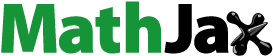
ABSTRACT
Low-carbon and efficient city, a sustainable city development mode, requires the land to carry more people and economy, but cause no ecological damage, which is essentially a trade-off among diverse land functional zones (LFZs), e.g. living, productive, and ecological lands, from a land system perspective. Unfortunately, there is little knowledge about how to trade off LFZs for building low-carbon and efficient cities. To fill the gap, the study first proposes a fine-grained LFZ mapping method to sort the land into seven kinds of LFZs based on multimodal geographic data; then, the study defines carbon efficiency (CE) as a reference to evaluate efficient cities considering their economics, society, and ecology; finally, the study presents a cross lasso regression method to explore how LFZ patterns as well as their temporal changes influence CE, based on which LFZ features are optimized at both national and city levels for improving CE and facilitating efficient cities. The proposed methods are verified in 30 major Chinese cities from 2015 to 2020. The experimental results indicate that the 30 cities have different LFZ patterns in area proportion, morphology, and temporal change, where LFZ proportions are master control factors to CE; and LFZ feature trade-off at national level works better than per city, as it can improve CE by 58.5% compared to that in 2020, which increases population carrying capacity by 88.5% and economy producing capacity by 81.0% with no change in carbon emission, or reduces carbon emission by 55.2% by keeping population and economy constant. These findings directly contribute to low-carbon and efficient city planning, and facilitating sustainable city development.
1. Introduction
The inhabited land can have different functional zones of living, productive, and ecological services (Ellis et al. Citation2019), where living functional zones mainly carry population, productive ones carry economic activities, and ecological ones support the survival and reproduction of species (Duan et al. Citation2021). Sustainable city development requires the land to carry more people and economy, but cause no ecological damage, which is essentially a trade-off among land functional zones (LFZs) from a land system perspective (Cheng et al. Citation2022; Yang, Bao, and Liu Citation2020), where LFZs refer to the lands that have diverse structures with variant functional services and socio-economic activities (Verburg et al. Citation2009). The trade-off among LFZs is an effective way to facilitate low-carbon and efficient cities, because it can improve living environment and productive efficiency without the shift of productivity and energy mix (Schwender et al. Citation2004). Unfortunately, there is a lack of knowledge about how to trade off LFZs for low-carbon and efficient city construction. As indicated by , larger living and productive lands can have larger population and better economics but cause more carbon emission; on the contrary, ecological lands reduce carbon emission but have few contributions to residence and economics; thus, it is very complicated to trade off diverse LFZs to develop population, economy, and ecology simultaneously (Zhou, Xu, and Lin Citation2017).
Figure 1. A quandary: how to trade off living-productive-ecological lands for facilitating low-carbon and efficient cities? Living lands carry population, productive lands carry economic activities, and ecological lands support the survival and reproduction of species, but the total amount of the land is fixed. Three classic development theories, i.e. GDP growth, living planet, and prosperity without growth, are one-sided and contradictory, but the carbon efficiency from an ecological-economics perspective serves as a potential solution.

Inspired by ecological economics researches (Jackson and Victor Citation2016, Citation2019, Citation2020; Tumin, Koryakov, and Nikiforova Citation2013; Victor Citation2012), a comprehensive measure considering economics, ecology, and society is needed. Carbon efficiency can be a potential solution and can serve as a reference to trade off LFZs, because it measures the relationship among population, economics, and carbon emission, and a larger carbon efficiency means that the land can shelter more people, develop more economy, and reduce ecological damage; thus, carbon efficiency can be used as a reference to evaluate the effectiveness of trades-offs among LFZs. Carbon efficiency is derived from energy research field and originally measures effective energy converted from fossil fuel combustion per unit mass (Jae et al. Citation2010; Zhang et al. Citation2022). This study considers carbon efficiency as a ratio of population and economy to carbon emission, which essentially measures population and economic growth per unit mass of carbon emissions. As presented in , LFZs play different and mixed roles in influencing carbon efficiency. For the productive land, it contributes to production and economy, which positively influence carbon efficiency, but it is also the major carbon source and increases carbon emission (Liao et al. Citation2019; Wei et al. Citation2021), which negatively affects carbon efficiency; thus, whether a productive land improves or reduces carbon efficiency is unknown. Similarly, more living land improves population but also increases carbon emission (Wu et al. Citation2021); thus, its contribution to carbon efficiency is also uncertain. In addition, the ecological land reduces carbon emission but limits population and economy development synergistically, which has contradictory impacts on carbon efficiency. As demonstrated above, productive, living, and ecological lands all play mixed roles and have uncertain impacts on carbon efficiency. Furthermore, spatial LFZ patterns can influence carbon efficiency by influencing job-housing balance and productive efficiency (Bellmann and Hübler Citation2020). Accordingly, it is very difficult to explore how LFZ influence carbon efficiency, and it can be even harder to find the best solution for trading off LFZs.
Accordingly, this study aims to explore how LFZs influence carbon efficiency, based on which the study can further trade off LFZs as well as their spatial patterns to improve carbon efficiency. There, however, can be four technical issues to resolve.
How to extract LFZs? Each LFZ contains diverse types of land covers and land uses, and has ambiguous boundaries and abstract categories; thus, LFZs are difficult to extract (Huang, Zhao, and Song Citation2018; Wang Citation2022). Previous studies delineate LFZs based on reclassifications of land covers which, however, were highly heterogeneous and have variant representations in each LFZ, and thus could not generate accurate extraction results for LFZs (Li and Fang Citation2016; Liao et al. Citation2019). In addition, previous studies considered a rough resolution of 30 m for mapping LFZs, which further made LFZ boundaries more ambiguous and category labels more inaccurate (Zhang, Du, and Wang Citation2017).
How to express the spatial LFZ patterns? Diverse LFZ categories with complex spatial relations make it difficult to measure spatial LFZ patterns (Zhang, Du, and Zhang Citation2018). Existing studies focused on measuring intra-LFZ textures and landscape indices (Pacifici, Chini, and Emery Citation2009), but ignored inter-LFZ spatial relations, and could not measure spatial LFZ patterns comprehensively (Pontius, Cornell, and Hall, 2001).
How to measure LFZs’ impacts on carbon efficiency? As demonstrated above, different LFZs as well as their spatial patterns have variant impacts on carbon efficiency, and the influence mechanisms are still unclear. Previous researches employed complex models, e.g. random forest (Zhang et al. Citation2020) and deep neural network (Campos-Taberner et al. Citation2020; Feizizadeh et al. Citation2021) to link land features to environmental indicators. However, these methods above cannot explain whether a LFZ feature have a positive or negative impact on carbon efficiency, and are unable to quantify the impact intensity and knowledge (Deng and Yang Citation2021; Zhang, Du, and Zhang Citation2019).
How to trade off LFZs for improving carbon efficiency? LFZs are mutually binding to each other and have complex impacts on carbon efficiency (Rounsevell et al. Citation2012). The carbon efficiency improvement with complex correlations and substantial restrictions is often non-mathematically solvable, and belong to complicated optimization problems (Deb et al. Citation2002). However, optimization methods have not been applied to LFZ trade-offs, as the objective functions and restrictions for LFZ optimization are unstudied; thus, it is still doubtful whether existing optimizations are effective in trading off LFZs for improving carbon efficiency.
The study will resolve the technical issues above and will make three contributions: it 1) maps LFZs for 30 major Chinese cities from 2015 to 2020 at a fine resolution of 2 m; 2) measures how LFZs influence carbon efficiency by quantifying each LFZ feature’s positive/negative impact; and 3) optimizes LFZ features at both city and national levels to improve carbon efficiency. These contributions are totally novel and play an important role in city planning and sustainable development (UNDP Citation2015).
2. Study area and used data
The study considers 30 major cities in China, having a total area of about 0.5 million km2 and covering all provincial capitals and province-level municipalities of the Chinese mainland (). The 30 cities are representative for three reasons: 1) these cities contain different classes according to Globalization and World Cities (GaWC) 2020 (https://www.lboro.ac.uk/microsites/geography/gawc/world2020t.html); 2) these cities are distributed in different climatic zones and geographical regions; and 3) these cities are regional hubs and have strong influences on the surrounding cities. Accordingly, the study has strong implications for other cities, but it is also challengeable for the study to accurately extract LFZ patterns and measure their impacts on carbon efficiency in these 30 cities.
In addition, the study focuses on a period from 2015 to 2020, because 1) it covers the time period of China’s 13th Five-Year Plan when the 30 cities had a rapid urbanization with their LFZs changed significantly; and 2) the period started from 2015 which was regarded as the first year of sustainable development (UNDP Citation2015). Accordingly, the study considering 2015 ~ 2020 can help evaluate the last Five-Year Plan in China and also the progress of implementing sustainable development goals. Here, the following six datasets are employed to extract LFZ maps and measure carbon efficiencies of the 30 cities in 2015 and 2020.
2.1 Satellite images
Satellite images covering the 30 cities are acquired from GF-1 & 6 in 2015 and 2020. For these images, their multispectral bands are merged with panchromatic band to produce pan-sharpened images at 2-m resolution (Rahmani et al. Citation2010). In addition, these images are ortho-rectified to produce accurate image features for LFZ recognition. Compared to 30-m or even-rougher-resolution images used in previous studies for landscape mapping, the 2-m-resolution images can provide more details for classifying LFZ categories and delineating LFZ boundaries. For LFZ boundary delineation, most LFZs have fuzzy boundaries, and rough-resolution images can exacerbate the problem, because there will be substantial mixed pixels on LFZ boundaries at rough resolutions. While high-resolution satellite images can resolve the fuzzy boundary issue for extracting diverse land uses and functional zones, as they can clearly represent land entities’ edges and reduce mixed pixels. For LFZ category identification, it can be very difficult/even impossible to identify LFZ categories at resolutions rougher than 10 m, because, at those resolutions, visual interpretation cannot even recognize LFZ categories (Campos-Taberner et al. Citation2020). Instead, at the 2-m resolution, land uses can be visually distinguished and extracted from satellite images, which are further fundamental to recognizing LFZ categories. Accordingly, 2-m-resolution satellite images are employed in the study to extract LFZs.
2.2 Buildings
In total, 9.24 million building polygons covering the 30 cities were collected from AutoNavi Map (https://www.amap.com/) in Sep 2015 and Aug 2020. The AutoNavi Map is a widely used navigation software, and thus its building data are updated timely and are accurate in location and boundary. The building data present building footprint and height information for LFZ classification.
2.3 Points of interests (POIs)
14.40 million POIs in the 30 cities were also collected from AutoNavi Map in both 2015 and 2020. As demonstrated above, the AutoNavi Map aims at high-accurate navigation and thus its POI data are reliable. Totally, 20 kinds of POIs are contained in the dataset, including infant, primary, high schools, colleges, entertainments, companies, restaurants, banks, hospitals, clinics, pharmacies, public services, scenic spots, parking lots, hotels, factories, administrative offices, traffic stations, markets, and shops in malls. These POIs represent distributions of socio-economic activities and help recognize LFZ categories.
2.4 Demographic and economic data
Resident population and gross domestic product (GDP) data of each city are fundamental to calculating the city’s carbon efficiency and can be retrieved from the “China City Construction Statistical Yearbook in 2015 and 2020” (https://www.mohurd.gov.cn/gongkai/fdzdgknr/sjfb/tjxx/), where resident population refer to both registered and temporary residents living there for more than six months in a year.
2.5 Energy carbon release data
Energy carbon release is essential for calculating carbon emission and carbon efficiency. Energy carbon release of each city in 2015 and 2020 can be retrieved from the corresponding City Statistical Yearbook (https://data.cnki.net/yearBook/). Generally, the energy carbon release data document living and productive carbon consumption from diverse fossil fuels, e.g. coal, clean coal, coke, coking gas, crude oil, gasoline, kerosene, fuel oil, liquefied petroleum, and natural gas.
2.6 Net ecosystem productivity (NEP) data
NEP refers to net primary productivity minus photosynthetic products consumed by heterotrophic respiration from vegetation and soil microorganisms, and it is important for calculating the absolute carbon emission and carbon efficiency. Global daily NEP analog data with a resolution of 0.07 are used, which are generated by a mechanistic ecological model using vegetation parameter products (i.e. leaf area index, aggregation index, and coverage), meteorological data, and CO2 concentration (He et al. Citation2021). The data are provided by National Ecosystem Science Data Center, National Science & Technology Infrastructure of China (http://www.nesdc.org.cn/). Although the NEP data have a significant scale/resolution gap from the used satellite images, the data are relatively fine to calculate the carbon efficiency of each city.
3. Methodology
This study aims to map fine-grained LFZs, reveal how LFZ features influence carbon efficiency, and optimize LFZ features to facilitate low-carbon and efficient cities. Here, five methods are presented (), including 1) fine-grained LFZ mapping, 2) features for measuring spatial LFZ patterns, 3) carbon efficiency for assessing low-carbon and efficient cities, 4) lasso regression for modeling LFZ patterns’ impacts on the carbon efficiency, and 5) genetic algorithm for trade-offs and optimizations of LFZ features.
3.1. Fine-grained LFZ mapping based on multimodal data
LFZ mapping provides LFZ distribution information and is fundamental to this study. However, LFZs are very difficult to manually delineate or automatically extract from remote-sensing images, especially for large-scale and fine-grained LFZ mapping, because LFZs have great heterogeneity inside and ambiguity on boundary (Verburg et al. Citation2009). Accordingly, this section proposes a novel idea which employs land-use categories as intermediation to bridge the gap from multimodal geographic data to LFZs. The method will be capable of resolving the two issues above, because 1) it can reduce the heterogeneity inside LFZs, as each LFZ is composed of a limited number of land-use categories and patches; and 2) it can reduce the ambiguity on LFZ boundaries, as land uses often have physical enclosures or visible boundaries.
The fine-grained LFZ mapping method can be divided into three parts: i.e. multimodal feature fusion, land-use encoder, and LFZ classification (). For multimodal feature fusion, physical and socio-economic features are extracted from VHR satellite images, buildings, and POIs. In detail, buildings are firstly clustered by considering buildings’ three-dimensional information (i.e. shapes, heights, volumes, spectrums, and textures) and a state-of-the-art clustering approach of measuring local direction centrality is used (Peng et al. Citation2022), which is skilled at sorting buildings with strong heterogeneity and weak connectivity. The spatial and semantic correlations of the clustered buildings and POIs are represented by a Place2Vec model to generate socio-economic feature maps, where Place2Vec measures occurrence probabilities of different buildings and POIs in K-neighbors considering occurrence frequency and distance (Yan et al. Citation2017). Then, the generated socio-economic feature maps are stacked with VHR images and fed into land-use encoder.
For land-use encoder, it contains a ResNet-101 and atrous spatial pyramid pooling method (Chen et al. Citation2018), which integrates land uses’ physical and socio-economic features, and predicts land-use category for each pixel. According to “the classification codes and planning standards of urban and rural land uses” issued by the Chinese Ministry of Housing and Urban-rural Development, 12 categories of land uses are considered, including levels 1, 2, and 3 residential, industrial, commercial, institutional, transport, park, forest, water, farmland, and unused lands. The land-use encoder is trained by land-use samples from OpenStreetMap and manual collections, which are used to predict the probabilities of each pixel belonging to diverse land-use categories. A total number of more than 222,000 patches of land-use samples are collected from OpenStreetMap (OSM) (about 173,000) and manual labeling (about 49,000). These samples contain training, validation, and test one. For test samples, one-tenth of manually collected samples (4850) are employed as test ones, as they are accurately delineated and labeled by experts’ interpretations, which are more reliable compared to OSM samples. They are used to assess the land-use encoding results. Other 217,543 samples from both OSM and manual collection are randomly sorted into two sets, i.e. two-thirds for training and one-third for validation. The validation set is applied to adjusting hyperparameters, and it will be merged with training set to finally train the deep semantic segmentation model for producing land-use encoding results.
For LFZ classification, it uses the land-use probability prediction results above (i.e. the probabilities of each pixel belonging to diverse land-use categories) as input and considers the relationship between land uses and LFZs () to recognize seven LFZ categories, including living, productive, ecological, living-productive, living-ecological, productive-ecological, and no-function. In detail, the method accumulates the probabilities of land uses to measure the classification probabilities of diverse LFZs. For example, we accumulate the probabilities of water, forest, and grass in the land-use prediction results to calculate the classification probability of ecological land. Consequently, the land-use prediction results can be semantically and spatially aggregated into seven LFZ categories. Furthermore, small fragments in the LFZ classification results whose areas are smaller than 0.5 km2 are merged into their neighbors with the longest sharing boundaries (Zhang et al. Citation2020).
Table 1. Land functional zone (LFZ) categories and relationship with land uses.
3.2. Features for measuring spatial LFZ patterns
In order to quantitatively measure spatial LFZ patterns of each city, we propose a feature system, including area proportion, perimeter, shape index, minimum distance, and landscape index (), comprehensively characterizing spatial patterns of LFZs from quantity, geometry, spatial-relation, and landscape-pattern perspectives. For example, area proportion measures the quantities of LFZs; perimeter and shape index characterize geometries of LFZs; minimum distance measures spatial relationship between LFZs; and landscape indices describe LFZ spatial patterns (McGarigal Citation2014). These features have been widely used in characterizing spatial patterns of patches (Zhang et al. Citation2020) and can be totally extracted from the LFZ maps.
Table 2. Features characterizing spatial patterns of land functional zoning (LFZ).
3.3. Carbon efficiency for assessing low-carbon and efficient cities
To assess low-carbon and efficient cities, we propose three indicators, including carbon efficiency for carrying population (), carbon efficiency for producing GDP (
), and the integrated carbon efficiency (
), which can be calculated by EquationEquation 1
(1)
(1) and EquationEquation 3
(3)
(3) .
where refers to the absolute carbon emission of a city and equals carbon release from fossil fuels (
) minus the product of net ecosystem productivity (
) and the city’s
.
is the gross primary productivity of vegetation minus the carbon release from vegetation and soil respiration.
and
denote the resident population and gross domestic product of the city.
and
can be retrieved from the demographic and economic data (Section 2),
can be obtained from statistical yearbooks, and
is derived from the global daily NEP analog data.
As indicated by EquationEquation 1(1)
(1) and EquationEquation 2
(2)
(2) ,
and
essentially measure the population and GDP per mass of carbon emission; thus, larger
and
mean that a city carries larger population and GDP but emits less carbon and further indicates a higher carbon efficiency of the city. Accordingly, large
,
, and
measure a city that is low-carbon and efficient.
3.4. Cross lasso regression for measuring LFZ features’ impacts on carbon efficiency
To model the relationship between LFZ features and carbon efficiency and further measure how LFZs influence carbon efficiency, lasso regression is considered (Tibshirani Citation2011), because lasso regression has three advantages: 1) lasso regression can directly quantify each feature’s impact on carbon efficiency by its regression coefficient; 2) lasso regression resolve the collinearity among features by using a L1-regularization; and 3) lasso regression has a strong generalization capability with acceptable regression errors (Roth Citation2004).
The lasso regression is essentially a linear regression method with a L1-regularization, and it aims to minimize the following cost function and estimate the coefficients of LFZ features :
where denotes the dimension of considered LFZ features (69 in ),
refers to the carbon efficiency of a city and
represents the LFZ features of the city. They are similar with the ordinary linear regression. Specifically, lasso regression considers a L1- regularization of
, i.e.
, which tends to make the weights of colinear features close to 0 and thus resolves the collinearity issue among LFZ features, and
is the weight of the L1-regularization. Aim at minimizing the
in EquationEquation 4
(4)
(4) ,
can be estimated by coordinate descent algorithm (Sergeyev, Famularo, and Pugliese Citation2001).
This study uses lasso regression to measure LFZ features’ impacts on carbon efficiency in 30 cities across two years, i.e. 2015 and 2020, and thus there are samples for training the lasso. However, the number of samples is smaller than that of LFZ features (69 dimensions in ), which can produce uncertain regression results. Furthermore, the 60 samples have strong correlations, for example, a city’s
in 2015 and 2020 can have a correlation, which, however, is not considered in the lasso regression. To resolve the two issues, the section proposes a novel cross lasso regression to increase the sample size and measure the temporal correlation between samples.
The cross lasso regression essentially adds “s and
s temporal changes into the original lasso regression (Eq. 5 ~ 7) and builds 30 additional samples for measuring differences from 2015 to 2020. According to the linear assumption,
, that is,
,
, and thus
. These three linear models can share the same coefficient
, and thus the 30 additional samples calculated from the differences can be used together with the existing 60 samples in regression modelling. Accordingly, a total of
samples are used for training the cross lasso regression, which is not only larger than the number of features but also capable of measuring LFZs temporal changes in each city. The cross lasso regression aims to minimize the cost functions of Eq. 5 ~ 7, and extracts three sets of coefficients
,
, and
which measure LFZ features” impacts on
,
, and
, respectively:
where refers to the change in LFZ features of a city from 2015 to 2020,
,
, and
the changes in three carbon efficiency indicators, i.e.
,
, and
. It should be noticed that
as an important parameter in EquationEquation 5.
(5)
(5) EquationEquation 7
(7)
(7) can influence the estimated coefficients of LFZ features and thus a set of
in increment of ten times are considered in the study (i.e.
= 0.001, 0.01, 0.1, 1, and 10), and average coefficients are used to represent LFZ features’ impacts on carbon efficiency.
In summary, the section proposes a cross lasso regression to measure LFZ features’ impacts on carbon efficiency. Compared to original lasso regression, the cross lasso regression can have a better representation capacity and a higher regression accuracy, because it considers a city’s temporal changes in LFZ feature and carbon efficiency.
3.5. Genetic algorithm for trade-offs and optimization of LFZ features
To optimize LFZs and improve the carbon efficiency of each city, a genetic algorithm (Goldberg and Holland Citation1988) is considered, because LFZ feature trade-off is essentially a complex optimization problem with no mathematical model to solve, and the genetic algorithm is a widely applied metaheuristics which can approximate the global optimal solution with strong robustness and noise resistance (Katoch, Chauhan, and Kumar Citation2021). Accordingly, this study considers the impacts of LFZ features on CE measured by cross lasso regression (Section 3.4) to build the objective function and uses genetic algorithm to estimate the optimal LFZ features of each city.
Genetic algorithm is inspired from biological evolution process, and it finds the optimal solution by features’ crossover, recombination, mutation, and selection based on the fitness function (i.e. objective function). The technical details of genetic algorithm refer to the reference (Hassanat et al. Citation2019). Specifically, four hyperparameters in genetic algorithm for optimizing LFZs are clarified as follows: 1) population represents the number of solutions that are compared and changed in one iteration, and it is set as 200 in the study considering previous researches (Katoch, Chauhan, and Kumar Citation2021); 2) the maximum number of iterations is set as 5000 which exceeds the iteration number used in most researches and guarantees the convergence of optimization results; 3) objective function sets a target of the LFZ feature optimization and considers the carbon efficiency and the cost of LFZ conversions; and 4) restrictions can be represented by a set of equations or inequalities which are detailed in the following sections.
Furthermore, the LFZ feature optimization and trade-offs can be conducted at different levels, i.e. city and national level. At city level, genetic algorithm works per city and optimizes the LFZ features of each city separately, where both objective function and restrictions are imposed on each city (Section 3.5.1); at national level, the 30 cities are considered as a whole, and genetic algorithm is conducted to optimize the LFZ features of these cities synergistically, where objective function and restrictions are imposed on all cities (Section 3.5.2).
3.5.1. Genetic algorithm for LFZ feature optimization at city level
The section conducts genetic algorithm at city level and optimizes LFZ features city by city. It aims to maximize carbon efficiency of each city and minimize the cost of LFZ conversions, thus the objective function is set as follows:
where calculates the carbon efficiency of a city, and
measures the cost by LFZ changes,
refers to the optimized 69 LFZ features () of the city,
denotes the feature coefficients estimated by the cross lasso regression which essentially measures the impacts of LFZ features on carbon efficiency (Section 3.4),
represents the weight of the cost by LFZ changes,
the original
-th LFZ feature in 2020,
that in the optimization result. The
should be subject to the following restrictions:
Eq. 9 is an assumption that the optimized LFZ features cannot exceed the original feature range. Eq. 10 essentially represents a calculation restriction that the accumulated proportions of different LFZs in a city should be 100%. That is, . Similarly, EquationEquation 11
(11)
(11) is another calculation restriction of
. Additionally, some restrictions for specific features are classified:
EquationEquation 12(12)
(12) assumes that the no-function land will not increase. EquationEquation 13
(13)
(13) and EquationEquation 14
(14)
(14) essentially reflect two policy restrictions, i.e. the Ecological Red Line (Bai et al. Citation2018) and the Permanent Basic Cropland (Zahoor et al. Citation2022) that the ecological and productive-ecological (mainly cropland) land cannot be reduced.
3.5.2. Genetic algorithm for LFZ feature optimization at national level
The section conducts genetic algorithm at national level and optimizes LFZ features of the 30 cities synergistically. It aims to maximize carbon efficiency of the 30 cities and minimize the cost of LFZ conversions. Accordingly, the objective function is set as follows:
where calculates the area-weighted average carbon efficiency of the 30 cities,
measures the cost by LFZ conversions in these cities,
refers to 69 LFZ features () of the 30 cities,
contains the coefficients of
, estimated by the cross lasso regression and representing LFZ features’ impacts on carbon efficiency,
represents the
-th city,
, and
is the area of the
-th city.
denotes the
-th feature of the
-th city in 2020,
that in
-th city’s optimization result. The restrictions on
are generally similar to Eq. 9 ~ 12, but other specific restrictions can be different, such as:
where represents the total area of the
-th city. Different from EquationEquation 13
(13)
(13) and EquationEquation 14
(14)
(14) , EquationEquation 16
(16)
(16) and EquationEquation 17
(17)
(17) consider that the ecological and productive-ecological (mainly cropland) lands can be reduced at city level, but not at national level; thus, EquationEquation 16
(16)
(16) and EquationEquation 17
(17)
(17) accumulate the areas of ecological and productive-ecological lands of the 30 cities, which are restricted not to be reduced after the optimization.
3.5.3. Evaluation on the optimization effectiveness
To evaluate the effectiveness of the LFZ feature optimization, three evaluation matrices are proposed, including the improved population and GDP under the current carbon emission, namely, , respectively, and the reduced carbon emission for carrying the current population and GDP, namely,
. The former two, i.e.
and
, aim at assessing how much more population and economy each city can carry caused by the LFZ feature optimization, while keeping the carbon emission constant. The latter one, i.e.
, measures how much carbon emission can be reduced owing to the increased carbon efficiency, while keeping population and GDP constant.
The ImpPOP and ImpGDP of the city can be calculated by Eq. 18 and 19,
where ,
,
,
refer to the original and optimized population and GDP of the city
,
is the city’s carbon emission in 2020,
and
represent the city’s carbon efficiency of population and GDP in 2020.
and
those of the optimized results. Furthermore,
can be measured by Eq. 20:
where represents the optimized carbon emission of the city,
represents the optimized carbon efficiency of the city
caused by its LFZ feature optimization.
4. Experimental result and analysis
4.1. Mapping results of LFZs
The section employs fine-grained LFZ mapping method (Section 3.1) to generate LFZ maps for the 30 cities in 2015 and 2020, where the overall accuracy of land-use classifications is 91.2%, accessed by 4850 patches of test samples. The land-use classification itself has a relatively high accuracy whose errors can have few impacts on LFZ classifications. Supposing that a residential area is misclassified as industrial in the land-use classification, it has 40% probability of being an industrial zone, 35% as a residential area, and 25% as a villa, in the LFZ classification stage, it can be correctly labeled as a living area as it has 35%+25% = 60% probability to be a living land, but only 40% as productive.
The LFZ mapping results of the 30 cities’ urban areas in 2015 and 2020 are presented in . In general, the generated LFZ maps have a high resolution of 2 m and clearly show the distributions of seven kinds of LFZs in each city.
Furthermore, giving a deep insight into the mapping results, six cities scattered across the country and belonging to different classes are selected, and their LFZ maps are shown in . These cities have different LFZ patterns from the following three aspects. First, the LFZ areas and proportions vary among cities. For example, the largest proportion of LFZ in Nanjing ( h & i) is living-ecological land, but that in Xining and Yinchuan ( g~m) are ecological land, while Beijing, Guangzhou, and Chengdu ( b~g) have balanced ecological and living-ecological lands. In addition, living and productive lands in tier 1 and 2 cities are larger than those in tier 3. Second, the spatial distribution and morphology of LFZs are different among cities. For example, LFZs in Beijing, Chengdu, and Nanjing are spread out in a radial pattern, but LFZs in other three cities have significant anisotropy, mainly resulting from their undulating terrains. Third, the temporal changes in LFZ are variant in these cities. For example, many productive-ecological lands in Chengdu and Nanjing are transformed into the living land, but those in other cities are mainly transformed into the productive land. Accordingly, different cities can have different LFZ patterns in proportion, spatial morphology, and temporal change.
We can find different LFZ patterns because the generated LFZ maps have a very high resolution of 2 m and a detailed category system containing seven kinds of LFZs. Therefore, the generated LFZ maps outperforms existing datasets, such as PLEL (Liao et al. Citation2019) with rough administrative units and rough category system, in measuring LFZ patterns, and the generated LFZ maps are freely available on https://geoscape.pku.edu.cn/.
4.2. Calculation results of carbon efficiency
The section uses EquationEquation 1(1)
(1) –EquationEquation 3
(3)
(3) (Section 3.3) to calculate each city’s carbon efficiency for carrying population (
), carbon efficiency for producing GDP (
), and the integrated carbon efficiency (
) in 2015 and 2020 (). Basically, most cities’ carbon efficiency increases from 2015 to 2020. The average
of the 30 cities increased by 39.0% from 0.385 to 0.535 people/ton, the average
increased by 92.2% from 0.231 to 0.445
105 RMB/ton, and
increased by 228.5% from 0.172 to 0.564
105
; thus, the carbon efficiency in the 30 major Chinese cities has been improved significantly. However, there still are eight cities whose
decreased from 2015 to 2020, including Harbin (−0.03
), Jinan (−0.02), Shijiazhuang (−0.003), Urumqi (−0.003), Tianjin (−0.003), Huhhot (−0.002), Yinchuan (−0.001), and these cities are mainly undergoing energy or economic restructuring with small population and economic growths.
Table 3. Carbon efficiency of 30 cities from 2015 to 2020.
In order to reveal the spatial distribution knowledge of cities’ carbon efficiency, we map ,
, and
, as well as their changes in The three indicators themselves in 2015 and 2020 do not have clear spatial distribution pattern, but their temporal changes show a clear north-south difference. Carbon efficiencies decrease or have no growth in most northern cities, but carbon efficiencies increase totally in southern China (), indicating an imbalance between northern and southern Chinese cities in carbon efficiency change, and northern cities lag far behind those in the south.
Furthermore, in order to find the correlation knowledge among these carbon efficiency indicators, we draw the . Generally, we find a significant linear correlation between and
, as well as a linear correlation between
and
( a~c), indicating that if a city has a large carbon efficiency for carrying population, it will have a large carbon efficiency for producing GDP, and if a city has a great change in
, it will have a great change in
simultaneously. In addition, (d~f) indicate that carbon efficiencies (i.e.
,
, and
) in 2015 and 2020 have linear correlation, especially for
which has a large
of 0.91 between 2015 and 2020; thus, temporal changes in carbon efficiency conform to the linear pattern.
4.3. Impacts of LFZ features on carbon efficiency
The section uses cross lasso regression (Section 3.4) to estimate the regression coefficients of LFZ features, measuring impact of each LFZ feature on carbon efficiencies (i.e. ,
, and
). The impacts of diverse LFZ features are quantified and shown in . It should be noticed that the feature coefficients in actually represent the integrated impacts of LFZ features on carbon efficiency. Taking the ecological proportion as an example, a larger ecological proportion can lead to a lower absolute carbon emission, but also a smaller population and a lower GDP, which can reduce the numerator and denominator of CE
(EquationEquation 3
(3)
(3) ) simultaneously, and its positive and negative effects can offset each other; thus, the estimated coefficient of ecological proportion actually represents its integrated impact on CE.
From the feature type perspective (), area proportions of diverse LFZs are the master control factors to carbon efficiency, and they make 46.8%, 62.1%, and 57.5% impacts on ,
, and
, respectively. It is the evidence that LFZ areas and proportions are keys to influencing carbon efficiency. Secondly, the morphologic features including perimeters and shape index also play important roles, where shape index make 33.7%, 16.9%, and 36.2% differences to
,
, and
, respectively, and perimeter make 11.2%, 18.3%, and 3.9% differences. It demonstrates that the LFZ morphology is important to carbon efficiency, as it can influence the internal morphology and structures of LFZs. Thirdly, the minimum distances make 7.5%, 2.3%, and 1.7% impacts on carbon efficiencies, because the minimum distances can influence the interactions among LFZ and further change carbon efficiency. For example, the distance between living and productive areas can affect the job-housing balance and commuting distances, which, in turn, affects productivity and carbon emission. Fourthly, although landscape indices have few impacts on carbon efficiency, their contributions cannot be ignored, because they can make 0.9%, 0.5%, and 0.6% differences to the three carbon efficiency indicators.
From the individual feature perspective ( a), 11 features positively influence carbon efficiency, 16 negatively, but other 3 have both positive and negative impacts on the three carbon efficiency indicators. For the positive factors, their larger values can result in higher carbon efficiency. Among those, ,
, and
are three key positive factors, indicating that increasing ecological land can improve carbon efficiency, and developing living and productive-ecological lands close to square also contribute to carbon efficiency, because square-shaped living spaces can reduce the radius of residents’ movements in their daily lives, and square-shaped productive-ecological lands (mainly farmland) can increase production and harvesting efficiency. Apart from these features, increasing landscape evenness, complexity, and fragmentation can also improve the carbon efficiency, according to the positive impacts of SHEI, PD, and Division (). For negative factors, their larger values can result in lower carbon efficiency. Among those,
,
, and
are three key negative factors, indicating that increasing proportions of mixed-function or no-function lands can affect carbon efficiency. That is, enlarging no function area (e.g. mainly soil) will significantly reduce carbon efficiency; on the other hand, developing living-productive (e.g. institutions) and living-ecological lands (e.g. parks) will occupy the land for other functions, but contribute little to population and GDP, and will reduce carbon efficiency. For other three features who make both positive and negative impacts on carbon efficiency,
,
and
all increase
but reduce
, indicating that increasing bumpiness of LFZ boundaries can contribute to
but negatively affect
.
Figure 10. Correlations between land-functional-zone (LFZ) features on carbon efficiency. a. impacts of LFZ features on carbon efficiency, and the features whose coefficients equal 0 have no impact on carbon efficiency and are abandoned. b~d. different types () of LFZ features’ impacts on carbon efficiency, which are represented by the percentages of absolute impacts.

4.4. Optimization results of LFZ features at city level
The section considers LFZs’ impacts on carbon efficiency and uses genetic algorithm (Section 3.5.1) to optimize LFZ features per city for improving each city’s , where both the objective function (EquationEquation 8
(8)
(8) ) and restrictions (EquationEquation 9
(9)
(9) and EquationEquation 14
(14)
(14) ) are imposed on each city. Furthermore, the
in EquationEquation 8
(8)
(8) , i.e. the weight of the cost by LFZ changes, is set as 0.7, which will be discussed in Section 5.1
Consequently, LFZ feature optimization results in each city can be obtained. We take the Beijing, the capital city, as an example, whose original and optimized LFZ features are presented in . Overall, the changes from the original to the optimized LFZ features generally fit the features’ impacts estimated by cross lasso regression (). For example, ,
,
, and [PD] have positive impacts and are significantly increased by optimization; on the contrary,
,
,
,
, and [PROX] whose impacts are negative will be reduced in the optimization result. However, a special case can be found that the
has a negative impact but increases after optimization, because the optimization considers a policy of Permanent Basic Cropland which is measured by EquationEquation 14
(14)
(14) . Similarly, LFZs of 30 cities can be optimized in the same way, and their optimized LFZ features are averaged and shown in . In general, the optimization results of LFZ features in 30 cities () have similar pattern to Beijing (). Taking LFZ proportions as examples, after optimization, the 30 cities’ proportions of living, productive, ecological, and productive-ecological lands increase by 0.1%, 0.3%, 0.2%, and 1.0%; on the contrary, proportions of living-productive, living-ecological, and no-function lands decrease by 0.2%, 0.3%, and 1.1%, respectively.
Figure 11. Comparisons between original and optimized land-functional-zone (LFZ) features in Beijing. a~d. The proportions, shape index, perimeters, landscape indices of LFZs. e. Distances between different LFZs.

Figure 12. Comparisons between original land-functional-zone (LFZ) features in 2020 and optimized ones. Each feature value is calculated by averaging features of 30 cities. a~d. The proportions, shape index, perimeters, landscape indices of LFZs. e. Distances between different LFZs.

After LFZ feature optimization, carbon efficiency of the 30 cities can be improved (), and the optimization effectiveness can be measured by ,
, and
(Section 3.5.3), which refer to population growth, GDP increase, and carbon emission reduction caused by LFZ feature optimization and carbon efficiency improvement. As presented in , Tianjin has the largest
of 2.3
people. It means that while keeping carbon emission constant, Tianjin can carry such more people after LFZ feature optimization. Taiyuan has the largest
of 1.9
RMB, indicating Taiyuan can produce such more GDP with no change in carbon emission. Taiyuan also has the largest
of 8.4
tons of carbon. It means that while keeping population and GDP constant, Taiyuan can reduce such carbon emission after LFZ feature optimization. Similarly, Chongqing, Huhhot, Shijiazhuang, Wuhan, and Yinchuan can have great improvements in carrying population and producing GDP while keeping their carbon emissions constant, or can reduce carbon emission by keeping their populations and GDP constant, because these cities have low ratios of built-up area to administrative city area (Zhang et al. Citation2022) and they have substantial room for developing.
Table 4. Comparisons between the original and optimized CE in 30 cities, where the unit of CE is 105 RMB/ton2.
To further demonstrate the effectiveness of the optimization, the evaluation indicators, i.e. ,
, and
(EquationEquation 18
(18)
(18) and EquationEquation 20
(20)
(20) ), are calculated for the 30 cities. In 2020, the 30 cities originally carried 313.9 million people, produced a total GDP of 29.4 trillion RMB and released 144.4 trillion tons of CO2; thus, the average carbon efficiency of the 30 cities is 6.4
(EquationEquation 1
(1)
(1) - EquationEquation 3
(3)
(3) ). After LFZ feature optimization, the average carbon efficiency can reach 9.6
(
in EquationEquation 8
(8)
(8) ), improved by 3.2
. Owing to the improvement of carbon efficiency, the 30 cities can carry 582.1 million people and produce a total GDP of 52.5 trillion RMB with no change in carbon emission, or release 66.8 trillion tons of CO2 by keeping population and GDP constant. Accordingly, compared to those in 2020, LFZ feature optimization at city level improves population carrying capacity by (582.1–313.9)/313.9 = 85.4% (EquationEquation 18
(18)
(18) ) and GDP producing capacity by (52.5–29.4)/29.4 = 78.6% (EquationEquation 19
(19)
(19) ), or reduces carbon emission by (144.4–66.8)/144.4 = 53.7% (EquationEquation 20
(20)
(20) ).
4.5. Optimization results of LFZ features at national level
Different from the last section, this section optimizes LFZ features at national level, where the objective function (EquationEquation 16(16)
(16) ) and restrictions (EquationEquation 16
(16)
(16) - EquationEquation 17
(17)
(17) ) are defined at national level and optimize the 30 cities’ LFZ features synergistically.
From the perspective of 30 cities, the national-level results are similar to the city-level (), as their values are close and they have similar relative magnitude to original ones. However, from the perspective of each city, the two levels of optimizations can have different results. Here, we also take the Beijing as an example. As indicated by , in Beijing can be reduced by national-level optimization, but it is improved by city-level optimization, because the two levels of optimizations have different restrictions on the
. For the city level, it demands no decrease in every city’s
. For the national level, it allows a city’s
to decrease, but it requires the
of the total 30 cities not decline. In other words, the national-level optimization has no restriction for city individuals, and it considers using inter-city coordination to meet policy constraints and improve the optimization effectiveness.
Consequently, the LFZ feature optimization at national level can improve carbon efficiency by 3.3 on average. Using the evaluation methods in Section 3.5.3 (EquationEquation 18
(18)
(18) - EquationEquation 20
(20)
(20) ), we find that, compared to those in 2020, LFZ feature optimization improves population carrying capacity by 88.5% and GDP producing capacity by 81.0%, or reduces carbon emission by 55.2%. Compared to city-level LFZ feature optimization, the national-level LFZ optimization brings more ideal results, as it further improves population carrying and GDP producing with no change in carbon emission, or further reduces carbon emission by keeping population and GDP constant; thus, national-level LFZ feature optimization outperforms the city-level.
Figure 14. Comparisons among original land-functional-zone (LFZ) features, optimized LFZ features at city and national levels, where each feature value is calculated by averaging features of 30 cities. a~d. The proportions, shape index, perimeters, landscape indices of LFZs. e. Distances between different LFZs.

5. Discussion
5.1. Impact of cost weight on LFZ feature optimization results
As demonstrated in Section 3.5, LFZ feature optimization essentially aims to maximize carbon efficiency () of each city and minimize the cost by LFZ conversions (
). These two objectives are often contradictory, because more carbon efficiency improved often requires more LFZ conversed. Accordingly, to balance the two objectives, a weight is assigned to the LFZ conversion’s cost, i.e.
in EquationEquation 8
(8)
(8) and EquationEquation 15
(15)
(15) , which essentially reflects the weight of conversion cost in LFZ feature optimization and has a significant impact on optimization results; thus, the section will discuss how the
influence the optimization result.
For easier comparison, we first adjust and
to the same order of magnitude (
), and employ a set of increasing
from 0.1 to 2.0 with an interval of 0.1 (i.e.
) to make LFZ feature optimizations at national level. The changes in optimization result with increasing
are shown in , where optimization results are measured by three indicators, including 1) carbon efficiency
, 2) cost by LFZ conversions
, and 3) their rate, R
, which measures carbon efficiency growth per LFZ conversion cost. As indicated by ,
and
first slowly decline before
, then sharply decline when
, and eventually converge. It is because that, as
increases, LFZ conversions will be limited, and thus the
of LFZ conversions will decrease; on the other hand,
improvement depends on LFZ conversions, and thus fewer LFZ conversions lead to the decrease in
. From the
perspective, it measures the trade-off between
and
. It fluctuates and reaches a peak when
, then falls and eventually levels off. Accordingly, LFZ feature optimization works best when
for both improving
and controlling
.
5.2. Recommendations on delineation of productive-living-ecological lands
This work explores how LFZs influence carbon efficiency (CE), and it attempts to optimize LFZs for facilitating low carbon and efficient cities. Based on the experimental results demonstrated above, the section gives three recommendations on delineation of productive-living-ecological lands.
Firstly, increasing the fragmentation of LFZs contributes to CE improvement. The impacts of two landscape indices on CE are the evidence (). Division () measures the fragmentation of LFZs and has positive impact on CE; on the contrary, Proximity measures the proximity of LFZs and has negative impact. Accordingly, it is can be concluded that increasing the fragmentation of LFZs can promote CE improvement, because broken LFZs can improve the interaction among diverse LFZs (Brinck et al. Citation2017; Schwartz et al. Citation2017); thus, cities should increase LFZ fragmentation by reducing LFZs’ areas and increasing the sharing boundaries of different LFZs. For example, more greenbelts may be planned to increase LFZ fragmentation for improving CE.
Secondly, reducing distances between living and productive, and distances between living and living-ecological lands can improve CE. The distance between living and productive lands is a great concern of job-housing balance (Bellmann and Hübler Citation2020; Huang et al. Citation2019), and the distance between living and living-ecological lands influences living environment quality (Ayala-Azcárraga, Diaz, and Zambrano Citation2019; Jahani and Saffariha Citation2020). Focusing on job-housing balance and increasing greenbelts can help reduce those distances, which can further promote compact and efficient cities by reduce commuting and improve living environments (Bibri, Krogstie, and Kärrholm Citation2020; Hansen et al. Citation2019).
Thirdly, the LFZ feature trade-off at national level outperforms that at city level. It is mainly because that the national-level optimization allows inter-city coordination to obey Ecological Red Line and Permanent Basic Cropland policies, which leaves more room for LFZ conversion in each single city; on the contrary, the city-level optimization is conducted per city, allowing no inter-city coordination and having stricter restrictions on LFZ conversion. Accordingly, national-level optimization cannot only obey policy restrictions but also obtain better carbon efficiency with greater changing room of LFZs (Li et al. Citation2020). In addition to the above theoretical analysis, experimental results in the Section 4.5 also confirm this view that the national-level LFZ feature optimization is more effective than the city-level; thus, LFZ feature optimization should be conducted at national level and consider the coordination among cities.
In summary, in order to improve CE, planners should 1) increase LFZ fragmentation, 2) reduce distances between living and productive land, and distances between living and living-ecological lands, and 3) considers regional coordination among cities and make LFZ feature optimization at national level.
5.3. Pros and cons
This study may contribute to the field knowledge of geographic information and sustainability. For geographic information, the study proposes a fine-grained LFZ mapping method, produces LFZ data at 2-m resolution for 30 cities from 2015 ~ 2020, and reveals how spatial LFZ patterns influence carbon efficiency. Firstly, the fine-grained LFZ mapping method considers land uses as an intermediation to reduce heterogeneity in LFZs and ambiguity on LFZ boundaries, and presents a deep semantic segmentation approach which cannot only integrate both physical and socio-economic features to recognize LFZs but also generate accurate land-use encoding results (the probabilities of each pixel belonging to diverse land-use categories) as fundamental to recognizing diverse LFZs. It is totally different from previous LFZ extraction methods which rely on manually designed productive-living-ecological indicators (e.g. carbon sink, air pollutant purification, residential land price) and substantial field survey data (Li and Fang Citation2016). Secondly, the generated LFZ maps have a finer resolution compared to existing LFZ/urban functional zone/urban functional space data at 30-m or rougher resolution (Liao et al. Citation2019; Song et al. Citation2019; Yang, Bao, and Liu Citation2020), and thus the newly developed LFZ data have not only accurate LFZ categories but also fine extractions of LFZ boundaries. Thirdly, the study is the first work to explore the impacts of spatial LFZ patterns on carbon efficiency, which can fill the knowledge gap and reveal the driving factors of carbon efficiency from the geographic perspective.
For sustainability, the study suggests a LFZ trade-off approach for sustainable city development. The 11th sustainable development goal (SDG 11) raised by the United Nation – sustainable cities and communities focus mainly on calculating indicators for measuring the sustainable development level of each city by considering housing, transport, heritage, disaster, public space, urbanization, and environmental impact (UNDP Citation2015). However, existing SDG 11 studies ignored the trade-off relationship among these indicators which constrain each other and are difficult to improve at the same time (Wu et al. Citation2022); thus, the indicator measurements can solely help evaluate sustainable development levels of cities, but are weak in assisting urban planning and development (Lusseau and Mancini Citation2019). Differently, the study considers trade-offs among diverse LFZs, which takes full account of the competitive relationship between land functions and extracts the optimal LFZ proportions as well as spatial patterns. Accordingly, the study generates a novel idea of trading off LFZs for sustainable development, which is different from previous SDG 11 studies and directly contributes to sustainable urban planning.
Despite the contributions made by the work, it still has some limitations. First, the work optimizes the LFZ features and obtain optimal proportions of LFZs, but does not generate the LFZ optimization maps to represent the spatial allocation of optimized LFZs, because existing spatial allocation methods, e.g. future land-use simulation (Liu, Liu, and Li Citation2017), assigned LFZs according to their proportions but not their spatial patterns, and thus cannot generate LFZ optimization maps according to the optimized LFZ features (Section 3.3). Accordingly, LFZ optimization in the study aims to extract optimal LFZ proportions and spatial pattern features, but the spatial solution of LFZ optimization will be the focus of our future study.
Second, although the 30 study cities are representative, the number of cases is relatively small, which may cause two uncertainties. On the one hand, the selected city sites are mainly located in central and eastern China, with a sparse distribution in western; thus, the conclusion that CEs’ temporal changes show a north-south difference may not be comprehensive. On the other hand, we use 90 samples (30 cities over 2 years and 30 samples additionally produced using the difference between 2015 and 2020) to train the cross lasso regression with 69 input variables (Section 3.4), may have under-training or non-convergence issue for the regression. Accordingly, it will better to consider a larger number and a more balanced distribution of cities for gleaning more reliable spatial patterns of CE and more robust regression model, which however requires more work for extracting fine-grained LFZs in additional cities.
Third, the study considers the carbon efficiency as reference to optimize diverse LFZs, but considers few environmental impacts. Although the study considers the NEP and carbon emission in measuring carbon efficiency, as well as keeps the Ecological Red Line (Bai et al. Citation2018) in LFZ feature optimization, it yet ignores other potential environmental impacts caused by LFZ changes, e.g. soil degradation and urban flooding (Richards and Edwards Citation2018). As suggested by the optimization result, the no-function land that is mainly composed of soil should be transformed into “functional” land, e.g. residential and industrial lands. It can not only decrease soil fertility but also increase urban heat island phenomenon and the risk of urban flooding owing to the enlarged impervious areas. These environmental impacts should be considered while LFZ optimization, which will be an important complementary to the work.
6. Conclusion
The study proposes methods for mapping land functional zones (LFZs), calculating carbon efficiency (CE), modeling their correlations, and optimizing LFZs to improve CE. These methods are applied to 30 major Chinese cities from 2015 to 2020 and draw four conclusions:
First, according to the generated LFZ maps, we find the 30 cities have different LFZ patterns in area proportion, morphology, and temporal change. This finding is special and different from existing studies, as existing LFZ datasets have rough units and rough category system, and they cannot measure and detect variant LFZ patterns within administrative districts. Differently, the generated LFZ maps have a very high resolution of 2 m and a detailed category system containing seven kinds of LFZs. Therefore, the generated LFZ maps outperforms existing datasets in measuring LFZ patterns.
Second, three CE indicators, i.e. ,
, and
, are first defined in the study, and we find that the three indicators do not have clear spatial distribution pattern but their temporal changes show a north-south difference. Carbon efficiency decreases in northern China but increase in southern, indicating an imbalance between northern and southern Chinese cities for improving carbon efficiency, and northern cities fell behind the southern.
Third, LFZ patterns have significant impacts on CE. We find that increasing LFZ fragmentation, reducing distances between living and productive land, and distances between living and living-ecological lands can further improve CE. These are evidences for promoting job-housing balance and compact cities.
Fourth, LFZ feature trade-off and optimization can improve CE by 58.5% from 2020, which improves population carrying capacity by 88.5% and GDP producing capacity by 81.0% with no change in carbon emission, or reduces carbon emission by 55.2% by keeping population and GDP constant. Furthermore, LFZ feature optimization at national level considers inter-city coordination and outperforms that per city. These findings directly contribute to low-carbon and efficient cities, and are important to sustainable city development.
Acknowledgments
The work presented in this paper is funded by the National Key Research and Development Program of China (No. 2022YFF1301102) and the National Natural Science Foundation of China (No. 42001327 and 42271469).
Disclosure statement
The authors declare that they have no known competing financial interests or personal relationships that could have appeared to influence the work reported in this paper.
Data availability statement
Data openly available in a public repository that does not issue DOIs. The data that support the findings of this study are openly available at http://geoscape.pku.edu.cn/en.html.
Additional information
Funding
References
- Ayala-Azcárraga, C., D. Diaz, and L. Zambrano. 2019. “Characteristics of Urban Parks and Their Relation to User Well-Being.” Landscape and Urban Planning 189: 27–28. https://doi.org/10.1016/j.landurbplan.2019.04.005.
- Bai, Y., C. P. Wong, B. Jiang, A. C. Hughes, M. Wang, and Q. Wang. 2018. “Developing China’s Ecological Redline Policy Using Ecosystem Services Assessments for Land Use Planning.” Nature Communications 9 (1): 30–34. https://doi.org/10.1038/s41467-018-05306-1.
- Bellmann, L., and O. Hübler. 2020. “Working from Home, Job Satisfaction and Work–Life Balance–Robust or Heterogeneous Links.” International Journal of Manpower 42 (3): 424–441. https://doi.org/10.1108/IJM-10-2019-0458.
- Bibri, S. E., J. Krogstie, and M. Kärrholm. 2020. “Compact City Planning and Development: Emerging Practices and Strategies for Achieving the Goals of Sustainability.” Developments in the Built Environment 4: 100021. https://doi.org/10.1016/j.dibe.2020.100021.
- Brinck, K., R. Fischer, J. Groeneveld, S. Lehmann, M. Dantas De Paula, S. Pütz, J. Sexton, D. Song, and A. Huth. 2017. “High Resolution Analysis of Tropical Forest Fragmentation and Its Impact on the Global Carbon Cycle.” Nature Communications 8 (1): 1–6. https://doi.org/10.1038/ncomms14855.
- Campos-Taberner, M., F. García-Haro, B. Martínez, E. Izquierdo-Verdiguier, C. Atzberger, G. Camps-Valls, and M. Gilabert. 2020. “Understanding Deep Learning in Land Use Classification Based on Sentinel-2 Time Series.” Scientific Reports 10 (1): 17188. https://doi.org/10.1038/s41598-020-74215-5/.
- Cheng, Z., Y. Zhang, L. Wang, L. Wei, and X. Wu. 2022. “An Analysis of Land-Use Conflict Potential Based on the Perspective of Production–Living–Ecological Function.” Sustainability 14 (10): 1–20. https://doi.org/10.3390/su14105936/.
- Chen, L. C., G. Papandreou, I. Kokkinos, K. Murphy, and A. L. Yuille. 2018. “Deeplab: Semantic Image Segmentation with Deep Convolutional Nets, Atrous Convolution, and Fully Connected CRFs.” IEEE Transactions on Pattern Analysis and Machine Intelligence 40 (4): 834–848. https://doi.org/10.1109/TPAMI.2017.2699184.
- Deb, K., A. Pratap, S. Agarwal, and T. A. M. T. Meyarivan. 2002. “A Fast and Elitist Multiobjective Genetic Algorithm: NSGA-II.” IEEE Transactions on Evolutionary Computation 6 (2): 182–197. https://doi.org/10.1109/4235.996017.
- Deng, Y., and R. Yang. 2021. “Influence Mechanism of Production-Living-Ecological Space Changes in the Urbanization Process of Guangdong Province, China.” Land 11 (6): 1–22. https://doi.org/10.3390/land10121357/.
- Duan, Y., H. Wang, A. Huang, Y. Xu, L. Lu, and Z. Ji. 2021. “Identification and Spatial-Temporal Evolution of Rural “Production-Living-Pcological” Space from the Perspective of Villagers’ Behavior – a Case Study of Ertai Town, Zhangjiakou City.” Land Use Policy 106: 105457. https://doi.org/10.1016/j.landusepol.2021.105457.
- Ellis, E., G. K. Klein, M.-J. Gaillard, J. O. Kaplan, A. Thornton, P. Jeremy, G. Santiago, et al. 2019. “Archaeological Assessment Reveals Earth’s Early Transformation Through Land Use.” Science 365 (6456): 897–902. https://doi.org/10.1126/science.aax1192.
- Feizizadeh, B., K. Mohammadzade Alajujeh, T. Lakes, T. Blaschke, and D. Omarzadeh. 2021. “A Comparison of the Integrated Fuzzy Object-Based Deep Learning Approach and Three Machine Learning Techniques for Land Use/Cover Change Monitoring and Environmental Impacts Assessment.” GIScience & Remote Sensing 58 (8): 1543–1570. https://doi.org/10.1080/15481603.2021.2000350.
- Goldberg, D. E., and J. H. Holland. 1988. “Genetic Algorithms and Machine Learning.” Machine Learning 3 (2): 95–99. https://doi.org/10.1023/A:1022602019183.
- Hansen, R., A. S. Olafsson, A. P. Van Der Jagt, E. Rall, and S. Pauleit. 2019. “Planning Multifunctional Green Infrastructure for Compact Cities: What is the State of Practice?” Ecological Indicators 96: 99–110. https://doi.org/10.1016/j.ecolind.2017.09.042.
- Hassanat, A., K. Almohammadi, E. A. Alkafaween, E. Abunawas, A. Hammouri, and V. S. Prasath. 2019. “Choosing Mutation and Crossover Ratios for Genetic Algorithms—A Review with a New Dynamic Approach.” Information 10 (12): 390. https://doi.org/10.3390/info10120390.
- He, Q., W. Ju, S. Dai, W. He, L. Song, S. Wang, X. Li, and G. Mao. 2021. “Drought Risk of Global Terrestrial Gross Primary Productivity Over the Last 40 Years Detected by a Remote Sensing‐Driven Process Model.” Journal of Geophysical Research: Biogeosciences 126 (6): e2020JG005944. https://doi.org/10.1029/2020JG005944.
- Huang, J., D. Levinson, J. Wang, and H. Jin. 2019. “Job-Worker Spatial Dynamics in Beijing: Insights from Smart Card Data.” Cities 86: 83–93. https://doi.org/10.1016/j.cities.2018.11.021.
- Huang, B., B. Zhao, and Y. Song. 2018. “Urban Land-Use Mapping Using a Deep Convolutional Neural Network with High Spatial Resolution Multispectral Remote Sensing Imagery.” Remote Sensing of Environment 214: 73–86. https://doi.org/10.1016/j.rse.2018.04.050.
- Jackson, T., and P. A. Victor. 2016. “Does Slow Growth Lead to Rising Inequality? Some Theoretical Reflections and Numerical Simulations.” Ecological Economics 121: 206–219. https://doi.org/10.1016/j.ecolecon.2015.03.019.
- Jackson, T., and P. A. Victor. 2019. “Unraveling the Claims for (And Against) Green Growth.” Science 366 (6468): 950–951. https://doi.org/10.1126/science.aay0749.
- Jackson, T., and P. A. Victor. 2020. “The Transition to a Sustainable Prosperity-A Stock-Flow-Consistent Ecological Macroeconomic Model for Canada.” Ecological Economics 177: 106787. https://doi.org/10.1016/j.ecolecon.2020.106787.
- Jae, J., G. A. Tompsett, Y. C. Lin, T. R. Carlson, J. Shen, T. Zhang, and G. W. Huber. 2010. “Depolymerization of Lignocellulosic Biomass to Fuel Precursors: Maximizing Carbon Efficiency by Combining Hydrolysis with Pyrolysis.” Energy & Environmental Science 3 (3): 358–365. https://doi.org/10.1039/b924621p.
- Jahani, A., and M. Saffariha. 2020. “Aesthetic Preference and Mental Restoration Prediction in Urban Parks: An Application of Environmental Modeling Approach.” Urban Forestry & Urban Greening 54: 126775. https://doi.org/10.1016/j.ufug.2020.126775.
- Katoch, S., S. S. Chauhan, and V. Kumar. 2021. “A Review on Genetic Algorithm: Past, Present, and Future.” Multimedia Tools and Applications 80 (5): 8091–8126. https://doi.org/10.1007/s11042-020-10139-6.
- Liao, G., P. He, X. Gao, L. Deng, H. Zhang, N. Feng, W. Zhou, and O. Deng. 2019. “The Production–Living–Ecological Land Classification System and Its Characteristics in the Hilly Area of Sichuan Province, Southwest China Based on Identification of the Main Functions.” Sustainability 11 (6): 1–16. https://doi.org/10.3390/su11061600/.
- Li, G., and C. Fang. 2016. “Quantitative Function Identification and Analysis of Urban Ecological-Production-Living Spaces.” Acta Geographica Sinica 71 (1): 49–65. https://doi.org/10.11821/dlxb201601004.
- Liu, J., Y. Liu, and Y. Li. 2017. “Classification Evaluation and Spatial-Temporal Analysis of “Production-Living-Ecological” Spaces in China.” Acta Geographica Sinica 72 (7): 1290–1304. https://doi.org/10.11821/dlxb201707013.
- Li, W., P. Yi, D. Zhang, and Y. Zhou. 2020. “Assessment of Coordinated Development Between Social Economy and Ecological Environment: Case Study of Resource-Based Cities in Northeastern China.” Sustainable Cities and Society 59: 102208. https://doi.org/10.1016/j.scs.2020.102208.
- Lusseau, D., and F. Mancini. 2019. “Income-Based Variation in Sustainable Development Goal Interaction Networks.” Nature Sustainability 2 (3): 242–247. https://doi.org/10.1038/s41893-019-0231-4.
- McGarigal, K. 2014. “Fragstats V4: Spatial Pattern Analysis Program for Categorical and Continuous Maps-Help Manual.” In University of Massachusetts. Amherst, MA: USA. https://doi.org/10.2737/PNW-GTR-351.
- Pacifici, F., M. Chini, and W. Emery. 2009. “A Neural Network Approach Using Multi-Scale Textural Metrics from Very High-Resolution Panchromatic Imagery for Urban Land-Use Classification.” Remote Sensing of Environment 113 (6): 1276–1292. https://doi.org/10.1016/j.rse.2009.02.014.
- Peng, D., Z. Gui, D. Wang, Y. Ma, Z. Huang, Y. Zhou, and H. Wu. 2022. “Clustering by Measuring Local Direction Centrality for Data with Heterogeneous Density and Weak Connectivity.” Nature Communications 13 (1): 5455. https://doi.org/10.1038/s41467-022-33136-9.
- Rahmani, S., M. Strait, D. Merkurjev, M. Moeller, and T. Wittman. 2010. “An Adaptive IHS Pan-Sharpening Method.” IEEE Geoscience and Remote Sensing Letters 7 (4): 746–750. https://doi.org/10.1109/LGRS.2010.2046715.
- Richards, D. R., and P. J. Edwards. 2018. “Using Water Management Infrastructure to Address Both Flood Risk and the Urban Heat Island.” International Journal of Water Resources Development 34 (4): 490–498. https://doi.org/10.1080/07900627.2017.1357538.
- Roth, V. 2004. “The Generalized LASSO.” IEEE Transactions on Neural Networks 15 (1): 16–28. https://doi.org/10.1109/TNN.2003.809398.
- Rounsevell, M. D., B. Pedroli, K. H. Erb, M. Gramberger, A. G. Busck, H. Haberl, and B. Wolfslehner. 2012. “Challenges for Land System Science.” Land Use Policy 29 (4): 899–910. https://doi.org/10.1016/j.landusepol.2012.01.007.
- Schwartz, N. B., M. Uriarte, R. DeFries, K. M. Bedka, K. Fernandes, V. Gutiérrez‐Vélez, and M. A. Pinedo‐Vasquez. 2017. “Fragmentation Increases Wind Disturbance Impacts on Gorest Structure and Carbon Stocks in a Western Amazonian Landscape.” Ecological Applications 27 (6): 1901–1915. https://doi.org/10.1002/eap.1576.
- Schwender, J., F. Goffman, J. B. Ohlrogge, and Y. Shachar-Hill. 2004. “Rubisco without the Calvin Cycle Improves the Ccarbon Efficiency of Developing Green Seeds.” Nature 432 (7018): 779–782. https://doi.org/10.1038/nature03145.
- Sergeyev, Y. D., D. Famularo, and P. Pugliese. 2001. “Index Branch-And-Bound Algorithm for Lipschitz Univariate Global Optimization with Multiextremal Constraints.” Journal of Global Optimization 21 (3): 317–341. https://doi.org/10.1023/A:1012391611462.
- Song, J., X. Tong, L. Wang, C. Zhao, and A. V. Prishchepov. 2019. “Monitoring Finer-Scale Population Density in Urban Functional Zones: A Remote Sensing Data Fusion Approach.” Landscape and Urban Planning 190: 103580. https://doi.org/10.1016/j.landurbplan.2019.05.011/.
- Tibshirani, R. 2011. “Regression Shrinkage and Selection via the Lasso: A Retrospective.” Journal of the Royal Statistical Society: Series B (Statistical Methodology) 73 (3): 273–282. https://doi.org/10.1111/j.1467-9868.2011.00771.x.
- Tumin, V. M., A. G. Koryakov, and E. P. Nikiforova. 2013. ““The Main Factors of Socio-Ecological-Economic Stability and Development of Industrial enterprises“.” World Applied Sciences Journal 25 (6): 945–949. https://doi.org/10.5829/idosi.wasj.2013.25.06.7066/.
- UNDP (United Nations Development Programme). 2015. “Sustainable Development Goals (SDG).” United Nations Development Programme (UNDP). Accessed February 15, 2023. https://www.undp.org/content/undp/en/home/sustainable-development-goals.html.
- Verburg, P. H., J. Van De Steeg, A. Veldkamp, and L. Willemen. 2009. “From Land Cover Change to Land Function Dynamics: A Major Challenge to Improve Land Characterization.” Journal of Environmental Management 90 (3): 1327–1335. https://doi.org/10.1016/j.jenvman.2008.08.005.
- Victor, P. A. 2012. “Growth, Degrowth and Climate Change: A Scenario Analysis.” Ecological Economics 84: 206–212. https://doi.org/10.1016/j.ecolecon.2011.04.013.
- Wang, Y. 2022. “Spatial–Temporal Evolution of “Production-Living-Ecologica” Function and Layout Optimization Strategy in China: A Case Study of Liaoning Province, China.” Environmental Science and Pollution Research 30 (4): 1–14. https://doi.org/10.1007/s11356-022-22899-9/.
- Wei, L., Y. Zhang, L. Wang, X. Mi, X. Wu, and Z. Cheng. 2021. “Spatiotemporal Evolution Patterns of “Production-Living-Ecological” Spaces and the Coordination Level and Optimization of the Functions in Jilin Province.” Sustainability 13 (23): 1–24. https://doi.org/10.3390/su132313192/.
- Wu, X., B. Fu, S. Wang, S. Song, Y. Li, Z. Xu, Y. Wei, and J. Liu. 2022. “Decoupling of SDGs Followed by Re-Coupling as Sustainable Development Progresses.” Nature Sustainability 5 (5): 452–459. https://doi.org/10.1038/s41893-022-00868-x.
- Wu, J., D. Zhang, H. Wang, and X. Lu. 2021. “What is the Future for Production-Living-Ecological Spaces in the Greater Bay Area? A Multi-Scenario Perspective Based on Dee.” Ecological Indicators 131: 108171. https://doi.org/10.1016/j.ecolind.2021.108171.
- Yang, Y., W. Bao, and Y. Liu. 2020. “Coupling Coordination Analysis of Rural Production-Living-Ecological Space in the Beijing-Tianjin-Hebei Region.” Ecological Indicators 117 (4): 106512. https://doi.org/10.1016/j.ecolind.2020.106512.
- Yan, B., K. Janowicz, G. Mai, and S. Gao. 2017. “From ITDL to Place2Vec – Reasoning About Place Type Similarity and Relatedness by Learning Embeddings from Augmented Spatial Contexts.” In ACM SIGSPATIAL 2017. ACM. https://doi.org/10.1145/3139958.3140054/.
- Zahoor, Z., M. I. Latif, I. Khan, and F. Hou. 2022. “Abundance of Natural Resources and Environmental Sustainability: The Roles of Manufacturing Value-Added, Urbanization, and Permanent Cropland.” Environmental Science and Pollution Research 29 (54): 82365–82378. https://doi.org/10.1007/s11356-022-21545-8.
- Zhang, X., S. Du, S. Du, and B. Liu. 2020. “How Do Land-Use Patterns Influence Residential Environment Quality? A Multiscale Geographic Survey in Beijing.” Remote Sensing of Environment 249: 112014. https://doi.org/10.1016/j.rse.2020.112014.
- Zhang, X., S. Du, H. W. Taubenböck, Y. Du, S. Liu, B. Y. Feng, and Y. Feng. 2022. “Underload City Conceptual Approach Extending Ghost City Studies.” Npj Urban Sustainability 2 (1): 15. https://doi.org/10.1038/s42949-022-00057-x/.
- Zhang, X., S. Du, and Q. Wang. 2017. “Hierarchical Semantic Cognition for Urban Functional Zones with VHR Satellite Images and POI Data.” ISPRS Journal of Photogrammetry and Remote Sensing 132: 170–184. https://doi.org/10.1016/j.isprsjprs.2017.09.007.
- Zhang, X., S. Du, and J. Zhang. 2019. “How Do People Understand Convenience-Of-Living in Cities? A Multiscale Geographic Investigation in Beijing.” ISPRS Journal of Photogrammetry and Remote Sensing 148: 87–102. https://doi.org/10.1016/j.isprsjprs.2018.12.016.
- Zhang, X., S. Du, and Y. Zhang. 2018. “Semantic and Spatial Co-Occurrence Analysis on Object Pairs for Urban Scene Classification.” IEEE Journal of Selected Topics in Applied Earth Observations and Remote Sensing 11 (8): 2630–2643. https://doi.org/10.1109/JSTARS.2018.2854159.
- Zhou, D., J. Xu, and Z. Lin. 2017. “Conflict or Coordination? Assessing Land Use Multi-Functionalization Using Production-Living-Ecology Analysis.” Science of the Total Environment 577: 136–147. https://doi.org/10.1016/j.scitotenv.2016.10.143.