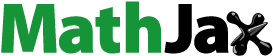
ABSTRACT
As the most commonly used driven data for gross primary productivity (GPP) estimation, satellite remote sensing vegetation indexes (VI), such as the leaf area index (LAI), often seriously suffer from data quality problems induced by cloud contamination and noise. Although various filtering methods are applied to reconstruct the missing data and eliminate noises in the VI time series, the impacts of these data quality problems on GPP estimation are still not clear. In this study, the accuracy differences of the GPP estimations driven by different VI series are comprehensively analyzed based on two light use efficiency (LUE) models (the big-leaf MOD17 and the two-leaf RTL-LUE). Four VI filtering methods are applied for comparison, and GPP data across 169 eddy covariance (EC) sites are used for validation. The results demonstrate that all the filtering methods can improve the GPP simulation accuracy, and the SeasonL1 filtering method exhibits the best performance both for the MOD17 model (∆R2 = 0.06) and the RTL-LUE model (∆R2 = 0.07). The reconstruction of the key change points in the temporally continuous gaps may be the primary reason for the different performance of the four methods. Moreover, the effects of filtering processes on GPP estimation vary with latitudes and seasons due to the differences in the primary data quality. More significant improvements can be observed during the growing season and in the regions near the equator, where the data quality is relatively poor with lower primary GPP estimation accuracy. This study can guide the preprocessing of the VI data before GPP estimation.
1 Introduction
Gross primary productivity (GPP), a measurement of the amount of organic carbon produced by vegetation photosynthesis, stands for the amount of carbon dioxide in the atmosphere fixed by vegetation per unit of space and time (Beer et al. Citation2010; Guan et al. Citation2021; Lin et al. Citation2022). GPP is a crucial indicator for vegetation growth and photosynthesis activity, which is helpful for advancing the understanding of the global carbon cycle. Therefore, accurate estimation of GPP at regional or global scales is particularly important under more and more severe global climate change (Ballantyne et al. Citation2012; Peng et al. Citation2022).
Currently, there are many methods to obtain GPP, which can be broadly classified into two categories: in-situ measurement-based and model-based estimates (Xie and Li Citation2020; Yuan et al. Citation2014). As a mainstream in-situ measurement-based method, the eddy covariance (EC) flux system enables direct measuring of the carbon exchange flux between the vegetation canopy and the atmosphere, thus providing accurate GPP estimates within a specific space around the tower (Baldocchi et al. Citation2001; Pastorello et al. Citation2020). However, the limitation in spatial footprint makes it challenging for large-scale terrestrial ecosystem monitoring (Pastorello et al. Citation2020; Yu et al. Citation2006). Therefore, lots of GPP estimation models have been developed in recent years and act as a feasible approach for regional and global studies. Especially with the development of remote sensing (RS) technology, repeated observation of large-area vegetation canopy can be achieved, and RS-based estimation models are going mainstream. There mainly includes three types: statistic-based models, process-based models, and light use efficiency (LUE) models (Guan et al. Citation2022; Lin et al. Citation2021). These models commonly utilize satellite-based vegetation indices (VI) as driven data, and lots of leaf area index (LAI), normalized difference vegetation index (NDVI), enhanced vegetation index (EVI), and other VIs products have been produced and applied. In this condition, the LUE models, which can be easily combined with RS VI data, have also become the most popular used models for GPP estimation at large scales, and several big-leaf and two-leaf models have been developed (Guan et al. Citation2021; He et al. Citation2013; Running et al. Citation2004).
Nevertheless, satellite-derived VI data is prone to be contaminated by clouds, aerosols, and other adverse atmospheric compositions, resulting in data quality problems like data missing and noise. These contaminations can cause sudden decreases in VI time series, introducing spatiotemporal discontinuity in the data and hindering the accurate representation of vegetation status and changes, which greatly impede the application of VIs (Huete et al. Citation2002; Michishita et al. Citation2014). Since GPP is estimated based on VIs, data quality problems in VIs should also impact the GPP estimations. Therefore, the reconstruction of contaminated information and acquisition of high-quality VI time series is crucial for the precise simulation of GPP (Shen et al. Citation2015), and numerous temporal reconstruction methods have been proposed to generate high-quality VI time series in the past decades. These methods can be generally grouped into three types according to the way they handle the data (Chu et al. Citation2021; Shen et al. Citation2015). The first type is the sliding window filter method, which filters the data within a moving window and the results are highly impacted by the selected filtering parameters (Julien and Sobrino Citation2010; Ma and Veroustraete Citation2006; Savitzky and Golay Citation1964; Viovy, Arino, and Belward Citation1992). The second type is the function-based curve fitting methods that use mathematical functions to fit the entire time-series VI curve, primarily used to obtain the outer envelope of VI time-series data (Beck et al. Citation2006; Jonsson and Eklundh Citation2002; Julien and Sobrino, Citation2019). The third type is the frequency domain method which reconstructs data and removes noise within the frequency domain (Ghafarian Malamiri et al. Citation2020; Lu et al. Citation2007; Verhoef, Menenti, and Azzali Citation1996). There are also some methods that cannot be classified into the aforementioned three categories, such as variation-based filtering methods like Whittaker (Atzberger and Eilers Citation2011) and SeasonL1 (Chu et al. Citation2022). Among them, SeasonL1 combines the regularization of local smoothness and inter-annual seasonal similarity, which can ensure the full utilization of the prior features in the whole time series, and has been demonstrated to outperform several classic filtering methods in reconstructing VI time series (Chu et al. Citation2022).
Although these filtering methods provide abundant tools to preprocess VI data before GPP estimation, the impact of VI quality problems on GPP estimation remains unclear. Since quality problems in VI are often manifested as abrupt decreases, which should also result in an underestimation in GPP, because the main spatiotemporal changes of GPP may largely come from the RS VI data (Yuan et al. Citation2014). If there is a false sudden decrease in VI, there should also be an underestimation of GPP at the same time. Consequently, the intermittent underestimation not only makes it difficult to simulate the continuous changes of GPP over time but also hinders the accurate representation of spatial patterns in large regions. However, although this problem is widely recognized, the precise magnitude of this impact has not been evaluated yet. Data filtering methods have been frequently used in pre-processing to improve the quality of remote sensing data, but there has been little in-depth discussion about the underlying mechanism. The impacts of VI quality improvement on GPP simulation have not been quantified in previous research. Furthermore, most current studies merely select a temporal reconstruction method arbitrarily for processing and application, lacking research on the selection of the specific optimal method for GPP estimation. There also lacks corresponding research on the impact of parameter settings in filtering methods. Therefore, it is necessary to systematically investigate the influence of VI quality issues on GPP estimation and understand the effectiveness of different filtering methods in addressing this problem, providing references for subsequent GPP estimation.
In this context, this study systematically quantifies the impacts of RS index filtering on GPP simulation based on the global EC datasets. The main purposes are: (1) to quantify the impact of VI data quality problems on GPP estimation; (2) to compare the performance of different filtering methods; (3) to compare the sensitivity of the big-leaf and two-leaf models to VI data.
2 Data and methods
2.1 Data
2.1.1 EC data
In this study, EC data from the FLUXNET2015 dataset (www.fluxdata.org) is employed to assess the estimation from different LUE models and different VI inputs (Baldocchi et al. Citation2001). The FLUXNET2015 dataset follows a standardized format and encompasses refined data gathered from various regional networks, covering a total of 212 sites across the globe (Pastorello et al. Citation2017, Citation2020). Consistent with prior studies, GPP partitioned using the daytime partitioning (DT) method (referred to as “GPP_DT_VUT_REF”) served as the reference GPP, which is derived from net ecosystem exchange (NEE) through the application of a hyperbolic light response curve (Reichstein et al. Citation2005). A total of 169 sites (Table S1) with more than one year of valid half-hour observations during the period from 2001 to 2015 are selected. These sites contain 12 vegetation types according to the International Geosphere-Biosphere Program (IGBP) classification system. The number of sites and site-years for each vegetation type are listed in , and the spatial distribution of sites is displayed in .
Table 1. Number of EC sites and site years for different vegetation types in the IGBP classification system.
2.1.2 VI data
The Moderate Resolution Imaging Spectroradiometer (MODIS) VI products provide a consistent and reliable means for monitoring global vegetation conditions (Didan et al. Citation2015). In this study, the MOD15A2H LAI product, provided in an 8-day composition with a spatial resolution of 500 m, is selected to drive LUE models. Moreover, to verify the consistency of results across different indices and enhance their credibility, NDVI and EVI data from the MOD13Q1 product are also selected as the RS-driven data. These two products are synthesized every 16 days and have a spatial resolution of 250 m. These products also include quality assurance (QA) flags that provide information about the quality of the VI products at the pixel level. These data were accessed free from the Land Processes Distributed Active Archive Center (LP DAAC) from the United States Geological Survey (USGS) website on Earth Explorer tool (https://earthexplorer.usgs.gov).
2.2 VI filtering methods
In this study, four commonly used filtering methods are selected to investigate the impact of filtering methods on the simulation accuracy of GPP using LUE models, including Savitzky-Golay (SG), Whittaker, harmonic analysis of time series (HANTS), and SeasonL1.
2.2.1 SG method
The SG method, a representative sliding window filter method, is a local least squares fitting convolution used for smoothing and computing derivatives of a sequence of consecutive values (Chen et al. Citation2004). A description of the SG filter is as follows (Shen et al. Citation2015):
where is the original value of point x acquired at time
,
is the reconstructed value,
is the weight of
, and
is the window length of the convolution that determines not only the smoothness of the results but also the filtering efficiency.
2.2.2 HANTS method
The HANTS method (Verhoef, Menenti, and Azzali Citation1996) is one of the widely used frequency domain filtering methods, its formula can be expressed as (Zhou, Jia, and Menenti Citation2015):
where represents the original series,
represents the reconstructed series, and
represents the error series.
corresponds to the time at which x is obtained (observed), where j = 1, 2, …, N, and N is the maximum number of observations (samples) in the time series. The symbol
is the number of periodic terms in the series, i.e. the number of harmonic components associated with the frequencies
. Generally speaking, these selected harmonics consist of a base frequency and a series of integer multiples of the base frequency.
and
are the coefficients of the trigonometric components with frequencies
.
can be viewed as the coefficient at zero frequency, which is the average of the series. The harmonic fitting can be achieved by solving the equations above using the linear least square method.
2.2.3 Whittaker smoother
The Whittaker filtering method, a commonly used variation-based filtering method (Atzberger and Eilers Citation2011; Eilers Citation2003), employs the second-order difference of the time series to obtain smooth results. In particular, when the second-order difference approaches 0, it indicates temporal smoothness. As a result, a regularization term is formulated, leading to the creation of the following filtering model.
where represents the reconstructed series and
represents the original series.
is a regularization parameter that controls the contributions of local smoothness.
2.2.4 SeasonL1 method
The SeasonL1 filtering method is developed based on the Whittaker filtering method, by considering the inter-annual seasonal similarity of VI time series data. This method has been demonstrated to offer significant advantages in terms of recovering temporally contiguous missing values and preventing over-smoothing in the inflection points of vegetation growth (Chu et al. Citation2022). The SeasonL1 model can be described as:
where represents the weight of the corresponding VI point determined by data quality, while
is the regularization parameter that controls the contributions of inter-annual seasonal similarity,
indicates the length of the one-year time series. This formula consists of three terms: the data fidelity term, the temporal smoothness regularization term, and the inter-annual similarity regularization term, which are respectively used to reduce the deviation between x and y, capture the temporal patterns inherent in VI time series data, and the non-local similarity patterns across multiple years in VI time series.
2.3 GPP estimation models
Current LUE models can be typically divided into big-leaf and two-leaf categories. As a result, this study selects the MOD17 model to represent the big-leaf models (Running et al. Citation2004) and the RTL-LUE model to represent the two-leaf models (Guan et al. Citation2021), to analyze whether the VI filtering effects are stable among different types of LUE models.
2.3.1 MOD17 model
The MOD17 model was proposed based on the radiation conversion efficiency concept of Monteith (1972). It can be described as (Running et al. Citation2004):
where is the maximum LUE varied with different vegetation types;
is the fraction of photosynthetically active radiation (PAR) absorbed by the canopy;
and
are the scalars of vapor pressure deficit (VPD) and minimum temperature.
where k is the light extinction coefficient and set as 0.5; VPD is the daily average VPD at day- time and Ta is the daily minimum temperature; VPDmax, VPDmin, Tmax, and Tmin are parameters specific to vegetation types.
2.3.2 RTL-LUE model
Considering the significant differences in the photosynthetically active radiation (APAR) and LUE of sunlit and shaded leaves (Propastin et al. Citation2012; Wang et al. Citation2015), two-leaf models are developed to improve the GPP estimations. The TL-LUE model is the first two-leaf model (Chen et al. Citation2020; He et al. Citation2018; Liu et al. Citation1997), and then, the RTL-LUE model is modified taking the impacts of radiation intensity on LUE into account. The GPP of a plant canopy is calculated as follows:
where represents the maximum LUE observed across all the leaves within the canopy. The scalar of radiation on vegetation photosynthesis in sunlit and shaded leaves is denoted by
and
, respectively.
and
are calculated using the same method as in the MOD17 algorithm.
and
indicate the incoming PAR absorbed by sunlight and shaded leaves, and are computed using the following formulas:
where represents the canopy albedo that is associated with specific vegetation types. Meanwhile,
corresponds to the mean leaf-sun angle and is set to 60 degrees for a canopy with a spherical leaf angle distribution according to previous research and empirical knowledge (Chen Citation1996, Citation1999; Guan et al. Citation2021; He et al. Citation2013; Zhou et al. Citation2016).
represents the solar zenith angle. Additionally,
,
,
, and
denote the diffuse and direct components of incoming PAR, the diffuse PAR under the canopy, and the multiple scattering of direct radiation (Chen Citation1999).
and
indicate the LAI of sunlit and shaded leaves, respectively, which are separated based on the canopy LAI, clumping index (Ω), and solar zenith angle.
2.4 Model evaluation
The parameters VPDmax, VPDmin, Tmax, Tmin, α, Ω, εmax and a are empirically set in LUE models according to the previous literature (Guan et al. Citation2021, Citation2022; He et al. Citation2013; Zhou et al. Citation2016), which are listed in Table S2. To assess the accuracy of the GPP simulations from the two models against the EC GPP, the coefficient of determination (R2) and the root-mean-square error (RMSE) are used for quantitative evaluation:
where N is the total simulated experiment data point; and
represent the predicted GPP from the MOD17 or RTL-LUE models and observed EC GPP, respectively; and
and
are the mean values of observations and predictions for all experimental data points.
3 Results
This section firstly evaluates the accuracy of GPP simulations calculated by different VI data at all 169 sites and analyzed after grouping them into different vegetation types. Then the differences across different LUE models and VI filtering methods are compared, and the spatial and temporal heterogeneities are further investigated along latitude and season.
3.1 Validation in all sites
The accuracies of the 8-day GPP simulation result using the raw LAI and the four filtering results are evaluated using EC GPP data from 169 sites worldwide. provides the density scatterplot of all data points with different filtering methods. It can be observed that all four filtering methods can improve the accuracy of GPP simulation from both the MOD17 and the RTL-LUE models. When comparing the accuracy within the same model, the utilization of filtered VIs leads to higher R2, lower RMSE, and a fitted slope approaching 1, which suggests that four filtering methods can improve the accuracy of LUE-GPP simulations. Due to the deterministic nature of the model, which is hard to conduct significance testing for the R2 changes between different models (Casella and Fienberg Citation2009), we only test the significance of R2 for each model. The results indicate all the results passed the significant test with p < 0.05. Among the four filtering methods, SeasonL1 filtering exhibits the most notable enhancement in simulation accuracy (∆R2 is 0.06 in MOD17 and 0.07 in RTL-LUE), indicating it is more effective in mitigating GPP underestimation caused by VI data quality. Furthermore, the slope of the MOD17 model is generally lower than that of the RTL-LUE model, indicating a more severe underestimating tendency in the MOD17 model, which has been demonstrated to be due to inadequate consideration of the contribution of shaded leaves (He et al. Citation2018; Zhang et al. Citation2012).
3.2 Validation in different vegetation types
3.2.1 Quantitative accuracy comparison
and present the R2 and RMSE between EC GPP and GPP estimations for different vegetation types. It can be observed in that the GPP simulation accuracy improves for all vegetation types after using filtering VIs, with a higher R2 and lower RMSE compared to the results using raw LAI. Within the MOD17 model, CSH sites show the most improvement in R2, reaching 0.171, while the sites with vegetation type EBF experience the greatest reduction in RMSE, with a decrease of 5.405 g C m−2 8day−1. There are seven vegetation types that show an R2 improvement exceeding 0.05, and six vegetation types that show an RMSE reduction exceeding 1 g C m−2 8day−1. Various filtering methods have different performances in different vegetation types. The SeasonL1 method exhibits the highest R2 for all the 12 vegetation types with an average value of 0.597, whereas the R2 for SG, Whittaker, and HANTS methods are 0.561, 0.577, and 0.581, respectively. Additionally, the RMSE from the SeasonL1 method is also lower in most vegetation types, with a mean value of 16.440 g C m−2 8 day−1, while they are 16.579, 16.746 and 16.462 g C m−2 8 day−1 for SG, Whittaker, and HANTS methods, respectively. These results suggest that the SeasonL1 method outperforms the other three classical filtering methods in improving the GPP simulation of the MOD17 model in different vegetation types.
Table 2. R2 and RMSE of different vegetation types acquired from MOD17.
Table 3. R2 and RMSE of different vegetation types acquired from RTL-LUE.
The same conclusion can also be found for RTL-LUE models. As shown in , the largest R2 increase within the RTL-LUE model is 0.299 observed in the CSH type, and the largest reduction in RMSE is 7.373 g C m−2 8 day−1 observed in the EBF type, indicating the RTL-LUE model exhibits a greater accuracy improvement compared to the MOD17 model. When comparing the results for different filtering methods, the SeasonL1 method also demonstrates higher R2 and lower RMSE in almost all the vegetation types, than that of the other three filtering methods. As a result, it indicates that the GPP estimation can be improved in different vegetation types both for big-leaf and two-leaf models after filtering methods enhance the quality of VI data, and the SeasonaL1 method performs the best in almost all vegetation types.
3.2.2 Qualitative interannual variations
To further investigate how filtering methods improve the accuracy of GPP simulation, seasonal variation curves of GPP and their corresponding LAI time series from representative site years are drawn for qualitative assessment, as shown in and 4. Only the results of the filtering method with the best performance, i.e, SeasonL1, are provided to avoid confusion caused by too many data. It can be observed that there are synchronies between the sudden decrease in the raw LAI time series and the underestimation in the corresponding GPP sequence, which is mainly attributed to cloud contamination in the RS-driven data. However, the underestimations of GPP are effectively suppressed when using the reconstructed LAI time series. The results are consistent both for the MOD17 and the RTL-LUE model. The application of the SeasonL1 method mitigates the underestimation of GPP by reconstructing the LAI time series, resulting in GPP simulations being closer to EC GPP because the unreasonable sudden estimations are well corrected. Although the overall tendency of the GPP estimations is similar when using raw LAI or filtered results, the quantitative indexes can be much better for the latter. Furthermore, reveal that the interannual variations of the RTL-LUE model results exhibit a closer resemblance to those of EC, in contrast to the MOD17 model. It can be observed that there is also a discrepancy between the EC GPP and the model estimations, either using raw LAI or filtered data. This discrepancy could be caused by various sources of uncertainties in the model structure and vegetation index data obtained from satellites, such as observation errors, sensor degeneration, and spatial heterogeneity (Fensholt and Proud Citation2012; Ruppert and Linstädter Citation2014).
Figure 3. Intra-annual variation of EC GPP and GPP simulated by the MOD17 model for different vegetation types. Sites in the figure are selected to represent the 12 vegetation types in the IGBP classification system and the detailed location information is provided in table S1. The title of each subplot is named “vegetation types/site year/site name,” DOY means the day of the year. For each subplot, the upper color figure is the variation of GPP, and the under gray figure is the variation of LAI.

Figure 4. Intra-annual variation of EC GPP and GPP simulated by the RTL-LUE model for different vegetation types. The detailed location information is provided in table S1. The title of each subplot is named “vegetation types/site year/site name,” DOY means the day of the year. For each subplot, the upper color figure is the variation of GPP, and the under gray figure is the variation of LAI.

3.3 Spatial and temporal heterogeneity
3.3.1 Variation along latitude
Since the data quality of VI may vary along space and time, its impacts on GPP simulation should also show spatial and temporal heterogeneities. To further explore the spatial patterns of VI reconstruction effects on GPP estimation, all the EC sites are divided into six groups according to their latitudes (i.e. 20 ~ 40°S, 0 ~ 20°S, 0 ~ 20°N, 20 ~ 40°N, 40 ~ 60°S, >60°S), and the improvements of GPP simulation accuracy (ΔR2, ΔRMSE) along different latitude zones are shown in . The number in each grid denotes the R2 and RMSE of GPP simulation using different LAIs, and the color in the right four grids denotes the improvements of using filtered LAI compared with the raw LAI results. It can be observed that the sites located between 20°N and 20°S show the greatest improvement in GPP simulation accuracy after filtering among the six latitude groups, which suggests that the filtering process is more important in low latitude regions around the equator. The color variations also indicate an uneven accuracy change across different latitude zones, demonstrating the spatial heterogeneity of the filtering impacts on the VI data-driven LUE GPP model. Furthermore, as illustrated by the numbers in , the simulation accuracy at low latitudes is lower than that at high latitudes in both the MOD17 and RTL-LUE models, which is mainly due to the higher frequency of overcast and cloudy days in low-latitude regions, leading to reduced reliability of satellite VI data. In addition, due to the neglect of diffuse PAR in in the MOD17 model (Yuan et al. Citation2014), the RTL-LUE model also shows an obvious increase in R2 and a decrease in RMSE along all latitude zones (Guan et al. Citation2021).
3.3.2 Accuracy differences in different seasons
The quality of VI data also varies in different seasons due to differences in cloudy and rainy weather. As the vegetation above 20°N is considered to exhibit strong seasonality in productivity, the sites in these regions are selected, and the GPP simulation accuracies are compared in the growing and dormant seasons. In general, the growing season for plants in the Northern Hemisphere is roughly considered to be from May to October, and the other six months are the dormant season. As shown in , GPP simulations from the two models both exhibit lower R2 and higher RMSE during the growing season compared to the dormant season, which can be attributed to the increased frequency of overcast and cloudy days in this period. As a result, more severe cloud cover effects on the VI data are acquired by remote sensing satellites. Furthermore, the filtering process also improves the GPP simulation accuracy more during the growing season, because it can correct more unreasonable slips. Among them, the SeasonL1 method still performs the best in the RTL-LUE model, with an R2 improvement of 0.11 and an RMSE reduction of 3.11 g C m−2 8 day−1 during the growing season, and the value is 0.07 and 0.85 g C m−2 8 day−1 for the dormant season. This may be attributed to the lower quality of the LAI time series in the growing season, which provides greater potential to improve.
4 Discussion
4.1 Impact of NDVI and EVI data quality on LUE-GPP estimation
Except for LAI, remote sensing spectral vegetation indices such as NDVI and EVI are also widely used to calculate the fPAR and drive the big-leaf LUE models (Prince and Goward Citation1995; Running et al. Citation1999; Xiao et al. Citation2004; Yuan et al. Citation2007), the related equations are shown in Table S3. To further validate whether the obtained results are consistent among different remote sensing indices, the GPP simulation accuracy differences from the big-leaf LUE model using raw and filtered NDVI and EVI are also compared, to enhance the robustness and reliability of results. Due to the NDVI and EVI products being provided with a time interval of 16 days, the 8-day LAI time series are also synthesized into 16-day intervals using the maximum-value composite (MVC) method (Holben Citation1986). presents the GPP simulation accuracy driven by three different remote sensing VI time series, as well as their results using raw data and filtered results. It can be observed that the accuracy using 16-day LAI is higher than that of 8-day LAI in the MOD17 model (Section 3.1), which may be attributed to the MVC method partially mitigating the contamination of remote sensing data (Holben Citation1986). The GPP simulation accuracies are all improved after using the filtered data for the three VIs, it indicates the importance of the filtering process. However, huge differences are found among the three VIs, with the greatest improvements being found for EVI, and NDVI demonstrating more stable results. Additionally, although the four filtering methods show various performances in three VIs, the SeasonL1 method still exhibits the best improvements. After the process using SeasonL1, the R2 for NDVI-driven, EVI-driven, and LAI-driven simulations are increased by 0.03, 0.08, and 0.05, respectively. The results suggest that, in addition to the LAI time series, filtering methods also contribute to similar improvements in the data quality of NDVI and EVI.
Table 4. Statistic of the 16-day GPP simulation from the MOD17 model driven by NDVI and EVI.
4.2 What makes SeasonL1 the most effective?
The results indicate that the process of data filtering can improve the GPP estimation accuracy to a certain extent (∆R2 ~0.07), which is even higher than the improvement by changing the model structure from big leaf to two leaf (∆R2 ~0.06). It proves that filtering methods can indeed influence the simulation accuracy of the LUE-GPP model by improving the quality of VI data. Among the four filtering methods, SeasonL1 performs the best in improving the accuracy of LUE-GPP simulations, which warrants further investigation. Previous studies have shown that traditional temporal filtering methods do not consider the prior characteristics of the VI time series and perform poorly in cases of continuous time gaps (Atkinson et al. Citation2012; Chu et al. Citation2021). However, the SeasonL1 method innovatively introduces the information from adjacent years combined with assistance from temporal neighbors, and the L1-norm is imposed to establish the two corresponding regularization terms and thus can better characterize their statistical distributions (Chu et al. Citation2022). displays the comparison of interannual NDVI time series between the SeasonL1 method and the SG method. It can be observed that the SG method fails to reconstruct the deep valley during vegetation senescence, while the SeasonL1 method successfully reconstructs the real NDVI trajectory, which is in good agreement with the original time series. The satisfactory and stable performance of the SeasonL1 method in addressing consecutive gaps is mainly because the newly employed inter-annual similarity term introduces valid information from adjacent years. Compared with the other three typical filters, the SeasonL1 filter shows obvious advantages in recovering the time-continuous missing values of vegetation growth inflection points and preventing over-smoothing, which can extend the ability of the temporal methods in advancing the quality of VI time-series data. Thus, using the filtering results from the SeasonL1 method to simulate GPP can certainly obtain higher accuracy than the other methods, with much better agreements in the temporal continuous missing areas.
4.3 Impact of filtering parameter settings on LUE-GPP estimation
Parameters are important for all the VI filtering methods. Current research lacks a discussion on the parameters of filtering methods, and the impact of filtering parameter settings on their effectiveness is still unclear. To further investigate the impact of the filtering parameter on GPP reconstruction results, we selected the SeasonL1 method that shows the best performances in previous results and analyzed the GPP simulation accuracy (R2) changed with the smoothness parameter using the RTL-LUE model. As shown in , R2 first increases and then decreases with the increasing smoothness parameter, while RMSE is the opposite, which is a common feature for all the smoothing algorithms. The two lines both reach the optimal value around a smoothness parameter of 0.13. The results suggest that the correlation between filter smoothness and GPP reconstruction accuracy is not always positive, and there exists a range where optimal reconstruction accuracy can be achieved. The accuracy will drop beyond this range, which has important implications for the selection and application of filters in subsequent research on terrestrial ecosystem productivity estimation. Therefore, it is necessary to determine the optimal parameter range for GPP simulation.
5 Conclusion
This study has investigated the impacts of the VI data quality on GPP estimation by comparing the GPP simulation results of two LUE models (MOD17 and RTL-LUE), using raw VI data and the results from four data filtering methods (SG, Whittaker, HANTS, and SeasonL1). The main findings are:
All four filtering methods can improve the accuracy of GPP estimation both using the big-leaf LUE model (∆R2 = 0.06) and the two-leaf LUE model (∆R2 = 0.07) for most vegetation types. The improvements by VI data filtering are even more significant than the change of model structure from big-leaf to two-leaf.
The SeasonL1 method outperforms the other three classical filtering methods in improving the GPP simulations using both the two LUE models, because it can effectively recover the missing values and noise in VI data and preserve the key change points of vegetation growth in temporal continuous missing conditions.
The improvements in GPP simulation accuracy impacted by VI reconstruction vary across different spatial and temporal contexts. The improvements in GPP estimation accuracy are more pronounced for low latitude regions (20 °S ~ 20 °N) and the vegetation growing seasons.
Although our study provides useful insights for future studies related to modeling and forecasting GPP using LUE models, there are also some limitations. Only 169 sites are applied to investigate the impacts of the VI data quality on GPP simulation, which is insufficient to reveal the distribution of these impacts on a global scale. Therefore, it is important to evaluate the quality and uncertainty of VI data and their impacts on GPP estimation at a global scale using multiple approaches and datasets. Furthermore, even concerning previous research parameters, the uncertainty of LUE model parameters may affect the accuracy of the MOD17 model and RTL-LUE model. In addition to the choice of filtering method, the uncertainty in filter smoothing settings can also influence the performance of filtering and, consequently, affect the simulation accuracy of the LUE models. Future research could address these issues and explore more advanced filtering methods and models for better GPP simulation.
Supplemental Material
Download MS Word (81.5 KB)Disclosure statement
The authors declare that they have no known competing financial interests or personal relationships that could have appeared to influence the work reported in this paper.
Data availability statement
The authors acknowledge NASA for free access to MODIS VI products and the FLUXNET community for providing the EC data. The VI data are accessed free from the Earth Explorer tool from the United States Geological Survey (USGS) website (https://earthexplorer.usgs.gov). All the flux and meteorological data are available from the website (https://fluxnet.org/data/fluxnet2015-dataset/), which are acquired and shared by global collaborated regional networks supporting the FLUXNET.
Supplementary material
Supplemental data for this article can be accessed online at https://doi.org/10.1080/15481603.2023.2275421.
Additional information
Funding
References
- Atkinson, P. M., C. Jeganathan, J. Dash, and C. Atzberger. 2012. “Inter-Comparison of Four Models for Smoothing Satellite Sensor Time-Series Data to Estimate Vegetation Phenology.” Remote Sensing of Environment 123:400–18. https://doi.org/10.1016/j.rse.2012.04.001.
- Atzberger, C., and P. H. C. Eilers. 2011. “A Time Series for Monitoring Vegetation Activity and Phenology at 10-Daily Time Steps Covering Large Parts of South America.” International Journal of Digital Earth 4 (5): 365–386. https://doi.org/10.1080/17538947.2010.505664.
- Baldocchi, D., E. Falge, L. Gu, R. Olson, D. Hollinger, S. Running, P. Anthoni, C. Bernhofer, K. Davis, and R. Evans. 2001. “FLUXNET: A New Tool to Study the Temporal and Spatial Variability of Ecosystem–Scale Carbon Dioxide, Water Vapor, and Energy Flux Densities.” Bulletin of the American Meteorological Society 82 (11): 2415–2434. https://doi.org/10.1175/1520-0477(2001)082<2415:FANTTS>2.3.CO;2.
- Ballantyne, A. P., C. B. Alden, J. B. Miller, P. P. Tans, and J. W. C. White. 2012. “Increase in Observed Net Carbon Dioxide Uptake by Land and Oceans During the Past 50 Years.” Nature 488 (7409): 70–72. https://doi.org/10.1038/nature11299.
- Beck, P. S., C. Atzberger, K. A. Høgda, B. Johansen, and A. K. Skidmore. 2006. “Improved Monitoring of Vegetation Dynamics at Very High Latitudes: A New Method Using MODIS NDVI.” Remote Sensing of Environment 100 (3): 321–334. https://doi.org/10.1016/j.rse.2005.10.021.
- Beer, C., M. Reichstein, E. Tomelleri, P. Ciais, M. Jung, N. Carvalhais, C. Rödenbeck, et al. 2010. “Terrestrial Gross Carbon Dioxide Uptake: Global Distribution and Covariation with Climate.” Science 329 (5993): 834–838. https://doi.org/10.1126/science.1184984.
- Casella, G., and S. Fienberg. 2009. “Statistics Texts in Statistics.”
- Chen, J. M. 1996. “Canopy Architecture and Remote Sensing of the Fraction of Photosynthetically Active Radiation Absorbed by Boreal Conifer Forests.” IEEE Transactions on Geoscience and Remote Sensing 34 (6): 1353–1368. https://doi.org/10.1109/36.544559.
- Chen, J. M. 1999. “Spatial Scaling of a Remotely Sensed Surface Parameter by Contexture.” Remote Sensing of Environment 69 (1): 30–42. https://doi.org/10.1016/S0034-4257(99)00006-1.
- Chen, B., M. A. Arain, J. M. Chen, S. Q. Wang, H. L. Fang, Z. H. Liu, G. Mo, and J. Liu. 2020. “Importance of Shaded Leaf Contribution to the Total GPP of Canadian Terrestrial Ecosystems: Evaluation of MODIS GPP.” Journal of Geophysical Research-Biogeosciences 125 (10): 125. https://doi.org/10.1029/2020JG005917.
- Chen, J., P. Jonsson, M. Tamura, Z. H. Gu, B. Matsushita, and L. Eklundh. 2004. “A Simple Method for Reconstructing a High-Quality NDVI Time-Series Data Set Based on the Savitzky–Golay Filter.” REMOTE SENSING of ENVIRONMENT 91 (3–4): 332–344. https://doi.org/10.1016/j.rse.2004.03.014.
- Chu, D., H. F. Shen, X. B. Guan, J. M. Chen, X. H. Li, J. Li, and L. P. Zhang. 2021. “Long Time-Series NDVI Reconstruction in Cloud-Prone Regions via Spatio-Temporal Tensor Completion.” Remote Sensing of Environment 264:112632. https://doi.org/10.1016/j.rse.2021.112632.
- Chu, D., H. F. Shen, X. B. Guan, and X. H. Li. 2022. “An L1-Regularized Variational Approach for NDVI Time-Series Reconstruction Considering Inter-Annual Seasonal Similarity.” International Journal of Applied Earth Observation and Geoinformation 114:114. https://doi.org/10.1016/j.jag.2022.103021.
- Didan, K., A. B. Munoz, R. Solano, and A. Huete. 2015. MODIS Vegetation Index User’s Guide, 35. MOD13 series ed. University of Arizona: Vegetation Index and Phenology Lab.
- Eilers, P. H. 2003. “A Perfect Smoother.” Analytical Chemistry 75 (14): 3631–3636. https://doi.org/10.1021/ac034173t.
- Fensholt, R., and S. R. Proud. 2012. “Evaluation of Earth Observation Based Global Long Term Vegetation Trends—Comparing GIMMS and MODIS Global NDVI Time Series.” Remote Sensing of Environment 119:131–147. https://doi.org/10.1016/j.rse.2011.12.015.
- Ghafarian Malamiri, H. R., H. Zare, I. Rousta, H. Olafsson, E. Izquierdo Verdiguier, H. Zhang, and T. D. Mushore. 2020. “Comparison of Harmonic Analysis of Time Series (HANTS) and Multi-Singular Spectrum Analysis (M-SSA) in Reconstruction of Long-Gap Missing Data in NDVI Time Series.” Remote Sensing 12 (17): 2747. https://doi.org/10.3390/rs12172747.
- Guan, X., J. M. Chen, H. Shen, and X. Xie. 2021. “A Modified Two-Leaf Light Use Efficiency Model for Improving the Simulation of GPP Using a Radiation Scalar.” Agricultural and Forest Meteorology 307:108546. https://doi.org/10.1016/j.agrformet.2021.108546.
- Guan, X., J. M. Chen, H. Shen, X. Xie, and J. Tan. 2022. “Comparison of Big-Leaf and Two-Leaf Light Use Efficiency Models for GPP Simulation After Considering a Radiation Scalar.” Agricultural and Forest Meteorology 313:108761. https://doi.org/10.1016/j.agrformet.2021.108761.
- He, L., J. M. Chen, A. Gonsamo, X. Luo, R. Wang, Y. Liu, and R. Liu. 2018. “Changes in the Shadow: The Shifting Role of Shaded Leaves in Global Carbon and Water Cycles Under Climate Change.” Geophysical Research Letters 45 (10): 5052–5061. https://doi.org/10.1029/2018GL077560.
- He, M., W. Ju, Y. Zhou, J. Chen, H. He, S. Wang, H. Wang, et al. 2013. “Development of a Two-Leaf Light Use Efficiency Model for Improving the Calculation of Terrestrial Gross Primary Productivity.” Agricultural and Forest Meteorology 173:28–39. https://doi.org/10.1016/j.agrformet.2013.01.003.
- Holben, B. N. 1986. “Characteristics of Maximum-Value Composite Images from Temporal AVHRR Data.” International Journal of Remote Sensing 7 (11): 1417–1434. https://doi.org/10.1080/01431168608948945.
- Huete, A., K. Didan, T. Miura, E. P. Rodriguez, X. Gao, and L. G. Ferreira. 2002. “Overview of the Radiometric and Biophysical Performance of the MODIS Vegetation Indices.” Remote Sensing of Environment 83 (1–2): 195–213. https://doi.org/10.1016/S0034-4257(02)00096-2.
- Jonsson, P., and L. Eklundh. 2002. “Seasonality Extraction by Function Fitting to Time-Series of Satellite Sensor Data.” IEEE Transactions on Geoscience and Remote Sensing 40 (8): 1824–1832. https://doi.org/10.1109/TGRS.2002.802519.
- Julien, Y., and J. A. Sobrino. 2010. “Comparison of Cloud-Reconstruction Methods for Time Series of Composite NDVI Data.” Remote Sensing of Environment 114 (3): 618–625. https://doi.org/10.1016/j.rse.2009.11.001.
- Julien, Y, and Sobrino, J. A. (2019).“Optimizing and comparing gap-filling techniques using simulated NDVI time series from remotely sensed global data” International Journal of Applied Earth Observation and Geoinformation, 76: 93–111. https://doi.org/10.1016/j.jag.2018.11.008.
- Lin, S., D. L. Hao, Y. Zheng, H. Zhang, C. Wang, and W. P. Yuan. 2022. “Multi-Site Assessment of the Potential of Fine Resolution Red-Edge Vegetation Indices for Estimating Gross Primary Production.” International Journal of Applied Earth Observation and Geoinformation 113:102978. https://doi.org/10.1016/j.jag.2022.102978.
- Lin, S., J. Li, Q. H. Liu, B. Gioli, E. Paul-Limoges, N. Buchmann, M. Gharun et al. 2021. “Improved Global Estimations of Gross Primary Productivity of Natural Vegetation Types by Incorporating Plant Functional Type.” International Journal of Applied Earth Observation and Geoinformation 100:102328. https://doi.org/10.1016/j.jag.2021.102328.
- Liu, J., J. M. Chen, J. Cihlar, and W. M. Park. 1997. “A Process-Based Boreal Ecosystem Productivity Simulator Using Remote Sensing Inputs.” Remote Sensing of Environment 62 (2): 158–175. https://doi.org/10.1016/S0034-4257(97)00089-8.
- Lu, X., R. Liu, J. Liu, and S. Liang. 2007. “Removal of Noise by Wavelet Method to Generate High Quality Temporal Data of Terrestrial MODIS Products.” Photogrammetric Engineering & Remote Sensing 73 (10): 1129–1139. https://doi.org/10.14358/PERS.73.10.1129.
- Ma, M., and F. Veroustraete. 2006. “Reconstructing Pathfinder AVHRR Land NDVI Time-Series Data for the Northwest of China.” Advances in Space Research 37 (4): 835–840. https://doi.org/10.1016/j.asr.2005.08.037.
- Michishita, R., Z. Jin, J. Chen, and B. Xu. 2014. “Empirical Comparison of Noise Reduction Techniques for NDVI Time-Series Based on a New Measure.” ISPRS Journal of Photogrammetry and Remote Sensing 91:17–28. https://doi.org/10.1016/j.isprsjprs.2014.01.003.
- Pastorello, G., D. Papale, H. Chu, C. Trotta, D. Agarwal, E. Canfora, D. Baldocchi, and M. Torn. 2017. “A New Data Set to Keep a Sharper Eye on Land-Air Exchanges.” Eos, Transactions American Geophysical Union (Online) 98. https://doi.org/10.1029/2017EO071597.
- Pastorello, G., C. Trotta, E. Canfora, H. Chu, D. Christianson, Y.-W. Cheah, C. Poindexter, J. Chen, A. Elbashandy, and M. Humphrey. 2020. “The FLUXNET2015 Dataset and the ONEFlux Processing Pipeline for Eddy Covariance Data.” Scientific Data 7 (1): 1–27. https://doi.org/10.1038/s41597-020-0534-3.
- Peng, S., B. Fu, Y. Lai, J. Li, and B. Li. 2022. “Uncertainty Analysis of Gross Primary Productivity Estimates Based on a Light Use Efficiency Meta-Model.” Beijing da Xue Xue Bao Zhe Xue She Hui Ke Xue Ban 58 (2): 361–371. https://doi.org/10.13209/j.0479-8023.2022.003.
- Prince, S. D., and S. N. Goward. 1995. “Global Primary Production: A Remote Sensing Approach.” Journal of Biogeography 22 (4/5): 815–835. https://doi.org/10.2307/2845983.
- Propastin, P., A. Ibrom, A. Knohl, and S. Erasmi. 2012. “Effects of Canopy Photosynthesis Saturation on the Estimation of Gross Primary Productivity from MODIS Data in a Tropical Forest.” Remote Sensing of Environment 121:252–260. https://doi.org/10.1016/j.rse.2012.02.005.
- Reichstein, M., E. Falge, D. Baldocchi, D. Papale, M. Aubinet, P. Berbigier, C. Bernhofer, et al. 2005. “On the Separation of Net Ecosystem Exchange into Assimilation and Ecosystem Respiration: Review and Improved Algorithm.” Global Change Biology 11 (9): 1424–1439. https://doi.org/10.1111/j.1365-2486.2005.001002.x.
- Running, S. W., R. Nemani, J. M. Glassy, and P. E. Thornton. 1999. “MODIS Daily Photosynthesis (PSN) and Annual Net Primary Production (NPP) Product (MOD17) Algorithm Theoretical Basis Document.” 679–706.
- Running, S. W., R. R. Nemani, F. A. Heinsch, M. S. Zhao, M. Reeves, and H. Hashimoto. 2004. “A Continuous Satellite-Derived Measure of Global Terrestrial Primary Production.” Bioscience 54 (6): 547–560. https://doi.org/10.1641/0006-3568(2004)054[0547:ACSMOG]2.0.CO;2.
- Ruppert, J. C., and A. Linstädter. 2014. “Convergence Between ANPP Estimation Methods in Grasslands—A Practical Solution to the Comparability Dilemma.” Ecological Indicators 36:524–531. https://doi.org/10.1016/j.ecolind.2013.09.008.
- Savitzky, A., and M. J. Golay. 1964. “Smoothing and Differentiation of Data by Simplified Least Squares Procedures.” Analytical Chemistry 36 (8): 1627–1639. https://doi.org/10.1021/ac60214a047.
- Shen, H., X. Li, Q. Cheng, C. Zeng, G. Yang, H. Li, and L. Zhang. 2015. “Missing Information Reconstruction of Remote Sensing Data: A Technical Review.” IEEE Geoscience and Remote Sensing Magazine 3 (3): 61–85. https://doi.org/10.1109/MGRS.2015.2441912.
- Verhoef, W., M. Menenti, and S. Azzali. 1996. “Cover a Colour Composite of NOAA-AVHRR-NDVI Based on Time Series Analysis (1981-1992).” International Journal of Remote Sensing 17 (2): 231–235. https://doi.org/10.1080/01431169608949001.
- Viovy, N., O. Arino, and A. Belward. 1992. “The Best Index Slope Extraction (BISE): A Method for Reducing Noise in NDVI Time-Series.” International Journal of Remote Sensing 13 (8): 1585–1590. https://doi.org/10.1080/01431169208904212.
- Wang, S., K. Huang, H. Yan, H. Yan, L. Zhou, H. Wang, J. Zhang, J. Yan, L. Zhao, and Y. Wang. 2015. “Improving the Light Use Efficiency Model for Simulating Terrestrial Vegetation Gross Primary Production by the Inclusion of Diffuse Radiation Across Ecosystems in China.” Ecological Complexity 23:1–13. https://doi.org/10.1016/j.ecocom.2015.04.004.
- Xiao, X., Q. Zhang, B. Braswell, S. Urbanski, S. Boles, S. Wofsy, B. Moore III, and D. Ojima. 2004. “Modeling Gross Primary Production of Temperate Deciduous Broadleaf Forest Using Satellite Images and Climate Data.” Remote Sensing of Environment 91 (2): 256–270. https://doi.org/10.1016/j.rse.2004.03.010.
- Xie, X. Y., and A. N. Li. 2020. “An Adjusted Two-Leaf Light Use Efficiency Model for Improving GPP Simulations Over Mountainous Areas.” Journal of Geophysical Research 125 (13): Atmospheres 125. https://doi.org/10.1029/2019JD031702.
- Yuan, W., W. Cai, J. Xia, J. Chen, S. Liu, W. Dong, L. Merbold, et al. 2014. “Global Comparison of Light Use Efficiency Models for Simulating Terrestrial Vegetation Gross Primary Production Based on the LaThuile Database.” Agricultural and Forest Meteorology 192-193:108–120. https://doi.org/10.1016/j.agrformet.2014.03.007.
- Yuan, W., S. Liu, G. Zhou, G. Zhou, L. L. Tieszen, D. Baldocchi, C. Bernhofer, H. Gholz, A. H. Goldstein, and M. L. Goulden. 2007. “Deriving a Light Use Efficiency Model from Eddy Covariance Flux Data for Predicting Daily Gross Primary Production Across Biomes.” Agricultural and Forest Meteorology 143 (3–4): 189–207. https://doi.org/10.1016/j.agrformet.2006.12.001.
- Yu, G.-R., X.-F. Wen, X.-M. Sun, B. D. Tanner, X. Lee, and J.-Y. Chen. 2006. “Overview of ChinaFlux and Evaluation of Its Eddy Covariance Measurement.” Agricultural and Forest Meteorology 137 (3–4): 125–137. https://doi.org/10.1016/j.agrformet.2006.02.011.
- Zhang, F., J. M. Chen, J. Chen, C. M. Gough, T. A. Martin, and D. Dragoni. 2012. “Evaluating Spatial and Temporal Patterns of MODIS GPP Over the Conterminous U.S. Against Flux Measurements and a Process Model.” Remote Sensing of Environment 124:717–729. https://doi.org/10.1016/j.rse.2012.06.023.
- Zhou, J., L. Jia, and M. Menenti. 2015. “Reconstruction of Global MODIS NDVI Time Series: Performance of Harmonic Analysis of Time Series (HANTS).” Remote Sensing of Environment 163:217–228. https://doi.org/10.1016/j.rse.2015.03.018.
- Zhou, Y., X. Wu, W. Ju, J. M. Chen, S. Wang, H. Wang, W. Yuan, T. Andrew Black, R. Jassal, and A. Ibrom. 2016. “Global Parameterization and Validation of a Two‐Leaf Light Use Efficiency Model for Predicting Gross Primary Production Across FLUXNET Sites.” Journal of Geophysical Research: Biogeosciences 121 (4): 1045–1072. https://doi.org/10.1002/2014JG002876.