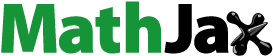
ABSTRACT
Aboveground biomass (AGB) can serve as an indicator when estimating various biogeochemical processes in peatlands, an ecosystem which provides countless ecosystem services and plays a key role in climate regulation. While remote sensing has been extensively employed to assess AGB across vast areas in forested peatlands its application to small and treeless peatlands, which are typical of the alpine regions, has been limited. Due to the characteristics of peatlands, innovative approaches capable of capturing their fine-scale, highly heterogeneous and short-stature vegetation cover are needed. Likewise, other key requirements include an ability to overcome site accessibility barriers, cost-effective acquisition of datasets and minimizing damage of these protected habitats. Hence, the utilization of Unmanned Aerial Vehicles (UAVs) offers a viable means for mapping AGB in alpine peatlands. In this study, the AGB of the Val di Ciampo alpine peatland (Veneto Region, Italy) was estimated by combining datasets derived from in situ vegetation samples as well as UAV-based LiDAR, hyperspectral and RGB sensors. A limited number of vegetation samples were used to reduce the impact of the study on the ecosystem. The results indicate that a linear regression can model the relationship between AGB and Leaf Area Index (LAI) with a significant explanatory ability (R2 = 0.72; p < 0.001). Several indices derived from digital terrain model (DTM) morphologies, hyperspectral data, and orthophotos were tested using a multiple regression approach to determine their potential to enhance the model’s performance. Among these only the Double Difference (DD) index, derived from hyperspectral data, was found to slightly improve the model’s explanatory ability (R2 = 0.76). Overall, the findings of this study suggest that UAV LiDAR data provides the most reliable solution for estimating AGB in alpine peatlands, while the inclusion of hyperspectral data provides only a minor improvement in accuracy.
Introduction
Aboveground biomass (AGB) is a vegetation cover property that is challenging to quantify in a spatially accurate manner since the sparse information derived from in situ point sampling fails to effectively characterize the complex spatial distribution. Remote sensing has been used for decades to estimate biomass spatial patterns (Lu Citation2006). It provides a fast and cost-effective approach to capture large research areas and uniform plant phenology states and ensures a greater level of repeatability compared to field sampling approaches (Czapiewski and Szumińska Citation2021). The primary difficulty in estimating AGB in alpine peatlands stems from their small size and limited accessibility. These sites are often smaller than 1 hectare and feature highly complex and heterogeneous herbaceous vegetation cover (Bragazza Citation1996, Citation2006; Bragazza and Gerdol Citation1999; Bragazza, Rydin, and Gerdol Citation2005; Gerdol and Tomaselli Citation1984; Gerdol, Tomaselli and Bragazza Citation1994). Therefore, even though satellite remote sensing has been widely applied to study peatlands in general (Czapiewski and Szumińska Citation2021), very-high to ultra-high spatial resolution remote sensing datasets should be used in the case of alpine peatlands to avoid erroneous landscape-level estimates of vegetation properties and associated biogeochemical processes (Czapiewski Citation2022; Räsänen et al. Citation2020; Steenvoorden, Bartholomeus, and Limpens Citation2023). Unmanned Aerial Vehicles (UAVs) provide an ideal solution to study alpine peatlands, not just because of the extremely high spatial resolution of the data that they can capture, but even more importantly because of their notable cost-effectiveness when surveying sites which are difficult to access and unevenly distributed across very large territories.
Characterizing and monitoring aboveground vegetation attributes can supply information regarding many peatland processes, at a both superficial and subsurface level (Bragazza and Gerdol Citation1999; Bragazza, Rydin, and Gerdol Citation2005; Tuittila et al. Citation2013; Palozzi and Lindo Citation2017). This ultimately aids the conservation of these vitals (Kimmel and Mander Citation2010) but threatened (Antala et al. Citation2022; Turetsky et al. Citation2015; Ward et al. Citation2007; Maljanen et al. Citation2010) and sparsely mapped (FAO Citation2020; Minasny et al. Citation2019) ecosystems. For instance, according to Räsänen et al. (Citation2020), biomass (i.e. total mass of plants) is one of the most important vegetation properties linked to biogeochemical processes. In particular, AGB in peatlands has been found to be related to the water table depth as well as the below ground biomass (BGB), a key property which is difficult to sample (Murphy, McKinley, and Moore Citation2009). Furthermore, AGB can be used as a proxy to estimate peatland carbon stock (Lopatin et al. Citation2019) and to evaluate the impacts of anthropogenic management approaches (Cabezas et al. Citation2015).
A common method used for estimating AGB from remote sensing data is to derive Vegetation Indices (VIs) based on combinations of spectral bands. The Normalized Difference Vegetation Index (NDVI) is one of the most popular VIs (Huang et al. Citation2021), due to its simplicity and long-standing implementation in this field of research. Studies have demonstrated that NDVI can be effective in estimating AGB both in forest (e.g. Zhu and Liu Citation2015) and herbaceous ecosystems (e.g. Gao et al. Citation2013). In peatlands NDVI has been found to be correlated with aboveground carbon stock (Cabezas et al. Citation2015) as well as AGB (Räsänen et al. Citation2020) and has been incorporated into predicting models and other research topics (e.g. McPartland et al. Citation2019; Pajula and Purre Citation2021; Rastogi et al. Citation2019; Šimanauskienė et al. Citation2019). However, NDVI has some inherent limitations, such as the well-documented saturation effect, and therefore should be used with caution (Huang et al. Citation2021; Huete, Liu, and van Leeuwen Citation1997); this is especially true in densely vegetated herbaceous alpine ecosystems, where the saturation effect is present even at low values of biomass (Vescovo and Gianelle Citation2008). Limitations in the use of NDVI to model AGB in northern herbaceous peatlands and similar systems have been demonstrated by several authors (Bratsch et al. Citation2017; Cunliffe et al. Citation2020; Räsänen et al. Citation2019, Citation2021). Similar limitations for NDVI indices retrieved using hyperspectral data have been shown in Räsänen et al. Citation2020), which tests 80 hyperspectral vegetation indices using random forests regressions to identify those with the greatest ability to estimate AGB in a northern peatland. It was found that NDVI scored lower than several other analyzed indices, for instance the Double Difference (DD) index (le Maire, François, and Dufrêne, Citation2004), the Simple Ratio SR1 (Gitelson and Merzlyak Citation1997), the Simple Ratio SR6 (Zarco-Tejada and Miller Citation1999), Boochs2 (Boochs et al. Citation1990), MCARI2 (Wu et al. Citation2008), OSAVI2 (Wu et al. Citation2008), and MCARI2/OSAVI2 (Wu et al. Citation2008).
Another well-known proxy of AGB is the Leaf Area Index (LAI), which is defined as the ratio of the one-sided leaf surface area per unit ground surface area. LAI is a vegetation parameter that characterizes leaf density and canopy structure, hence it is strongly related to various biogeochemical parameters (Tang et al. Citation2022), including biomass (e.g. Aase Citation1978; Crabbe et al. Citation2019; Räsänen et al. Citation2020). Field LAI measurements can be accurate, but are labor-intensive, time-consuming, expensive, and do not allow spatial repeatability over large areas. Therefore, estimating LAI via remote sensing appears to be a comparably appealing operational choice (Bajocco et al. Citation2022). One of the commonly used approaches is to measure LAI with LiDAR sensors, which actively probe the canopy with narrow laser beams (Wang and Fang Citation2020; Zheng and Moskal Citation2009). The applied methods depend on the type of LiDAR sensor (Tian, Qu, and Qi Citation2021), but one of the most common types is gap-fraction. This uses a theoretical framework, built on the probability of a laser beam penetrating the canopy and reaching the ground, to infer the LAI of the vegetation cover (Houldcroft et al. Citation2005; Richardson, Moskal, and Kim Citation2009). This is similar to the approach often taken with passive field devices. An advantage of LiDAR is that it allows simultaneous extrapolation of both vegetation and geomorphological structures. LiDAR is long established and widely applied in the forestry context. Yousefi Lalimi et al. (Citation2017) has shown its applicability for herbaceous vegetation; nevertheless, LiDAR can give poor results if dense, short‐sward vegetation (as found in peatlands) disrupts the return of the laser beams from the ground surface (Luscombe et al. Citation2015). One solution to this problem in short-canopy ecosystems is to increase the number of beams per square meter. This increases the total number of returns and provides more robust statistics with which to separate the returns coming from the ground from those reflected by the canopy. LiDAR systems mounted on UAVs represent a viable and promising solution to increase the return density. Moreover, the integration of LiDAR technology with other remote sensing datasets has the potential to improve the accuracy of the AGB results, as shown by Räsänen et al. (Citation2020).
In this study a multisensory approach was used to study an alpine peatland located in Italy. The primary goal of this research was to explore the reliability of UAV spectral and LiDAR data based AGB and LAI estimates in alpine peatlands. With this end in view, we investigated the performance of (i) an approach exclusively based on deriving LAI from UAV LiDAR data; (ii) an approach purely based on VIs calculated from spectral data (i.e. UAV hyperspectral data and RGB aerial photos); (iii) an approach based on the combination of UAV LiDAR and spectral data. To verify the performance of the sensors and assess the accuracy of the results, a dataset of field observations was collected. The number of vegetation samples was minimized in order to reduce impacting or damaging protected flora.
The methods and results are presented below, followed by a discussion on the merits and limitations of the examined approaches. The significance of the methodology for the characterization of alpine peatlands in general is also discussed, bearing in mind that remote sensing applications in peatland studies outside the tropics, the arctic and sub-arctic regions are sparse and that new approaches are required (Czapiewski and Szumińska Citation2021). To the best of our knowledge, this is the first study that estimates herbaceous peatland LAI and AGB using UAV LiDAR in alpine regions.
Materials and methods
Study area
The Val di Ciampo peatland (135,000 m2) is located in the northernmost extent of the Belluno province (Veneto region, northeastern Italy) at an altitude between 1,358 and 1,425 m a.s.l. It is part of an association of dolomitic pristine peat biotopes in the surroundings of the Danta di Cadore village which form a European Community Interest Site (SIC), identified by reference number IT320060 (DIR 92/43/CEE). This group of peatlands is typical of the Alpine region, where peatlands are relict environments on the edge of their latitude distributional range (Tanneberger et al. Citation2017), mainly located in small valleys created by past glaciers (Gerdol, Tomaselli and Bragazza Citation1994) and usually less than 1 hectare in size.
Climate-wise, the area is characterized by a sub-arctic climate, typical of the Alpine region, with harsh cold winters and cool summers. Over a three-year period (2020–2022), average annual temperatures varied between 6.6 and 8.0°C, while the winter average ranged between −4.0 and 1.5°C and the summer average ranged between 13.0 and 17.6°C. The total annual rainfall was 906–1332 mm spread over 99–113 rainy days at Casamazzago station (ARPA Veneto Citation2023). The snowpack covers the study area from approximately October to April.
The selected study site within this peatland has an area of 24,000 m2 () and takes the form of an herbaceous clearing bordered by a Picea forest. The Val di Ciampo peatland has a remarkable heterogeneity in its assemblages of plant species which can be grouped into three main phytosociological alliances, in accordance with the Vegetation Prodrome of Italy (Biondi et al. Citation2014). Specifically, the coexistence of species such as Schoenus ferrugineus L., Trichophorum alpinum (L.) Pers., Trichophorum cespitosum (L.) Hartm., Eriophorum latifolium Hoppe, Tofieldia calyculata (L.) Wahlenb. Or Carex davalliana Sm., are attributed to the alliance Caricion davallinae Klika 1934, typical of “fen” community. The height of the plants forming this community varies between 0.1 m and 0.9 m (Pignatti Citation1982). A similar species composition was found in a depression in the central-east portion of the peatland (), where however, the conspicuous presence of Rhynchospora alba (L.) Vahl, Drosera anglica Huds., and Sphagnum majus (Russ.) C. Jens. led us to consider this area as part of the Rhynchosporion albae Koch 1926 alliance. The central-west portion of the peatland, where hummocks and lawns of Sphagnum magellanicum Brid. are covered by Eriophorum vaginatum L., Andromeda polifolia L., Calluna vulgaris (L.) Hull, Vaccinium spp., or Pinus mugo Turra, was instead attributed to the alliance Sphagnion magellanici Kästner and Flössner 1933 nom. Mut. Propos. Ex Steiner 1992, which represents a typical “raised bog” community. The height of the herbaceous plants forming this community varies between 0.1 m and 0.4 m (Pignatti Citation1982). In the northernmost and southernmost portions of the peatland, both fen and bog communities are partially invaded by Phragmites australis (Cav.) Trin. Ex Steud, a perennial tall reed (reaching up to a height of 6 m) that generates facies with the appearance of a reedbed (). In this paper the terms “bog” and “fen” are, respectively, linked to ombrotrophic (i.e., fed by rain) and minerotrophic (i.e. fed by underground water) typical vegetations, as used in the “Habitats Directive” (DIR 92/43/CEE). Thus, bogs are dome-shaped areas (raised above the surrounding water table) with a strongly acidic and nutrient-poor environment. Whereas fens grow on flat areas or on slightly sloping surfaces whose proximity to the water table allows the enrichment of the substrate with nutrients but also makes conditions in the root zone more anoxic. Fen vegetation tends to be richer in species and more productive than bog vegetation, but both habitats cause stress to the species growing there. Thus, imposing physiological adaptations which cost energy and limit the photosynthetic efficiency and productivity.
Figure 1. Study area. The background is the UAV-extracted orthophoto. The grey polygons highlight the core area of the vegetational alliances (cd = Caricion davallinae, ra = Rhynchosporion albae, sm = Sphagnion magellanici), while GPS colored crosses indicate the morphotypes. The coordinate reference system is WGS 84/UTM zone 33N (EPSG: 32633).

The peat accumulated at the study site was formed above moraine deposits of varying grain size (Lithostratigraphic map, scale 1:250k). The remnants of genus Pisidium shells and the gyttja found at the base of the peatland revealed the presence of a paleolake (Poto et al. Citation2013). Therefore, the genesis of this peatland is attributed to terrestrialization, i.e. the infilling of a shallow open water body by organic sediments (Joosten and Clarke Citation2002; Lindsay Citation2018).
Vegetation sampling and aboveground biomass measurements
To capture both the ecological and morphological heterogeneity, 19 sampling sites were subjectively distributed ensuring that at least three plots were allocated for each vegetation alliance/morphotype as well as including additional plots to improve the measurement of spatial variability within each category (). These sites were geolocated using a Topcon Hyper V GNSS system (0.010 m of horizontal accuracy and 0.015 m of vertical accuracy in RTK mode). The number of vegetation samples was purposely limited in order to reduce the impact of the study on the ecosystem. Only the area without arboreal vegetation was considered as part of this study, and tall shrubs (i.e. P. mugo and Juniperus communis L.) were not sampled even if they were present in some locations. Open water vegetated sites (e.g. pools) were also excluded because the infrared laser beam is absorbed by water (Pope and Fry Citation1997).
In 2021, during the peak growing season (July), field sampling was performed using a 0.5 m x 0.5 m quadrat within which vegetation cover, vegetation height and aboveground biomass were measured. The vegetation height was measured at the center and also at the four corners of each plot. The biomass of vascular plants present within the plots was harvested, with the exception of the moss layer, which was treated as belowground biomass in accordance to Cabezas et al. (Citation2015). There were also technical reasons for this decision: the laser is likely to be incapable of penetrating the dense layer of Sphagnum spp; hence, the moss surface would behave like a ground surface when captured by the LiDAR sensor. Moreover, the peatland moss layer includes live canopy near the surface, which gradually degrades with depth becoming dead biomass. It is therefore unfeasible to accurately separate the moss living and dead layers. The vegetation cover within the plots was attributed by direct observation in the field employing percentage terms in intervals of 5% (i.e. from 0% to 100%) and considering only the vascular flora cover for the reasons mentioned above. Biomass samples collected in each plot were dried at 70°C for at least 72 h, until a stable weight is reached (Cabezas et al. Citation2015). The biomass produced during the peak growing season is a good approximation of the total productivity of the ecosystem if all the dominant species reach their peak simultaneously (Malone Citation1968), as is the case for alpine peatlands.
UAV data acquisition and pre-processing
In July 2021, two UAVs were used to fly over the study area to collect hyperspectral, LiDAR and aerophotogrammetric data, covering a total area of approximately 160,000 m2. A sunny, dry, windless day was selected for the flight as weather conditions can affect the quality of the acquired data. It was intended to complete the UAV survey before collecting the vegetation samples, but repeated unfavorable weather conditions caused a delay and the flights were performed during the sampling days. Thus, the UAV data were partially affected by the presence of the field crew, and this aspect will be discussed in depth.
Prior to the flight, seven 0.5 m2 square-shaped Ground Control Points (GCPs) were homogeneously distributed within the study area, and the position of their midpoint was localized with a Topcon HR (0.010 m of horizontal accuracy and 0.015 m of vertical accuracy in RTK mode). The GNSS points were collected using the UTM 33 planar system and the calculation of the orthometric elevation was derived from the Military Geographic Institute (IGM) Grid, which has a planimetric accuracy of ±2 cm and an altimetric accuracy of ±4 cm with respect to the I-order leveling network.
Commercial drones were selected to enhance reproducibility and sustainability, from both a financial and computational perspective. It was necessary to balance the flight complexity/duration and quality of the UAV products with the available financial resources.
The UAV employed was a DJI Matrice 300 RTK quadcopter equipped with DJI Zenmuse L1, which combines a LiDAR sensor (laser wavelength at 905 nm) with a 1’’ CMOS camera of 20 MP resolution. This is able to acquire both RGB images and a RGB colored LiDAR point cloud. The flight was conducted at a speed of 5 m s−1 (18 km h−1) and height of 100 m above ground level. These parameters were automatically calculated by the flight planning application in accordance with the desired LiDAR pulse density. Virtual reference station (VRS) – Real-time kinematic (RTK) mode (with NTRIP function connected to the ITAL-POS Internet service) was activated in order to achieve the maximum declared Hovering (0.1 m both vertical and horizontal) and Positioning Accuracy (1 cm + 1 ppm horizontal; 1.5 cm + 1 ppm vertical) of the UAV. The Zenmuse L1 sensor has data accuracy of 10 cm horizontal and 5 cm vertical (Zenmuse L1 User Manual Citation2021).
Being aware of the challenges faced when scanning a short and dense canopy, the LiDAR sensor was set up to obtain a cloud with a very high point resolution (). Thus, it was enabled to collect up to three discrete returns (i.e. the maximum number possible) with a pulse repetition rate of 160 Hz (i.e. the highest possible rate when capturing three returns) resulting in an expected pulse density of 250–300 per square meter. Moreover, the non-repetitive scan mode was selected as this can increase the level of detail recorded for objects in the Field of View (FoV) by integrating the continuous scans in the time domain (Lai et al. Citation2022) as well as statistically increasing the probability of penetrating vegetation. The lateral flight overlap was set at 30%, a compromise to balance precision and noise in the LiDAR data. A greater lateral overlap guarantees a greater number of beams with smaller return angles of incidence, but the overlapping areas present greater inconsistencies in terms of point placement in the LiDAR cloud. Two datasets were produced and compared. The first dataset is referred to as “Raw” and is the point cloud resulting from the alignment and optimization of scanning strips (based on tie-line position and overlap points) processed with DJI Terra Pro software (v. 3.6.2) to resolve heading, roll, pitch and elevation parameter flaws. The data were georeferenced in UTM 33 via the “shift” algorithm of the TerraMatch software included in the Spatix Platform (v. 22.07). This linearly calibrates the horizontal and vertical positioning of the cloud based on the GCPs positions, without deforming the cloud (average Dz = 0.000 ± 0.074 m; min Dz = −0.090 m; max Dz = +0.107). The second dataset analyzed is referred to herein as the “Pre-processed” point-cloud. In this version the overlap point removal function was applied to the Raw dataset using the TerraScan software included in Spatix Platform (v. 22.07).
Table 1. Details of the high spectral resolution vegetation indices calculated for the hyperspectral data.
Table 2. Vegetation variables grouped by alliances and morphotypes. Averages ± standard deviation are reported. Same superscripted letters indicate no significant differences according to pairwise Wilcoxon rank sum tests (significance threshold p ≤ 0.05).
Table 3. Main descriptive statistics of the three analyzed LiDAR datasets.
A total of 434 RGB frames were collected in automatic shooting mode with a frontal and lateral overlap of 80% (i.e. the maximum recommended overlap in the device user manual) to maximize the number of tie-points. Images were aligned and georeferenced in UTM 33, corrected by means of GCPs (2 cm of average horizontal accuracy; 3 cm of average vertical accuracy), to produce an orthomosaic with a 2 cm x 2 cm pixel resolution. In addition, the Structure from Motion (SfM) technique (Iglhaut et al. Citation2019) was applied to extract a three-dimensional (3D) point cloud that was transformed into a 10 cm x 10 cm pixel resolution Digital Surface Model (DSM). All these operations were performed using the Agisoft Metashape software (v. 1.8.4).
In order to acquire the hyperspectral images, a DJI Matrice 600 hexacopter was equipped with a Nano Hyperspec sensor that can collect 273 spectral bands within the visible and NIR spectral ranges (from 400 to 1,000 nm). The flight was conducted at a speed of 6.5 m s−1 (about 23 km h−1) and a height of 80 m above ground level. In total 33 images with a 2.5 cm x 2.5 cm pixel resolution were collected in pushbroom linear scanning mode with a 40% frontal and lateral flight overlap, following the same rationale as for the RGB sensor. Two 9 m2 square-shaped white and gray targets were placed in the field to allow for the post-processing radiometric correction of the hyperspectral dataset and the retrieval of the spectral reflectance (as per procedure described in the sensor manual). The hyperspectral images were georeferenced in UTM33, and a single mosaic was created using the Seamless Mosaic tool provided in ENVI (v. 5.3).
GPS sampling points
In order to verify elevation accuracy of the remotely sensed data, the XYZ location of a total of 529 points were determined with a Leica Viva GS15 (0.008 m of horizontal accuracy and 0.015 m of vertical accuracy in RTK mode). Two hundred and forty-three out of the 529 points were located along flat or sub-flat artificial surfaces (a boardwalk and a wooden platform built for tourism purposes), while the remaining 286 were randomly distributed among different patches of vegetation alliances ().
Data analyses
LAI estimation
The “lidR” package (Roussel et al. Citation2020) in the R environment (v. 4.2.2) was utilized for the LiDAR data processing. The main objective of the LiDAR data analyses is the extraction of LAI. The approach applied in this study is based on gap probability, i.e. the probability that a laser beam penetrates the canopy to reach the ground (Houldcroft et al. Citation2005; Richardson, Moskal, and Kim Citation2009; Yousefi Lalimi et al. Citation2017):
where θ is the view zenith angle and G(θ) describes leaf clumping and the mean leaf projection in the direction θ. G(θ) varies between 0 and 1.
To estimate LAI from EquationEquation (1)(1)
(1) the LiDAR data must be first gridded so that P(θ) can be approximated for each grid cell, using the fraction of LiDAR returns reflected by the ground (Rg) with respect to the total number of returns in that cell (Rt). The assumptions made are: (i) a random foliage orientation (Yousefi Lalimi et al. Citation2017), which implies G(θ) = 0.5 (Campbell Citation1986) and (ii) a negligible effect of the scan angle on P(θ), particularly for small θ values, which allows us to assume θ = 0 for all returns. In order to meet the second assumption, a thorough analysis of the scan angles of the returns provided by the sensor was fundamental.
The implementation of this method encompasses six steps, as described in the flowchart of : 1) removal of duplicate points; 2) detection of the maximum acceptable scan angle and elimination of all the returns with angles in exceedance of this; 3) detection and elimination of outliers; 4) selection of the ground points and DTM extraction; 5) computation of the LAI by inverting EquationEquation (1)(1)
(1) ; 6) detection of the scan angle effect on LAI and the elimination of any higher scan angle cells which are affected.
The processing was initiated with the removal of duplicate points (i.e. points that share the same x, y, and z coordinates with others); however, they were present in irrelevant number (n = 19). The second step involved the removal of returns from points with scan angles larger than a set threshold. The threshold was defined analyzing the magnitude of effect which varying scan angles had on the results. While the scan angle effect can be ignored when it is small (e.g. <10° in Richardson, Moskal, and Kim Citation2009), a high scan angle can bias the LAI computation (e.g. a scan angle higher than 23 degrees in Liu et al. Citation2018). This is because increasing the length of the laser path through the canopy reduces the probability that the beam actually reaches the ground (Lovell et al. Citation2005). To understand how this could affect our data and results, the relationship between mean scan angle and point density for a grid with 0.5 m cells was investigated using a linear b-spline placed every 5° intervals. Based on this, it was decided to retain only points which have a scan angle modulus less than 24° (83% of the dataset), representative of the least restrictive threshold capable of stabilizing the effect of the angle on point density (Figure S4 and S5). It was taken into account that the observed relationship may be spurious since it could result from the greater presence of trees, whose greater vertical occupancy of space means they are more likely to collect extreme angles and thus a greater number of points per unit area. However, the removed points (Figure S5) were always located within the areas affected by the flight overlap stripes (Figures S3), leading to the assumption that these points represent a overlap artifact. Although the 24° threshold initially appeared to function well, the calculation of the LAI using EquationEquation (1)(1)
(1) provided poor results for angles between 24° and 20° (Figure S6). Therefore, finally only cells with mean scan angle modulus between 0° and 20° were retained.
The dataset density was further reduced through de-noising algorithms applied to decrease errors of vertical point placement caused by an imperfect stabilization of the inertial measurement unit (IMU) of the UAV. The methods provided with the R lidR package were not effective in homogeneously removing noise (Table S1), so as an alternative noise removal method all the points not included within the 1.5 times interquartile range above the upper quartile and below the lower quartile with a point-to-raster approach (Roussel et al. Citation2020) were removed. Since the product is cell-size dependent, the algorithm was tested on grids of 0.1, 0.2, 0.5, and 1 m resolution. The best performing resolutions (i.e. 0.2 and 0.5 m), the 0.5 m resolution was selected because it retained more data and coincides with the resolution at which the vegetation plots were sampled. The use of this process allowed the preservation of 96.6% of the dataset and considerably reduced the range of vertical variability of the cloud at flat reference surfaces (see paragraph 3.2). To verify the accuracy of the method, the outlier removal process was repeated and no improvement in the vertical accuracy was found (Table S2). Hence, the result of a single noise filtering is considered sufficient.
Two alternative methods were examined for ground-point classification: the Progressive Morphological Filter (PMF) (Zhang et al. Citation2003) and the Cloth Simulation Filter (CSF) (Zhang et al. Citation2016). The outputs of these methods were compared visually. The most satisfactory classification was obtained using the PMF method (), parameterized with incremental size windows (0.25, 0.50, 2, 5, and 10 m) and highly restrictive elevation thresholds (0.02, 0.05, 0.50, 1.5, and 3.5 m), which was possible due to the nearly flat morphologies of the study area (Navarro et al. Citation2020). Alternative ground-point classification approaches such as the Excessive Greenness approach proposed by Anders et al. (Citation2019) which is based on point cloud RGB coloring was also explored but was found to not be applicable in such a densely vegetated environment.
Figure 3. DTM colored by elevation gradient (quantiles) with overlaid 20 cm contour lines (c); transects (T1, T2, T3) derived from PMF classification of the point cloud (green dots = nonground-points; brown dots = ground-points; red dots = lowest ground-point contained in each cell of a grid of 0.5 m resolution) (a). Graph showing the linear relationship between DTM cells and the corresponding orthophoto-derived DEM cells on the flat reference surfaces (b).

Based on the PMF results, the Digital Terrain Model (DTM) was created by assigning to each cell of a 0.5 m resolution grid the lowest elevation value of the ground-points falling therein (Yousefi Lalimi et al. Citation2017). This method was applied to maximize the probability of selecting an elevation value that actually reached the ground. Alternative approaches based on ground-point interpolation (TIN, IDW, and Kriging) were discarded because they produced positive elevation deformations in correspondence to vegetation patches, a phenomenon absent in the gridded minimum point DTM. Moreover, the range of vertical variability of the ground-points suggested a weak distinction between ground and non-ground points (see results chapter for further detail). Holes in the DTM were not filled with interpolative algorithms because the LAI equation used in this work (EquationEquation 1(1)
(1) ) would produce values of infinity in almost all of these cells. To estimate the accuracy of the terrain model, summary statistics of the deviations between the elevations of GPS measurements and the respective cell value, for each morphotype, were produced. Accuracy was evaluated based on the R2 of the linear regression between DTM and GPS as well as DTM and DSM elevations extracted from SfM.
The direct use of the number of ground points to calculate the LAI using EquationEquation (1)(1)
(1) was not implemented due to the excessive range of vertical variability of the ground-points cloud compared to the vertical variability range of the cloud observed on flat reference surfaces. Lidar returns were instead considered to be generated by ground reflections if the height, relative to the local ground elevation (i.e. DTM cell value) at which the reflection occurred is below a fixed threshold (Yousefi Lalimi et al. Citation2017). This threshold was set at 5 cm, which is equivalent to the median range of vertical variability of the cloud on the flat and bare reference surfaces. Note that the median value was preferred to the mean value due to the highly asymmetric distribution of the data.
Extracting morphological metrics
Five basic terrain indices were extracted from the DTM using the “Basic terrain analysis” algorithms included in SAGA GIS software (v. 8.5.0). These included: Slope and Topographic Position Index (TPI) to describe small-scale morphologies; Topographic Wetness Index (TWI) and Channel Network Distance (CND) to model the effect of water at small scales; and Valley Depth (VD) to combine topological and moisture information to create a large-scale smoothed pattern. The single required input parameter was left as default (channel density = 5).
Extracting spectral indices
A variety of hyperspectral indices were found in the literature, but the focus was placed on the six best performing indices ranked by (Räsänen et al. Citation2020) for a peatland environment similar to that of this study, i.e. DD (le Maire et al. Citation2004), SR1 (Gitelson and Merzlyak Citation1997), SR6 (Zarco-Tejada and Miller Citation1999), Boochs2 (Boochs et al. Citation1990), MCARI2 (Wu et al. Citation2008), OSAVI2 (Wu et al. Citation2008), MCARI2/OSAVI2 (Wu et al. Citation2008). Moreover, also indices calculated using wavelengths larger than 800 nm (not present in the analyses performed by Räsänen et al. Citation2020) were included alongside some indices specifically created for this study (). Some indices (i.e. SR1, SR6, Boochs2, and MCARI2) proved to be excessively noisy and were ultimately excluded from the analysis.
Two orthophoto-derived indices identified by Niu et al. (Citation2019) - the Normalized Green-Red Difference Index (NGRDI) and the Excess Green minus excess Red index (ExGR) – were extracted using the Raster Calculator tool provided in QGIS (v. 3.28.3). Each raster was then up scaled to match the DTM 0.5 m resolution on SAGA GIS with the Mean Value (cell area weighted) method of grid resampling. Detailed information regarding the seven spectral indices analysis can be found in .
AGB estimation
Given the small size of the field biomass dataset available (due to the protected status of the study area any invasive activities are limited), a linear regression between observed AGB and UAV’s vegetation indices was considered the best model option to explore correlations between these parameters. The UAV indices’ values are the arithmetic averages of an orthophoto-based expert selection of 20 0.5 m cells located nearby each of the sampling sites (avoiding the cells disturbed by sampling activity). A random test was also conducted to define the minimum number of cells that would allow the linear relationship to be stabilized: the Pearson correlation coefficient was calculated from the arithmetic averages of the LAI values (taken as a reference) of n-cells (with n from 1 to 20), randomly extracted from each plot, for k-times (k = 10,000).
In addition, three sets of 20 cells chosen on bare artificial surfaces (i.e. with AGB = 0) were included to improve the dataset and a linear regression between the variables and the AGB values was then performed using R software (v. 4.2.2). Analyzing the multiple regressions, each independent variable with Variance Inflation Factor (VIF) > 5 (Fox and Monette Citation1992; package “car”) was removed to avoid multicollinearity and the best model was chosen by combining the Akaike Information Criterion (AIC) selection with a backward stepwise algorithm and the consecutive validation with Fisher’s F-test.
Hypothesis tests
A pairwise Wilcoxon Rank Sum Test for independent samples (Bauer Citation1972) with the Benjamini and Hochberg (Citation1995) p-value correction was performed to test for differences in vegetation attributes between sites grouped by alliance and vegetation morphotypes. For the comparisons, only cells falling in open herbaceous areas, away from tree shadows and totally contained within the polygons of were considered; a random selection of 1,000 cells for each group was then made in order to standardize the sample size.
The Pearson’s product moment correlation coefficient was tested with a t-test with n-2 degrees of freedom.
In linear regression, Fisher’s F-test was used both to test the significance of the model against the null model and to test the significance of the contribution of the individual variables. Residuals Normality and homoscedasticity (i.e. “same variance”) were checked, respectively, with the Shapiro-Wilk Normality Test (Royston Citation1982) and the Breusch–Pagan test (Breusch and Pagan Citation1979; “lmtest” package).
All the tests were performed using R software (v. 4.2.2). The significance threshold adopted was 0.05 unless otherwise indicated.
Results
Field observations
In the 19 plots sampled, the average vegetation height of vascular plants varies between 8.6 and 62.4 cm (µ = 32.2 cm; σ = 16.3). The AGB varies between 88.88 and 501.16 g/m2 (µ = 271.65 g/m2; σ = 87.21). The two variables show a strong positive linear correlation (r = 0.81; p < 0.001). Vegetation cover varies between 50 and 100% (µ = 83%; σ = 13%) and is linearly correlated with both vegetation height (r = 0.50, p = 0.031) and, above all, AGB (r = 0.74, p < 0,001).
Significant differences were found for AGB and vegetation height between the different vegetation categories, but not for vegetation cover (). The morphotype classification allows us to emphasize the biometric differences, especially for the high productivity of P. australis whose facies show a significant increase in both biomass and height in respect to the surrounding. The bog vegetation shows a high intra-class variability, reflecting the heterogeneity of the sampled microhabitats (Sphagnum-dominated hummocks; hummocks colonized by small moorland shrubs; Sphagnum carpets covered by Cyperaceae). Due to this variability, no significant differences are found compared to the fen vegetation, which shows higher average values. In contrast, no differences emerge by grouping sites by alliances (i.e. by ecological characters), but this is also because the presence of Phragmites increases the variability of Caricion davallinae and Sphagnion mgellanici.
LiDAR data filtering and classification
The main statistics describing the distribution of return echoes in the three datasets considered are shown in . The higher density of the raw point cloud is due to the overlapping strips, which also generates high heterogeneity in the density distribution of points (Fig. S3). Therefore, the removal of overlapping points has been applied.
In all three datasets the incidence of pulses that produced only one return (i.e. single returns) is very high, although it decreases as processing increases (82% in the raw cloud; 80% in the pre-processed cloud; 77% in the fully processed cloud). This result highlights the difficulties experienced by the laser scanner of collecting more than one return in herbaceous and low-shrub vegetation (Fig. S1 and S2).
The collected UAV data cover the area of interest in three survey strips in a north–south direction (Fig. S4). In the pre-processed cloud, 19% of that area was scanned near NADIR (i.e. almost perpendicular to the ground) with a scan angle between 0 and 5°; 39% of that area was scanned with an angle between 5 and 15° and the remaining 42% of the area with angle between 15 and 35°. Filtering the cloud for only those returns with a modulus of scan angle less than 24° essentially nullifies the effect of scan angle on point density (compare Fig. S4 and S5) leaving the points that are essential to the analysis. In fact, the resulting cloud retained 83% of the data, without affecting areas of herbaceous vegetation and minimally affecting the crowns of the trees, which are not considered in this study (Fig. S4 and S5). The density of this cloud (256.8 points/m2) is only slightly higher than that of the fully processed de-noised cloud; the latter, however, has an average vertical range on flat reference surfaces of 0.09 m ±0.23 (n = 181; median value = 0.05), while the non-de-noised cloud has an average vertical range of 0.23 m ±0.41 (n = 181; median value = 0.11). Therefore, the fully processed cloud was considered the most suitable dataset of the three to use for calculating LAI using Equationequation (1)(1)
(1) .
The PMF classification system identified 3.23 million points (27% of the total) as ground-points. The vertical range of the ground-points cloud (0.11 ± 0.08; n = 139,984) was significantly greater than the vertical range of the cloud on the flat reference surfaces. This result suggests that the algorithm provides an imperfect distinction between ground and non-ground points. The use of more restrictive thresholds in the algorithm, however, led to the elimination of entire micro-morphologies (not shown data). This finding justified the use of the method of taking the minima for when creating the DTM, as described in the Methods section.
DTM information and its reliability
The DTM produced () represents the best estimate of the actual ground position in each 0.5 m x 0.5 m cell of the grid. Based on this it was possible to observe the geomorphology of the study area, which is a sub-flat basin characterized by the following configurations: (i) a northern portion marked by a modest slope falling in the north–south direction (approximately 3.5 decimal degrees, on average); (ii) a depression in the central-western portion, which has a complex micromorphology of ridges and depressions (stretched out perpendicularly to the main slope direction) bordered by forested surfaces that are gently sloping (by about 1 decimal degree, on average) toward the peatland; (iii) and a southern portion that shows both a moderate north-south or east–west slope (about 3 decimal degrees, on average) toward the mouth of the main channel that emerges above the surface at the southernmost point of the study area and drains water from the entire basin.
The DTM on flat reference surfaces (i.e. boardwalk and platform) proved to be accurate both when compared with the orthophoto-derived DEM elevations and the GPS measurements. Respectively, the R2 indicator takes values of 0.999 () and 0.997 (n = 243; p < 0.001). Nevertheless, when the DTM is compared to the GPS values collected across the peatland, the accuracy decreases in the vegetated areas and the DTM overestimates the actual ground elevation (). This result confirms that the laser pulse struggled to penetrate the vegetation across the entire peatland, especially for areas dominated by P. australis, which is notably dense and tall (i.e. 51.7 cm on average).
Figure 4. Gap between DTM-estimated elevation and actual ground elevation for different landscape elements. Red dots indicate the average value. The black dotted line marks the actual terrain level. Round brackets indicate the sample size. The blue crossed circles represent the average vegetation height observed in the field.

Estimated AGB
In order to assess the efficacy of using different sensors, the results obtained for the estimation of the AGB are shown for three cases: (i) using exclusively LiDAR, (ii) using exclusively hyperspectral and RGB data, and (iii) using both LiDAR and spectral data.
LiDAR data
The main statistics of the DTM derived metrics at the peatland scale are summarized in .
Table 4. Main statistics of DTM derived metrics across the study area.
The relationships of these metrics to each other and to the observed AGB are listed in (and depicted in Fig. S9). The four indices TPI (r = −0.59; p = 0.0038; n = 22), TWI (r = 0.59; p = 0.0040; n = 22), CND (r = −0.49; p = 0.0194; n = 22), and LAI (r = 0.85; p < 0.001; n = 22), taken individually, correlate with the AGB values.
Table 5. Pearson’s correlation matrix with significance thresholds (* < 0.05; ** < 0.01; *** < 0.001) between LiDAR metrics and observed AGB (n = 22).
According to both the AIC indicator and Fisher’s test, LAI is the only independent variable retained in the regression model since it incorporates the contribution of the other variables. Thus, it was found that when using exclusively the average LAI in the model, the AGB (R2 = 0.72, F[1, 20] = 51.324; p < 0.001) () can be significantly explained. The residuals were normally distributed (W = 0.98146, p = 0.9579) and had a constant variance (BP = 0.11338, df = 1, p = 0.8991).
Figure 5. A selection of the UAV indices obtained from LiDAR (a), hyperspectral (b & c), and orthophoto (d) alongside their univariate linear relationship with the observed AGB.

Observing , certain vegetation patches could be recognized based on the LAI values. The highest values were observed in some areas occupied by P. australis as well as the wooded areas and the individual P. mugo shrubs. Considering only cells in open herbaceous areas, the estimated LAI values in the reedbed areas are clearly higher than those of the other categories (Tab S3).
Hyperspectral and RGB data
The key statistics of hyperspectral and orthophoto-derived vegetation indices (VIs) at the peatland scale are summarized in . The relationships of these indices to each other and also to the observed AGB are listed in (and depicted in Fig. S10). All indices are highly intercorrelated (0.71 <|r|< 0.99; p < 0.001; n = 22). Among these, the indices that are least correlated to the observed AGB are Wetness index (r = 0.64; p = 0.001; n = 22), NGRDI (r = 0.67; p < 0.001; n = 22) and ExGR (r = 0.67; p < 0.001; n = 22). On the other hand, HNDVI, DD, NDWI, and OSAVI2 provide approximately the same level of information (0.77 <|r|< 0.80; p < 0.001; n = 22).
Table 6. Mean statistics of the chosen spectral indices across the entire study area.
Table 7. Pearson’s correlation matrix with significance thresholds (* < 0.05; ** < 0.01; *** < 0.001) between hyperspectral/optical indices and observed AGB (n = 22).
HNDVI, NDWI, and OSAVI2 contain overlapping information. Thus, multicollinearity (VIF >5) can occur when these are combined in the same regression model. Therefore, HNDVI was selected from among the three indices because of its clear physical link to plant biomass (Huang et al. Citation2021). HNDVI significantly explained AGB (R2 = 0.61, F[1, 20] = 31.668; p < 0.001) () with normally distributed residuals (W = 0.94443, p = 0.244) and a constant variance (BP = 1.3308, df = 1, p = 0.2487). The same level of deviance was explained by the model based on the DD index alone (R2 = 0.62, F[1, 20] = 32.052; p < 0.001) () and an assumption that there is normality of the residuals (W = 0.9587, p = 0.4634) and that homoscedasticity (BP = 0.024485, df = 1, p = 0.8757) is respected. Moreover, DD turned out to be the more reliable index due to its high correlation in the interval 85–550 g/m2 AGB. Further information regarding this will be discussed in the next section.
The best performing orthophoto-derived model was produced based on NGRDI (R2 = 0.45, F[1, 20] = 16.414; p < 0.001) (), with normally distributed residuals (W = 0.96733, p = 0.6494) and a constant variance (BP = 0.30049, df = 1, p = 0.5836). This model was preferable over ExGR despite the identical R2 since the former fits the AGB values better within the range of 85–550 g/m2 (R2 = 0.55 vs R2 = 0.35).
The model performance when using indices obtained from both orthophoto and hyperspectral data was also tested. When both NDGRI and HNDVI were considered, no significant improvement was observed (F = 0.1734; p = 0.6818). A similar finding was also observed when both ExGR and DD were used in the model (F = 2.245; p = 0.1535). The other combinations examined cause multicollinearity according to the VIF indicator.
As shown in , NGRDI, HNDVI, and DD are in accord when attributing high values (NGRDI >0.15, HNDVI > 0.9, DD > 0.1) to the areas occupied by P. australis and in locating a higher biomass in the reedbed compared to the other categories (Tab. S4 and S5), areas also identified with high LiDAR LAI values. High contrast values are also identified along the numerous ridges that make up the depressed central part of the peatland in all the three indices. Furthermore, there is agreement in assigning low values (NGRDI <0.05, HNDVI < 0.8, DD < 0.01) to pools. High values of HNDVI (>0.9) and low values of DD (<0.03) were found only in areas shaded by trees. High values of DD (>0.1) and low values of HNDVI (<0.8) were mainly found in areas of more sparsely colonization by P. australis.
LiDAR and spectral data combined
When NGRDI and HNDVI were added to the LAI model to improve its explanatory power of AGB, it was demonstrated that neither of these two indices was able to increase the performance (respectively: F = 1.5608, p = 0.2267; F = 3.1382, p = 0.0925). Instead, it was only the DD index that was found to have a significant additional contribution (F = 5.6704, p = 0.0279) which improved the model performance (F = 14.333, p = 0.0012). Thus, the best model was generated by the integration of LiDAR and hyperspectral data, and it was able to explain a substantial fraction of the AGB variability (Adj. R2 = 0.76, F [2, 19] = 33.88; p < 0.001). The final fitted linear regression model was: AGB = 9.786 + 106.996*LAI +1239.529*DD. Residuals were normally distributed (W = 0.95687, p = 0.4288) and had a constant variance (BP = 1.2851, df = 2, p = 0.5259).
The AGB map based on this model is shown in . Considering only the 75,556 cells (18,889 m2) entirely contained within the study area perimeter, the estimated AGB varies between 0 to 1,314.35 g/m2. The mean value was 321.58 ± 217.29 g/m2, and the median value was 309.10 g/m2. Approximately 75% of the data are contained within the range 0–450 g/m2. Values greater than 750 g/m2 were only exhibited on the reedbed areas, shrubs, or around the no data gaps (i.e. in the immediate proximity of dense tree vegetation). Values greater than 1,000 g/m2 are extremely rare in open herbaceous or low shrub vegetation.
Figure 6. AGB map produced by combining LiDAR and hyperspectral information. The orthomosaic-derived DEM serves as the base map. The coordinate reference system is WGS 84/UTM zone 33N (EPSG: 32633).

Considering 1,000 pixels randomly selected as described in the Methods section, shows that the estimated AGB in the different vegetation categories for the herbaceous area reflects what was observed in the field (see paragraph 3.1). In the studied peatland, classification using morphotypes proved to be the most suitable method for highlighting biometric diversity. Many positive outliers were also present in each considered category, which suggests the presence of noise in the independent variables chosen to constrain the model.
Figure 7. Comparison between the observed AGB in the field and the AGB estimated by the multiparametric linear model, grouped by vegetation type. Red dots indicate the average value. Round brackets indicate the sample size. In square brackets, the outcome of the Kruskal-Wallis test is summarized (different letters indicate significant differences; threshold: p < 0.05).

Discussion
Performance of tested AGB variables
The primary objective of this study was to combine multi-source remotely sensed datasets to estimate the AGB spatial patterns across an herbaceous alpine peatland, calibrating these datasets by means of minimal ground-truth samples in order to limit the vegetation harvesting required. Another objective of our study was to compare the results obtained using LiDAR and optical data to determine which dataset yields the best performance with the least investment of effort and resources.
Our results indicate that if only one sensor is to be selected for assessing peatland AGB, the optimal choice would be a LiDAR sensor. The LiDAR-derived LAI proved to be the variable with the highest explanatory power for AGB, which allowed us to explain over 70% of its variability (R2 = 0.72, ). The inclusion of non-vegetated plots was an essential step to improve the calibration of the model, especially considering that in herbaceous ecosystems there is a limited range of AGB values (85–550 g/m2 in this case study). In fact, if the model is trained exclusively using AGB values between 85 and 550 g/m2 the explanatory ability decreases (R2 = 0.41, Fig. S11). Moreover, only the model with zero-AGB values returns an intercept that is not significantly different from zero (p = 0.499).
Other studies have demonstrated that LiDAR-derived LAI is highly correlated to AGB, including other non-forest ecosystems characterized by short vegetation such as dunes (Yousefi Lalimi et al. Citation2017). The main concern when using this methodology on dense and short peatland vegetation () was related to the poor penetration of the laser beams through the canopy, thus limiting its ability to reach the ground (Luscombe et al. Citation2015). Our results showed that the number of laser beams intercepted by the vegetation is higher for tall and dense plants, as is the case for P. australis which reduced the ability of the model to detect the ground surface. In fact, although the DTM shows good levels of precision and accuracy on flat artificial surfaces (i.e. boardwalk and platform) (), the accuracy decreases in vegetated areas and the DTM overestimates the actual ground elevation. The overestimation appears to be positively correlated to the vegetation height (), and only a few beams seem to have been able to reach the ground in areas covered by dense and tall vegetation. However, when comparing the average gap of the DTM relative to the ground with the average vegetation height measured in the field in those specific vegetation types (), it can be seen that the penetration of the laser increases as the average vegetation height increases. Thus, the beam tends to stop almost immediately in the bog vegetation, after a few centimeters in the fen vegetation and after a few tens of centimeters in the reeds. It follows that the LAI values correlate better with the mean vegetation height (r = 0.86, p < 0.001, n = 22) than with the percentage cover (r = 0.76, p < 0.001, n = 22). The close relationship that has been observed between AGB and the mean height rather than between AGB and the canopy percentage cover (r = 0.81 vs r = 0.74) supports the good performance of the LiDAR LAI as a proxy of AGB in this ecosystem, despite the poor penetrability of the laser beam in the peatland vegetation.
The decent performance of the LiDAR data was not unexpected. Unlike passive remote sensing, which records an integrated signal from reflected energy, LiDAR provides a chance to characterize the canopy in 3D with a high level of detail in the vertical axis (Tian, Qu, and Qi Citation2021). Moreover, the gap fraction model based on the Beer-Lambert law has been proven useful to estimate LAI in other studies (Tian, Qu, and Qi Citation2021). Finally, the linear relationship between LAI and AGB could also be anticipated based on previous literature (Crabbe et al. Citation2019).
The strong and significant correlation between the LAI derived by LiDAR and the AGB makes this index the most reliable single UAV system-based variable for explaining the peatland vegetation biomass variability. However, the low penetrability of the laser beams has important implications on the application of EquationEquation (1)(1)
(1) to derive the LAI. For the model developed by Houldcroft et al. (Citation2005) and then by Richardson et al. (Citation2009) to derive realistic values of LAI, it requires a realistic portioning of the beams reaching and not reaching the ground. If, as in our case, the number of beams that could reach the ground is smaller than the actual value, the LAI will be overestimated. Only a few studies have measured LAI directly on peatland vegetation in temperate or boreal belts (i.e. where the vegetation is similar to that of our study area). Rastogi et al. (Citation2018; Rastogi et al. Citation2022) measured the LAI of vascular plants in a Polish peatland and recorded values ranging between 0.4 and 2.5 m2 m−2. Similar values were reported for an ombrotrophic peatland in Ontario by Moore et al. (Citation2002). Mohammedshum and Kooistra (Citation2015) measured LAI directly in a Dutch peatland, focusing only on tree vegetation, and obtained values between 0.3 and 1.2 m2 m−2. Räsänen et al. (Citation2020) collected samples and determined the LAI by means of a laboratory scanner, obtaining an average LAI of 0.465 m2 m−2, a value significantly lower than ours (2.99 m2 m−2). Garisoain et al. (Citation2022) collected samples of sphagnum mosses in a peatland in the French Pyrenees and then in the laboratory ascertained values of LAI as high as 6 m2 m−2. However, this type of vegetation is so dense and impenetrable that we consider it to behave similar to the ground surface. Taking the aforementioned studies into account, the LAI values derived from LiDAR data in the present study are disproportionately high and should not be considered as true LAI values but as a proxy. In the case that a true LAI estimation based on LiDAR data is needed, an ad hoc calibration using field measurements should be applied (Tian, Qu, and Qi Citation2021).
Despite these remarks, it must be underlined that LiDAR data may also provide valuable information about the peatland morphology and possible impacts or links with the ecosystem characteristics in general. In this study, LiDAR-derived morphological metrics highlight a trend where a greater AGB is detected in areas with increased proximity to water (high TWI, low CND, and low TPI). This aspect could be explained by a higher affinity for water adjacent habitats by P. australis. This species is considered an indicator species for a marsh or fen water’s edge (Rydin and Jeglum Citation2006) and furthermore its presence significantly increases the AGB ().
The results show that morphological metrics based on LiDAR may provide interesting ecological insights, even though their contribution did not improve the AGB mapping ability. In fact, these metrics correlate weakly or insignificantly in the range 85–550 g/m2, and in the regression model, their effects are discarded according to AIC indicator and Fisher’s test. This result was in part unexpected because in this ecosystem the distance from the water table is considered the most important factor determining the different species associations (Gerdol, Tomaselli and Bragazza Citation1994; Bragazza Citation1996; Bragazza and Gerdol Citation1999), which are in turn, indicators of AGB spatial variability. As already hypothesized for the LAI retrieval, it appears that the poor penetration of the laser into the canopy played a major role in negatively affecting the ability to describe the general microtopography.
Analyzing the performances of specific indices, derived from an RGB camera and a hyperspectral sensor, showed that common optical data acquired with a UAV three-band camera does not provide indices which can improve the performances of the model. Not surprisingly, among the spectral indices, the hyperspectral ones showed higher explanatory power when compared to the RGB camera indices. VIs are developed to maximize sensitivity to vegetation characteristics while minimizing confounding factors such as soil background reflectance, directional, or atmospheric effects. It is well known that these conditions are satisfied above all others by the information contained within the red and near-infrared (NIR) bands (Fang and Liang Citation2014). Despite containing these important red and NIR bands, the hyperspectral indices gave mediocre performances. The HNDVI index, as well as the other indices closely related to it (NDWI and OSAVI2), is clearly affected by the saturation effect (). Fig. 5.2 clearly shows that HNDVI retains a satisfactory level of explanatory capacity (R2 = 0.62) only when the zero-AGB values are included (i.e. from the non-vegetated plots), whereas, if only the AGB range 85–550 g/m2 was used, the performance of the model would drop drastically (R2 = 0.08, Fig. S11). On the contrary, the DD index showed a high explanatory ability (R2 = 0.62) and did not seem to be affected by the saturation problem ( and Fig. S11). Thus, the DD index can be judged as the best explanatory variable within the set of spectral indices considered. This result agrees with Räsänen et al. (Citation2020), who place the DD index at the top of a ranking of importance for a peatland AGB assessment where only hyperspectral variables were employed. Nonetheless, the use of DD alone as the sole independent variable (like the other hyperspectral indices) provides results that are less reliable than the LAI from LiDAR, probably because of its sensitivity to the optical properties of the soil background. These optical properties are affected by shadows and humid depressions, resulting in very contrasting LAI values being attributed at the margins and along the system of riffles and pools in the center of the peatland ().
Testing the model using several combinations of indices extracted from the data collected by three sensors, it was found that the most reliable and robust model combines indices retrieved from both LiDAR and hyperspectral data (AGB = 9.786 + 106.996*LAI +1239.529*DD). This provides a higher explanatory power (i.e. Adj. R2 = 0.76) and an intercept even closer to zero with respect to the model with solely LAI. One possible explanation for the strong performance of the model combining LAI and DD lies in its high sensitivity to the background setting. Hence, the DD index seems to have a greater ability to identify low AGB in sparsely vegetated temporary pools in comparison to the LAI, which instead reported increased levels of noise. Therefore, the DD index may contribute to the model by better defining the presence of bare soil. Not by chance, the DD index correlates slightly better with the percentage cover (r = 0.72, p < 0.001, n = 22) than with the mean vegetation height (r = 0.70, p < 0.001, n = 22). However, it should be pointed out that the increase of R2 is very limited, from 0.72 to 0.76. The limited improvement provided by the DD index is even more evident if the model was only trained for the 85–550 g/m2 AGB range, in which case the DD index does not even significantly improve the performance (F = 3.0827; p = 0.09825). It follows that in this range the additive effect of DD decreases the explanatory power of the model (R2 = 0.63).
In general, the AGB predicted with the model that combines LAI and DD agrees well with the field observations. Specifically, the field measurements of AGB vary in the range 89–501 g/m2 with an average value of 271.65 ± 87.21 g/m2, that is in line with previous literature data for boreal peatlands (Moore et al. Citation2002; Murphy, McKinley, and Moore Citation2009; Räsänen et al. Citation2020), but the differing nature of the northern peatlands makes a valid comparison difficult to make. The AGB estimated with the model varies across the marsh in the range of 13.48–1,123.46 g/m2 with an average value of 319.56 ± 136.83 g/m2. Looking at the sampled plots, estimated AGB values have high standard deviations, both overall and in between vegetation categories (), including some anomalous and meaningless values. Variables retained in the model – but also those excluded – cause large variability (see Tab S3, S4, and S5). Moreover, in the applied sampling scheme, shrubs were not considered, which prevented the calibration of the regression model for large values. Therefore, it is possible that the equation applied in this study may have underestimated the AGB in the area occupied by P. mugo.
In general, the AGB statistics produced in this study confirms the well-known trophic trend of peatlands vegetation (Rydin and Jeglum Citation2006), since the nutrient-poor bogs have a lower biomass than the more nutrient-rich fens (). Moreover, the AGB map () shows areas characterized by a greater biomass in some parts of the reedbed (as well as near trees and shrubs), where the presence of P. australis alters both the parameters biomass and height (). In particular, the highest AGB values (<600 g/m2) are registered in the central-southern portion of the study area, in the middle of the P. australis southern patch. It should be pointed out that all maps in detect high AGB values in that area. The same result was also obtained by calculating the difference between the 10 cm resolution DEM derived from SfM and the DTM extracted from LiDAR data with an equal resolution (data not shown).
Considerations on merits and limitations of LiDAR data acquisition and processing
The LiDAR data acquisition phase using UAV systems is an essential step for the success of the applied methodology. The gap probability approach used to extract the LAI requires a high point-density cloud and information on the vertical structure of individual plants to perform at its maximum potential. Although mounting LiDAR systems on UAVs allows these statistics to be maximized, one must be aware that the application of LiDAR in short-canopy systems such as peatlands means taking the sensors to the limit of their discriminatory skills. Therefore, it is necessary to accurately examine the available acquisition methods and select the one that increases the number of beams per square meter and enables the greatest vegetation penetrability. In this study, the DJI Zenmuse L1 sensor was functioning at its maximum capacity (i.e. 3 returns at 160 Hz) and retrieved a high-density cloud with 321 points/m2 on average (pre-processed point cloud). Moreover, a non-repetitive acquisition scheme which is unique to the L1 sensor’s LiDAR technology was selected, wherein a very large vertical FoV is employed (greater than the horizontal FoV thus generating a near-circular FoV) such that the point density should significantly increase even after 0.3 s of integration time. This scanning mode reveals more detailed information regarding the surroundings in comparison to a traditional scanning pattern (see L1 sensor’s User Manual for more details). Despite using this scanning advancement, the results show a prevalence of the first-return group () and no points belonging to the second- and third-return groups were recorded within the study area, only in the surrounding areas characterized by the presence of trees and shrubs (Fig. S2). According to Sofonia et al. (Citation2019) statistics related to key UAV LiDAR point cloud metrics can be accurately predicted before the flight. Thus, improved flight design can increase the performance of the sensor. For example, the best overall performance of UAV LiDAR density and accuracy occurs at relatively low flight altitudes (<30 m) and when flight time is increased over the target area (“cross-flight”) (Sofonia et al. Citation2019). The latter is also suggested based on the results of Qin et al. (Citation2017) that showed how overlapping strips of different scan angles (up to 25°) could improve the reconstruction of the leaf profile. Moreover, general trends indicate that speed is inversely proportional to density. The results of this study may reflect a current intrinsic limitation of the available technology (see, for example, overlap). But the use of waveform LiDAR instead of discrete LiDAR returns seems a promising solution for the problem (e.g. Miura et al. Citation2019), although this would increase the complexity of dataset analysis.
The flip side of using the non-repetitive acquisition scheme is that, along flight strips (Fig. S3), the point-cloud is less homogeneous: the sensor explores the FoV with flower petal-shaped scans, which by converging toward the center generate a higher point-density within a radius of 10° of the center of the FoV (i.e. from the nadir). Thus, a fundamental aspect to consider is whether this non-uniformity generates biases in deriving the LAI or the DTM for specific scan angle ranges. Based on the results, scan angles of 20° should not be exceeded (Fig. S7). This threshold is more conservative than the one adopted by Yousefi Lalimi et al. (Citation2017) in a similar study, but is in line with the findings of Liu et al. (Citation2018) and – partially– Dayal et al. (Citation2020) for airborne forestry applications. The parallelism with the latter research is corroborated by simulations of Qin et al. (Citation2017), which showed that flight height does not affect the choice of the best scan angle.
To extract the DTM from the LiDAR cloud, the method proposed by Roussel et al. (Citation2020) was slightly modified. Noise removal based on the interquartile range (IQR) was used, as it proved effective on this data set (Tab. S1) and performed better than the methods included in the “liDR” package proposed by Roussel et al. (Citation2020), i.e. SOR and IVF algorithms. The SOR method was parameterized to function for contours comprising the average number of points within 0.5 m2 (to emulate our approach; but also similar to the choice of Zeybek and Şanlıoğlu Citation2019) and with standard deviation intervals of 3 and 2 to have a high level of certainty of not committing a Type II error. The IVF method was parameterized as both stringent (classifying returns as outliers when they have at most 1 other neighbor return within 0.1 m resolution contours) and lenient (5 neighbors within 1 m resolution voxels). Both SOR and IVF proved to over-penetrate the considerable vertical range, i.e. detecting outliers only on trees (or even on shrubs and reeds when parameterized in an stringent way). Therefore, both methods failed to filter within the small vertical ranges imposed by short-canopy vegetation, which are only partially penetrated by the laser. In the case of the SOR, this could be due to the standard deviation value, wherein the algorithm is methodologically based and is influenced by the outliers themselves (whereas this is not the case using position indices such as the interquartile range). Regarding the IVF method, the 27 voxels (3 × 3 x 3) imposed may encompass too wide a neighborhood. Therefore, this study entails limited conditions for the applicability of methods created to work effectively in forest canopies such as those implemented in the “lidR” package. The IQR method proved to be the most effective probably because the target vegetation shows a threadlike, dense and rather homogeneous development within short distances. Therefore, the subset of data on which the algorithm works is also rather coherent. On the other hand, the proposed method might be too strict in the case of vertically stratified vegetation, such as in forest ecosystems. Hence, forested areas surrounding the study site were not considered.
Our results also showed that ground-point classification may be an unreliable method for the creation of the DTM. As described in the previous chapters, the minimum value within each cell of a grid system (as per Yousefi Lalimi et al. Citation2017) was selected because the vertical range of the ground-points seemed disproportionate compared to the same range measured on flat surfaces. Despite the reliability of the results obtained, one difficulty encountered was evaluating the performance of different methods and different parameterizations objectively and in a timely manner. Zeybek and Şanlıoğlu (Citation2019) have proposed an analytical approach but it relies on having a reference dataset previously classified by an operator. More often, therefore, one settles for a visual transect inspection approach (Navarro et al. Citation2020). In the case of this study, the PMF method was preferred. For this method, the selection of the window size and elevation difference threshold when applying filter is critical to achieve good results. Zhang et al. (Citation2003) suggested the use of simple equations to increase both parameters in a standard way after choosing the first value (i.e. the most restrictive). However, these equations are not solvable in the case of windows of less than one unit (i.e. 1 m in this case). Despite this, windows of less than 1 unit seem to be an indispensable condition when working with ultra-high resolution data. We have considerably widened the range of possible parameter combinations, which complicates the search for the most suitable one. A valid selection of many ground-points would have been preferable to the few selected points in discrete intervals used to construct the DTM. Just as it would have been preferable to directly apply ground and non-ground classification for the calculation of the gap fraction to extract the LAI instead of applying a uniform threshold throughout the cloud. However, a combination of parameters capable of satisfactorily reducing the average vertical range without removing entire micromorphology (i.e. the various riffles in the center of the peatland) was difficult to obtain.
As previously mentioned, the UAV surveys were performed during the sampling days instead of prior to, a consequence of repeated unfavorable weather conditions. For this reason, the data collected by drone in the immediate vicinity of the sampling sites (radius of about 1 m) had to be masked. As described in the Methods section, this issue was resolved by selecting a set of 20 cells in the vicinity of each sampling site that we considered to be representative of the vegetation and topographical characteristics of the reference site. The appropriateness of this selection was based on orthophotos and depth knowledge of the study area. To validate this, the LAI was used as the reference variable and the random test was performed. The results (Fig. S8) demonstrate that both average Pearson’s correlation coefficient and its standard deviation range maintain positive values across the entire range of abscissae, i.e. selecting from 1 to 20 cells per site. Since LAI is being used as a proxy for AGB, this result is reassuring in terms of the goodness of the chosen cells. It should also be noted that one of the randomized combinations of three cells would maximize the correlation (r = 0.92), although only the use of > 10 cells guarantees a stable correlation. Thus, the results show that n = 20 is far too conservative a choice.
Conclusions
Our results suggest that the AGB of herbaceous peatlands can be accurately mapped by calculating the LAI from UAV LiDAR data and then using the LAI in a linear regression model. In our study we obtained a significant explanatory power (R2 = 0.72) using solely UAV LiDAR data collected on a typical alpine peatland covered with dense and highly heterogeneous herbaceous vegetation. When hyperspectral data are also available, spectral vegetation indices may add explanatory power to the model, even though their contribution is limited to only marginally improving the model’s explanatory ability. In our case, combining the LiDAR-derived LAI with the DD index calculated using hyperspectral data increased the R2 to 0.76. Finally, our results show that when the regression is attempted using exclusively spectral indices obtained from hyperspectral data, the explanatory power of the model is lessened.
The results obtained from this research highlight both the positive aspects and the limitations of the examined approaches. The use of LiDAR as a single sensor simplifies the data collection phase and reduces the costs, especially if one considers the limited general availability of hyperspectral sensors. Another positive aspect is that LiDAR sensors can be operated in poor visibility conditions. On the other hand, the methodology customized for this study is greatly dependent upon the presence of a highly dense point cloud, thereby exhibiting great sensitivity to the characteristics of the LiDAR sensor and the acquisition geometry. Moreover, the parameters chosen for processing the LiDAR data in this research are primarily based on an intense and laborious empirical process and there is a need to validate the replicability of the method in other peatlands or similar ecosystems.
The limited contribution offered by hyperspectral-derived vegetation indices investigated in this research confirms the outcomes of prior studies conducted on herbaceous peatlands. This poses the challenge of finding novel methods to exploit the potential of hyperspectral and multispectral data for estimating vegetation aboveground biomass (AGB) in alpine peatlands.
Our study stimulates two lines of ongoing research. The first investigates how to best retrieve LAI in herbaceous ecosystems using UAV LiDAR data, testing among other aspects different flight parameters and sensor types. The second line of research includes the testing of other hyperspectral sensors that contain more NIR bands and possibly also SWIR (Short Wave InfraRed) bands, since unfortunately the sensor used in this study could only sample up to 1000 nm.
Supplemental Material
Download MS Word (5.2 MB)Acknowledgments
This work was carried out as part of the project “CHANGED - CHAracteriziNG pEatlands from Drones”, funded by the University of Padua (Italy) by means of the STARS Consolidator Grants. We thank the anonymous reviewers and the guest editor for having provided precious comments and suggestions that noticeably improved the quality of the paper.
Disclosure statement
The authors declare that they have no known competing financial interests or personal relationships that could have appeared to influence the work reported in this paper. Antonio Persichetti and Cristiano Miele work for a private company that runs UAV surveys with the sensors used in this paper.
Data availability statement
The data that support the findings of this study are openly available in the Zenodo repository at http://doi.org/10.5281/zenodo.7912969. They include:
“Danta_dem_10cm_px.tif:” orthomosaic-derived DEM;
“Danta_rgb_2.2cm_px.tif:” orthophoto;
“GPS points:” list of GPS samples points;
“Main data:” field vegetation data and indices used for the regression models;
“Raw PointCloud:” Lidar original dataset;
“Pre-processed PointCloud:” Lidar dataset after pre-processing (see paper’s Methods).
Supplementary material
Supplemental data for this article can be accessed online at https://doi.org/10.1080/15481603.2023.2270791
Additional information
Funding
References
- Aase, J. K. 1978. “Relationship Between Leaf Area and Dry Matter in Winter Wheat 1.” Agronomy Journal 70 (4): 563–27. https://doi.org/10.2134/agronj1978.00021962007000040011x.
- Anders, N., J. Valente, R. Masselink, and S. Keesstra. 2019. “Comparing Filtering Techniques for Removing Vegetation from UAV-Based Photogrammetric Point Clouds.” Drones 3 (3): 61. https://doi.org/10.3390/drones3030061.
- Antala, M., R. Juszczak, C. Van der Tol, and A. Rastogi. 2022. “Impact of Climate Change-Induced Alterations in Peatland Vegetation Phenology and Composition on Carbon Balance.” Science of the Total Environment 827:154294. https://doi.org/10.1016/j.scitotenv.2022.154294.
- ARPA Veneto. 2023. Meteorological Data. https://wwwold.arpa.veneto.it/bollettini/storico/Mappa_2022_TEMP.htm?t=BL.
- Bajocco, S., F. Ginaldi, D. Morelli, M. Scaglione, D. Fanchini, E. Raparelli, S. U. M. Bregaglio, and F. Savian. 2022. “On the Use of NDVI to Estimate LAI in Field Crops: Implementing a Conversion Equation Library.” Remote Sensing 14 (15): 3554. https://doi.org/10.3390/rs14153554.
- Bauer, D. F. 1972. “Constructing Confidence Sets Using Rank Statistics.” Journal of the American Statistical Association 67 (339): 687–690. https://doi.org/10.1080/01621459.1972.10481279.
- Benjamini, Y., and Y. Hochberg. 1995. “Controlling the False Discovery Rate: A Practical and Powerful Approach to Multiple Testing.” Journal of the Royal Statistical Society, Series B 57 (1): 289–300. https://doi.org/10.1111/j.2517-6161.1995.tb02031.x.
- Biondi, E., C. Blasi, M. Allegrezza, M. Anzellotti, M. M. Azzella, E. Carli, S. Casavecchia, et al. 2014. “Plant Communities of Italy: The Vegetation Prodrome.” Plant Biosystems 148 (4): 728–814. https://doi.org/10.1080/11263504.2014.948527.
- Boochs, F., G. Kupfer, K. Dockter, and W. Kuhbauch. 1990. “Shape of the Red Edge as Vitality Indicator for Plants.” Plants International Journal of Remote Sensing 11 (10): 1741–1753. https://doi.org/10.1080/01431169008955127.
- Bragazza, L. 1996. “Delimitation of the Aerobic Peat Layer in a Sphagnum Mire on the Southern Alps.” Oecologia montana 5:41–46.
- Bragazza, L. 2006. “A Decade of Plant Species Changes on a Mire in the Italian Alps: Vegetation-Controlled or Climate-Driven Mechanisms?” Climatic Change 77:415–429. https://doi.org/10.1007/s10584-005-9034-x.
- Bragazza, L., and R. Gerdol. 1999. “Hydrology, Groundwater Chemistry and Peat Chemistry in Relation to Habitat Conditions in a Mire on the South – Eastern Alps of Italy.” Plant Ecology 144 (2): 243–256. https://doi.org/10.1023/A:1009868113976.
- Bragazza, L., H. Rydin, and R. Gerdol. 2005. “Multiple Gradients in Mire Vegetation: A Comparison of a Swedish and And Italian Bog.” Plant Ecology 177 (2): 223–236. https://doi.org/10.1007/s11258-005-2182-2.
- Bratsch, S., H. Epstein, M. Buchhorn, D. Walker, and H. Landes. 2017. “Relationships Between Hyperspectral Data and Components of Vegetation Biomass in Low Arctic Tundra Communities at Ivotuk, Alaska.” Environmental Research Letters 12 (2): 025003. https://doi.org/10.1088/1748-9326/aa572e.
- Breusch, T. S., and A. R. Pagan. 1979. “A Simple Test for Heteroscedasticity and Random Coefficient Variation.” Econometrica 47 (5): 1287–1294. https://doi.org/10.2307/1911963.
- Cabezas, J., M. Galleguillos, A. Valdés, J. P. Fuentes, C. Pérez, and J. F. Perez-Quezada. 2015. “Evaluation of Impacts of Management in an Anthropogenic Peatland Using Field and Remote Sensing Data.” Ecosphere 6 (12): 1–24. https://doi.org/10.1890/ES15-00232.1.
- Campbell, G. S. 1986. “Extinction Coefficients for Radiation in Plant Canopies Calculated Using an Ellipsoidal Inclination Angle Distribution.” Agricultural and Forest Meteorology 36 (4): 317–321. https://doi.org/10.1016/0168-1923(86)90010-9.
- Crabbe, R. A., D. W. Lamb, C. Edwards, K. Andersson, and D. Schneider. 2019. “A Preliminary Investigation of the Potential of Sentinel-1 Radar to Estimate Pasture Biomass in a Grazed Pasture Landscape.” Remote Sensing 11 (7): 872. https://doi.org/10.3390/rs11070872.
- Cunliffe, A. M., J. J. Assmann, G. Daskalova, J. T. Kerby, and I. H. Myers-Smith. 2020. “Aboveground Biomass Corresponds Strongly with Drone-Derived Canopy Height but Weakly with Greenness (NDVI) in a Shrub Tundra Landscape.” Environmental Research Letters 15 (12): 125004. https://doi.org/10.1088/1748-9326/aba470.
- Czapiewski, S. 2022. “Assessment of the Applicability of UAV for the Creation of Digital Surface Model of a Small Peatland.” Frontiers in Earth Science 10:834923. https://doi.org/10.3389/feart.2022.834923.
- Czapiewski, S., and D. Szumińska. 2021. “An Overview of Remote Sensing Data Applications in Peatland Research Based on Works from the Period 2010–2021.” Land 11 (1): 1–14. https://doi.org/10.3390/land11010024.
- Dayal, K. R., S. Durrieu, S. Alleaume, F. Revers, E. Larmanou, J. P. Renaud, and M. Bouvier. 2020. “Scan Angle Impact on Lidar-Derived Metrics Used in Aba Models for Prediction of Forest Stand Characteristics: A Grid Based Analysis.” The International Archives of the Photogrammetry, Remote Sensing and Spatial Information Sciences 43:975–982. https://doi.org/10.5194/isprs-archives-XLIII-B3-2020-975-2020.
- Fang, H., and S. Liang. 2014. “Leaf Area Index Models”. In Reference Module in Earth Systems and Environmental Sciences, Elsevier reference collections. https://doi.org/10.1016/B978-0-12-409548-9.09076-X.
- FAO. 2020. Peatlands Mapping and Monitoring – Recommendations and Technical Overview. Rome. https://doi.org/10.4060/ca8200en.
- Fox, J., and G. Monette. 1992. “Generalized Collinearity Diagnostics.” Journal of the American Statistical Association 87 (417): 178–183. https://doi.org/10.2307/2290467.
- Gao, T., X. Yang, Y. Jin, H. Ma, J. Li, H. Yu, Q. Yu, X. Zheng, and B. Xu. 2013. “Spatio-Temporal Variation in Vegetation Biomass and Its Relationships with Climate Factors in the Xilingol Grasslands, Northern China.” PLoS ONE 8 (12): e83824. https://doi.org/10.1371/journal.pone.0083824.
- Garisoain, R., C. Delire, B. Decharme, and L. Gandois. 2022. “Seasonality of Sphagnum LAI in a Mountainous Peatland (Pyrenees, France)”. Proceedings of EGU General Assembly 2022, Wien.
- Gerdol, R., and M. Tomaselli. 1984. “Evaluation of Vegetation Classifications from Peatlands in the Dolomites (S-Alps).” Studia Geobotanica 4:25–39. http://hdl.handle.net/10077/14992.
- Gerdol, R., M. Tomaselli, and L. Bragazza. 1994. “A Floristic-Ecologic Classification of Five Mire Sites in the Montane Subalpine Belt of South Tyrol (S Alps, Italy).” Phyton Annales Rei Botanicae 34 (1): 35–56.
- Gilalbert, M., J. González-Piqueras, F. García-Haro, and J. Meliá. 2002. “A Generalized Soil-Adjusted Vegetation Index.” Remote Sensing of Environment 82 (2–3): 303–310. https://doi.org/10.1016/S0034-4257(02)00048-2.
- Gitelson, A. A., and M. N. Merzlyak. 1997. “Remote Estimation of Chlorophyll Content in Higher Plant Leaves.” International Journal of Remote Sensing 18 (12): 2691–2697. https://doi.org/10.1080/014311697217558.
- Houldcroft, C. J., C. L. Campbell, I. J. Davenport, R. J. Gurney, and N. Holden. 2005. “Measurement of Canopy Geometry Characteristics Using LiDar Laser Altimetry: A Feasibility Study.” IEEE Transactions on Geoscience and Remote Sensing 43 (10): 2270–2282. https://doi.org/10.1109/TGRS.2005.856639.
- Huang, S., L. Tang, J. P. Hupy, Y. Wang, and G. Shao. 2021. “A Commentary Review on the Use of Normalized Difference Vegetation Index (NDVI) in the Era of Popular Remote Sensing.” Journal of Forestry Research 32 (1): 1–6. https://doi.org/10.1007/s11676-020-01155-1.
- Huete, A. R., H. Liu, and W. J. D. van Leeuwen. 1997. “The Use of Vegetation Indices in Forested Regions: Issues of Linearity and Saturation”. IEEE International Geoscience and Remote Sensing Symposium Proceedings. Remote Sensing - A Scientific Vision for Sustainable Development, 4: 1966–1968. https://doi.org/10.1109/IGARSS.1997.609169.
- Iglhaut, J., C. Cabo, S. Puliti, L. Piermattei, J. O’Connor, and J. Rosette. 2019. “Structure from Motion Photogrammetry in Forestry: A Review.” Current Forestry Reports 5 (3): 155–168. https://doi.org/10.1007/s40725-019-00094-3.
- Joosten, H., and D. Clarke. 2002. Wise Use of Mires and Peatlands – Background and Principles, Including a Framework for Decision-Making. Saarijärvi: International Mire Conservation Group and International Peat Society.
- Kimmel, K., and Ü. Mander. 2010. “Ecosystem Services of Peatlands: Implications for Restoration.” Progress in Physical Geography 34 (4): 491–514. https://doi.org/10.1177/0309133310365595.
- Lai, Z., Y. Wang, S. Guo, X. Meng, J. Li, W. Li, and S. Han. 2022. “Laser Reflectance Feature Assisted Accurate Extrinsic Calibration for Non-Repetitive Scanning LiDar and Camera Systems.” Optics Express 30 (10): 16242–16263. https://doi.org/10.1364/OE.453449.
- Le Maire, G., C. François, and E. Dufrene. 2004. “Towards Universal Broad Leaf Chlorophyll Indices Using PROSPECT Simulated Database and Hyperspectral Reflectance Measurements.” Remote Sensing of Environment 89 (1): 1–28. https://doi.org/10.1016/j.rse.2003.09.004.
- le Maire, G. C., K. Francois, D. Soudani, J.-Y. Berveiller, N. Pontailler, H. Breda, H. Genet, H. Davi, and E. Dufrêne. 2008. “Calibration and Validation of Hyperspectral Indices for the Estimation of Broadleaved Forest Leaf Chlorophyll Content, Leaf Mass per Area, Leaf Area Index and Leaf Canopy Biomass.” Remote Sensing of Environment 112 (10): 3846–3864. https://doi.org/10.1016/j.rse.2008.06.005.
- Lindsay, R. 2018. ““Peatland (Mire Types): Based on Origin and Behavior of Water, Peat Genesis, Landscape Position, and climate”.” In The Wetland Book (Part II): Distribution, Description and Conservation, edited by C. M. Finlayson, G. R. Milton, R. Prentice, and N. C. Davidson, 251–273. Heidelberg: Springer Netherlands. https://doi.org/10.1007/978-94-007-4001-3_279.
- Liu, J., A. K. Skidmore, S. Jones, T. Wang, M. Heurich, X. Zhu, and Y. Shi. 2018. “Large Off-Nadir Scan Angle of Airborne LiDar Can Severely Affect the Estimates of Forest Structure Metrics.” ISPRS Journal of Photogrammetry and Remote Sensing 136:13–25. https://doi.org/10.1016/j.isprsjprs.2017.12.004.
- Lopatin, J., T. Kattenborn, M. Galleguillos, J. F. Perez-Quezada, and S. Schmidtlein. 2019. “Using Aboveground Vegetation Attributes as Proxies for Mapping Peatland Belowground Carbon Stocks.” Remote Sensing of Environment 231:111217. https://doi.org/10.1016/j.rse.2019.111217.
- Lovell, J. L., D. L. B. Jupp, G. J. Newnham, N. C. Coops, and D. S. Culvenor. 2005. “Simulation Study for Finding Optimal Lidar Acquisition Parameters for Forest Height Retrieval.” Forest Ecology and Management 214 (1–3): 398–412. https://doi.org/10.1016/j.foreco.2004.07.077.
- Lu, D. 2006. “The Potential and Challenge of Remote Sensing‐Based Biomass Estimation.” International Journal of Remote Sensing 27 (7): 1297–1328. https://doi.org/10.1080/01431160500486732.
- Luscombe, D. J., K. Anderson, N. Gatis, A. Wetherelt, E. Grand‐Clement, and R. E. Brazier. 2015. “What Does Airborne LiDar Really Measure in Upland Ecosystems?” Ecohydrology 8 (4): 584–594. https://doi.org/10.1002/eco.1527.
- Maljanen, M., B. D. Sigurdsson, J. Guðmundsson, H. Óskarsson, J. T. Huttunen, and P. J. Martikainen. 2010. “Greenhouse Gas Balances of Managed Peatlands in the Nordic Countries – Present Knowledge and Gaps.” Biogeosciences 7: 2711–2738. https://doi.org/10.5194/bg-7-2711-2010.
- Malone, C. R. 1968. “Determination of Peak Standing Crop Biomass of Herbaceous Shoots by the Harvest Method.” The American Midland Naturalist 79 (2): 429–435. https://doi.org/10.2307/2423188.
- McFeeters, S. K. 1996. “The Use of the Normalized Difference Water Index (NDWI) in the Delineation of Open Water Features.” International Journal of Remote Sensing 17 (7): 1425–1432. https://doi.org/10.1080/01431169608948714.
- McPartland, M. Y., E. S. Kan, M. J. Falkowski, R. Kolka, M. R. Turetsky, B. Palik, and R. A. Montgomery. 2019. “The Response of Boreal Peatland Community Composition and NDVI to Hydrologic Change, Warming, and Elevated Carbon Dioxide.” Global Change Biology 25 (1): 93–107. https://doi.org/10.1111/gcb.14465.
- Meyer, G. E., and J. C. Neto. 2008. “Verification of Color Vegetation Indices for Automated Crop Imaging Applications.” Computers and Electronics in Agriculture 63 (2): 282–293. https://doi.org/10.1016/j.compag.2008.03.009.
- Minasny, B., Ö. Berglund, J. Connolly, C. Hedley, F. de Vries, A. Gimona, B. Kempen, et al. 2019. “Digital Mapping of Peatlands: A Critical Review.” Earth Science Review 196:102870. https://doi.org/10.1016/j.earscirev.2019.05.014.
- Miura, N., T. F. Koyanagi, S. Yokota, and S. Yamada. 2019. “Can UAV LiDar Derive Vertical Structure of Herbaceous Vegetation on Riverdike?” ISPRS Annals of the Photogrammetry, Remote Sensing and Spatial Information Sciences 4:127–132. https://doi.org/10.5194/isprs-annals-IV-2-W5-127-2019.
- Mohammedshum, A. A., and L. Kooistra. 2015. “Mapping Tree Distribution and LAI in Peatlands Using Field Methods and Imaging Spectroscopy: A Case Study for the Haaksbergerveen, the Netherlands.” International Journal of Remote Sensing 36 (18): 4535–4549. https://doi.org/10.1080/01431161.2015.1084064.
- Moore, T. R., J. L. Bubier, S. E. Frolking, P. M. Lafleur, and N. T. Roulet. 2002. “Plant Biomass and Production and CO2 Exchange in an Ombrotrophic Bog.” Journal of Ecology 90 (1): 25–36. https://doi.org/10.1046/j.0022-0477.2001.00633.x.
- Murphy, M. T., A. McKinley, and T. R. Moore. 2009. “Variations in Above-And Below-Ground Vascular Plant Biomass and Water Table on a Temperate Ombrotrophic Peatland.” Botany 87 (9): 845–853. https://doi.org/10.1139/B09-052.
- Navarro, A., M. Young, B. Allan, P. Carnell, P. Macreadie, and D. Ierodiaconou. 2020. “The Application of Unmanned Aerial Vehicles (UAVs) to Estimate Above-Ground Biomass of Mangrove Ecosystems.” Remote Sensing of Environment 242:111747. https://doi.org/10.1016/j.rse.2020.111747.
- Niu, Y., L. Zhang, H. Zhang, W. Han, and X. Peng. 2019. “Estimating Above-Ground Biomass of Maize Using Features Derived from UAV-Based RGB Imagery.” Remote Sensing 11 (11): 1261. https://doi.org/10.3390/rs11111261.
- Pajula, R., and A. H. Purre. 2021. “NDVI as a Proxy to Estimate the CO2 Fluxes in Peatlands: Example of Alkaline Fen.” 16th International Peatland Congress, Virtual Event.
- Palozzi, J., and Z. Lindo. 2017. “Boreal Peat Properties Link to Plant Functional Traits of Ecosystem Engineers.” Plant and Soil 418: 1–15. https://doi.org/10.1007/s11104-017-3291-0.
- Pignatti, S. 1982. Flora d’Italia. Bologna, Italia: Edagricole.
- Pope, R. M., and E. S. Fry. 1997. “Absorption Spectrum (380–700 Nm) of Pure Water. II. Integrating Cavity Measurements.” Applied Optics 36 (33): 8710–8723. https://doi.org/10.1364/AO.36.008710.
- Poto, L., J. Gabrieli, S. J. Crowhurst, P. G. Appleby, P. Ferretti, N. Surian, G. Cozzi, et al. 2013. “The First Continuous Late Glacial–Holocene Peat Bog Multi-Proxy Record from the Dolomites (NE Italian Alps).” Quaternary International 306:71–79. https://doi.org/10.1016/j.quaint.2013.05.001.
- Qin, H., C. Wang, X. Xi, J. Tian, and G. Zhou. 2017. “Simulating the Effects of the Airborne Lidar Scanning Angle, Flying Altitude, and Pulse Density for Forest Foliage Profile Retrieval.” Applied Sciences 7 (7): 712. https://doi.org/10.3390/app7070712.
- Räsänen, A., S. Juutinen, M. Aurela, and T. Virtanen. 2019. “Predicting Aboveground Biomass in Arctic Landscapes Using Very High Spatial Resolution Satellite Imagery and Field Sampling.” International Journal of Remote Sensing 40 (3): 1175–1199. https://doi.org/10.1080/01431161.2018.1524176.
- Räsänen, A., S. Juutinen, M. Kalacska, M. Aurela, P. Heikkinen, K. Mäenpää, A. Rimali, and T. Virtanen. 2020. “Peatland Leaf-Area Index and Biomass Estimation with Ultra-High Resolution Remote Sensing.” GIScience & Remote Sensing 57 (7): 943–964. https://doi.org/10.1080/15481603.2020.1829377.
- Räsänen, A., J. Wagner, G. Hugelius, and T. Virtanen. 2021. “Aboveground Biomass Patterns Across Treeless Northern Landscapes.” International Journal of Remote Sensing 42 (12): 4536–4561. https://doi.org/10.1080/01431161.2021.1897187.
- Rastogi, A., S. Bandopadhyay, M. Strózecki, and R. Juszczak. 2018. “Monitoring the Impact of Environmental Manipulation on Peatland Surface by Simple Remote Sensing Indices”. ITM Web Conferences 23:00030. https://doi.org/10.1051/itmconf/20182300030.
- Rastogi, A., M. Stróżecki, H. M. Kalaji, D. Łuców, M. Lamentowicz, and R. Juszczak. 2019. “Impact of Warming and Reduced Precipitation on Photosynthetic and Remote Sensing Properties of Peatland Vegetation.” Environmental and Experimental Botany 160:71–80. https://doi.org/10.1016/j.envexpbot.2019.01.005.
- Richardson, J. J., L. M. Moskal, and S. H. Kim. 2009. “Modeling Approaches to Estimate Effective Leaf Area Index from Aerial Discrete-Return LIDAR.” Agricultural and Forest Meteorology 149 (6–7): 1152–1160. https://doi.org/10.1016/j.agrformet.2009.02.007.
- Roussel, J.-R., D. Auty, N. C. Coops, P. Tompalski, T. R. H. Goodbody, A. S. Meador, J.-F. Bourdon, F. De Boissieu, and A. Achim. 2020. “lidR: An R Package for Analysis of Airborne Laser Scanning (ALS) Data.” Remote Sensing of Environment 251:112061. https://doi.org/10.1016/j.rse.2020.112061.
- Royston, P. 1982. “Algorithm as 181: The W Test for Normality.” Applied Statistics 31 (2): 176–180. https://doi.org/10.2307/2347986.
- Rydin, H., and J. K. Jeglum. 2006. The Biology of Peatlands. Oxford: Oxford University Press. https://doi.org/10.1093/acprof:oso/9780198528722.001.0001.
- Šimanauskienė, R., R. Linkevičienė, M. Bartold, K. Dąbrowska‐Zielińska, G. Slavinskienė, D. Veteikis, and J. Taminskas. 2019. “Peatland Degradation: The Relationship Between Raised Bog Hydrology and Normalized Difference Vegetation Index.” Ecohydrology 12 (8): e2159. https://doi.org/10.1002/eco.2159.
- Sofonia, J. J., S. Phinn, C. Roelfsema, F. Kendoul, and Y. Rist. 2019. “Modelling the Effects of Fundamental UAV Flight Parameters on LiDar Point Clouds to Facilitate Objectives-Based Planning.” ISPRS Journal of Photogrammetry and Remote Sensing 149:105–118. https://doi.org/10.1016/j.isprsjprs.2019.01.020.
- Steenvoorden, J., H. Bartholomeus, and J. Limpens. 2023. “Less is More: Optimizing Vegetation Mapping in Peatlands Using Unmanned Aerial Vehicles (UAVs).” International Journal of Applied Earth Observation and Geoinformation 117:103220. https://doi.org/10.1016/j.jag.2023.103220.
- Tang, Z., J. Guo, Y. Xiang, X. Lu, Q. Wang, H. Wang, M. Cheng, et al. 2022. “Estimation of Leaf Area Index and Above-Ground Biomass of Winter Wheat Based on Optimal Spectral Index.” Agronomy 12 (7): 1729. https://doi.org/10.3390/agronomy12071729.
- Tanneberger, F., C. Tegetmeyer, S. Busse, A. Barthelmes, S. Shumka, A. Moles Mariné, K. Jenderedjian, et al. 2017. “The Peatland Map of Europe.” Mires and Peat 19:1–17. https://doi.org/10.19189/MaP.2016.OMB.264.
- Tian, L., Y. Qu, and J. Qi. 2021. “Estimation of Forest LAI Using Discrete Airborne LiDar: A Review.” Remote Sensing 13 (12): 2408. https://doi.org/10.3390/rs13122408.
- Tuittila, E.-S., S. Juutinen, S. Frolking, M. Väliranta, A. M. Laine, A. Miettinen, M.-L. Seväkivi, A. Quillet, and P. Merilä. 2013. “Wetland Chronosequence as a Model of Peatland Development: Vegetation Succession, Peat and Carbon Accumulation.” The Holocene 23 (1): 25–35. https://doi.org/10.1177/0959683612450197.
- Turetsky, M. R., B. Benscoter, S. Page, G. Rein, G. R. van der Werf, and A. Watts. 2015. “Global Vulnerability of Peatlands to Fire and Carbon Loss.” Nature Geoscience 8 (1): 11–14. https://doi.org/10.1038/ngeo2325.
- Vescovo, L., and D. Gianelle. 2008. “Using the MIR Bands in Vegetation Indices for the Estimation of Grassland Biophysical Parameters from Satellite Remote Sensing in the Alps Region of Trentino (Italy).” Advances in Space Research 41 (11): 1764–1772. https://doi.org/10.1016/j.asr.2007.07.043.
- Wang, Y., and H. Fang. 2020. “Estimation of LAI with the LiDar Technology: A Review.” Remote Sensing 12 (20): 3457. https://doi.org/10.3390/rs12203457.
- Ward, S. E., R. D. Bardgett, N. P. McNamara, J. K. Adamson, and N. J. Ostle. 2007. “Long-Term Consequences of Grazing and Burning on Northern Peatland Carbon Dynamics.” Ecosystems (New York, N Y) 10 (7): 1069–1083. https://doi.org/10.1007/s10021-007-9080-5.
- Wu, C., Z. Niu, Q. Tang, and W. Huang. 2008. “Estimating Chlorophyll Content from Hyperspectral Vegetation Indices: Modeling and Validation.” Agricultural and Forest Meteorology 148 (8–9): 1230–1241. https://doi.org/10.1016/j.agrformet.2008.03.005.
- Yousefi Lalimi, F., S. Silvestri, L. J. Moore, and M. Marani. 2017. “Coupled Topographic and Vegetation Patterns in Coastal Dunes: Remote Sensing Observations and Ecomorphodynamic Implications.” JGR Biogeosciences 122 (1): 119–130. https://doi.org/10.1002/2016JG003540.
- Zarco-Tejada, P. J., and J. R. Miller. 1999. “Land Cover Mapping at BOREAS Using Red Edge Spectral Parameters from CASI Imagery.” Journal of Geophysical Research: Atmospheres 104 (D22): 27921–27933. https://doi.org/10.1029/1999JD900161.
- Zenmuse L1 User Manual. 2021. https://dl.djicdn.com/downloads/Zenmuse_L1/20210518/Zenmuse_L1%20_User%20Manual_EN_1.pdf.
- Zeybek, M., and İ. Şanlıoğlu. 2019. “Point Cloud Filtering on UAV Based Point Cloud.” Measurement 133:99–111. https://doi.org/10.1016/j.measurement.2018.10.013.
- Zhang, K., S. C. Chen, D. Whitman, M. L. Shyu, J. Yan, and C. Zhang. 2003. “A Progressive Morphological Filter for Removing Nonground Measurements from Airborne LIDAR Data.” IEEE Transactions on Geoscience and Remote Sensing 41 (4): 872–882. https://doi.org/10.1109/TGRS.2003.810682.
- Zhang, W., J. Qi, P. Wan, H. Wang, D. Xie, X. Wang, and G. Yan. 2016. “An Easy-To-Use Airborne LIDAR Data Filtering Method Based on Cloth Simulation.” Remote Sensing 8 (6): 501. https://doi.org/10.3390/rs8060501.
- Zheng, G., and L. M. Moskal. 2009. “Retrieving Leaf Area Index (LAI) Using Remote Sensing: Theories, Methods and Sensors.” Sensors 9 (4): 2719–2745. https://doi.org/10.3390/s90402719.
- Zhu, X., and D. Liu. 2015. “Improving Forest Aboveground Biomass Estimation Using Seasonal Landsat NDVI Time-Series.” ISPRS Journal of Photogrammetry and Remote Sensing 102:222–231. https://doi.org/10.1016/j.isprsjprs.2014.08.014.