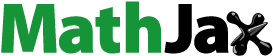
ABSTRACT
In this study, Eco-hydrometeorological Remote Sensing-based Penman-Monteith algorithm (Eh-RSPM) was developed by implementing the gross primary productivity into the revised Remote Sensing based Penman-Monteith algorithm (RS-PM). Evaluation of Eh-RSPM was conducted through comparison with in-situ measurements as well as model-based products (e.g. MODerate resolution Imaging Spectroradiometer (MODIS) 16 global ET products (MOD16 ET) and Surface Energy Balance System (SEBS)) during two years (2004 and 2012) in Northeast Asia. Comparison of ET from Eh-RSPM algorithm with five flux tower measurement agreed well with the flux tower datasets at the entire validation sites. Especially, Eh-RSPM showed advantages in improving the accuracy of ET at stations with relatively short canopy height (e.g. QHB and KBU site) as well as the forest site (e.g. SMK). Focusing on the forest site, Eh-RSPM exhibited slightly better statistical performance compared to MOD16. Specifically, the temporal mean bias and RMSD showed a slight improvement, decreasing from −15.40 W m−2 to −12.58 W m−2 and from 28.41 W m−2 to 25.26 W m−2, respectively. This is a key finding of this study, demonstrating the applicability of the improved ET algorithm to regions with significant forest cover. Similarly, spatial distribution of Eh-RSPM showed similar patterns with MOD16 and SEBS. Eh-RSPM strongly showed advantages over the land cover types with relatively shorter canopy height (e.g. grassland and alpine meadow) as well as the heterogeneous forest showed significant improvement in Eh-RSPM through considering the actual physiological behavior variation and influence of photosynthesis into ET calculation.
1. Introduction
The solar radiation that reaches the Earth’s surface contributes to evapotranspiration (ET), a process in which approximately 60 to 90% of the total precipitation is converted into water vapor and released into the atmosphere (Jung et al. Citation2010; McMahon et al. Citation2013). It is critical for monitoring and predicting regional and continental climate change as well as the crop yield and streamflow (Bastiaanssen et al. Citation1998; Masenyama et al. Citation2022). Traditionally, ground-based instruments (e.g. evaporation pan, lysimeter, and eddy covariance flux tower) have been used to measure ET while it has difficulty in capturing regional or continental ET due to the heterogeneous near-surface conditions (Li et al. Citation2009).
To resolve these limitations, numerous researchers have used remote sensing imageries in order to estimate spatio-temporal ET variation across the large spatial domain (Aksu and Arikan Citation2017; Cetin et al. Citation2023; Glenn et al. Citation2007; Hwang and Choi Citation2013; Jang et al. Citation2010, Citation2013; Kalma, McVicar, and McCabe Citation2008; Kim, Mu, and Choi Citation2012; Ryu et al. Citation2012; Tang et al. Citation2021; Wu et al. Citation2022). Remote sensing data is provided with various spatio-temporal resolutions which makes it possible to acquire various inland surface fluxes through a variety of methods. In terms of acquiring ET estimates with remote sensing imagery, surface energy balance algorithm (Allen, Tasumi, and Trezza Citation2007; Bastiaanssen et al. Citation1998; Kustas and Norman Citation1997; Masenyama et al. Citation2022; Su Citation2002) and the Penman-Monteith (P-M) based algorithm (Cleugh et al. Citation2007) are the two most applied algorithms. The surface-energy balance method simply calculates the net radiation, equivalent to the available energy (i.e. sum of latent (LE) and sensible heat flux (H)) and ground heat flux (G)). Bastiaanssen et al. (Citation1998) firstly suggested the estimation of H with the surface temperature (Ts), reflectance of land surface, and normalized difference vegetation index (NDVI). Moreover, Verstraeten et al. (Citation2005) used a ratio of LE to available energy (i.e. evaporative fraction) by using broadband surface albedo and Ts. A Surface Energy Balance System (SEBS; Su Citation2002) is one of the energy balance models designed for estimating surface evaporation as well as atmospheric turbulent fluxes in virtue of satellite observations and ancillary meteorological datasets over large scale (Su Citation2002; Su et al. Citation2005). The SEBS model has been widely utilized and evaluated in worldwide (Gokmen et al. Citation2012; Wang et al. Citation2012).
Comparing with the energy balance models, the Penman-Monteith (P-M) algorithm has been regarded as an efficient approach because it does not specifically require in-situ ancillary dataset such as specific humidity (Cleugh et al. Citation2007; Kim, Mu, and Choi Citation2012; Mu et al. Citation2007). Cleugh et al. (Citation2007) firstly calculated the regional ET with P-M algorithm using MODIS imageries and ground-based meteorological datasets and evaluated with the Fluxnet observations. However, they found that simple estimation of surface canopy conductance was a significant source of uncertainties. This motivates Mu et al. (Citation2007) to develop revised RS-PM, using enhanced vegetation index (EVI) for estimating vegetation cover fraction and employing the leaf area index (LAI), vapor pressure deficit, and minimum air temperature to improve the accuracy of canopy conductance. Furthermore, Mu et al. (Citation2011) further improved the Mu et al. (Citation2007)’s algorithm and it has been utilized in producing global ET estimates, which provide essential information on global energy and water balance. Previous researchers have evaluated the MOD16 ET products in worldwide (Jang et al. Citation2010; more citation) as well as improved the MOD16 algorithm through improving parameterizations in MOD16 algorithm.
The objectives of this study can be approached from two distinct viewpoints. The first objective pertains to the enhancement of ET estimation methods based on the physically-based P-M equation. As previously discussed, the RSPM model has undergone continuous refinements, especially concerning the estimation of vegetation cover fraction, over an extended period. It initially employed NDVI as input data and later shifted to EVI, as seen in the MOD16 ET algorithm. This study aims to augment the practicality of ET estimation by introducing Gross Primary Productivity (GPP) as input data, thereby accounting for vegetation activity instead of merely vegetation distribution represented by NDVI and EVI. We refer to this as the eco-hydrological remote sensing-based Penman-Monteith model (Eh-RSPM). The second objective involves validating the applicability of physically-based approaches through a comparison between evapotranspiration values derived from various estimation methods, including physically-based results and those obtained through the energy balance-based SEBS model. Through the validation process employing data collected from five eddy-covariance flux towers situated within the study area, we examined the regional suitability of Eh-RSPM.
2. Study area and datasets
2.1. Study area
This study selected five stations (approximately 15 × 15 km footprints) for evaluation of model-based ETs which were belonging to the Asia Flux network (), including two cropland sites: CFK (Cheongmicheon; South Korea) and MSE (Mass paddy; Japan), two grassland sites: KBU (Kherlenbayan; Mongolia) and QHB (Qinghai; China), and one forest site: SMK (Seolmacheon; South Korea). Five flux tower sites have distinctive geographical and climatic conditions, which helps to assess Eh-RSPM over various conditions. QHB site located in highest altitude (3,250 m) region, with an alpine meadow and clay loam soil covered at the northeastern part of the Qinghai-Tibet Plateau, regarded as major water resources for central China. Kato et al. (Citation2006) reported the mean annual precipitation and Ta during 1981–2000 as 561 mm and −1.7°C, respectively. QHB site has large seasonal variation in monthly mean temperature ranged from −15.0°C (January) and 10.0°C (July) (Gu et al. Citation2005). More than 80% precipitation occurs during monsoon period (i.e. May to September) with maximum LAI reached up to 3.8 during the growing season (Gu et al. Citation2006). KBU site is located in Mongolia at the elevation of 1,235 m and dominant vegetation of dry grass steppe. During 1993 to 2002, KBU showed a mean annual temperature and precipitation of 1.2°C and 181 mm, respectively (Li et al. Citation2014). Especially, approximately 88% of annual precipitation falls during June to September along with the maximum LAI as 1 (Li et al. Citation2014). Among the five stations, two stations (e.g. MSE and CFK) are classified as cropland sites covered with loam soil texture while SMK site is classified as mixed forest with dominant soil texture of loamy sand and loam. MSE and CFK sites show annual average of precipitation about 1,300 mm while SMK shows mean annual precipitation of 1,220 mm. These sites have similar characteristics in that most of precipitation falls during the monsoon period with relative higher annual mean Ta at summer, which significantly drops during winter season. summarizes the geographic and climate conditions of the selected flux tower sites.
Figure 1. Geographical locations of the study area encompassing five flux towers for the validation of ET estimation results.

Table 1. Summary of the geographical characteristics for five selected study sites.
2.1.1. Datasets
Four land products and one atmospheric product from Moderate Resolution Imaging Spectroradiometer (MODIS) are utilized to compute Eh-RSPM as well as SEBS ET: MODIS atmospheric profiles (MOD07), MODIS Land Surface Temperature from Terra (MOD11A1), MODIS Vegetation indices from Terra (MOD13A1), MODIS LAI product from Terra (MOD15A2H), and MODIS albedo product, which are combined measurement from Terra and Aqua (MCD43A3). MODIS land surface temperature product (MOD11A1).
MOD11A1 land surface temperature product is provided with spatial and temporal resolution of 1 km and daily, respectively. MOD13A1 contains the Normalized Difference Vegetation Index (NDVI) and Enhanced Vegetation Index (EVI) is provided with spatial and temporal resolution of 500 m and 16 days, respectively. MOD15A2H products include 8-day composite of Leaf Area Index (LAI) and Fraction of Photosynthetically Active Radiation (FPAR) with 500 m spatial resolution. In this study, we utilized the LAI for calculating the canopy conductance, which is described in Section 3.1. MCD43A3 albedo products contain the white- and black-sky albedo with the spatial and temporal resolution of 500 m and 16 days, respectively. Except the MOD07 product, all other products are re-projected onto an earth-reference grid in space and time (Masuoka et al. Citation1998). In addition to these products, Eh-RSPM utilized MOD17A2H Gross Primary Productivity (GPP) product for better representation of vegetation activities with consideration of photosynthesis and carbon cycle (Running et al. Citation2000). The GPP product has a spatial resolution of 500 m and represents cumulative results every 8 days. For the evaluation of Eh-RSPM ET, 8-day composite of MOD16 ET product (MOD16A2; Mu, Zhao, and Running Citation2011) having a spatial resolution of 500 m was used in study. In addition to MODIS datasets, Global Land Data Assimilation System (GLDAS) was also utilized in order to estimate the ET through SEBS model which will be described in section 3.2. GLDAS 2.0 provides optimal hydrometorological fluxes at various spatial and temporal resolutions through combining satellite imagery and in-situ measurements with an advanced land surface models (Noah 3.6) and data assimilation framework (Park and Choi Citation2015; Rodell et al. Citation2004). This study utilized the GLDAS version 2 dataset with 25-km spatial resolution and a 1-month temporal interval. Overall characteristics of datasets utilized in this study are described in .
Table 2. Description of the MODIS data used for Eh-RSPM and the GLDAS used for SEBS.
3. Methodology
3.1. Ecohydrological RSPM algorithm
As the baseline of Eh-RSPM algorithm is the revised remote sensing-based Penman-Monteith algorithm (revised RS-PM; Mu et al. Citation2007; Mu, Zhao, and Running Citation2011), brief formulations and new implementation in Eh-RSPM are presented here. The revised remote sensing-based ET algorithm proposed by Mu et al. (Citation2007) is an updated version of remote sensing-based P-M algorithm (RS-PM) (Cleugh et al. Citation2007), which is designed to apply remote sensing observations into the Penman-Monteith (P-M) equation. The major modification of the revised RS-PM has: 1) improved the parameterization of stomatal conductance using minimum air temperature and pressure deficit (VPD); 2) enhanced the accuracy of calculated vegetation cover fraction by replacing NDVI with EVI; and 3) introduced new terms, soil evaporation calculation (Mu et al. Citation2007).
The stomatal conductance (Cs) is converted to the canopy conductance (Cc) using LAI (Landsberg and Gower Citation1997):
The stomatal conductance (Cs) of plants is generally related to the variation of VPD as well as the minimum and maximum air temperature. However, Mu et al. (Citation2007) mentioned that maximum air temperature was ignored for the calculation of Cs as the influence of maximum air temperate is often inferred in the high VPD. Accordingly, Cs is computed as follows:
where CL is defined as an average of potential stomatal conductance per unit leaf area, and m(Tmin) and m(VPD) are the CL-related constraint multipliers regarding the minimum air temperature (Tmin) and VPD, respectively (Mu et al. Citation2007).
To calculate fraction of land surface reached at maximum vegetation canopy, Mu et al. (Citation2011) replaced the FPAR instead of NDVI. In this study, we considered new schemes to prescribe the vegetation cover fraction (hereafter Fcc) for considering photosynthesis (Sur et al. Citation2019). Calculation of Fcc was a used GPP value as follows:
where Fcc stands for ith day’s vegetation cover fraction; the subscript max and min symbolizes the maximum and minimum value of all GPP and EVI values attained for all observation periods. The Fcc calculated by average value (σ) between normalized EVI and GPP. Using the , incoming net radiation (A) can be partitioned into vegetation and soil linearly as follows:
where and
denote the partitioned net radiation to vegetation and soil, respectively. Using the estimated ASOIL, ET on the soil surface can be calculated. Eq. (6) below is the ET calculation process on the soil surface, and the total ET calculation process is same as Mu et al. (Citation2011).
where ETSOIL and ETwet_SOIL represent the ET estimated at the soil surface and wet soil surface, respectively. Fwet represents the fraction of wet surface over soil surface. ETSOILPOT denotes the potential ET over soil surface and s represents the slope of the temperature versus saturated water vapor pressure curve. β is a constant, set as 200 and γ is defined as psychrometric constant and RH symbolizes the relative humidity (%). Terms with r represent the resistance terms; aerodynamic resistance term (ras) and total aerodynamic resistance (rtot) calculated based on Mu et al., (Citation2007). Cp represents a specific heat capacity of air and ρ indicates the air density. The general procedure of calculating total ET is detailed described in Mu et al. (Citation2011).
3.2. MOD16 ET and SEBS algorithm
Both the MOD16 ET algorithm and the Eh-RSPM are grounded in the P-M equation. This study aims to substantiate the considerable influence of vegetation-related factors on ET by adjusting the constituents of Fcc within the P-M equation. In the conventional P-M equation, the MOD16 ET algorithm employed EVI in lieu of NDVI to delineate Fcc, while Eh-RSPM adopted GPP instead of EVI. These modifications enable the evaluation of vegetation’s impact when contrasting outcomes between the Eh-RSPM and MOD16 ET algorithm.
Within the realm of diverse techniques employed for evapotranspiration estimation, an energy balance-driven model of notable significance is the SEBS model (Su Citation2002). The SEBS model, which is regarded as extended version of the SEBI (Menenti and Choudhury Citation1993), estimates ET based on the surface energy balance with constraining dry and wet pixels separately to derive turbulent heat fluxes, evaporative fraction, and actual ET. This study delves into an investigation of the applicability of the P-M equation-based evapotranspiration estimation model, achieved through a comparative analysis between ET computed through energy balance and ET derived using the P-M equation.
4. Results and discussion
4.1. Comparisons of penman-monteith algorithms through sensitivity analysis
The purpose of the development of the Eh-RSPM algorithm is to improve the accuracy of estimated ET by improving the available net radiation on the soil surface (Asoil) of the existing RS-PM equation through implementing MODIS GPP product. Accordingly, prior to evaluate the Eh-RSPM ET, the sensitivities of NDVI, EVI and GPP, which were included for the calculation of Asoil of Eh-RSPM and MOD16, were explored by increasing and decreasing the uncertainty of each variable (−20%, −10%, 10%, and 20%). Then, computed LE for different percentage of uncertainty was compared with the reference (i.e. no uncertainty injection) to calculate mean of relative error and it was set as the sensitivity coefficient. When mean relative error of the factor has larger variation with respect to change of uncertainty, it indicates the greater sensitivity of the factor in terms of ET calculation.
represents the mean of relative errors of calculated ET with respect to the percentage change in GPP, NDVI, and EVI across the five different flux tower sites. Among three different variables, GPP showed the highest sensitivity throughout five different sites. Comparing the sensitivity of NDVI (used in Cleugh et al. Citation2007) and EVI (used in Mu, Zhao, and Running Citation2011), EVI showed relatively higher sensitivity than NDVI. This phenomenon can be described through differences in spectral resolution. NDVI uses only the red and near infrared regions while EVI additionally includes the blue band region, and in turn, resulted in reducing the background influence rather than vegetation (e.g. soil and atmospheric influence) (Jiang et al. Citation2008). Superior sensitivity of GPP over both NDVI and EVI is resulted from the fact that more physical characteristics contained in GPP. Both NDVI and EVI contain only spectral characteristics while GPP considers both spectral and physical characteristics of vegetation activity. Through sensitivity analysis, it was confirmed that the highest sensitivity coefficient appears when GPP is used.
Figure 2. Sensitivity analysis results of the three input data (NDVI, EVI, and GPP) for vegetation cover fraction to identify the most impacted factor in ET estimation.

In the matter of various land cover types, cropland and forest areas showed relatively higher variation of mean relative error than alpine meadow and grassland sites. Especially, SMK site, a forest area, showed the largest deviation of the sensitivity coefficient for GPP. In general, forest areas have relatively larger leaf area than other land cover types. Accordingly, accurate quantification of seasonal variation in photosynthesis capacity is important to acquire accurate ET estimation over forest region (Asner, Scurlock, and Hicke Citation2003; Medvigy et al. Citation2013). As GPP reflects the phenology, it showed highest sensitivity with GPP over forest region. On the other hand, in the case of alpine meadow or grassland, it was confirmed that all three variables did not change significantly, and this was due to the influence of the surface resistance value with the land cover. That is, Li et al. (Citation2013) and Bie et al. (Citation2014) addressed that the change of surface resistance significantly influenced the estimated ET over forest, crop, and rice paddy region while the other land cover types did not show variation in accordance with the change of surface resistance term.
4.2. Temporal variations of ET and evaluation against ground observations
illustrates the temporal variation of monthly-averaged LE from three different approaches (i.e. Eh-RSPM, SEBS, and MOD16) as well as the in-situ measurements at five flux tower sites during 2004 for KBU, QHB, and MSE sites and 2012 for SMK and CFK. The overall result confirmed that LE from three different models showed analogous seasonal pattern with the in-situ measurement throughout five different sites. Both modeled and observed LE generally showed increasing trend until august and changes to decreasing trend in general. However, LE temporally showed a slight decreasing trend from July to August. The main reason for this phenomenon is that all the validation sites are located under the influence of monsoon climate. Sur et al. (Citation2015) mentioned that influence of continental wind during the summer season over monsoon climate zone triggers heavy rainfall. During this period, since the amount of cloud increases, the amount of surface radiation decreases and the LE value temporally decreases.
(Legend of the solid line and symbol pairs represented at the bottom of the plots.)
summarizes the statistical behavior of estimated ET from three different models with the flux tower measurements. The result showed that relatively high correlations (r > 0.61) were observed for all three algorithms with ground-based ET at all measurement sites except KBU. Comparing the statistical performance of MOD16 versus Eh-RSPM, all five stations showed improvement in r, indicating that Eh-RSPM showed more similar seasonal variation with in-situ observations than MOD16. Furthermore, Eh-RSPM yielded slightly better bias and root mean square differences (RMSD) compared to MOD16 across the different flux tower sites except two cropland sites. Especially, bias and RMSD of KBU and QHB sites with Eh-RSPM showed significant improvement comparing with both SEBS and MOD16 (). This phenomenon can be explained through the difference of vegetation indices (e.g. NDVI and EVI) versus GPP. MODIS 16 and SEBS ET determine vegetation-related components such as canopy height and vegetation cover based on the spectral reflectance while it lacks to reflect the actual physiological activities. For example, KBU site is classified as grassland while actual condition is much closer to bare soil with average canopy height of 2 cm, inferring the less physiological activities than usual grassland locations. Accordingly, implementation of GPP allows to consider physical characteristics of photosynthesis in Eh-RSPM, and hence, attributed to increase the accuracy of ET.
Table 3. Summary of the statistical metrics between estimated and measurements of ET at five flux tower locations.
Focusing on the forest land cover type (SMK site), Eh-RSPM showed slightly better statistical performance in comparison with the MOD16 (). More specifically, temporal mean of bias and RMSD showed slight improvement from −15.40 W m−2 to −12.58 W m−2 and 28.41 W m−2 to 25.26 W m−2, respectively. In terms of capturing the seasonal variation of ET, Eh-RSPM showed more analogous trend with in-situ observation than MOD16. The main difference of Eh-RSPM versus MOD16 ET over the forest was the different parameterization of surface resistance, GPP versus vegetation indices (e.g. NDVI and EVI). indicated that GPP showed the highest sensitivity toward ET over the station located at forest cover (e.g. SMK). In general, stations located at forest cover have steep slope along covered with dense forest with large heterogeneity. Thus, accurate parameterization of resistance term in RS-PM equation is important to obtain high-quality ET estimates.
4.3. Spatial variations of ET using various models
depict the spatial distributions of the monthly-averaged ET estimated from Eh-RSPM, SEBS, and MOD16 ET across the study area from May to September for 2004 and 2012, which are corresponding to the available time span for flux tower datasets. The dark blue and red colors in the color ramp denote the maximum (500 W m−2 during May to August and the others 300 W m−2) and minimum (approximately 0 W m−2) values, respectively, of all results on the spatial mapping.
Overall spatial distribution of ET from three different methods showed analogous patterns in that north-western part of study domain yielded relative lower monthly-averaged ET while Eastern side of the study area showed relatively higher monthly-averaged ET. Among three different ET estimates, SEBS-based ET represented a relatively higher range of ET than MODIS-based ET results (i.e. Eh-RSPM and MOD16) across the study domain. However, focusing on the Korean peninsula, SEBS showed relatively lower magnitude of ET while MOD16 revealed relatively high magnitude of ET (). This behavior was also revealed in previous studies. Jang et al. (Citation2013) calculated MODIS-based ET over Northeast Asia and found that MOD16 ET showed overestimated patterns at forest area. Kim et al. (Citation2012) also mentioned that MOD16 ET showed overestimation at 8 out of 13 forest sites across the East Asia. Lee et al. (Citation2017) mentioned that more than 70% of the Korean peninsula is classified as mountainous terrain with complex terrain characteristics (e.g. high elevations with steep slope on northeastern part) and heterogeneous forest type. Thus, accurate parameterization of vegetation-related information is one of the key factors to obtain reasonable ET estimates. This phenomenon leads to the conclusion that Eh-RSPM showed capability of improving the accuracy of ET over forested area through considering the physiological behavior.
5. Conclusion
This study introduced the Eh-RSPM, which is designed to implement the GPP into RS-PM to improve the representation of the physiological behavior in the estimation of ET. Eh-RSPM was evaluated through the inter-comparison with SEBS and MOD16 ET as well as ground-based ET measurements across the East Asia continent. Especially, we selected five flux tower sites which has different land cover type (e.g. mixed forest, grassland, and cropland regions) to examine the performance of Eh-RSPM over different land cover types.
The sensitivity analysis for quantifying the influence of different vegetation indices (e.g. NDVI, EVI, and GPP) toward the accuracy of ET showed that GPP showed the largest sensitivity across the five sites, followed by EVI and NDVI. In terms of different land cover types, forest region (e.g. SMK) represented the largest sensitivity of vegetation indices while grassland and alpine meadow sites showed relatively small sensitivity due to the relatively small vegetation mass along with little sensitivity of resistance term. Forest covered regions have steep slope along covered with dense forest with large heterogeneity. Due to these topographical characteristics, accurate parameterization of resistance term in RS-PM equation is important to obtain high-quality ET estimates.
Overall comparison of ET from three different models revealed that Eh-RSPM showed significant improvement in comparison with the MOD16. Especially, Eh-RSPM showed advantages in improving the accuracy of ET at stations with relatively short canopy height (e.g. QHB and KBU) as well as the forest site (e.g. SMK). The primary reason for the improvement is the implementation of GPP into ET calculation so that accurate seasonal variation of phenology as well as the influence of actual photosynthesis can be explicitly considered. Combining photosynthetic factors has contributed to improving the accuracy of ET, but it still has limitations. Specifically, further research is needed on the calculation algorithm of ET that occurs during the dormant periods from November to March. In addition, this study area, which belongs to the monsoon climate zone, has heavy rainfall between June and July, and additional research is needed on the ET fluctuations that occur during this period.
Although several studies have assessed the applicability of various ET algorithms in virtue of remote sensing imagery, no unanimity has been made regarding which model is the most feasible option. Such studies are important because they evaluate the applicability of different algorithms implemented with the inherent physical parameterization in each algorithm and distinctive forcings. This, therefore, provides impetus for future development about consideration of utilizing other physically-meaningful variables to overcome the inherent uncertainties across the heterogeneous regions.
Acknowledgments
This work was supported by the National Research Foundation of Korea(NRF) grant funded by the Korea government(MSIT). (No. NRF-2021R1A2C1093245)
Disclosure statement
No potential conflict of interest was reported by the author(s).
Data availability statement
The MODIS data that support the findings of this study are available in “Earthdata” athttps://search.earthdata.nasa.gov/search?fpc0=Earth+Observation+Satellites&fps0=Terra&fpb0=Space-based+Platforms. The GLDAS data are obtained through the following link from Earthdata (https://search.earthdata.nasa.gov/search?q=GLDAS_NOAH025_M_2.0).
Additional information
Funding
References
- Aksu, H., and A. Arikan. 2017. “Satellite-Based Estimation of Actual Evapotranspiration in the Buyuk Menders Bain Turkey.” Hydrology Research 48 (2): 559–13. https://doi.org/10.2166/nh.2016.226.
- Allen, R. G., M. Tasumi, and R. Trezza. 2007. “Satellite-Based Energy Balance for Mapping Evapotranspiration with Internalized Calibration (METRIC)—Model.” Journal of Irrigation & Drainage Engineering 133 (4): 380–394. https://doi.org/10.1061/(ASCE)0733-9437(2007)133:4(380).
- Asner, G. P., J. M. O. Scurlock, and J. A. Hicke. 2003. “Global Synthesis of Leaf Area Index Observations: Implications for Ecological and Remote Sensing Studies.” Global Ecology and Biogeography 12 (3): 191–205. https://doi.org/10.1046/j.1466-822X.2003.00026.x.
- Bastiaanssen, W. G. M., M. Menenti, R. A. Feddes, and A. A. M. Holtslag. 1998. “A Remote Sensing Surface Energy Balance Algorithm for Land (SEBAL): 1. Formulation.” Canadian Journal of Fisheries and Aquatic Sciences 212-213:198–212. https://doi.org/10.1016/S0022-1694(98)00253-4.
- Bie, W., M. C. Casper, P. Reiter, and M. Vohland. 2014. “Surface Resistance Calibration for a Hydrological Model Using Evapotranspiration Retrieved from Remote Sensing Data in Nahe Catchment Forest Area.” Proceedings of the International Association of Hydrological Sciences 368:81–86. https://doi.org/10.5194/piahs-368-81-2015.
- Cetin, M., O. Alsenjar, H. Aksu, M. S. Golpinar, and M. A. Akgul. 2023. “Estimation of Crop Water Stress Index and Leaf Area Index Based on Remote Sensing Data 2023.” Water Supply 23 (3): 1390–1404. https://doi.org/10.2166/ws.2023.051.
- Cleugh, H. A., R. Leuning, Q. Mu, and S. W. Running. 2007. “Regional Evaporation Estimates from Flux Tower and MODIS Satellite Data.” Remote Sensing of Environment 106 (3): 285–304. https://doi.org/10.1016/j.rse.2006.07.007.
- Glenn, E. P., A. R. Huete, P. L. Nagler, K. K. Hirschboeck, and P. Brown. 2007. “Integrating Remote Sensing and Ground Methods to Estimate Evapotranspiration.” Critical Reviews in Plant Sciences 26 (3): 139–168. https://doi.org/10.1080/07352680701402503.
- Gokmen, M., Z. Vekerdy, A. Verhoef, W. Verhoef, O. Batelaan, and C. van der Tol. 2012. “Integration of Soil Moisture in SEBS for Improving Evapotranspiration Estimation Under Water Stress Conditions.” Remote Sensing of Environment 121:261–274. https://doi.org/10.1016/j.rse.2012.02.003.
- Gu, Y. X., S. Bélair, J. F. Mahfouf, and G. Deblonde. 2006. “Optimal Interpolation Analysis of Leaf Area Index Using MODIS Data.” Remote Sensing of Environment 104 (3): 283–296. https://doi.org/10.1016/j.rse.2006.04.021.
- Gu, S., Y. Tang, X. Cui, T. Kato, M. Du, Y. Li, and X. Zhao. 2005. “Energy Exchange Between the Atmosphere and a Meadow Ecosystem on the Qinghai–Tibetan Plateau.” Agricultural and Forest Meteorology 129 (3–4): 175–185. https://doi.org/10.1016/j.agrformet.2004.12.002.
- Hwang, K., and M. Choi. 2013. “Seasonal Trends of Satellite-Based Evapotranspiration Algorithms Over a Complex Ecosystem in East Asia.” Remote Sensing of Environment 137:244–263. https://doi.org/10.1016/j.rse.2013.06.006.
- Jang, K., S. Kang, J. Kim, C. B. Lee, T. Kim, J. Kim, R. Hirata, and N. Saigusa. 2010. “Mapping evapotranspiration using MODIS and MM5 Four-Dimensional Data Assimilation.” Remote Sensing of Environment 114:657–673. https://doi.org/10.1016/j.rse.2009.11.010.
- Jang, K., S. Kang, Y. J. Lim, S. Jeong, J. Kim, J. S. Kimball, and S. Y. Hong. 2013. “Monitoring daily evapotranspiration in Northeast Asia using MODIS and a regional Land Data Assimilation System.” Journal of Geophysical Research Atmospheres 118:12927–12940. https://doi.org/10.1002/2013JD020639.
- Jiang, Z., A. R. Huete, K. Didan, and T. Miura. 2008. “Development of a Two-Band Enhanced Vegetation Index without a Blue Band.” Remote Sensing of Environment 112:3833–3845. https://doi.org/10.1016/j.rse.2008.06.006.
- Jung, M., M. Reichstein, P. Ciais, S. I. Seneviratne, J. Sheffield, M. L. Goulden, G. Bonan, et al. 2010. “Recent decline in the global land evapotranspiration trend due to limited moisture supply.” Nature 467 (7318): 951–954. https://doi.org/10.1038/nature09396.
- Kalma, J., T. McVicar, and M. McCabe. 2008. “Estimating Land Surface Evaporation: A Review of Methods Using Remotely Sensed Surface Temperature Data.” Surveys in Geophysics 29 (4–5): 421–469. https://doi.org/10.1007/s10712-008-9037-z.
- Kato, T., Y. Tang, S. Gu, Hirota, M., Du, M., Y. Li, and X. Zhao. 2006. “Temperature and biomass influences on interannual changes in CO2 exchange in an alpine meadow on the Qinghai‐Tibetan Plateau.” Global Change Biology 12 (7): 1285–1298. https://doi.org/10.1111/j.1365-2486.2006.01153.x.
- Kim, H., M. Mu, and Q. Choi. 2012. “Validation of MODIS16 Global Terrestrial ET Product in Various Climate and Land Cover Types in Asia.” KSCE Journal of Civil Engineering 16 (2): 229–238. https://doi.org/10.1007/s12205-012-0006-1.
- Kustas, W. P., and J. M. Norman. 1997. “A Two-Source Approach for Estimating Turbulent Fluxes Using Multiple Angle Thermal Infrared Observations.” Water Resources Research 33 (6): 1495–1508. https://doi.org/10.1029/97WR00704.
- Landsberg, J. J., and S. T. Gower. 1997. Applications of Physiological Ecology to Forest Management. San Diego, California, U.S: Academic.
- Lee, C., J. Park, J. Shin, and J. Jang. 2017. “Improvement of AMSR2 Soil Moisture Products Over South Korea.” IEEE Journal of Selected Topics in Applied Earth Observations and Remote Sensing 10 (9): 3839–3849. https://doi.org/10.1109/JSTARS.2017.2723923.
- Li, S., S. Kang, L. Zhang, F. Li, X. Hao, S. Ortega-Farias, W. Guo, S. Ji, J. Wang, and X. Jiang. 2013. “Quantifying the Combined Effects of Climatic, Crop and Soil Factors on Surface Resistance in a Maize Field.” Canadian Journal of Fisheries and Aquatic Sciences 489 (10): 124–134. https://doi.org/10.1016/j.jhydrol.2013.03.002.
- Li, Z. L. R. Tang, Z. Wan, Y. Bi, C. Zhou, B. Tang, G. Yan, and X. Zhang. 2009. “A Review of Current Methodologies for Regional Evapotranspiration Estimation from Remotely Sensed Data.” Sensors 9:3801–3853. https://doi.org/10.3390/s90503801.
- Li, X. J., Z. Q. Xiao, J. D. Wang, Y. Qu, and H. A. Jin. 2014. “Dual Ensemble Kalman Filter Assimilation Method for Estimating Time Series LAI.” Journal of Remote Sensing 18 (1): 27–44.
- Masenyama, A., O. Mutanga, T. Dube, T. Bangira, M. Sibanda, and T. Mabhaudhi. 2022. “A Systematic Review on the Use of Remote Sensing Technologies in Quantifying Grasslands Ecosystem Services.” GIScience & Remote Sensing 59 (1): 1000–1025. https://doi.org/10.1080/15481603.2022.2088652.
- Masuoka, E., A. Fleig, R. E. Wolfe, and F. Patt. 1998. “Key Characteristics of MODIS Data Products.” IEEE Transactions on Geoscience and Remote Sensing 36 (4): 1313–1323. https://doi.org/10.1109/36.701081.
- McMahon, T. A., M. C. Peel, L. Lowe, R. Srikanthan, and T. R. McVicar. 2013. “Estimating Actual, Potential, Reference Crop and Pan Evaporation Using Standard Meteorological Data: A Pragmatic Synthesis.” Hydrology and Earth System Sciences 17:1331–1363. https://doi.org/10.5194/hess-17-1331-2013.
- Medvigy, D., S. J. Jeong, K. L. Clark, N. S. Skowronski, and K. V. R. Schafer. 2013. “Effects of Seasonal Variation of Photosynthetic Capacity on the Carbon Fluxes of a Temperature Deciduous Forest.” Journal of Geophysical Research: Biogeosciences 118 (4): 1703–1714. https://doi.org/10.1002/2013JG002421.
- Menenti, M., and B. J. Choudhury. 1993. “Parametrization of Land Surface Evapotranspiration Using a Location-Dependent Potential Evapotranspiration and Surface Temperature Range. In: Exchange Processes at the Land Surface for a Range of Space and Time Scales”. Bolle H.J. et al. IAHS Publ. (212):561–568. http://iahs.info/redbooks/212.htm.
- Mu, Q., F. A. Heinsch, M. Zhao, and S. W. Running. 2007. “Development of a Global Evapotranspiration Algorithm Based on MODIS and Global Meteorology Data.” Remote Sensing of Environment 111 (4): 519–536. https://doi.org/10.1016/j.rse.2007.04.015.
- Mu, Q., M. Zhao, and S. W. Running. 2011. “Improvements to a MODIS Global Terrestrial Evapotranspiration Algorithm.” Remote Sensing of Environment 115 (8): 1781–1800. https://doi.org/10.1016/j.rse.2011.02.019.
- Park, J., and M. Choi. 2015. “Estimation of evapotranspiration from ground-based meteorological data and global land data assimilation system (GLDAS).” Stochastic Environmental Research and Risk Assessment 29 (8): 1963–1992. https://doi.org/10.1007/s00477-014-1004-2.
- Rodell, M., P. R. Houser, U. Jambor, J. Gottschalck, K. Mitchell, C. J. Meng, K. Arsenault, et al. 2004. “The Global Land Data Assimilation System.” Bulletin of the American Meteorological Society 85:381–394. https://doi.org/10.1175/BAMS-85-3-381.
- Running, S. W., P. E. Thornton, R. Nemani, and J. Glassy. 2000. “Global Terrestrial Gross and Net Primary Productivity from the Earth Observing System.” In Methods in Ecosystem Science, edited by O. E. Sala and R. B. Jackson, 44–57. New York, NY, USA: Springer-Verlag.
- Ryu, Y., D. D. Baldocchi, T. A. Black, M. Detto, B. E. Law, R. Leuning, A. Miyata, et al. 2012. “On the Temporal Upscaling of Evapotranspiration from Instantaneous Remote Sensing Measurements to 8-Day Mean Daily-Sums.” Agricultural and Forest Meteorology 152:212–222. https://doi.org/10.1016/j.agrformet.2011.09.010.
- Su, Z. 2002. “The Surface Energy Balance System (SEBS) for Estimation of Turbulent Heat Fluxes.” Hydrology and Earth System Sciences 6 (1): 85–99. https://doi.org/10.5194/hess-6-85-2002.
- Su, H., M. F. McCabe, E. F. Wood, Z. Su, and J. H. Prueger. 2005. “Modeling Evapotranspiration During SMACEX: Comparing Two Approaches for Local- and Regional-Scale Prediction.” Journal of Hydrometeorology 6 (6): 910–922. https://doi.org/10.1175/JHM466.1.
- Sur, C., S. Kang, J. Kim, and M. Choi. 2015. “Remote Sensing-Based Evapotranspiration Algorithm: A Case Study of All Sky Conditions on a Regional Scale.” GIScience & Remote Sensing 52 (5): 627–642. https://doi.org/10.1080/15481603.2015.1056288.
- Sur, C., D. Kim, J. Lee, M. M. Iqbal, and M. Choi. 2019. “Hydrological Drought Assessment of Energy-Based Water Deficit Index (EWDI) at Different Geographical Regions.” Advances in Meteorology 8512727:1–11. https://doi.org/10.1155/2019/8512727.
- Tang, L., S. Zhang, J. Zhang, Y. Liu, and Y. Bai. 2021. “Estimating Evapotranspiration Based on the Satellite-Retrieved Near-Infrared Reflectance of Vegetation (NIRv) Over Croplands.” GIScience & Remote Sensing 58 (6): 889–913. https://doi.org/10.1080/15481603.2021.1947622.
- Verstraeten, W. W., F. Veroustraete, and J. Feyen. 2005. “Estimating Evapotranspiration of European Forests from NOAA-Imagery at Satellite Overpass Time: Towards an Operational Processing Chain for Integrated Optical and Thermal Sensor Data Products.” Remote Sensing of Environment 96 (2): 256–276. https://doi.org/10.1016/j.rse.2005.03.004.
- Wang, K. C., R. E. Dickinson, L. Su, and K. E. Trenberth. 2012. “Contrasting Trends of Mass and Optical Properties of Aerosols Over the Northern Hemisphere from 1992 to 2011.” Atmospheric Chemistry and Physics 12 (19): 9387–9398. https://doi.org/10.5194/acp-12-9387-2012.
- Wu, T., X. Zhao, S. Wang, X. Zhang, K. Liu, and J. Yang. 2022. “Phenology-Based Cropland Retirement Remote Sensing Model: A Case Study in Yan’an.Loess Plateau, China.” GIScience & Remote Sensing 59 (1): 1103–1120. https://doi.org/10.1080/15481603.2022.2100043.