effects on Light Interception and Canopy Photosynthesis.” Agricultural and Forest Meteorology 307: 108525. doi:10.1016/j.agrformet.2021.108525.
- Beland, M., D. D. Baldocchi, J. L. Widlowski, R. A. Fournier, and M. M. Verstraete. 2014. “On Seeing the Wood from the Leaves and the Role of Voxel Size in Determining Leaf Area Distribution of Forests with Terrestrial LiDar.” Agricultural and Forest Meteorology 184: 82–97. doi:10.1016/j.agrformet.2013.09.005.
- Beland, M., H. Kobayashi, and S. Goslee. 2021. “Mapping Forest Leaf Area Density from Multiview Terrestrial Lidar.” Methods in Ecology and Evolution 12 (4): 619–633. doi:10.1111/2041-210X.13550.
- Beland, M., J. L. Widlowski, R. A. Fournier, J. F. Cote, and M. M. Verstraete. 2011. “Estimating Leaf Area Distribution in Savanna Trees from Terrestrial LiDar Measurements.” Agricultural and Forest Meteorology 151 (9): 1252–1266. doi:10.1016/j.agrformet.2011.05.004.
- Bonan, G. B., S. Levis, L. Kergoat, and K. W. Oleson. 2002. “Landscapes as Patches of Plant Functional Types: An Integrating Concept for Climate and Ecosystem Models.” Global Biogeochemical Cycles 16 (2): 5–23. doi:10.1029/2000GB001360.
- Boucher, P. B., S. Hancock, D. A. Orwig, L. Duncanson, J. Armston, H. Tang, K. Krause, et al. 2020. “Detecting Change in Forest Structure with Simulated GEDI Lidar Waveforms: A Case Study of the Hemlock Woolly Adelgid (HWA; Adelges Tsugae) Infestation.” Remote Sensing 12: 25. doi:10.3390/rs12081304.
- Bouvier, M., S. Durrieu, R. A. Fournier, and J. -P. Renaud. 2015. “Generalizing Predictive Models of Forest Inventory Attributes Using an Area-Based Approach with Airborne LiDar Data.” Remote Sensing of Environment 156: 322–334. doi:10.1016/j.rse.2014.10.004.
- Chen, J. M., and T. A. Black. 1992. “Defining Leaf Area Index for Non-Flat Leaves.” Plant, Cell & Environment 15: 421–429. doi:10.1111/j.1365-3040.1992.tb00992.x.
- Chen, X. T., M. I. Disney, P. Lewis, J. Annston, J. T. Han, and J. C. Li. 2014. “Sensitivity of Direct Canopy Gap Fraction Retrieval from Airborne Waveform Lidar to Topography and Survey Characteristics.” Remote Sensing of Environment 143: 15–25. doi:10.1016/j.rse.2013.12.010.
- Clark, D. B., P. C. Olivas, S. F. Oberbauer, D. A. Clark, and M. G. Ryan. 2008. “First Direct Landscape-Scale Measurement of Tropical Rain Forest Leaf Area Index, a Key Driver of Global Primary Productivity.” Ecology Letters 11: 163–172. doi:10.1111/j.1461-0248.2007.01134.x.
- Dhargay, S., C. S. Lyell, T. P. Brown, A. Inbar, G. J. Sheridan, and P. N. J. Lane. 2022. “Performance of GEDI Space-Borne LiDar for Quantifying Structural Variation in the Temperate Forests of South-Eastern Australia.” Remote Sensing 14 (15): 3615. Remote Sensing, 14. doi:10.3390/rs14153615.
- Dubayah, R., J. B. Blair, S. Goetz, L. Fatoyinbo, M. Hansen, S. Healey, M. Hofton, et al. 2020. “The Global Ecosystem Dynamics Investigation: High-Resolution Laser Ranging of the Earth’s Forests and Topography.” Science of Remote Sensing 1: 100002. doi:10.1016/j.srs.2020.100002.
- Dubayah, R., H. Tang, J. Armston, S. Luthcke, M. Hofton, and J. Blair. 2020. GEDI L2B Canopy Cover and Vertical Profile Metrics Data Global Footprint Level V001 [Data Set]. In: NASA EOSDIS Land Processes DAAC.
- Dubayah, R., H. Tang, J. Armston, S. Luthcke, M. Hofton, and J. Blair. 2021. “GEDI L2B Canopy Cover and Vertical Profile Metrics Data Global Footprint Level V002.” PLos One 16 (8). doi:10.1371/journal.pone.0256571.
- Fang, H. L., F. Baret, S. Plummer, and G. Schaepman-Strub. 2019. “An Overview of Global Leaf Area Index (LAI): Methods, Products, Validation, and Applications.” Reviews of Geophysics 57: 739–799. doi:10.1029/2018RG000608.
- Fang, H. L., W. J. Li, S. S. Wei, and C. Y. Jiang. 2014. “Seasonal Variation of Leaf Area Index (LAI) Over Paddy Rice Fields in NE China: Intercomparison of Destructive Sampling, LAI-2200, Digital Hemispherical Photography (DHP), and AccuPar Methods.” Agricultural and Forest Meteorology 198: 126–141. doi:10.1016/j.agrformet.2014.08.005.
- Fang, H. L., Y. H. Zhang, S. S. Wei, W. J. Li, Y. C. Ye, T. Sun, and W. W. Liu. 2019. “Validation of Global Moderate Resolution Leaf Area Index (LAI) Products Over Croplands in Northeastern China.” Remote Sensing of Environment 233: 19. doi:10.1016/j.rse.2019.111377.
- García, M., D. Riaño, E. Chuvieco, and F. M. Danson. 2010. “Estimating Biomass Carbon Stocks for a Mediterranean Forest in Central Spain Using LiDar Height and Intensity Data.” Remote Sensing of Environment 114: 816–830. doi:10.1016/j.rse.2009.11.021.
- Gastellu-Etchegorry, J. P., E. Martin, and F. Gascon. 2004. “DART: A 3D Model for Simulating Satellite Images and Studying Surface Radiation Budget.” International Journal of Remote Sensing 25: 73–96. doi:10.1080/0143116031000115166.
- Gatziolis, D. 2011. “Dynamic Range-Based Intensity Normalization for Airborne, Discrete Return Lidar Data of Forest Canopies.” Photogrammetric Engineering & Remote Sensing 77: 251–259. doi:10.14358/PERS.77.3.251.
- Goetz, S., D. Steinberg, R. Dubayah, and B. Blair. 2007. “Laser Remote Sensing of Canopy Habitat Heterogeneity as a Predictor of Bird Species Richness in an Eastern Temperate Forest, USA.” Remote Sensing of Environment 108: 254–263. doi:10.1016/j.rse.2006.11.016.
- Guo, Q. H., Y. J. Su, T. Y. Hu, H. C. Guan, S. C. Jin, J. Zhang, X. X. Zhao, et al. 2021. “Lidar Boosts 3D Ecological Observations and Modelings: A Review and Perspective.” IEEE Geoscience and Remote Sensing Magazine 9: 232–257. doi:10.1109/MGRS.2020.3032713.
- Hirata, R., T. Hirano, N. Saigusa, Y. Fujinuma, K. Inukai, Y. Kitamori, Y. Takahashi, and S. Yamamoto. 2007. “Seasonal and Interannual Variations in Carbon Dioxide Exchange of a Temperate Larch Forest.” Agricultural and Forest Meteorology 147: 110–124. doi:10.1016/j.agrformet.2007.07.005.
- Hofle, B., and N. Pfeifer. 2007. “Correction of Laser Scanning Intensity Data: Data and Model-Driven Approaches.” Isprs Journal of Photogrammetry & Remote Sensing 62: 415–433. doi:10.1016/j.isprsjprs.2007.05.008.
- Hopkinson, C., and L. Chasmer. 2009. “Testing LiDar Models of Fractional Cover Across Multiple Forest Ecozones.” Remote Sensing of Environment 113: 275–288. doi:10.1016/j.rse.2008.09.012.
- Hopkinson, C., J. Lovell, L. Chasmer, D. Jupp, N. Kljun, and E. van Gorsel. 2013. “Integrating Terrestrial and Airborne Lidar to Calibrate a 3D Canopy Model of Effective Leaf Area Index.” Remote Sensing of Environment 136: 301–314. doi:10.1016/j.rse.2013.05.012.
- Hosoi, F., Y. Nakai, and K. Omasa. 2010. “Estimation and Error Analysis of Woody Canopy Leaf Area Density Profiles Using 3-D Airborne and Ground-Based Scanning Lidar Remote-Sensing Techniques.” IEEE Transactions on Geoscience & Remote Sensing 48: 2215–2223. doi:10.1109/TGRS.2009.2038372.
- Hosoi, F., and K. Omasa. 2006. “Voxel-Based 3-D Modeling of Individual Trees for Estimating Leaf Area Density Using High-Resolution Portable Scanning Lidar.” IEEE Transactions on Geoscience & Remote Sensing 44: 3610–3618. doi:10.1109/TGRS.2006.881743.
- Jonckheere, I., S. Fleck, K. Nackaerts, B. Muys, P. Coppin, M. Weiss, and F. Baret. 2004. “Review of Methods for in situ Leaf Area Index Determination - Part I. Theories, Sensors and Hemispherical Photography.” Agricultural and Forest Meteorology 121: 19–35. doi:10.1016/j.agrformet.2003.08.027.
- Lawrence, P. J., and T. N. Chase. 2007. “Representing a New MODIS Consistent Land Surface in the Community Land Model (CLM 3.0).” Journal of Geophysical Research: Biogeosciences 112. doi:10.1029/2006JG000168.
- Li, L. Y., J. Chen, X. H. Mu, W. H. Li, G. J. Yan, D. H. Xie, and W. M. Zhang. 2020. “Quantifying Understory and Overstory Vegetation Cover Using UAV-Based RGB Imagery in Forest Plantation.” Remote Sensing 12 (1): 18.
- Liu, J., A. K. Skidmore, S. Jones, T. J. Wang, M. Heurich, X. Zhu, and Y. F. Shi. 2018. “Large Off-Nadir Scan Angle of Airborne LiDar Can Severely Affect the Estimates of Forest Structure Metrics.” Isprs Journal of Photogrammetry & Remote Sensing 136: 13–25. doi:10.1016/j.isprsjprs.2017.12.004.
- Lovell, J. L., D. L. B. Jupp, D. S. Culvenor, and N. C. Coops. 2003. “Using Airborne and Ground-Based Ranging Lidar to Measure Canopy Structure in Australian Forests.” Canadian Journal of Remote Sensing 29: 607–622. doi:10.5589/m03-026.
- Luo, S. Z., J. M. Chen, C. Wang, A. Gonsamo, X. H. Xi, Y. Lin, M. J. Qian, D. L. Peng, S. Nie, and H. M. Qin. 2018. “Comparative Performances of Airborne LiDar Height and Intensity Data for Leaf Area Index Estimation.” IEEE Journal of Selected Topics in Applied Earth Observations & Remote Sensing 11: 300–310. doi:10.1109/JSTARS.2017.2765890.
- Luo, S. Z., C. Wang, F. F. Pan, X. H. Xi, G. C. Li, S. Nie, and S. B. Xia. 2015. “Estimation of Wetland Vegetation Height and Leaf Area Index Using Airborne Laser Scanning Data.” Ecological Indicators 48: 550–559. doi:10.1016/j.ecolind.2014.09.024.
- Luo, S., C. Wang, X. Xi, S. Nie, X. Fan, H. Chen, X. Yang, D. Peng, Y. Lin, and G. Zhou. 2019. “Combining Hyperspectral Imagery and LiDar Pseudo-Waveform for Predicting Crop LAI, Canopy Height and Above-Ground Biomass.” Ecological Indicators 102: 801–812. doi:10.1016/j.ecolind.2019.03.011.
- Nilson, T. 1971. “A Theoretical Analysis of the Frequency of Gaps in Plant Stands.” Agricultural Meteorology 8: 25–38. doi:10.1016/0002-1571(71)90092-6.
- Ni-Meister, W., D. L. B. Jupp, and R. Dubayah. 2001. “Modeling Lidar Waveforms in Heterogeneous and Discrete Canopies.” IEEE Transactions on Geoscience & Remote Sensing 39: 1943–1958. doi:10.1109/36.951085.
- Ni-Meister, W., S. Y. Lee, A. H. Strahler, C. E. Woodcock, C. Schaaf, T. A. Yao, K. J. Ranson, G. Q. Sun, and J. B. Blair. 2010. “Assessing General Relationships Between Aboveground Biomass and Vegetation Structure Parameters for Improved Carbon Estimate from Lidar Remote Sensing.” Journal of Geophysical Research-Biogeosciences 115 (G2): 12. doi:10.1029/2009JG000936.
- Pang, Y., Z. Y. Li, H. B. Ju, H. Lu, W. Jia, L. Si, Y. Guo, et al. 2016. “LiChy: The Caf’s LiDar, CCD and Hyperspectral Integrated Airborne Observation System.” Remote Sensing 8: 16. doi:10.3390/rs8050398.
- Parker, G. G., M. A. Lefsky, and D. J. Harding. 2001. “Light Transmittance in Forest Canopies Determined Using Airborne Laser Altimetry and In-Canopy Quantum Measurements.” Remote Sensing of Environment 76: 298–309. doi:10.1016/S0034-4257(00)00211-X.
- Qi, J., D. Xie, J. Jiang, and H. Huang. 2022. “3D Radiative Transfer Modeling of Structurally Complex Forest Canopies Through a Lightweight Boundary-Based Description of Leaf Clusters.” Remote Sensing of Environment 283. doi:10.1016/j.rse.2022.113301.
- Riano, D., F. Valladares, S. Condes, and E. Chuvieco. 2004. “Estimation of Leaf Area Index and Covered Ground from Airborne Laser Scanner (Lidar) in Two Contrasting Forests.” Agricultural and Forest Meteorology 124: 269–275. doi:10.1016/j.agrformet.2004.02.005.
- Richardson, J. J., L. M. Moskal, and S. H. Kim. 2009. “Modeling Approaches to Estimate Effective Leaf Area Index from Aerial Discrete-Return LIDAR.” Agricultural and Forest Meteorology 149 (6–7): 1152–1160. doi:10.1016/j.agrformet.2009.02.007.
- Roy, D. P., H. B. Kashongwe, and J. Armston. 2021. “The Impact of Geolocation Uncertainty on GEDI Tropical Forest Canopy Height Estimation and Change Monitoring.” Science of Remote Sensing 100024. doi:10.1016/j.srs.2021.100024.
- Ryu, Y., G. Lee, S. Jeon, Y. Song, and H. Kimm. 2014. “Monitoring Multi-Layer Canopy Spring Phenology of Temperate Deciduous and Evergreen Forests Using Low-Cost Spectral Sensors.” Remote Sensing of Environment 149: 227–238. doi:10.1016/j.rse.2014.04.015.
- Sánchez-Azofeifa, G. A., M. Kalácska, M. M. D. Espírito-Santo, G. W. Fernandes, and S. Schnitzer. 2009. “Tropical Dry Forest Succession and the Contribution of Lianas to Wood Area Index (WAI).” Forest Ecology and Management 258 (6): 941–948. doi:10.1016/j.foreco.2008.10.007.
- Shao, G., S. C. Stark, D. R. A. de Almeida, and M. N. Smith. 2019. “Towards High Throughput Assessment of Canopy Dynamics: The Estimation of Leaf Area Structure in Amazonian Forests with Multitemporal Multi-Sensor Airborne Lidar.” Remote Sensing of Environment 221: 1–13. doi:10.1016/j.rse.2018.10.035.
- Solberg, S., A. Brunner, K. H. Hanssen, H. Lange, E. Naesset, M. Rautiainen, and P. Stenberg. 2009. “Mapping LAI in a Norway Spruce Forest Using Airborne Laser Scanning.” Remote Sensing of Environment 113: 2317–2327. doi:10.1016/j.rse.2009.06.010.
- Sumnall, M. J., T. R. Fox, R. H. Wynne, C. Blinn, and V. A. Thomas. 2016. “Estimating Leaf Area Index at Multiple Heights Within the Understorey Component of Loblolly Pine Forests from Airborne Discrete-Return Lidar.” International Journal of Remote Sensing 37: 78–99. doi:10.1080/01431161.2015.1117683.
- Sumnall, M., A. Peduzzi, T. R. Fox, R. H. Wynne, V. A. Thomas, and B. Cook. 2016. “Assessing the Transferability of Statistical Predictive Models for Leaf Area Index Between Two Airborne Discrete Return LiDar Sensor Designs Within Multiple Intensely Managed Loblolly Pine Forest Locations in the South-Eastern USA.” Remote Sensing of Environment 176: 308–319. doi:10.1016/j.rse.2016.02.012.
- Sumnall, M. J., A. Trlica, D. R. Carter, R. L. Cook, M. L. Schulte, O. C. Campoe, R. A. Rubilar, R. H. Wynne, and V. A. Thomas. 2021. “Estimating the Overstory and Understory Vertical Extents and Their Leaf Area Index in Intensively Managed Loblolly Pine (Pinus taeda L.) Plantations Using Airborne Laser Scanning.” Remote Sensing of Environment 254: 112250. doi:10.1016/j.rse.2020.112250.
- Tang, H., and J. Armston. 2020. GEDI ATBD for Footprint Canopy Cover and Vertical Profile Metrics. https://lpdaac.usgs.gov/documents/588/GEDI_FCCVPM_ATBD_v1.0.pdf
- Tang, H., R. Dubayah, M. Brolly, S. Ganguly, and G. Zhang. 2014. “Large-Scale Retrieval of Leaf Area Index and Vertical Foliage Profile from the Spaceborne Waveform Lidar (GLAS/ICESat).” Remote Sensing of Environment 154: 8–18. doi:10.1016/j.rse.2014.08.007.
- Tang, H., R. Dubayah, A. Swatantran, M. Hofton, S. Sheldon, D. B. Clark, and B. Blair. 2012. “Retrieval of Vertical LAI Profiles Over Tropical Rain Forests Using Waveform Lidar at La Selva, Costa Rica.” Remote Sensing of Environment 124: 242–250. doi:10.1016/j.rse.2012.05.005.
- Tang, H., S. Ganguly, G. Zhang, M. A. Hofton, R. F. Nelson, and R. Dubayah. 2016. “Characterizing Leaf Area Index (LAI) and Vertical Foliage Profile (VFP) Over the United States.” Biogeosciences 13: 239–252. doi:10.5194/bg-13-239-2016.
- Tian, Y., R. E. Dickinson, L. Zhou, X. Zeng, Y. Dai, R. B. Myneni, Y. Knyazikhin, et al. 2004. “Comparison of Seasonal and Spatial Variations of Leaf Area Index and Fraction of Absorbed Photosynthetically Active Radiation from Moderate Resolution Imaging Spectroradiometer (MODIS) and Common Land Model.” Journal of Geophysical Research Atmospheres 109 (D1). doi:10.1029/2003JD003777.
- Wang, Y., and H. Fang. 2020. “Estimation of LAI with the LiDar Technology: A Review.” Remote Sensing 12 (20): 3457. doi:10.3390/rs12203457.
- Wang, Y. S., D. R. Miller, J. M. Welles, and G. M. Heisler. 1992. “Spatial Variability of Canopy Foliage in an Oak Forest Estimated with Fisheye Sensors.” Forest Science 38: 854–865.
- Wang, Y., W. Ni, G. Sun, H. Chi, Z. Zhang, and Z. Guo. 2019. “Slope-Adaptive Waveform Metrics of Large Footprint Lidar for Estimation of Forest Aboveground Biomass.” Remote Sensing of Environment 224: 386–400. doi:10.1016/j.rse.2019.02.017.
- Weiss, M., and F. Baret. 2017. CAN-EYE V6.491 User Manual. https://www6.paca.inrae.fr/can-eye/Documentation/Documentation
- Weiss, M., F. Baret, R. B. Myneni, A. Pragnere, and Y. Knyazikhin. 2000. “Investigation of a Model Inversion Technique to Estimate Canopy Biophysical Variables from Spectral and Directional Reflectance Data.” Agronomie 20: 3–22. doi:10.1051/agro:2000105.
- Weiss, M., F. Baret, G. J. Smith, I. Jonckheere, and P. Coppin. 2004. “Review of Methods for in situ Leaf Area Index (LAI) Determination Part II. Estimation of LAI, Errors and Sampling.” Agricultural and Forest Meteorology 121: 37–53. doi:10.1016/j.agrformet.2003.08.001.
- Wei, S., T. Yin, M. A. Dissegna, A. J. Whittle, G. L. F. Ow, M. L. M. Yusof, N. Lauret, and J. -P. Gastellu-Etchegorry. 2020. “An Assessment Study of Three Indirect Methods for Estimating Leaf Area Density and Leaf Area Index of Individual Trees.” Agricultural and Forest Meteorology 292-293: 108101. doi:10.1016/j.agrformet.2020.108101.
- Widlowski, J. -L., C. Mio, M. Disney, J. Adams, I. Andredakis, C. Atzberger, J. Brennan, et al. 2015. “The Fourth Phase of the Radiative Transfer Model Intercomparison (RAMI) Exercise: Actual Canopy Scenarios and Conformity Testing.” Remote Sensing of Environment 169: 418–437. doi:10.1016/j.rse.2015.08.016.
- Yang, P., C. van der Tol, T. Yin, and W. Verhoef. 2020. “The SPART Model: A Soil-Plant-Atmosphere Radiative Transfer Model for Satellite Measurements in the Solar Spectrum.” Remote Sensing of Environment 247: 111870. doi:10.1016/j.rse.2020.111870.
- Yan, G., R. Hu, J. Luo, M. Weiss, H. Jiang, X. Mu, D. Xie, and W. Zhang. 2019. “Review of Indirect Optical Measurements of Leaf Area Index: Recent Advances, Challenges, and Perspectives.” Agricultural and Forest Meteorology 265: 390–411. doi:10.1016/j.agrformet.2018.11.033.
- Yin, T., B. D. Cook, and D. C. Morton. 2022. “Three-Dimensional Estimation of Deciduous Forest Canopy Structure and Leaf Area Using Multi-Directional, Leaf-On and Leaf-Off Airborne Lidar Data.” Agricultural and Forest Meteorology 314: 108781. doi:10.1016/j.agrformet.2021.108781.
- Yin, T., J. Qi, B. D. Cook, D. C. Morton, S. Wei, and J. -P. Gastellu-Etchegorry. 2020. “Modeling Small-Footprint Airborne Lidar-Derived Estimates of Gap Probability and Leaf Area Index.” Remote Sensing 12 (1): 4. doi:10.3390/rs12010004.
- Zeng, W. J., and W. Wang. 2015. “Combination of Nitrogen and Phosphorus Fertilization Enhance Ecosystem Carbon Sequestration in a Nitrogen-Limited Temperate Plantation of Northern China.” Forest Ecology and Management 341: 59–66. doi:10.1016/j.foreco.2015.01.004.
- Zhang, Y. H., H. L. Fang, L. Ma, Y. C. Ye, and Y. Wang. 2020. “Estimation of Forest Leaf Area Index and Clumping Index from the Global Positioning System (GPS) Satellite Carrier-To-Noise-Density Ratio (C/N 0).” Remote Sensing Letters 11 (2): 146–155. doi:10.1080/2150704X.2019.1692386.
- Zhao, K. G., and S. Popescu. 2009. “Lidar-Based Mapping of Leaf Area Index and Its Use for Validating GLOBCARBON Satellite LAI Product in a Temperate Forest of the Southern USA.” Remote Sensing of Environment 113: 1628–1645. doi:10.1016/j.rse.2009.03.006.
- Zheng, G., L. X. Ma, J. U. H. Eitel, W. He, T. S. Magney, L. M. Moskal, and M. S. Li. 2017. “Retrieving Directional Gap Fraction, Extinction Coefficient, and Effective Leaf Area Index by Incorporating Scan Angle Information from Discrete Aerial Lidar Data.” IEEE Transactions on Geoscience & Remote Sensing 55 (1): 577–590. doi:10.1109/TGRS.2016.2611651.
- Zheng, G., and L. M. Moskal. 2009. “Retrieving Leaf Area Index (LAI) Using Remote Sensing: Theories, Methods and Sensors.” Sensors 9: 2719–2745. doi:10.3390/s90402719.
- Zheng, G., and L. M. Moskal. 2012. “Computational-Geometry-Based Retrieval of Effective Leaf Area Index Using Terrestrial Laser Scanning.” IEEE Transactions on Geoscience & Remote Sensing 50: 3958–3969. doi:10.1109/TGRS.2012.2187907.
- Zhu, X., A. K. Skidmore, T. Wang, J. Liu, R. Darvishzadeh, Y. Shi, J. Premier, and M. Heurich. 2018. “Improving Leaf Area Index (LAI) Estimation by Correcting for Clumping and Woody Effects Using Terrestrial Laser Scanning.” Agricultural and Forest Meteorology 263: 276–286. doi:10.1016/j.agrformet.2018.08.026.
Appendix A.
Calculation of the canopy and ground reflectance ratio (ρv/ρg, 1550 nm) from ALS
The vegetation and ground return energies (Rv and Rg) at each footprint are calculated as (Ni-Meister, Jupp, and Dubayah Citation2001):
where is the laser pulse energy and
is the canopy gap fraction.
Set and
, the above equations become:
Assuming constant and
for two adjacent footprints,
can be estimated from the
and
of two adjacent footprints (Ni-Meister et al. Citation2010):
In this study, ten pairs of footprints, each consisting of two adjacent footprints, were randomly selected (red circles in ). shows an example intensity image of three pairs of adjacent footprints (#1, #9 and #10). The return intensities and reflectance ratio (ρv/ρg) for the 10 pairs are shown in .
Figure A1. An example intensity image (in raw digital number) for 3 pairs of adjacent footprints (#1, #9 and #10) selected from . Left: bare ground returns; right: forest returns. The average return intensity of each footprint is shown in the lower right corner.

Table A1. The average return intensities (in raw digital number) for ρv/ρg calculated from 10 pairs of adjacent footprints (). The values of the standard deviations are shown in the brackets.
Appendix B.
Comparison of the DHP, TLS, and GEDI LAI profiles
shows the LAI profiles derived from the TLS and DHP from 1.5 m to the canopy top. Both TLS and DHP profiles are nearly the same, while the TLS LAI are slightly lower than the DHP LAI at <5.5 m. In general, the TLS and DHP LAI profiles are highly correlated (R2 = 0.97 and RMSE = 0.06). shows that the LAI profile derived from GEDI V2 agrees well with the TLS LAI for the layered (R2 = 0.87, RMSE = 0.13) and cumulative (R2 = 0.95, RMSE = 0.16) LAI values, respectively.
Figure B1. (a) The vertical LAI profiles derived from TLS (September 2020) and DHP (September 2021) during the leaf-on season. (b) The scatterplot between the TLS and DHP. The subscript “on” indicates that the data were obtained in the leaf-on season.

Figure B2. Comparison of the layered (a) and cumulative (b) LAI profiles derived from GEDI V2 (August 2020) and TLS (September 2020) during the leaf-on season.

Appendix C.
The 2 m LAI profiles derived from the GEDI waveform data
We derived the 2 m LAI profiles from the GEDI V2 waveform data using the same method as the GEDI standard product (Tang et al. Citation2014, Citation2012). The layered LAI was estimated as (Boucher et al. Citation2020):
where dz is the height interval for LAI calculation, dz = 2. The constants were set the same as in the GEDI standard product: G = 0.5, and ρv/ρg = 1.50.
The resultant LAI profiles show that the layered LAI peaks at 13.5 − 15.5 m in the study area during the different seasons ( and ).
Figure C1. The 2 m LAI profiles derived from the GEDI V2 waveform data during the leaf-on (11 footprints) and leaf-off (23 footprints) seasons.

Table C1. The mean and total LAI values obtained from the 2 m GEDI LAI profiles (Figure C1). The standard deviation values are shown in the brackets.
Appendix D.
Typical field measured canopy and ground reflectance ratio used in the RAdiation transfer Model Intercomparison IV (RAMI-IV)
Table D1. Typical field measured canopy and ground reflectance ratio (ρv/ρg) for different species used in the RAMI-IV during the leaf-off season* (Widlowski et al. Citation2015). The last row shows the mean (standard deviation) of the values.