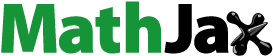
ABSTRACT
Knowledge of terrain impacts on land surface phenology (LSP) is crucial for understanding the responses of mountainous ecosystems to environmental changes. While the effects of terrain factors on LSP spatial patterns have been observed to vary across regions due to their different climate and terrain conditions, the specific effects across different elevations are still largely unclear, especially in regions with diverse hydrothermal conditions, such as the Tianshan Mountains located in arid and semiarid region. Here, we investigated the spatial relationships between LSP metrics and terrain factors (i.e. elevation and aspect) in the Tianshan Mountains in Xinjiang, China. Our analysis utilized surface reflectance at a 30 m spatial resolution from the Harmonized Landsat 8 and Sentinel-2 dataset for 2021 and 2022. We focused on two LSP metrics, vegetation greenup (GU20) and maturity (GU90), which were estimated using 20% and 90% thresholds of seasonal amplitude of the enhanced vegetation index (EVI) time series, respectively. We modeled the spatial relationships using ordinary least square (OLS) linear regression for the entire study region and then applied geographically weighted regression (GWR) with a 2.5 km bandwidth to explore local variations. Our results suggest that, at a large scale, elevation played a primary role in controlling the spatial variations in both LSP metrics, overshadowing the role of aspect. However, when examined at a local scale using GWR, aspect emerged as an important factor, with south-facing aspects associated with earlier dates of GU20 and GU90 for most regions. Furthermore, we found that the influences of terrain on the LSP metrics varied across elevations. The explanatory power of terrain was stronger at middle elevations (approximately 2000–3000 m) than at lower (<2000 m) and higher (>3000 m) elevations. In addition, the sensitivities of the LSP metrics to elevation and aspect demonstrated varying patterns at elevations above 2000 m. Our findings highlight the diverse environmental controls on LSP across elevations, with a particular emphasis on the phenological sensitivities to aspect-induced local climatic differences.
1 Introduction
Mountainous regions are experiencing rapid climate change (Pepin et al. Citation2022). Vegetation phenology has emerged as an essential variable for studying mountain ecosystem functions under the influences of climate change (Hwang et al. Citation2011; Kraus, Zang, and Menzel Citation2016; Vitasse, Signarbieux, and Fu Citation2018). For example, mountainous regions are typically characterized by high biodiversity (Perrigo, Hoorn, and Antonelli Citation2020), and shift in vegetation phenology is a critical factor affecting the suitability of habitats for certain species (Ettinger, Chamberlain, and Wolkovich Citation2022; Viña et al. Citation2016).
In mountainous regions, the large elevation gradient and complex terrain lead to a variety of sunlight, temperature, and moisture combinations, which consequently affect the spatial patterns of vegetation phenology (Adams et al. Citation2021; Dunn and de Beurs Citation2011; Hua, Ohlemüller, and Sirguey Citation2022; An et al. Citation2018). Knowledge of terrain controls (e.g. elevation, aspect, and slope) on vegetation phenology is critical to understanding the responses of vegetation phenology to climate variability and mountainous ecosystem functions (Hwang et al. Citation2011; Vitasse, Signarbieux, and Fu Citation2018). Satellite remote sensing serves as an indispensable tool for observing vegetation phenology in mountainous regions (Dunn and de Beurs Citation2011; Shahgedanova et al. Citation2021). Additionally, earth observation satellites generally measure the seasonality of a landscape or ecosystem rather than individual plants (Guan et al. Citation2014; Reed, Schwartz, and Xiao Citation2009); hence, satellite-derived vegetation phenology is also referred to as land surface phenology (LSP, de Beurs and Henebry Citation2004; Henebry and de Beurs Citation2013).
Numerous studies have investigated the impacts of elevation on the spatial patterns of LSP metrics (e.g. Ding et al. Citation2022; Misra, Asam, and Menzel Citation2021; Shen et al. Citation2014; Thompson and Paull Citation2017). In most cases, spring vegetation greenup is delayed along the elevation due to elevation-induced temperature differences (Shen et al. Citation2014; Vitasse, Signarbieux, and Fu Citation2018). In addition, the influences of elevation on LSP have been found to differ across elevations (Misra, Asam, and Menzel Citation2021). On the other hand, some regions also exhibited negative elevation gradients of spring LSP (Elmore et al. Citation2012; Fisher, Mustard, and Vadeboncoeur Citation2006). For instance, later greenup dates of deciduous forests at lower elevations were observed in a small valley in the USA (Fisher, Mustard, and Vadeboncoeur Citation2006). This phenomenon was attributed to a unique microclimate condition in which cold air drainage results in a cooler valley bottom (Fisher, Mustard, and Vadeboncoeur Citation2006). Moreover, Elmore et al. (Citation2012) reported a conversion of elevation gradients of spring LSP with a conversion point at 275 m. In addition to cold air drainage, Elmore et al. (Citation2012) suggest that the negative gradient below 275 m may be related to winter chilling and atmospheric boundary conditions.
Solar radiation intensity and photoperiod exhibit variations with aspect, which can lead to diverse near surface temperature and soil moisture conditions (Newman et al. Citation2006; Zapata-Rios et al. Citation2016). These aspect-induced local climatic differences reportedly affect LSP patterns in some mountains (e.g. Dunn and de Beurs Citation2011; Hua, Ohlemüller, and Sirguey Citation2022; Hwang et al. Citation2011; An et al. Citation2018). Specifically, spring LSP occurred earlier on south-facing slopes in some regions (Dunn and de Beurs Citation2011; Hwang et al. Citation2011; An et al. Citation2018). Hwang et al. (Citation2011) inferred that disparities in photoperiod may explain the variations in growing season length of forests caused by aspect in North Carolina, USA. Furthermore, different influences of aspect on LSP of subtypes of grasslands at different elevations were observed (Hua, Ohlemüller, and Sirguey Citation2022; An et al. Citation2018). The diverse roles of aspect in shaping LSP patterns are tied to the local environmental conditions (Dunn and de Beurs Citation2011; An et al. Citation2018). Although terrain slope can affect solar geometry and soil moisture (Beven and Kirkby Citation1979; Newman et al. Citation2006), its impacts on LSP spatial patterns were found to be minor for some regions (Adams et al. Citation2021; Dunn and de Beurs Citation2011; Reaves et al. Citation2018). Overall, the effects of aspect and slope on LSP were generally weaker than that of elevation in many studies (e.g. Elmore et al. Citation2012; Hwang et al. Citation2011; Misra et al. Citation2018).
Some uncertainties and problems persist in explaining the impacts of terrain on LSP spatial patterns. Firstly, the presence of mixed pixels, particularly in coarse spatial resolution satellite images, can lead to ambiguous results when modeling the influences of terrain on LSP. For example, many negative elevation gradients of the start of growing season in the Nanling Mountains of southern China were caused by mixed pixels at lower elevations (Ding et al. Citation2022). Secondly, a mountain slope may be represented by several coarse resolution pixels, or even a single pixel in complex terrain regions. This is likely to impede the analysis of the effects of aspect on LSP patterns (Hwang et al. Citation2011). Thirdly, the relative importance of terrain factors in shaping LSP patterns could be linked to the spatial extent of the study area (Elmore et al. Citation2012). Finally, it is essential to characterize how the terrain controls on LSP differ across environmental gradients (Misra et al. Citation2018). Accordingly, a multiscale spatial analysis that uses LSP metrics derived from high or median spatial resolution satellite measurements is needed (Elmore et al. Citation2012; An et al. Citation2018).
Here, we aim to investigate the impacts of terrain factors (elevation and aspect) on the spatial patterns of LSP metrics in the Tianshan Mountains in Xinjiang, China, by employing satellite observations with a median spatial resolution of 30 m. Terrain slope was excluded from this study due to its correlation with elevation over the study area. The Tianshan Mountains show a large environmental gradient with diverse hydrothermal conditions (Hu Citation2004). Hence, terrain controls on LSP patterns are expected to vary with hydrothermal conditions across elevations. To this end, this study seeks to answer two main questions: (1) What are the impacts of elevation and aspect on LSP metrics? (2) How do these impacts vary across elevations?
2 Materials and methods
2.1 Study area
This study was performed in the western of the Tianshan Mountains in Xinjiang, China (). This region encompasses an impressive elevation span exceeding 3500 m (). The region below 2000 m a.s.l. presents a flat topography. However, the landscape transforms dramatically beyond 2000 m, exhibiting a steep elevation gradient (). The region predominantly features western and eastern terrain aspects. Located in a temperate arid and semiarid region, the vegetation is dominated by grasslands. The species composition shows noticeable variations along the elevation gradient (Hu Citation2004). At low-lying elevations, the major vegetation type is temperate desert steppe (Hu Citation2004; Xu et al. Citation2011). As the elevation increases, a transition occurs to mountain steppe, followed by forests, alpine meadows, and ultimately, alpine tundra (Hu Citation2004; Xu et al. Citation2011).
2.2 Datasets
2.2.1 Surface reflectance time series
We used the Harmonized Landsat 8 and Sentinel-2 (HLS) surface reflectance product with a spatial resolution of 30 m generated by NASA (Claverie et al. Citation2018) to estimate LSP metrics. The HLS product has a revisit interval of approximately 2–3 days, a significant improvement over Landsat 8 and Sentinel-2A/2B. This enhancement is due to the integration of available images from all three satellites (Claverie et al. Citation2018). The shortened revisit interval is advantageous for LSP studies that require high frequency observations (Bolton et al. Citation2020; Li and Roy Citation2017; Zhang et al. Citation2020). The HLS product already incorporates atmospheric correction and bidirectional reflectance distribution function (BRDF) reflectance normalization. In addition, the HLS product adjusts the surface reflectance values of Sentinel-2 visible and infrared bands to align with the corresponding bandpass of the Landsat 8 OLI sensor using empirical functions (Claverie et al. Citation2018).
Version 2.0 of the HLS surface reflectance time series product contains two sub-datasets: the HLS L30 (derived from Landsat 8, Masek et al. Citation2021a) and S30 (derived from Sentinel-2A/2B, Masek et al. Citation2021b) data. We collected the HLS data for the years 2021 and 2022 from the Earthdata website (https://search.earthdata.nasa.gov/). It should be noted that the revisit interval of the HLS time series varies across locations. For the majority of pixels within our study area, there are more than 100 observations each year.
2.2.2 Digital elevation model (DEM) data
We obtained the DEM data provided by the NASADEM product (NASA JPL Citation2020) from https://search.earthdata.nasa.gov/search. This product is a refined version of the Shuttle Radar Topography Mission (SRTM) DEM data, delivered at a spatial resolution of 30′ under the WGS 84 geographical coordinate system. We adjusted the projection of the DEM data to match that of the HLS data.
First, we calculated the aspect in degrees, oriented clockwise from north. We then transformed the aspect to a linear format, with values ranging from 0 (corresponding to a northeast slope) to 1 (representing a southwest slope) through Eq. 1 (Neitlich and McCune Citation1997).
where TA represents the transformed aspect, and A represents the original aspect. TA is also referred to as the heat load index since the southwest slope tends to receive stronger solar radiation than other aspects (Neitlich and McCune Citation1997). displays the spatial distribution of TA across the study area. Additionally, the terrain slope is presented in . Notably, a strong correlation exists between slope and elevation in this region. To reduce the effects of multicollinearity, we opted to exclude terrain slope when modeling the spatial relationships between LSP metrics and terrain factors (Hwang et al. Citation2011).
2.2.3 Land cover map
The ESA WorldCover 2021 land cover map V2 with a 10 m spatial resolution (Zanaga et al. Citation2022) was downloaded from Zenodo (https://zenodo.org/record/7254221). This land cover product uses Sentinel-2 and Sentinel-1 time series images as primary inputs. We rescaled the land cover map to a 30 m resolution using a majority resampling rule (). It is noteworthy that forests predominantly exist at mid-range elevations. Here, we focus on herbaceous vegetation that spans a broad elevation spectrum and generally shows gradual changes in species composition along the elevation gradient. Therefore, we confined our LSP analysis to grasslands and moss and lichen.
2.3 Processing of HLS time series data and estimation of LSP metrics
We adopted the enhanced vegetation index (EVI) to estimate LSP metrics due to its advantage of reducing the effects from soil background and residual aerosols (Huete et al. Citation2002). In addition, we calculated the normalized difference snow index (NDSI, Hall, Riggs, and Salomonson Citation1995) to identify snow-affected EVI values. The equations for calculating the EVI and NDSI are denoted as follows.
Here, ,
,
,
, and
represent the surface reflectance values of the spectral bands blue (0.45–0.51 μm), green (0.53–0.59 μm), red (0.64–0.67 μm), near infrared (0.85–0.88 μm), and shortwave infrared (1.57–1.65 μm), respectively.
For each pixel, observations with NDSI > 0.1 during the day of year (DOY) periods 1–151 and 274–365 were considered as snow-affected values (Riggs, Hall, and Román Citation2016). We used the cloud mask provided in the quality assessment (QA) layer of the HLS product and the surface reflectance of the blue band to identify cloudy observations. This operation was taken to minimize the instances of misidentifying clear pixels as clouds, which can occur when solely using the QA layer. The criterion for identifying clouds is shown in Eq. 4.
The threshold of the reflectance of the blue band was set following Oreopoulos et al. (Citation2011). Cloud shadows were identified exclusively using the QA layer. In addition, it is important to note that in this mountainous region, there is a high prevalence of mountain shadows (i.e. dark pixels) in the HLS images, especially during the winter season. These dark pixels are generally associated with abnormal EVI values. We identified dark pixels using the surface reflectance values of the five HLS bands necessary for calculating the EVI and NDSI. Pixels with a surface reflectance value smaller than 0.02 in any of these five bands were regarded as dark pixels, and their corresponding EVI values were subsequently removed from the time series. Snow-affected EVI values were filled by the 5th percentile of the remaining clear EVI values (Gray, Sulla-Menashe, and Friedl Citation2019). Nonetheless, noisy observations may still exist due to the effects of undetected clouds and residual aerosols (Alvarez-Mendoza, Teodoro, and Ramirez-Cando Citation2019; Zhang et al. Citation2020). To mitigate the remaining noises in the EVI time series, we conducted a 3-day maximum value composite (Zhang et al. Citation2020).
We employed the asymmetric Gaussian function fitting in the TIMESAT 3.3 software to further smooth the EVI time series (Eklundh and Jönsson Citation2017; Jönsson and Eklundh Citation2002, Citation2004). The median filter with a spike value of 1.0 was implemented in TIMESAT to eliminate the remaining outliers in the EVI time series. The iteration times of fitting the envelope of the EVI time series were set as 3, with an adaptation strength of 3. We utilized the relative threshold method to estimate LSP metrics. Specifically, the timings of the EVI values corresponding to the 20% and 90% of EVI amplitude plus the minimum EVI value during the vegetation green-up period were identified as GU20 and GU90, respectively (Guan et al. Citation2014; Xie et al. Citation2022). The GU20 metric has been widely regarded as indicating the onset of vegetation greenup in spring in many studies (e.g. O’Connor et al. Citation2012; Xie et al. Citation2022). The GU90 metric represents the onset of maturity, which is close to the timing of peak greenness during the growing season (Gray, Sulla-Menashe, and Friedl Citation2019; Guan et al. Citation2014).
2.4 Statistical analysis
We analyzed the spatial relationships between the two LSP metrics and terrain factors (elevation and TA) at two spatial scales, herein referred to as spatial extents. Ordinary least square (OLS) linear regression between the LSP metrics and terrain factors was performed for the entire study region. We also used geographically weighted regression (GWR), a local form of regression model, to quantify spatially nonstationary local relationships (Brunsdon, Fotheringham, and Charlton Citation1996; Fotheringham, Brunsdon, and Charlton Citation2002). GWR executes a local linear regression for each data point. For the local regression of a target point, an input data point is assigned a weight (W) based on its distance (d) to the target point. Here we used a bisquare kernel function with a bandwidth of 2.5 km (b = 2.5 km) to quantify W (Eq. 5, Fotheringham, Brunsdon, and Charlton Citation2002)
Using this kernel function, only the points located within a 2.5 km buffer of the target point were included in the local regression. Although GWR was performed for the entire region, we used the local regression coefficients and the local determination coefficient (R2) within each spatial window with a radius of 2.5 km. Hence, the GWR outputs for each window can be regarded as local scale results.
We used the boxplot method to eliminate outliers of GU20 and GU90 (Duarte et al. Citation2018). Then, we performed OLS regression and GWR via a spatially random selection of 20% of the pixels (accounting for 709,773 pixels). The regression slopes derived from OLS and GWR can be interpreted as the sensitivities of the LSP metrics to terrain factors.
3 Results
3.1 Spatial patterns of the LSP metrics
The spatial distributions of GU20 and GU90 in 2021 and 2022 are shown in . The spatial patterns of each LSP metric remained relatively consistent between the two years. Nevertheless, considerable spatial variabilities were observed in both LSP metrics. For example, the interquartile range of GU20 in 2021 exceeded 30 days across the region (). The mean dates of GU20 over the region were DOY 124 and 125 in 2021 and 2022, respectively. Moreover, the mean dates of GU90 were delayed by 36 and 35 days in comparison to GU20 in 2021 and 2022, respectively.
3.2 Spatial variations in the LSP metrics modeled by OLS
Across the entire study region, the spatial variations in GU20 were highly influenced by elevation for both years, as indicated by the results of the OLS linear regression (). For example, elevation alone explained 71% of the spatial variability in GU20 in 2021. In contrast, TA exhibited minor effects on the spatial patterns of GU20 at the scale of the entire study region, as evidenced by the very small R2 values of OLS regression ().
Table 1. Statistics of the OLS linear regression models between the two LSP metrics and terrain factors. All OLS regression slopes were statistically significant (p < 0.05). ELEV represents elevation.
The spatial variations in GU90 were also significantly influenced by elevation, rather than TA, across the entire region (). However, the explanatory ability of elevation decreased with vegetation growth, as seen in the considerably lower R2 values of OLS regressions for GU90. For example, elevation explained only 31% of the variation in GU90 in 2022. Scatter plots illustrating the relationships between the LSP metrics and elevation are presented in . We found numerous instances of later GU90 dates at lower elevations (<2000 m) than at elevations of 2000–2500 m (). The spatial belts of later GU90 at lower elevations can also be clearly observed in .
3.3 Spatial variations in the LSP metrics modeled by GWR
Statistics of the local R2 and regression slopes of the GWR models are presented in . Unlike the OLS models, the GWR models exhibited comparable local R2 values between the regression models, with elevation and TA each functioning as a single explanatory variable (). Over 10% of the local R2 values for TA exceed 0.3 for both LSP metrics (), indicating that TA can substantially affect GU20 and GU90 at a local scale (2.5 km radius in this study) in some regions.
Figure 4. Box charts for the GWR model outputs. Local R2 values for the GWR models with (a) elevation, (b) TA, and (c) elevation and TA as explanatory variables, and the local regression slopes of (d) elevation and (e) TA. The gray and dark cyan boxes represent data in 2021 and 2022, respectively. The black square in the box chart indicates the mean value.

Consistent with the OLS models, most regression slopes of elevation were positive for the GWR models (). This suggests that in most local regions, elevation increases can lead to later GU20 and GU90 dates. The regression slopes of TA for GU20 and GU90 were mostly negative, indicating that grasslands on south-facing slopes generally experience greenup and maturity earlier than those on north-facing slopes (). Specifically, more than 10% of the samples in 2021 showed TA regression slopes for GU20 steeper than −10. This result indicates that GU20 can occur over 10 days earlier on the southwest slope than on the northeast slope in some regions. On the other hand, the proportions of positive slopes for TA regarding both LSP metrics were nonnegligible.
The spatial patterns of the local R2 for the GWR models with both elevation and TA as explanatory variables in 2021 are displayed in . The relationships between terrain factors (elevation and TA) and the two LSP metrics (GU20 and GU90) were dependent on elevation. More specifically, stronger correlations (indicated by local R2 values > 0.4) were mostly found at elevations ranging from approximately 2000 m to 3000 m. The fact that these phenomena were consistent in 2022 (as shown in ) provides additional evidence supporting this finding.
Figure 5. Spatial patterns of the local R2 for the GWR models with elevation and TA as explanatory variables in 2021. Subfigures (a) and (b) depict GU20, while (c) and (d) illustrate GU90. The brown circle and error bar in (b) and (d) represent the mean value and one standard deviation of the local R2 values within the 200 m elevation bin, respectively.

In analyzing the sensitivities of the LSP metrics to elevation, as shown through the GWR regression slopes, we found that negative values primarily occurred in regions with lower elevations, specifically those less than 2000 m (). For areas with elevations above 2000 m, a noticeable pattern emerged in the regression slopes of GU90, showing a trend of increasing and then decreasing (resembling a parabolic shape) along with the elevation. The specific elevation bins that exhibited the largest mean regression slopes showed differences between GU20 and GU90.
Figure 6. Spatial patterns of the local regression slopes of elevation for the GWR models with elevation and TA as explanatory variables in 2021. Subfigures (a) and (b) depict GU20, while (c) and (d) illustrate GU90. The brown circle and error bar in (b) and (d) represent the mean value and one standard deviation of the regression slope within the 200 m elevation bin, respectively.

The regression slopes of TA for GU20 and GU90 were also elevation-dependent (). Positive regression slopes of TA were primarily found at lower elevations (<2000 m). The sensitivities to TA demonstrated an increasing trend with elevations between 2000 and 3000 m for both LSP metrics. For areas above 3000 m, the sensitivities of GU90 to TA slightly decreased with elevation. In addition, GU20 typically exhibited stronger sensitivities to TA compared to GU90 for areas above 2000 m.
Figure 7. Spatial patterns of the local regression slopes of TA for the GWR models with elevation and TA as explanatory variables in 2021. Subfigures (a) and (b) depict GU20, while (c) and (d) illustrate GU90. The brown circle and error bar in (c) and (d) represent the mean value and one standard deviation of the regression slope within the 200 m elevation bin, respectively.

4 Discussion
Spatial variations in GU20 and GU90 were observed to be predominantly influenced by elevation across the whole study area. This is in agreement with previous findings that have highlighted elevation as a principal determinant of LSP spatial variations, compared to other terrain factors (Dunn and de Beurs Citation2011; Elmore et al. Citation2012; Hua, Ohlemüller, and Sirguey Citation2022; Hwang et al. Citation2011; Misra et al. Citation2018). Furthermore, the degree to which elevation explained GU90 was considerably less than its influence on GU20 (). This was largely due to a higher incidence of later GU90 dates at lower elevations (<2000 m) as opposed to those observed between 2000 m and 2500 m elevations (). There are two potential explanations for this finding. Firstly, grasslands at lower elevations within this region are generally drier compared to their counterparts at higher elevations (Hu Citation2004). Such water limitation may hinder vegetation growth and thereby lead to later maturity dates (Liu et al. Citation2022). Secondly, human activities, such as livestock grazing and forage planting, may also influence phenological patterns at lower elevations (Han et al. Citation2015; Zhao, Li, and Qi Citation2007). Evidence of human influences can be inferred from the LSP metrics images, specifically from the sharp and erratic phenological transitions ().
With respect to TA, its explanatory powers for GU20 and GU90 were very limited over the whole region, as indicated by the R2 values of the global OLS regression (). In contrast, the local R2 values of elevation and TA from the GWR models with a bandwidth of 2.5 km were close (), which highlights the importance of TA in shaping the spatial patterns of GU20 and GU90 at a local scale. The ability to perform this local analysis in complex terrain was facilitated by the 30 m spatial resolution of the HLS dataset. The GWR results reveal that both GU20 and GU90 generally occur earlier on south-facing slopes (), a pattern that echoes findings from previous studies in the Rocky Mountains in North America (Dunn and de Beurs Citation2011) and the meadow area on the Qinghai-Tibet Plateau (An et al. Citation2018). This impact could be partially attributed to the fact that south-facing slopes, which receive more solar radiation, tend to be warmer than north-facing slopes (An et al. Citation2018).
The influences of terrain on both LSP metrics were found to be elevation dependent (-7). In lower elevation regions, the local regression slopes of elevation and TA showed a heterogeneous mix of both negative and positive values. As mentioned above, water availability and human activities might be the primary drivers of the spatial variations in LSP for lower elevation regions (<2000 m). In addition, the large percentage of positive regression coefficients for TA in lower elevation regions may reflect the impacts of water availability on LSP spatial patterns, as sun-exposed slopes are generally drier than shaded slopes. The mechanisms differ from those reported in some small valleys, where cold air drainage may lead to the varying controls of elevation on spring LSP (Elmore et al. Citation2012; Fisher, Mustard, and Vadeboncoeur Citation2006). It is noteworthy that the sensitivities of both LSP metrics to elevation and TA demonstrated nonlinear changes along elevation for regions above 2000 m. These patterns may stem from two interconnected factors. Firstly, different responses of vegetation growth to terrain factors may be caused by differences in species composition (Shen et al. Citation2022; Xie et al. Citation2015) and soil conditions (Liu et al. Citation2022) across elevations. For example, soil moisture has been reported to regulate the response of spring phenology to air temperature in grasslands (Liu et al. Citation2022). Secondly, the changing impacts of terrain on climate conditions, such as local temperature and snow cover durations, across elevations may also partially explain the varying sensitivities (Misra, Asam, and Menzel Citation2021; Shen et al. Citation2016; Tomaszewska, Nguyen, and Henebry Citation2020; Xie et al. Citation2021). These findings suggest that LSP at the highest elevations may not be the most sensitive to terrain-induced climatic differences. Further studies are required to elucidate the underlying mechanisms driving the varying responses of LSP to terrain across elevations.
5 Conclusions
In this study, we investigated the impacts of elevation and TA on the spatial patterns of two LSP metrics during the vegetation green-up period (GU20 and GU90) in the Tianshan Mountains, China using the HLS dataset with a 30 m spatial resolution. The results showed that the impacts of elevation and TA on the spatial variations in GU20 and GU90 were dependent on the spatial scale of analysis. At the scale of the entire study area, spatial variations in both LSP metrics were primarily controlled by elevation. However, when the GWR model with a 2.5 km bandwidth was applied, TA emerged as a significant factor affecting the two LSP metrics at a more local scale. For most areas, a larger TA was associated with an earlier date of GU20 and GU90. Additionally, the effects of terrain on GU20 and GU90 varied across elevations. Stronger explanatory powers of elevation and TA were observed at middle elevations (approximately 2000–3000 m) than at lower and higher elevations. The sensitivities of GU20 and GU90 to elevation and TA exhibited nonlinear but different change patterns along elevation. Our results highlight the diverse responses of the LSP metrics to terrain-induced local climatic differences in the arid and semiarid Tianshan Mountains. Specifically, LSP at the highest elevations may not be most sensitive to terrain-induced climatic differences.
Acknowledgments
We are grateful to the anonymous reviewers for their constructive comments.
Disclosure statement
No potential conflict of interest was reported by the author(s).
Data availability statement
The data that support the findings of this study were obtained from publicly available datasets, and the websites providing access to these datasets are included in the article.
Additional information
Funding
References
- Adams, B. T., S. N. Matthews, L. R. Iverson, A. M. Prasad, M. P. Peters, and K. Zhao. 2021. “Spring Phenological Variability Promoted by Topography and Vegetation Assembly Processes in a Temperate Forest Landscape.” Agricultural and Forest Meteorology 308–309:108578. https://doi.org/10.1016/j.agrformet.2021.108578.
- Alvarez-Mendoza, C. I., A. Teodoro, and L. Ramirez-Cando. 2019. “Improving NDVI by Removing Cirrus Clouds with Optical Remote Sensing Data from Landsat-8 – a Case Study in Quito, Ecuador.” Remote Sensing Applications: Society & Environment 13:257–17. https://doi.org/10.1016/j.rsase.2018.11.008.
- An, S., X. Zhang, X. Chen, D. Yan, and G. M. Henebry. 2018. “An Exploration of Terrain Effects on Land Surface Phenology Across the Qinghai–Tibet Plateau Using Landsat ETM+ and OLI Data.” Remote Sensing 10 (7): 1069. https://doi.org/10.3390/rs10071069.
- Beven, K. J., and M. J. Kirkby. 1979. “A Physically Based, Variable Contributing Area Model of Basin Hydrology / Un Modèle à Base Physique de Zone d’appel Variable de l’hydrologie du Bassin Versant.” Hydrological Sciences Bulletin 24 (1): 43–69. https://doi.org/10.1080/02626667909491834.
- Bolton, D. K., J. M. Gray, E. K. Melaas, M. Moon, L. Eklundh, and M. A. Friedl. 2020. “Continental-Scale Land Surface Phenology from Harmonized Landsat 8 and Sentinel-2 Imagery.” Remote Sensing of Environment 240:111685. https://doi.org/10.1016/j.rse.2020.111685.
- Brunsdon, C., A. S. Fotheringham, and M. E. Charlton. 1996. “Geographically Weighted Regression: A Method for Exploring Spatial Nonstationarity.” Geographical Analysis 28 (4): 281–298. https://doi.org/10.4135/9781412939591.n478.
- Claverie, M., J. Ju, J. G. Masek, J. L. Dungan, E. F. Vermote, J. C. Roger, S. V. Skakun, and C. Justice. 2018. “The Harmonized Landsat and Sentinel-2 Surface Reflectance Data Set.” Remote Sensing of Environment 219:145–161. https://doi.org/10.1016/j.rse.2018.09.002.
- de Beurs, K. M., and G. M. Henebry. 2004. “Land Surface Phenology, Climatic Variation, and Institutional Change: Analyzing Agricultural Land Cover Change in Kazakhstan.” Remote Sensing of Environment 89 (4): 497–509. https://doi.org/10.1016/j.rse.2003.11.006.
- Ding, C., W. Huang, M. Liu, and S. Zhao. 2022. “Change in the Elevational Pattern of Vegetation Greenup Date Across the Tianshan Mountains in Central Asia During 2001 – 2020.” Ecological Indicators 136:108684. https://doi.org/10.1016/j.ecolind.2022.108684.
- Ding, C., W. Huang, Y. Meng, and B. Zhang. 2022. “Satellite-Observed Spatio-Temporal Variation in Spring Leaf Phenology of Subtropical Forests Across the Nanling Mountains in Southern China Over 1999–2019.” Forests 13 (9): 1486. https://doi.org/10.3390/f13091486.
- Duarte, L., A. C. Teodoro, A. T. Monteiro, M. Cunha, and H. Gonçalves. 2018. “QPhenoMetrics: An Open Source Software Application to Assess Vegetation Phenology Metrics.” Computers and Electronics in Agriculture 148:82–94. https://doi.org/10.1016/j.compag.2018.03.007.
- Dunn, A., and K. M. de Beurs. 2011. “Land Surface Phenology of North American Mountain Environments Using Moderate Resolution Imaging Spectroradiometer Data.” Remote Sensing of Environment 115 (5): 1220–1233. https://doi.org/10.1016/j.rse.2011.01.005.
- Eklundh, L., and P. Jönsson, 2017. Timesat 3.3 Software Manual. Lund and Malmö University, Sweden. https://web.nateko.lu.se/timesat/timesat.asp?cat=6.
- Elmore, A. J., S. M. Guinn, B. J. Minsley, and A. D. Richardson. 2012. “Landscape Controls on the Timing of Spring, Autumn, and Growing Season Length in Mid-Atlantic Forests.” Global Change Biology 18 (2): 656–674. https://doi.org/10.1111/j.1365-2486.2011.02521.x.
- Ettinger, A. K., C. J. Chamberlain, and E. M. Wolkovich. 2022. “The Increasing Relevance of Phenology to Conservation.” Nature Climate Change 12 (4): 305–307. https://doi.org/10.1038/s41558-022-01330-8.
- Fisher, J. I., J. F. Mustard, and M. A. Vadeboncoeur. 2006. “Green Leaf Phenology at Landsat Resolution: Scaling from the Field to the Satellite.” Remote Sensing of Environment 100 (2): 265–279. https://doi.org/10.1016/j.rse.2005.10.022.
- Fotheringham, A. S., C. Brunsdon, and M. Charlton. 2002. Geographically Weighted Regression: the Analysis of Spatially Varying Relationships. Chichester, UK: JOHN WILEY & SONS.
- Gray, J., D. Sulla-Menashe, and M. A. Friedl, 2019. User Guide to Collection 6 MODIS Land Cover Dynamics (MCD12Q2) Product 6. https://landweb.modaps.eosdis.nasa.gov/QA_WWW/forPage/user_guide/MCD12Q2_Collection6_UserGuide.pdf.
- Guan, K., E. F. Wood, D. Medvigy, J. Kimball, M. Pan, K. K. Caylor, J. Sheffield, X. Xu, and M. O. Jones. 2014. “Terrestrial Hydrological Controls on Land Surface Phenology of African Savannas and Woodlands.” Journal of Geophysical Research: Biogeosciences 119 (8): 1652–1669. https://doi.org/10.1002/2013JG002572.Received.
- Hall, D. K., G. A. Riggs, and V. V. Salomonson. 1995. “Mapping Global Snow Cover Using Moderate Resolution Imaging Spectroradiometer (MODIS) Data.” Remote Sensing of Environment 54 (2): 127–140. https://doi.org/10.1016/0034-4257(95)00137-P.
- Han, J., J. Chen, J. Xia, and L. Li. 2015. “Grazing and Watering Alter Plant Phenological Processes in a Desert Steppe Community.” Plant Ecology 216 (4): 599–613. https://doi.org/10.1007/s11258-015-0462-z.
- Henebry, G. M., and K. M. de Beurs. 2013. “Remote Sensing of Land Surface Phenology: A Prospectus.” In Phenology: An Integrative Environmental Science, edited by M. Schwartz, 385–411. Dordrecht, The Netherlands: Springer. https://doi.org/10.1007/978-94-007-6925-0_21.
- Hu, R. G. 2004. Physical Geography of the Tianshan Mountains in China. Beijing: China Environmental Science Press.
- Hua, X., R. Ohlemüller, and P. Sirguey. 2022. “Differential Effects of Topography on the Timing of the Growing Season in Mountainous Grassland Ecosystems.” Environmental Advances 8:100234. https://doi.org/10.1016/j.envadv.2022.100234.
- Huete, A., K. Didan, T. Miura, E. P. P. Rodriguez, X. Gao, and L. G. G. Ferreira. 2002. “Overview of the Radiometric and Biophysical Performance of the MODIS Vegetation Indices.” Remote Sensing of Environment 83 (1–2): 195–213. https://doi.org/10.1016/S0034-4257(02)00096-2.
- Hwang, T., C. Song, J. M. Vose, and L. E. Band. 2011. “Topography-Mediated Controls on Local Vegetation Phenology Estimated from MODIS Vegetation Index.” Landscape Ecology 26 (4): 541–556. https://doi.org/10.1007/s10980-011-9580-8.
- Jönsson, P., and L. Eklundh. 2002. “Seasonality Extraction by Function Fitting to Time-Series of Satellite Sensor Data.” IEEE Transactions on Geoscience and Remote Sensing 40 (8): 1824–1832. https://doi.org/10.1109/TGRS.2002.802519.
- Jönsson, P., and L. Eklundh. 2004. “TIMESAT—A Program for Analyzing Time-Series of Satellite Sensor Data.” Computers & Geosciences 30 (8): 833–845. https://doi.org/10.1016/j.cageo.2004.05.006.
- Kraus, C., C. Zang, and A. Menzel. 2016. “Elevational Response in Leaf and Xylem Phenology Reveals Different Prolongation of Growing Period of Common Beech and Norway Spruce Under Warming Conditions in the Bavarian Alps.” European Journal of Forest Research 135 (6): 1011–1023. https://doi.org/10.1007/s10342-016-0990-7.
- Li, J., and D. P. Roy. 2017. “A Global Analysis of Sentinel-2a, Sentinel-2b and Landsat-8 Data Revisit Intervals and Implications for Terrestrial Monitoring.” Remote Sensing 9 (9): 902. https://doi.org/10.3390/rs9090902.
- Liu, Z., Y. H. Fu, X. Shi, T. R. Lock, R. L. Kallenbach, and Z. Yuan. 2022. “Soil Moisture Determines the Effects of Climate Warming on Spring Phenology in Grasslands.” Agricultural and Forest Meteorology 323:109039. https://doi.org/10.1016/j.agrformet.2022.109039.
- Masek, J., J. Ju, J. Roger, S. Skakun, E. Vermote, M. Claverie, J. Dungan, Z. Yin, B. Freitag, and C. Justice. 2021a. HLS Operational Land Imager Surface Reflectance and TOA Brightness Daily Global 30m V2.0 [Data Set]. NASA EOSDIS Land Processes DAAC. https://doi.org/10.5067/HLS/HLSL30.002.
- Masek, J., J. Ju, J. Roger, S. Skakun, E. Vermote, M. Claverie, J. Dungan, Z. Yin, B. Freitag, and C. Justice. 2021b. HLS Sentinel-2 Multi-Spectral Instrument Surface Reflectance Daily Global 30m V2.0 [Data Set]. NASA EOSDIS Land Processes DAAC. https://doi.org/10.5067/HLS/HLSS30.002.
- Misra, G., S. Asam, and A. Menzel. 2021. “Ground and Satellite Phenology in Alpine Forests are Becoming More Heterogeneous Across Higher Elevations with Warming.” Agricultural and Forest Meteorology 303:108383. https://doi.org/10.1016/j.agrformet.2021.108383.
- Misra, G., A. Buras, M. Heurich, S. Asam, and A. Menzel. 2018. “LiDar Derived Topography and Forest Stand Characteristics Largely Explain the Spatial Variability Observed in MODIS Land Surface Phenology.” Remote Sensing of Environment 218:231–244. https://doi.org/10.1016/j.rse.2018.09.027.
- NASA JPL.2020. NASADEM Merged DEM Global 1 Arc Second V001 [Data Set]. NASA EOSDIS Land Processes DAAC. https://doi.org/10.5067/MEaSUREs/NASADEM/NASADEM_HGT.001.
- Neitlich, P. N., and B. McCune. 1997. “Hotspots of Epiphytic Lichen Diversity in Two Young Managed Forests.” Conservation Biology 11 (1): 172–182. https://doi.org/10.1046/j.1523-1739.1997.95492.x.
- Newman, B. D., B. P. Wilcox, S. R. Archer, D. D. Breshears, C. N. Dahm, C. J. Duffy, N. G. McDowell, F. M. Phillips, B. R. Scanlon, and E. R. Vivoni. 2006. “Ecohydrology of Water-Limited Environments: A Scientific Vision.” Water Resources Research 42 (6): W06302. https://doi.org/10.1029/2005WR004141.
- O’Connor, B., E. Dwyer, F. Cawkwell, and L. Eklundh. 2012. “Spatio-Temporal Patterns in Vegetation Start of Season Across the Island of Ireland Using the MERIS Global Vegetation Index.” ISPRS Journal of Photogrammetry & Remote Sensing 68:79–94. https://doi.org/10.1016/j.isprsjprs.2012.01.004.
- Oreopoulos, L., M. J. Wilson, and T. Várnai. 2011. “Implementation on Landsat Data of a Simple Cloud-Mask Algorithm Developed for MODIS Land Bands.” IEEE Geoscience & Remote Sensing Letters 8 (4): 597–601. https://doi.org/10.1109/LGRS.2010.2095409.
- Pepin, N. C., E. Arnone, A. Gobiet, K. Haslinger, S. Kotlarski, C. Notarnicola, E. Palazzi, et al. 2022. “Climate Changes and Their Elevational Patterns in the Mountains of the World.” Reviews of Geophysics 60 (1): e2020RG000730. https://doi.org/10.1029/2020RG000730.
- Perrigo, A., C. Hoorn, and A. Antonelli. 2020. “Why Mountains Matter for Biodiversity.” Journal of Biogeography 47 (2): 315–325. https://doi.org/10.1111/jbi.13731.
- Reaves, V. C., A. J. Elmore, D. M. Nelson, and B. E. McNeil. 2018. “Drivers of Spatial Variability in Greendown within an Oak-Hickory Forest Landscape.” Remote Sensing of Environment 210:422–433. https://doi.org/10.1016/j.rse.2018.03.027.
- Reed, B. C., M. D. Schwartz, and X. Xiao. 2009. “Remote Sensing Phenology.” In Phenology of Ecosystem Processes, edited by A. Noormets, 231–246. New York: Springer New York. https://doi.org/10.1007/978-1-4419-0026-5_10.
- Riggs, G. A., D. K. Hall, and M. O. Román, 2016. MODIS Snow Products Collection 6 User Guide. http://modis-snow-ice.gsfc.nasa.gov/uploads/C6_MODIS_Snow_User_Guide. pdf.
- Shahgedanova, M., C. Adler, A. Gebrekirstos, H. R. Grau, C. Huggel, R. Marchant, N. Pepin, V. Vanacker, D. Viviroli, and M. Vuille. 2021. “Mountain Observatories: Status and Prospects for Enhancing and Connecting a Global Community.” Mountain Research and Development 41 (2): A1–A15. https://doi.org/10.1659/MRD-JOURNAL-D-20-00054.1.
- Shen, Y.-J., Y. J. Shen, J. Goetz, and A. Brenning. 2016. “Spatial-Temporal Variation of Near-Surface Temperature Lapse Rates Over the Tianshan Mountains, Central Asia.” Journal of Geophysical Research 121 (14): 006–14,017. https://doi.org/10.1002/2016JD025711.
- Shen, M., S. Wang, N. Jiang, J. Sun, R. Cao, X. Ling, B. Fang, et al. 2022. “Plant Phenology Changes and Drivers on the Qinghai–Tibetan Plateau.” Nature Reviews Earth & Environment 3 (10): 633–651. https://doi.org/10.1038/s43017-022-00317-5.
- Shen, M., G. Zhang, N. Cong, S. Wang, W. Kong, and S. Piao. 2014. “Increasing Altitudinal Gradient of Spring Vegetation Phenology During the Last Decade on the Qinghai-Tibetan Plateau.” Agricultural and Forest Meteorology 189–190:71–80. https://doi.org/10.1016/j.agrformet.2014.01.003.
- Thompson, J. A., and D. J. Paull. 2017. “Assessing Spatial and Temporal Patterns in Land Surface Phenology for the Australian Alps (2000–2014).” Remote Sensing of Environment 199:1–13. https://doi.org/10.1016/j.rse.2017.06.032.
- Tomaszewska, M. A., L. H. Nguyen, and G. M. Henebry. 2020. “Land Surface Phenology in the Highland Pastures of Montane Central Asia: Interactions with Snow Cover Seasonality and Terrain Characteristics.” Remote Sensing of Environment 240:111675. https://doi.org/10.1016/j.rse.2020.111675.
- Viña, A., W. Liu, S. Zhou, J. Huang, and J. Liu. 2016. “Land Surface Phenology as an Indicator of Biodiversity Patterns.” Ecological Indicators 64:281–288. https://doi.org/10.1016/j.ecolind.2016.01.007.
- Vitasse, Y., C. Signarbieux, and Y. H. Fu. 2018. “Global Warming Leads to More Uniform Spring Phenology Across Elevations.” Proceedings of the National Academy of Sciences of the United States of America 115 (5): 1004–1008. https://doi.org/10.1073/pnas.1717342115.
- Xie, Y., K. F. Ahmed, J. M. Allen, A. M. Wilson, and J. A. Silander. 2015. “Green-Up of Deciduous Forest Communities of Northeastern North America in Response to Climate Variation and Climate Change.” Landscape Ecology 30 (1): 109–123. https://doi.org/10.1007/s10980-014-0099-7.
- Xie, Q., J. Cleverly, C. E. Moore, Y. Ding, C. C. Hall, X. Ma, L. A. Brown, et al. 2022. “Land Surface Phenology Retrievals for Arid and Semi-Arid Ecosystems.” ISPRS Journal of Photogrammetry & Remote Sensing 185:129–145. https://doi.org/10.1016/j.isprsjprs.2022.01.017.
- Xie, J., F. Hüsler, R. de Jong, B. Chimani, S. Asam, Y. Sun, M. E. Schaepman, and M. Kneubühler. 2021. “Spring Temperature and Snow Cover Climatology Drive the Advanced Springtime Phenology (1991–2014) in the European Alps.” Journal of Geophysical Research: Biogeosciences 126 (3): e2020JG006150. https://doi.org/10.1029/2020JG006150.
- Xu, Y., Y. N. Chen, W. Li, A. Fu, X. Ma, D. Gui, and Y. P. Chen. 2011. “Distribution Pattern of Plant Species Diversity in the Mountainous Region of Ili River Valley, Xinjiang.” Environmental Monitoring and Assessment 177 (1–4): 681–694. https://doi.org/10.1007/s10661-010-1665-3.
- Zanaga, D., R. Van De Kerchove, D. Daems, W. De Keersmaecker, C. Brockmann, G. Kirches, J. Wevers, et al. 2022. ESA WorldCover 10 m 2021 v200 https://doi.org/10.5281/zenodo.7254221.
- Zapata-Rios, X., P. D. Brooks, P. A. Troch, J. McIntosh, and Q. Guo. 2016. “Influence of Terrain Aspect on Water Partitioning, Vegetation Structure and Vegetation Greening in High-Elevation Catchments in Northern New Mexico.” Ecohydrology 9 (5): 782–795. https://doi.org/10.1002/eco.1674.
- Zhang, X., J. Wang, G. M. Henebry, and F. Gao. 2020. “Development and Evaluation of a New Algorithm for Detecting 30 M Land Surface Phenology from VIIRS and HLS Time Series.” ISPRS Journal of Photogrammetry & Remote Sensing 161:37–51. https://doi.org/10.1016/j.isprsjprs.2020.01.012.
- Zhao, W. Y., J. L. Li, and J. G. Qi. 2007. “Changes in Vegetation Diversity and Structure in Response to Heavy Grazing Pressure in the Northern Tianshan Mountains, China.” Journal of Arid Environments 68 (3): 465–479. https://doi.org/10.1016/j.jaridenv.2006.06.007.
Appendices
Figure A2. Spatial patterns of the local R2 values for the GWR models with elevation and TA as explanatory variables in 2022. Subfigures (a) and (b) depict GU20, while (c) and (d) illustrate GU90. The brown circle and error bar in (b) and (d) represent the mean value and one standard deviation of the local R2 values within the 200 m elevation bin, respectively.

Figure A3. Spatial patterns of the local regression slopes of elevation for the GWR models with elevation and TA as explanatory variables in 2022. Subfigures (a) and (b) depict GU20, while (c) and (d) illustrate GU90. The brown circle and error bar in (b) and (d) represent the mean value and one standard deviation of the regression slope within the 200 m elevation bin, respectively..

Figure A4. Spatial patterns of the local regression slopes of TA for the GWR models with elevation and TA as explanatory variables in 2022. Subfigures (a) and (b) depict GU20, while (c) and (d) illustrate GU90. The brown circle and error bar in (b) and (d) represent the mean value and one standard deviation of the regression slope within the 200 m elevation bin, respectively..
