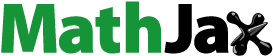
Abstract
To accurately calculate the impact of renewables on power production in complex electric power grids, high-resolution and ideally seamless data within the planetary boundary layer are required. Therefore, the quality of different regional reanalyses and hindcasts is evaluated with respect to the representation of the planetery boundary layer and related sub-daily processes. On the one hand, high resolution regional reanalysis from the UERRA (UE-SMHI, UE-UKMO) and a similar project (COSMO-REA6) are considered. On the other hand, two hindcasts based on the COSMO-REA6 configuration are included in this study, i.e. a simulation with perfect boundaries and a simulation additionally utilizing spectral nudging. The focus of the evaluation is on measurements at four flux towers that are not part of any assimilation procedure. In this paper, we will show that the model’s quality depends on both the complete model system – assimilation method, resolution and physical parameterization – as well as on the performance measure. The daily cycle is best depicted by the hindcasts and even COSMO-REA6 hardly introduces spurious variability. UE-SMHI (3D-Var) suffers from spin-up in particular visible at the elevated levels, whereas the spin-up is damped in UE-UKMO (4D-Var). Investigation of atmospheric stability reveals that diurnal variation of stratification is for the most part well reproduced, but strong deficits were found for all COSMO simulations in reproducing strong stratification and corresponding wind speed gradients. Moreover, an overestimation of superadiabatic lapse rates and corresponding overly weak turbulent mixing is found for UE-UKMO. Furthermore, a combination of ramp statistics and contingency tables is utilized to detect a clear advantage of sophisticated assimilation systems over hindcasts. The evaluation framework presented underpins the importance of ramp statistics and vertical measurement profiles, especially with respect to assessing long-term simulations.
1. Introduction
In recent years, the energy sector has become one of the most exciting and challenging applications for numerical weather predictions or – with respect to historical periods – reanalyses and hindcasts (integration where no observations have been assimilated). So-called energy system models aim to simulate the flow in complex power grids (Kaldemeyer et al., Citation2016). They take into account various system parameters (e.g. transformation in substations) and the simulated feed-in of different power plants, which is the electric energy generated by, for instance, a wind turbine or a heating power station. A multiyear simulation of the power grid allows establishing strategies for energy storage and grid expansion (Heide et al., Citation2011; Zerrahn and Schill, Citation2017).
To determine the feed-in of renewable power plants, having high-quality and highly resolved data about the atmospheric variability in three-dimensional space and time is essential. Measurement data at specific locations are very accurate but offer small spatial representativeness and are inappropriate for modeling in widespread power grids. Wind atlases rely on highly robust measurement data and very complex and highly resolved (large-eddy) simulations (Tammelin et al., Citation2011), but only statistics on climatological scales and a limited number of parameters are provided. In contrast, global reanalyses are known to provide reliable 4D data, such as the ERA-Interim (Dee et al., Citation2011), the MERRA and its successor MERRA-2 (Randles et al., Citation2017). Many in situ observations and remote-sensing products are input into the global reanalyses, so that the atmospheric data offer a rather good evaluation performance (Sharp et al., Citation2015). That is why these reanalyses are widely used for renewable energy applications (Cannon et al., Citation2015; Pfenninger and Staffell, Citation2016; Wohland et al., Citation2018).
However, global reanalyses exhibit a few fundamental weaknesses. Their temporal resolution is often limited to three/six hours, and moreover, the spatial resolution is comparably coarse ( km). Even more crucial, not all data that are relevant for energy system models are provided. Calculations on photovoltaic systems require data of incoming solar diffusive irradiation fluxes that are not delivered with the original reanalysis products. Hence, workarounds are applied for separating the global irradiation flux (Dürr, Citation2004; Helbig et al., Citation2009).
Having in mind the limitations of global reanalysis, we intend in our contribution to analyze and evaluate in detail the quality of regional reanalysis and hindcasts with respect to the lower planetary boundary layer (PBL, up to 300 m above ground) and related sub-daily variability. The focus is on measurements at four different flux towers. Our study considers the high-resolution reanalysis datasets of the Uncertainties in Ensembles of Regional ReAnalyses (UERRA, Unden, Citation2018) and a similar project (COSMO-REA6, Bollmeyer et al., Citation2015), because they comprise extensive data assimilation with the most recent observational database. Although the resolutions of the regional reanalyses are much higher than those of the global ones, to our knowledge, no extensive usage of these products for energy system modeling has occurred to date. However, the potential for solar energy applications has been analyzed (Frank et al., Citation2018; Urraca et al., Citation2018).
Our evaluation framework is extended to hindcast data because they are expected to provide seamless integrations on a sub-daily scale and deliver user-specified data with a frequency of e.g. 20 min. For the production of the hindcast data, we intend to use the same model system as used for one of the regional reanalysis. Thus, a lot of the factors influencing the model results can be minimized: resolution, physical parameterizations, numerical schemes. This allows to study more systematically the impact of assimilation on the model’s quality. This discussion is also valuable because the atmospheric quantities and the measurement locations discussed in the paper are typically not part of an assimilation.
Regarding the analysis of UERRA and COSMO-REA6 data sets, a limited number of studies exist about the evaluation of precipitation, 2 m temperature or cloud characteristics (Bollmeyer et al., Citation2015; Dahlgren et al., Citation2016). Moreover, there have been a few investigations about the representation of near-surface winds (Kaiser-Weiss et al., Citation2015; Dahlgren et al., Citation2016). Kaiser-Weiss et al. (Citation2015) reported that the COSMO-REA6 reanalysis offers a very high-level of correlation for most German weather stations (often more than 0.9), which is slightly better than global reanalysis ERA-Interim. It is indicated that complex orography and a low degree of spatial representativeness degrade the performance. However, investigations focusing on the development of the PBL and related sub-daily processes are quite rare. Borsche et al. (Citation2016) proposed first evaluating regional reanalyses on flux tower measurements. He analyzed the wind at flux towers and concluded that a) the mean diurnal cycle and annual cycle are well represented by the regional reanalyses COSMO-REA6 and ERA-Interim, and b) the temporal correlations tend to be very similar.
With our work presented here, we extend and push further the evaluation of regional reanalyses and hindcasts on flux tower measurements in different aspects. First, various regional reanalysis and hindcasts are considered for a systematic intercomparison. Second, the vertical temperature gradient and the related stratification are analyszed because they determine the wind profiles to a large extent. Third, we aim to pave the way for focusing also on small-scale variability using ramp statistics. Ramps are sudden jumps in a time series, and ramps in wind speed are of particular interest for the wind energy sector. Bianco et al. (Citation2016) previously showed how to analyze ramps and how to assess the performance of numerical weather predictions depending on different lead times (read further in Section 4.5). Here, we take up the idea of ramp statistics and apply it to the reanalyses and hindcasts. Finally, we hope to contribute to a better understanding of how well PBL processes are resolved in the reanalysis and hindcasts. In particular, we aim to assess the performance and limitations of the simulations regarding applications such as feed-in calculations in energy system models.
This paper is structured as follows. First, the observational and model datasets used for the investigation are introduced. Second, the methodology for measuring the model performance is discussed. Then, an extensive analysis of model quality is done. Finally, conclusions are drawn based on a summary of the results.
2. Reference datasets
Flux tower measurements at four different locations are used to analyze the performances of reanalysis and hindcasts: Hamburg, Falkenberg, Cabauw and Karlsruhe. The locations of the flux towers are shown in , and a short summary of the main characteristics is listed in . Further explanations and details about the towers can be found in Appendix A
Fig. 1. Model domains related to the different reanalyses and hindcasts (see ). CCLM-oF is integrated within the red polygon, COSMO-REA6 within the blue polygon and UE-UKMO within the green polygon. The UE-SMHI domain is denoted by the orography map [m].
![Fig. 1. Model domains related to the different reanalyses and hindcasts (see Table 2). CCLM-oF is integrated within the red polygon, COSMO-REA6 within the blue polygon and UE-UKMO within the green polygon. The UE-SMHI domain is denoted by the orography map [m].](/cms/asset/40f516b8-3e04-48a4-a1f1-fae3352d92e1/zela_a_1804294_f0001_c.jpg)
Table 1. Location, boom height above ground [m], estimation of roughness [m] and data availability for 2006–2007 [%] at the sites considered for evaluation. The roughnesses for the observational sites are not extracted in a consistent way (turbulent flux measurements at different heights vs. Richardson methods). The values of the hindcasts are extracted from the grid morphology near the site location. The values from UE-UKMO are not part of the standard output due to tile-based calculation within land-surface model JULES (Best et al., Citation2011; Clark et al., Citation2011).
3. Hindcast and reanalysis datasets
The representation of planetary boundary layer processes and short-term variability is analyzed for two types of model data. First, we consider the regional reanalysis products available from the UERRA project, i.e. UERRA-UKMO and UERRA-SMHI, as well as a product from a similiar project (COSMO-REA6). All of them comprise sophisticated data assimilation with the most recent observational database. Second, regional hindcasts are considered because they are expected to provide seamless simulations within the planetary boundary layer throughout the day and high-frequency data. Our strategy is to establish the hindcast based on the COSMO-REA6, which allows us to better distinguish in our analysis different factors influencing the model performance. In this way, for example, the physical parameterizations and numerical schemes remain unchanged, and the influence of the assimilation method can be better examined.
The main characteristics of all simulations are summarized in , and their domains are shown in . First of all an introduction to the UERRA reanalysis products is given, followed by the COSMO-REA6 product and the hindcasts based on COSMO-REA6.
Table 2. The models evaluated in this study. The PBL model levels are with respect to the lowest 1500 m. The positions of the model levels are related to flat terrain and to a surface pressure of 1013.26 hPa. For interpretation of the results, the datasets are indicated with the following abbreviations: CCLM-oF-SN, CCLM-oF, COSMO-REA6, UE-SMHI and UE-UKMO.
3.1. UERRA-SMHI
Within the UERRA project, different weather services have paved the way for producing regional reanalysis products with comparable model domains, unique forcing (ERA-Interim), porting to the same computer system and unification of output streams. The UERRA-SMHI reanalysis is produced using the data assimilation system HARMONIE. HARMONIE is jointly developed and used within the High Resolution Limited Area Model (HIRLAM) and Aire Limitée Adaptation dynamique Développement InterNational (ALADIN) consortia. The term HARMONIE (HIRLAM/ALADIN Research on Mesoscale Operational NWP in Euromed) refers to the scripting system, which facilitates data assimilation and observation handling, climate generation, lateral boundary coupling, and postprocessing required to run the forecast model operationally within the HIRLAM countries (Bengtsson et al., Citation2017). The forecast model used for the UERRA reanalysis is based on a hydrostatic version of HARMONIE (limited area model version of ARPEGE-IFS using a hydrostatic approximation Bubnov et al., Citation1995) and utilizes the ALADIN physics setup.
The UERRA-HARMONIE system has run for the entire reanalysis period from 1961 onward with four cycles per day performing analyses at 00, 06, 12 and 18 UTC, where the first guess of the atmospheric state produced by the forecast model is corrected on the basis of observations. The adjustment takes into account the statistics of the model and observational errors. The forecast lengths vary between 6 and 30 hours. The UERRA-HARMONIE system uses 65 vertical sigma-pressure hybrid levels and a grid mesh with approximately 11 km horizontal resolution. The data assimilation process consists of a 2D surface analysis (OI, 2 m temperature and humidity, snow depth) and 3D variational analyses (3D-Var) for the upper air variables (wind, temperature, specific humidity) and surface pressure. Further details about the assimilation system of the HIRLAM consortium, which was used for the predecessor product of UERRA EURO4M, are presented in Dahlgren et al. (Citation2016) and Landelius et al. (Citation2016).
The output data are accessible using the ECMWF MARS archive. We rely on the deterministic assimilation experiments – rather than the ensemble-based assimilation – for reasons of data availability, horizontal resolution and storage issues with 4D data. At the time of data retrieval at the end of 2017, the period from 2006 to 2010 was available for download by all members of UERRA. As of the beginning of 2019, data are available from 1961 onward, and UERRA-HARMONIE has been extended operationally as part of Copernicus Climate Change Service (C3S, https://climate.copernicus.eu/regional-reanalysis-europe).
The UERRA-SMHI model simulations are performed on a Lambert Conic Conformal grid. The output of interest is provided on height levels (15, 30, 50, 75, 100, 150, 200, 250 and 300 m above the Earth’s surface). To compare with flux tower measurements, the output on the height levels is combined with fields of the 10 m wind and the 2 m temperature. As the paper also focuses on high-frequency data and daily cycles, the data of the analysis at 00, 06, 12 and 18 UTC are linked to the directly following forecasts. Hourly forecast data was stored in the UERRA project for the lead times of 1, 2, 3, 4 and 5 hours. The motivation for this procedure of time series composition is that each assimilation cycle is bound to its predecessor by a warm-start procedure. We rejected to consider the forecast data for the lead time of 6 hours to 30 hours, because (a) the data are not available and (b) timeseries would still not be seamless.
3.2. UERRA-UKMO
The UERRA-UKMO product is established by the UK Met. Office (UKMO). The Unified Model (UM) comprises a semi-implicit semi-Lagrangian formulation to solve the non-hydrostatic compressible equations (ENDGAME, Wood et al., Citation2014). The UM is applied using 63 levels up to 40 km height and a latitude/longitude grid with 12 km resolution. Assimilation is performed on a 6-hour cycle using a 4D variational (4D-Var) scheme. That is, each analysis at time t uses observations throughout a 6-hour interval lasting from to
With the screen-level observations, a surface analysis scheme is applied. Moreover, the 10 m wind speed measurements are assimilated for stations located below 500 m height and only if the difference between the station height and model height is less than 200 m. For further details about UM and its data assimilation, the reader is referred to Wood et al. (Citation2014) and Rawlins et al. (Citation2007). For the physical parameterizations in the UM, the reader is referred to Walters et al. (Citation2017)
The UERRA-UKMO model outputs are provided on a rotated longitude/latitude grid. The available output levels and times are the same as it is for UERRA-SMHI. The composition of the time series is also created the same way as was done for the UERRA-SMHI product.
3.3. COSMO-REA6
COSMO-REA6 is a regional reanalysis performed by the University of Bonn in close cooperation with the German Weather Service’s Hans-Ertel Centre for Weather Research (HErZ). The lateral boundary conditions used are the same as in the UERRA project (ERA-Interim). The simulation is performed with the non-hydrostatic COSMO model using 40 terrain-following levels with geometric height coordinate and a grid spacing of 0.05° (approximately 6 km). The temporal integration is done using a two time-level, third-order Runge-Kutta scheme. The horizontal advection of wind components is performed with a third-order upwind scheme, and the vertical advection is performed by a third-order implicit advection. The scalars are transported using a second-order finite volume scheme and Strang splitting. More details about the COSMO model and the COSMO-REA6 configuration can be found in Baldauf et al. (Citation2011) and Bollmeyer et al. (Citation2015).
The assimilation of data is realized by a continuous nudging of the model to the observational data (Bollmeyer et al., Citation2015), which is supplemented by surface analysis of snow depth (6-hourly), SST and soil moisture (at 00 UTC). Note that the continuous nudging modifies the prognostic variables by analysis increments without consideration of the correlation between wind and mass fields. Therefore, a balancing procedure is applied: the pressure analysis increments at the lowest level are balanced by means of a hydrostatic upper-air temperature correction. Then, the mass and wind fields are balanced by a geostrophic correction. Finally, the upper-air pressure analysis increments are corrected by a hydrostatic balancing with the mass field. Only the balanced analysis increments are used to be added to the model equations. For further details about the data assimilation cycle, the reader is referred to Schraff and Hess (Citation2003) and Bollmeyer et al. (Citation2015).
The COSMO-REA6 outputs of interest are provided hourly on the model levels (those 6 located near the surface) and on a rotated longitude/latitude grid. The data are transformed from original model level data at terrain-following geometric heights to height levels above the Earth’s surface (10, 35, 69, 116, 179 and 259 m) applying interpolation methods taken from the source code of the COSMO model. Note, that the uppermost vertical model level provided to COSMO-REA6 users is located below the uppermost measurement height of the flux tower Hamburg.
3.4. CCLM-oF-SN and CCLM-oF
In order to investigate the impact of assimilation effort on the simulation of the PBL and the related sub-daily physical processes, two model experiments were additionally performed based on the setup of COSMO-REA6. First, a simulation with the COSMO model using perfect boundaries, i.e. the model is forced to the ‘truth’ only at the lateral boundaries. This simulation is called CCLM-oF. Second, a simulation with the COSMO model, called CCLM-oF-SN, using additionally the approach of spectral nudging, which is introduced briefly in the following.
In the framework of the CCLM-oF-SN experiment, the large scales of the model simulation are continuously nudged to the driving model, which is the state-of-the-art reanalysis system MERRA2. The observed ‘truth’ on large scales is expected to be represented at a high accuracy by the well-established global reanalysis MERRA2. Thus, spectral nudging keeps the simulation close to a state consistent with the observations. The spectral nudging method has been proven several times to have a positive impact on the reconstruction of the atmospheric state (von Storch et al., Citation2000; Geyer, Citation2014; Li et al., Citation2016). The spectral nudging is explained in detail by von Storch et al. (Citation2000). In short, it is a forcing of a simulated and Fourier-transformed field to the reanalysis field
(1)
(1)
and is performed after the calculation of the forcing due to all physical and dynamical processes
This approach requires to transform the reanalysis and simulation data from grid point space to spectral space and vice versa. The following specifications are defined:
prognostic variables X chosen for nudging,
the cutoff regarding zonal m and meridional wave numbers n,
the nudging coefficient and its vertical profile G(z), and
the temporal frequency at which spectral nudging is performed.
We utilize the horizontal wind components for assimilation down to a wavenumber cutoff equal to ≈380 km. The assimilation of rotational or divergent components would be beneficial, as shown in Schubert-Frisius et al. (Citation2017), but the related procedure is not practical for the COSMO model due to backward and forward transformations between wind components and vorticity and divergence. The number of assimilated variables should be kept small to ensure that the model can adapt to the disturbance introduced by the nudging term. Moreover, the vertical profile of the relaxation is chosen in such a way that above the PBL (850 hPa), the strength of the nudging is successively increasing following a parabolic-like function and is in line with the recommendation from Schubert-Frisius et al. (Citation2017) and Hong and Chang (Citation2012). The e-folding time at 700 hPa is chosen to be comparable to the optimal choice found by Schubert-Frisius et al. (Citation2017). The lower boundary for spectral nudging is occasionally chosen to be higher (750 hPa, Schubert-Frisius et al., Citation2017) due to orographic characteristics. Finally, the relaxation is applied at a cycle of 4 time steps for efficiency reasons.
The advantage of such an assimilation configuration is that the integration is disturbed only above the PBL, i.e. the model is free to develop its own variability in the PBL – but with certainty only within the range dictated by the ‘observed’ dynamics in the middle and upper troposphere. Regarding the near-surface physics, the simulation quality tends to be homogeneous because the near-surface observations are disregarded, and thus, no treatment of time-dependent quality of measurements or relocation of measurement sites is needed.
The model experiments CCLM-oF-SN and CCLM-oF are not integrated for the whole COSMO-REA6 period (1979–2017), but for the two years of 2006–2007. Keeping the long spin-up for obtaining the soil processes in an equilibrium state in mind (Yang et al., Citation2011), the soil moisture and other soil characteristics are taken from a 25-year coupled atmosphere-soil simulation with half of the horizontal resolution (≈13 km) created by Geyer (Citation2017). Then, the initial atmospheric state is extracted from the MERRA2 reanalysis. After a spin-up phase for November and December 2005, a continuous integration is performed for 2006 and 2007 utilizing the MERRA2 reanalysis as lateral boundary conditions with a frequency of every 3 hours and a horizontal resolution of approximately 50 km (Rienecker et al., Citation2011; Gelaro et al., Citation2017). The model domain used for CCLM-oF-SN and CCLM-oF is shown in and consists of 531 by 456 grid points.
As multi-year long-term simulations are evaluated in our study, the configuration of the COSMO model needs to be adapted for the climatological scale. COSMO-REA6 was configured unintentionally to run with a surface albedo dependent on soil type but fixed in time. For CCCLM-oF and CCLM-oF-SN, the albedo is chosen to be dependent on the soil moisture too in order to capture properly the feedback between soil and near-surface temperatures (large albedo values for dry soil and reduced albedo for wet soil). This is compensated in COSMO-REA6 by the surface analysis. In addition, the CCLM-oF-SN and CCLM-oF configurations were adapted following the recommendations of the CLM-Community: i.e. (a) the vertical extent of the soil layers is slightly different, (b) the type of root distribution is not uniform but exponential, (c) the calculation of heat conductivity considers not only soil moisture but also soil ice and (d) the parameterization of bare soil evaporation is not according to Dickinson (Citation1984) (BATS version) but set according to Noilhan et al. (Citation1989) (ISBA version). Finally, the resolution is chosen to be consistent with the operational NWP-setup for Europe (500 m coarser than COSMO-REA6) and the model output of CCLM-oF-SN is more comprehensive than in COSMO-REA6 (CCLM-oF-SN is intended to deliver even 15 years of data to specific users in the energy system modeling community).
4. Evaluation methodology
The evaluation is performed using the HZG-EvaSuite with source code adaptations (available at http://gitlab.dkrz.de for registered users). A Python program arranges the operations on the raw data of model simulations as well as reference datasets needed to arrive at exactly the data required for the evaluation: harmonized data in time and in space according to the frequency and grid chosen. Finally, the program computes the metric of interest and plots the result depending on the data dimension and user needs. The program extensively uses the package ‘xarray’ (Hoyer and Hamman, Citation2017). Interpolation is performed with the climate data operators (CDOs) developed and maintained at MPI Hamburg, https://code.mpimet.mpg.de/projects/cdo/.
4.1. Interpolation of model grids to tower grids
All model data must be interpolated to the location and levels of the tower’s measurement devices. A bilinear interpolation is applied for the scalar variables. Regarding the UERRA wind products, a nearest neighbor approach is applied for the determination of wind at the tower’s location. Regarding the COSMO wind products, the components are first interpolated to the tower’s location, and then the wind magnitude is calculated. The strategy for the vertical interpolation of model data is based on numerical discretization. The horizontal wind components are located on a staggered grid on so-called full levels, whereas other quantities (vertical velocity, turbulent fluxes) are located at layers in between the full levels. A reconstruction is needed during the discretization to harmonize the position, which is in our case realized by a second-order interpolation scheme for all models. Thus, we do not mimic any physical laws such as logarithmic profiles, but we rely on interpolation methods inherently used by numerical models during integration time.
The measurement averaging intervals must be harmonized with the instantaneous model output. Only those measurement values that approximately met the time stamps of the model data (hourly frequency) were reselected from the time series. The 10 min averaging period used for observational data is comparable with the instantaneous model output because the Reynolds-averaged models do not explicitly resolve the high-frequency spectra of turbulence; hence, all model variables are inherently subject to an averaging process.
4.2. Stability-dependent analysis
The stability in the atmosphere mainly controls the speed and directional shear of the wind within the PBL (Stull, Citation1988). Therefore, in the framework of our evaluation, the PBL is investigated under different regimes of stability. It is a common procedure to define the stability following the concept of Monin-Obukhov similarity theory (Monin and Obukhov, Citation1954). The widely used stability parameter (where L is the Obukhov length) provides an estimate of the ratio between buoyancy and shear effects in the lower boundary layer. Upward-directed turbulent heat fluxes are characteristic of unstable boundary layers, and downward-directed turbulent heat fluxes are characteristic of stably stratified boundary layers. The determination of the Obukhov length requires high-frequency eddy-covariance measurements; because the flux towers are not operationally equipped with such devices, we follow another approach for our stability analysis (also omitting the Richardson number approach).
One simplified measure for stability is the stratification of the atmosphere, i.e. the static stability. It is defined by the vertical gradient of the potential temperature. Considering the data available from tower measurements, the static stability is straightforward to compute for all masts. To obtain comparable results, we calculate the gradient of temperature from 10 m to those levels at each mast located closest to 100 m (), i.e. 80 m height for Cabauw, 98 m height for Falkenberg and 110 m height for Billwerder. The stratification is then classified as very unstable (referred to as ‘us’), near neutral (‘ns’), stable (‘st’) or very stable (‘vs’), as listed in . Previously used classification for the investigation of flux tower data have been finer (Mohan and Siddiqui, Citation1998). However, the use of (perhaps) seven stratification classes requires a vast sample size that is very likely not achieved when considering a period of a few years. The models are not able to resolve single events of very strong or unstable stratification pushed by onsite characteristics.
Table 3. The static stability classes used for investigation. The classification is shown to be dependent on the vertical gradients of (potential) temperature. Moreover, the likelihood of occurrence [%] of static stability is listed for the flux towers.
The static stability classes are extracted from the observations in an hourly cycle, i.e. the measured profiles are averaged over one hour, and the mean stratification is analyzed.
4.3. Time series analysis
Gupta et al. (Citation2009) proposed the Kling-Gupta efficiency (KGE) to analyze time series in hydrological modeling. Because the variability, correlation and bias affect the efficiency, KGE is also chosen in our investigation to examine the temporal development of the lower PBL. KGE is written as follows:
where r is the correlation coefficient and σr is the ratio between the variability of the model and observation. Moreover, μr is the relation between the mean value of the model and the observation. The coefficients sr,
and
are weighting factors to control the influence of the three different components on the KGE. These coefficients are specified later on in the discussion of the results.
4.4. Quantitative evaluation of the simulated distribution
As a visual inspection of the differences in probability density functions is hard to achieve, a quantitative evaluation is done (a) comparing Weibull fitting parameters to the distribution of wind speed and (b) calculating the earth mover’s distance EMD (also referred as Wasserstein distance Rabin et al., Citation2008; Frank et al., Citation2020). The Weibull distribution is known to sufficiently describe the observed distribution of wind, and a corresponding comparison between the fitting parameters of the simulated and observed wind speeds serves as an indicator of model performance (Kaiser-Weiss et al., Citation2015). The 2-parameter Weibull distribution is defined by the shape and the scale parameters:
Depending on the shape parameter β, the Weibull distribution is exponential (β = 1), positively skewed (right tail) or negatively skewed (left tail). The positive skewness is strengthened for values close to ‘1’ and becomes weaker for larger values. In the range between approximately and
the distribution becomes Gaussian. For even larger values, the distribution is negatively skewed. Furthermore, if the scale parameter η is increased, the distribution is stretched out and the peak of the distribution is decreased.
EMD measures the ‘work’ needed to rearrange the simulated distribution such that it fits the observed distribution. This score can be understood as the transportation cost to move a unit mass from one bin to another bin, and in addition the amount of mass carried from one bin to another. For the one-dimensional time series considered here, EMD equals the distance between two cumulative density functions F and G (Rabin et al., Citation2008):
4.5. Analyses of ramps
The short-term variability of the wind speed is of great interest to wind energy companies because it controls the variability in power generation. The generated power is defined mainly by the characteristic ‘power curve’ of a wind turbine. Thus, in our model evaluation study, the power curves are employed following the approach of Bianco et al. (Citation2016). The characteristics of the power curve considered here are illustrated in : a cut-in wind speed of 2.0 ms–1, an increase in power production following a polynomial of 7th order, a maximum power capacity reached at 16.0 ms–1 and a cutoff at 25 ms–1. These characteristics are comparable with the widely installed wind turbine ENERCON E70. For simplicity, the density is assumed to be constant, and the generated power is expressed in terms of the power capacity factor PCF, which is the power normalized by the full-rated power capacity.
Fig. 2. Characteristic power curve as given by the ENERCON E70 wind turbine (2310 kW, with a diameter of 70 m).

The fluctuations and rapid changes in PCF are analyzed and evaluated using ramp statistics, where a ramp is a large change in wind power over a short period of time. Ramp statistics are obtained by analyzing ramp events: counts, duration or amplitude. The crucial issue with ramp statistics is the mathematical definition and detection of ramps. There are few methods proposed in the literature to define and detect ramps (Sevlian and Rajagopal, Citation2013; Bianco et al., Citation2016). Considering the amount of data to be analyzed, we utilize a straightforward approach:
(2)
(2)
where β is a threshold with a minimum value of 0.15 and a maximum value dependent on the window length
(see the Results section). A negative step change is considered to be a down-ramp, and a positive step change is considered to be an up-ramp. Yang et al. (Citation2013) also applied the method in EquationEq. (2)
(2)
(2) (his second method) and evaluated the WRF model for the ability to predict ramp events in complex terrain. One drawback of this method is the missing merging of consecutive step changes to one ramp event, which is much harder to detect and computationally more expensive.
Because reanalysis experiments assimilate observations and thus a high temporal consistency is expected between the truth and the model, we push the evaluation of ramps towards temporal coherence. The aforementioned method of ramp detection brings us to a binary sequence for up- and down-ramps, and contingency-based scores are dedicated to evaluate such types of data (Yang et al., Citation2013). In this study, the probability of detection and the success ratio
(3)
(3)
are used to rank the model’s ability in timing (optimal score: 1). A hit is considered to be an overlap between an observed and simulated ramp, whereas no consistency appears in the case of a missing simulated event (MISS) or a false alarm (FA).
5. The lower PBL and its representation in hindcasts
5.1. Representation of the wind’s diurnal cycle
Considering the methodology explained in the previous section, a comparison is performed for the diurnal cycle of wind speed at the flux towers. The hourly values of a 2-year time series (2006–2007) were grouped for all 24 hours of a day, and for each hour of the day a mean was calculated (diurnal cycle). As the UERRA-products are not continuous simulations or do not use continuous nudging, it is expected to address some drawbacks with the daily cycles. Hourly forecast fields with lead times from 6 to 30 hours would give the opportunity to test at least the forecast’s performance but this was not considered for the UERRA data plan. illustrates the vertical profile at Hamburg and for the years 2006 and 2007 (note that spline interpolation was used to create a filled contour plot with an increment of 0.3 m s−1 and that measurements are available at 5 levels only). The tower data () clearly depict the subdaily variability of the PBL due to the shortwave radiation input at the Earth’s surface (Stull, Citation1988). Near the surface, calm wind conditions during the night disappear during the day thanks to buoyancy-driven turbulent mixing. In contrast, the observations above 150 m reveal comparably low wind speeds during the day due to the turbulent transport of large momentum from the upper levels down to the surface and vice versa. The high wind speeds during the night are associated with inertial oscillations developing in a stable and flat nocturnal boundary layer, which is decoupled from the residual layer above (Stull, Citation1988; Van de Wiel et al., Citation2010).
Fig. 3. Hamburg, diurnal cycle of wind speed [m/s] (hourly values) for the whole year 2006–2007 for observation (a) and five different assimilation experiments (b–f). Note, that the data for COSMO-REA6 is not available above 250 m.
![Fig. 3. Hamburg, diurnal cycle of wind speed [m/s] (hourly values) for the whole year 2006–2007 for observation (a) and five different assimilation experiments (b–f). Note, that the data for COSMO-REA6 is not available above 250 m.](/cms/asset/f65ee4e8-68c3-4372-adaa-854ab895620b/zela_a_1804294_f0003_c.jpg)
The aforementioned main features of PBL development can also be observed at other tower locations in European flatlands ( and or the study of Van Ulden and Wieringa, Citation1996). The diurnal variation is also depicted by the models with continuous nudging or no assimilation at all. The CCLM-oF simulation offers wind speed predictions that are often overestimated at the upper levels (), and the error is reduced in the assimilation experiments CCLM-oF-SN and COSMO-REA6 (). The prediction of wind speed conditions near the surface and particularly at night is challenging. Errors can be attributed to the imperfect representation of roughness characteristics and orographic features at the site location (e.g. discussion about uncommon wind steering in the Elbe river valley by Brümmer et al., Citation2012).
Fig. 4. Same as but for the tower at Falkenberg and only for the months of May to September in 2006 and 2007.

Fig. 5. Same as but for the tower at Karlsruhe and only for the months of May to September in 2006 and 2007.

From all sophisticated reanalyses, COSMO-REA6 offers the smoothest transition of PBL development. Here, the continuous analysis increments are corrected for a hydrostatic and geostrophic balance (Bollmeyer et al., Citation2015). Only the renewed assimilation cycle and surface analysis at 00 UTC lead to spurious leaps at the upper levels (). In contrast, the UE-SMHI reanalyses show moderate to strong interruptions in PBL development that increase with increasing height (). The model field is altered every 6 hours in the variational analysis to better match the observations (radiosonde stations near Hamburg: Bergen, Schleswig, Emden). Starting the integration from the analysis, the model physics generates its own solution, i.e. an abrupt increase in wind speed is detected during spin-up (see also and ). This phenomenon is heavily suppressed near the surface (10 m height). The producers of UERRA-SMHI did not face that problem analyzing near-surface winds and temperature (Semjon Schimanke, personal comment). One issue with the elevated levels could be that the initialization of model integration is performed from unbalanced turbulence, i.e. the forecast starts with zero TKE (Semjon Schimanke, personal comment). Thus, the turbulent fluxes must be reinitialized. Nevertheless, the high nocturnal wind speeds at the upper levels are resolved in the forecast at all three locations, but the midday increase in near-surface winds is hard to detect for Billwerder.
Considering the UE-UKMO reanalysis, strong interruptions in boundary layer development are dampened. Two main features are captured with the 4D-Var system: the determination of time-evolving analysis allows a better treatment of temporally spread observations within an assimilation window. In addition, it enables an analysis of the temporal development of correlations between variables, flow-dependent structures and error calculation in the background state (Lorenc and Rawlins, Citation2005). Therefore, 4D-Var is much more likely to provide a better balanced analysis field than 3D-Var, but the state of reanalysis is further from the observations. One issue to mention regarding diurnal variations is the linearized and simplified model used for UKMO analysis, which provides only a coarse parameterization of the PBL processes (Lorenc and Rawlins, Citation2005). However, the UE-UKMO reasonably resolves the midday increase in near-surface winds at all three locations, but the simulated diurnal cycle at the upper levels does not fully capture the measurements (). This result occurs because a smooth increase in wind speed is resolved for ongoing lead times up to the next assimilation window. One may conclude that shortcomings exist in roughness characteristics, but this aspect cannot be further examined because the roughness length from the pure model output is meaningful only over the sea – over land the roughness length is calculated within the online-coupled land surface model JULES (Best et al., Citation2011; Clark et al., Citation2011) considering individual tiles within one single grid cell (e.g. forests with season-dependent leaf area index and canopy height, snow-covered surfaces, and urban areas).
The boundary layer characteristic is dependent on the growth rates of the PBL depth, and thus, tower data are occasionally studied separately for different seasons (Brümmer et al., Citation2012). One distinctive feature of the summer PBL at Falkenberg () is a considerable short-term deceleration of wind speed in the morning. Due to radiative forcing in the morning, the decoupling between the stable nocturnal boundary layer and the residual layer above is dissolved (Neisser et al., Citation2002). Thus, the upper levels ‘feel’ the surface friction again, and the wind speed is reduced. This process is partly resolved by all simulations, although 4D-Var and 3D-Var tend to be influenced by the analysis cycle and related increments (); SYNOP and radiosonde station Lindenberg are located only 4 km away. In addition to the morning, a deceleration peak also occurs in the evening hours. The impact on the wind speed is reasonably resolved for the hindcasts (). However, COSMO-REA6 is the closest of the models to the measured wind speed data, which could be explained by a comparably well-resolved surface roughness ( and ) and a forcing of the modeled wind speed to nearby observations. The issue of high wind speeds at night developing in UE-SMHI is discussed in Section 5.4.
The flux tower observations at Karlsruhe () reveal a distinctive feature of PBL midday development in the upper levels that is not captured by the models (). The morning deceleration peak is followed by only small changes in the wind speed until late evening. The wind speed characteristics at the site location are difficult to simulate because the measurements near the surface are taken over treetops. The flow and related frictional forces are displaced mainly above the trees (concept of zero displacement). When further considering the long fetch of landscape roughness at the upper mast levels (Verkaik and Holtslag, Citation2007) due to the daytime increase in the larger-scale wind speed, the models are not able to capture the different footprints forming a complex boundary layer profile at Karlsruhe. At the least, a sufficient resolution and treatment of flow through porous media is missing.
To summarize for all towers, the Kling-Gupta efficiency (KGE) is calculated on the mean diurnal cycle in 2006 and 2007 separated between the ‘summer’ (May to September) and ‘winter’ (November to March) seasons (). The weighting coefficients for KGE are chosen to be and
thereby placing more emphasis on temporal development than mean values. All models depict the lower PBL better in summer than in winter. The diurnal variability is strongly driven by the PBL growth rate in summer, but vertical mixing processes within fronts dampen the PBL’s diurnal cycle in winter, and thus, the signal from assimilation cycles is enhanced. Moreover, the models fail to simulate the transition zone at Karlsruhe (40 to 80 m). Finally, the best performance of PBL development is observed for the continuous model integrations CCLM-oF-SN (mean over all flux towers for summer/winter, 0.61/0.50) and CCLM-oF (0.56/0.39) as well as for COSMO-REA6 (0.48/0.26). Another crucial point is that all three models offer a resolution of nearly 6–7 km, which means that the characteristics at the site locations are two times more finely resolved than in the UERRA simulations. Here, the 4D-Var analysis appears to be superior to the 3D-Var analysis, particularly for winter, when spin-ups disrupt the diurnal variation.
Table 4. Kling-Gupta efficiency for the mean diurnal cycle in ‘MJJA’ (summer) and ‘NDJFM’ (winter). The towers are listed, and the heights are grouped for the near-surface, above 100 m and in between. The bold numbers indicate that the performance exceeds 0.4, which requires, for instance, and
Model names are shortened by omitting the first part.
5.2. Characteristics of the mean wind profiles
To further understand the reanalysis’ error characteristics, the distributions of the simulated wind are compared with the distribution of the measurements. With such an analysis the temporal similarity between two data sets is ignored, which is later discussed in Section 5.5. We limit ourselves to the common analysis times at 00, 06, 12 and 18 UTC to filter out the aforementioned transition problems occurring between analysis and forecast.
The estimations of the shape and scale parameters of the Weibull distributions fitted to measurements at the flux towers (performed with the scipy package in Python) are listed in along with the values for the simulations. Compared to 10 m observations discussed by Kaiser-Weiss et al. (Citation2015), the range for the shape parameters is narrower because topographic features are smoothed out at elevated levels. The distributions observed at the towers are positively skewed, which is reasonably resolved by all simulations. The skewness is most pronounced at Karlsruhe, where the models even overestimate the positive skewness. The increase in the scale parameter with increasing height is also sufficiently depicted by the reanalyses, i.e. the distribution is stretched to the right because of higher wind speeds entering the data sample. Although the model performance depends on the location, it is clear that CCLM-oF suffers from missing assimilation. The overestimation of the scale parameter at the upper levels implies that higher wind speeds appear too often (see the diurnal cycle), and thus EMD is the worst of all models (overall mean: 0.54 m s−1).
Table 5. Estimation of the shape parameter (first number in each cell) and scale parameter (second number) of the Weibull distribution with respect to the selected measurement locations. Model names are shortened by omitting the first part. The ‘star’ indicates that the uncertainty of parameter estimation is larger than 0.1. In addition, EMD is given [m s−1].
Slightly more realistic distributions are simulated in the case of UE-UKMO (EMD: 0.47 m s−1) and UE-SMHI (0.42) as well as large-scale nudging (CCLM-oF-SN, 0.39). Even better is the result for COSMO-REA6 (0.24). CCLM-oF-SN as well as COSMO-REA6 benefit from a higher horizontal resolution than the UERRA products. Therefore, the CCLM-oF-SN even compensates for the missing sophisticated assimilation of PBL measurements (radiosondes, SYNOP reports) done in the UERRA reanalyses. This is also underpinned by the bias of wind speed computed as the average of all absolute values of bias over all measurement locations (not shown). In general, an overestimation of the wind speed is detected for all models in ‘summer’ (MJJAS) and ‘winter’ (NDJFM). The bias of CCLM-oF-SN (0.5 m s−1 in winter and 0.3 m s−1 in summer) is comparable to that of UE-SMHI (0.5 and 0.2) and UE-UKMO (0.7 and 0.5). The bias of COSMO-REA6 is still smaller, i.e. 0.3 and 0.1 m s−1. Here, the simulation of wind speed in the PBL very likely benefits from the combination of a) a comparably high resolution and b) continuous nudging, especially of near-surface winds at nearby SYNOP stations.
To further investigate the model errors, the vertical profile of the median bias is calculated and is shown in for Cabauw and Falkenberg. The vertical gradient is well resolved at noon and in summer (nearly constant error with height, red lines in , upper and central panel), where conditions of a well-mixed boundary layer and logarithmic wind profiles are expected to be well resolved in models. An exception is UE-UKMO at Falkenberg (, upper right), and related deficits were already addressed for the diurnal cycle but will also be further discussed for the temperature profiles in Section 5.4. Nighttime at summer is more problematic to simulate. For instance, the vertical gradient at Cabauw is underestimated below 100 m height in almost all simulations. The error is damped in the UE-SMHI data for levels below 50 m, but an error remains for the levels above (straight blacks line in the upper left of ). De Rooy and de Vries (Citation2017) also showed a systematic underestimation of the wind speed gradient for the operational model at that time and referred to a stability dependency. Furthermore, at Falkenberg, the magnitude of the model error in vertical gradients reduces compared to that of Cabauw, and here the UE-SMHI offers a good performance over the whole profile. We refer to Section 5.4 for a further discussion.
Fig. 6. Median wind speed bias at 00, 06, 12 and 18 UTC at the towers Cabauw (left panel) and Falkenberg (right panel). Top: The UE-SMHI (solid lines, lower x-axis) and UE-UKMO reanalyses (dashed lines, upper x-axis) are shown with respect to the years 2006 and 2007 for summer. Middle: The same is shown but for CCLM-oF-SN (solid lines, lower x-axis) and COSMO-REA6 (dashed lines, upper x-axis). Bottom: The same is shown as in the middle panel but for winter.

The winterly gradients in wind speed are more problematic to simulate at Cabauw and Falkenberg (only COSMO models, lower panel in ). The gradient at noon is always overestimated. In addition, the error in the nighttime profiles even changes with height at Cabauw, i.e. from underestimation to overestimation. In general, the COSMO model products share error characteristics for the vertical gradient in wind speed with a shift of approximately 0.3 m s−1. This implication also holds for Karlsruhe (not shown), where a pronounced underestimation of the vertical gradient occurs due to a missing representation of frictional-induced loss of momentum for flow over treetops. Despite different assimilation methods, the turbulent exchange processes of momentum in the boundary layer appear to be very similar. Considering a continuous integration without spin-up issues, our findings underpin the assumption that two main factors exist regarding insufficiently resolved vertical wind profiles and diurnal cycles: (1) the representation of topographic features improving using increased resolution, and (2) the PBL parameterizations. Therefore, the stability of the atmosphere is examined, one of the main influencing factors for PBL parameterizations.
5.3. Diurnal cycle of occurrence for different stabilities
In addition to the dynamical processes at fronts, the wind profiles are highly driven by the stratification of the atmosphere. Assuming a very stably stratified lower PBL, the turbulent mixing of momentum is reduced and a strong wind shear develops (Cuxart et al., Citation2006). Moreover, destabilization of the lower PBL enhances the turbulent exchange of momentum, and the gradient in wind speed is distributed over a growing boundary layer. Regarding the simulated wind profiles, different closure concepts, length scale formulations, stability-dependent functions and physical constants additionally control the shape of the wind direction and speed shear (see the discussions in Cuxart et al., Citation2006; Bengtsson et al., Citation2017). To obtain insights into the height dependency and diurnal variation of the model errors, the observed and simulated stratifications are compared with each other. The static stability (Section 4.2) is calculated for the measurement data as well as for the reanalysis experiments.
Note that the stratification over the location Karlsruhe is not analyzed here because of the thermal exchange processes over treetops are considered to be unresolved by models.
The observed diurnal cycle of stratification frequency is shown in (straight lines) for the towers at Cabauw, Falkenberg, and Billwerder. During summer (left figures), stable to very stable stratification dominates at night. The transition to day is accompanied by a local maximum of ‘st’ cases (see and ), and neutral to unstable stratification dominates during the day. The portion of unstable conditions is higher at Cabauw than at other towers, but the layer considered for stratification calculations is slightly thinner at Cabauw (70 m) than at Falkenberg (90 m) and Hamburg (100 m). This is a crucial issue regarding superadiabatic layers.
Fig. 7. Diurnal cycle of occurrence of 4 different types of stratification () for summer (‘MJJAS’, left) and winter (‘NDJFM’, right) and at towers Cabauw (a, b), Falkenberg (c, d) and Billwerder (e, f). The straight lines indicate the absolute frequency measured, and the symbols indicate the absolute frequencies simulated (‘x’: UE-UKMO, rectangle: UE-SMHI, range indicator: COSMO models). For readability, the simulated frequency of ‘st’ cases is shown only in winter. The colors belong to different stabilities (legend of the figures in the top row).

The simulated diurnal cycle of stratification in summer is, in general, reasonable and best represented at the Cabauw site (symbols in ). Here, the ratio between unstable and neutral conditions over daytime is well depicted. In contrast, the model quality is poor for unstable stratifications at Billwerder and Falkenberg. The ‘us’ cases are overestimated by a factor of 1.6 to 2.0 at the expense of neutral and stable conditions (‘st’ not shown because of readability). The error is larger for the UERRA products than for COSMO and is dependent on location. Although the diurnal variation of the ‘vs’ cases is convincing, they appear less frequently than those observed at night at Falkenberg and Cabauw. This is not the case for UE-SMHI. Furthermore, for some reason, the COSMO models tend to erroneously preserve some neutral stratifications overnight. This result might originate from missing effective radiative cooling conditions (too many clouds) or insufficient physical parameterization.
During winter, stable stratification dominates over the entire day and at night due to cyclonic activity (). The dominance is remarkable at Billwerder, but – among the weather conditions – the physical properties of the soil differ from those at other towers, i.e. meadow soil vs. sandy soil and river clay. The comparably small variation in ‘st’ cases over the day is also identified in the simulation experiments. However, at noon, the ratio between stable, neutral and unstable stratification is different in the models than in the observations, particularly at the location Billwerder. This reallocation is also related to the errors in wind profiles shown in (12 UTC). Investigating the monthly variation of false alarms regarding the ‘us’ cases reveals that too many events are simulated in February and March. Furthermore, the diurnal cycle of very stable stratification is sufficiently resolved in all models, although the reanalyses overvalue the development of very stable layers during the afternoon and night at Billwerder.
In general, it turns out from sensitivity experiments that our findings are robust and more or less independent of the threshold value chosen for stability classification (not shown). Moreover, the gradients derived from the model output might be associated with some uncertainties in reconstructing the simulated temperature at specific levels of the measurement devices, but the sensitivity turns out to be small as discussed in Appendix C. Finally, we can examine that the redistribution between stratification classes is challenging to resolve, particularly at Falkenberg and Billwerder and at noon. A relationship between the deficits of stratification statistics and the errors in vertical wind profiles becomes obvious for the winterly PBLs at noon: large deficits in the profile coincide with an incorrect ratio between stable and unstable cases. Other relationships are not straightforward. However, the UE-SMHI never underestimates cases of very stable stratification, and the related nighttime vertical gradients are convincing for the lower levels (, straight lines).
5.4. Gradients in the wind speed and temperature under different stratification regimes
One drawback of our previous stability analysis is that we assumed a homogeneous stratified layer in the lower PBL. In reality, the lower PBL might develop under changing weather conditions, and different stratifications are layered on top of each other. Therefore, the atmospheric profiles at each flux tower are computed such that they coincide with those time periods where one of the four stability classes ‘vs’, ‘st’, ‘ns’ and ‘us’ was detected. The model data are now joined with the observations, but only the distributional statistics are evaluated. The weakness of gradient calculations is the resolution of the measurement devices, e.g. approximately 0.01 K m−1 or 0.01 m s−1 m−1 for the lowest levels. However, the observational means are still reliable here thanks to the sample size of at least 1500 profiles for each tower and stability class. Moreover, we exclude the 10 m level from the wind gradient calculations to prevent roughness effects from becoming a dominant factor.
The profiles of the temperature gradient are shown in with respect to the full period 2006–2007 and for the stratifications ‘vs’ and ‘us’. In the case of unstable stratification, superadiabatic gradients are limited to the near-surface levels (as known from radiosonde or, e.g. remote-sensing Emeis et al., Citation2004). For the levels above, the lapse rate tends to be that for dry-adiabatic motions (approximately −0.01 K m−1). The different assimilation experiments also resolve a clear transition to dry-adiabatic lapse rates above 50 m height. Moreover, the observations indicate that the uppermost levels occasionally offer stable stratification (e.g. ), whereas the models simulate solely neutral conditions. Brümmer and Schultze (Citation2015) showed that during morning hours, near-surface unstable stratification can be superimposed by an elevated stable layering (such as an inversion).
Fig. 8. Temperature profiles depending on the stratification at Cabauw (a), Falkenberg (b) and Billwerder (c). Modified box plots are shown for the observations (gray), COSMO-REA6 (dark blue), CCLM-oF-SN (light blue), UE-SMHI (green) and UE-UKMO (red). The upper part of each figure refers to the very stable stratifications, and the lower part refers to the unstable stratifications. ‘Modified box plot’ means that only the upper and lower quartiles of the data are visualized by the box, and the median is indicated by the straight line in the box and the mean by the small black rectangle. Furthermore, transparency is used with the last gray boxes at Billwerder to distinguish between the data. Moreover, the horizontal dashed lines indicate the transition from a linear scale to a logarithmic scale (see the y-axis).

The near-surface superadiabatic gradients are overestimated particularly by the UE-UKMO reanalysis (and somehow by UE-SMHI); the 75th percentile of UE-UKMO ends at −0.06 K m−1 for Falkenberg. Such steep gradients should not sustain because turbulent exchange processes quickly dissolve near-surface overheating. One could speculate with the PBL formulation applied (Walters et al., Citation2017) that strong sensible heat fluxes massively heat the lowest 10 m but mixing to the levels above is suppressed. Consequently, the wind speed gradient is overestimated by UE-UKMO at the stations of Cabauw and Falkenberg over the whole range of gradients observed. This is seen in the quantile-quantile plots in , where the quantiles of the observed distribution are compared against the simulated distribution. However, the distribution of gradients at Falkenberg suggests deficits with all models regarding the ‘us’ cases, which is underpinned by the median bias profiles at 12 UTC (, right). And it is very unlikely that other stabilities enter the simulation results as indicated by .
Fig. 9. Two-year quantile-quantile plot of the vertical gradient in wind speed depending on the stratification at Cabauw (a) (80 m to 20 m), Falkenberg (b) (80 m to 20 m) and Billwerder (c) (110 m to 50 m). Each second quantile (p = 0.02, 0.04, 0.06,…, 0.98, 1.0) of the distribution of simulated gradients is plotted against the quantiles of the observed gradients. The upper part of each figure refers to the unstable stratifications (upper x-axis and left y-axis), and the lower part refers to the very stable stratifications (lower x-axis and right y-axis).

A positive temperature gradient is present in the case of stably stratified PBLs (, upper part). In particular, near the surface, the mean lapse rate reaches values of up to 4 K per 100 m for continental station Falkenberg. The distribution is always positively skewed for the observations and for the UE-SMHI and UE-UKMO reanalyses. The COSMO models fail to reproduce (1) the strong stratification and (2) the positively skewed distribution. In general, clear-sky nights with a negative net radiation budget lead to a drop in temperature at near-surface levels, but turbulent sensible heat fluxes from the atmosphere down to the near-surface act against radiative cooling. However, the generation of turbulence is crucial in stably stratified flows with minimal wind shear. In the COSMO model (a level 2.5 closure formulated in 1-D for the case of climate reanalysis, Baldauf et al., Citation2011), the concept of a threshold of minimum vertical turbulent exchange is applied to prevent the TKE from vanishing. Moreover, thermal circulations, resulting from differential heating over patchy surfaces or complex orography, are considered to give rise to a TKE source (Matthias Raschendorfer, personal comment).
From the perspective of stable stratification, the UERRA reanalyses offer the most convincing simulation results at all flux towers. In contrast to COSMO model results, a reduced coupling of near-surface levels with the levels above is detected, i.e. more effective cooling produces more realistic temperature gradients. In this context, the increased number of model levels within the lower PBL is beneficial for the UERRA-SMHI reanalyses (). Moreover, the observed weakening of stratification with increasing height is more suitably captured as in COSMO, i.e. negative medians above 150 m. The corresponding distribution of the wind speed gradients in the lower PBL is the most realistic for UE-SMHI, although an overestimation is apparent at the tail, consistent with cases of overestimated stratification (). de Rooy and de Vries (Citation2017) found with the HARMONIE model system in a comparable evaluation setup at Cabauw that the turbulence scheme used in that system (prognostic TKE closure Cuxart et al., Citation2000) still suffers from excessively strong turbulent mixing of momentum because of too little dynamic stability (gradients underestimated). The results could be further improved by using the HARATU turbulence scheme (Bengtsson et al., Citation2017). Regarding UE-UKMO, the wind speed gradients are not better resolved than for COSMO despite more reasonable temperature gradients. The turbulent mixing at the tail appears to be much too strong.
Evaluating the stratification statistics in terms of a value chain of assimilation reveals for these three specific locations that the COSMO model simulations slightly improve if data assimilation is present: the occurrence frequency of classes ‘ns’ and ‘vs’ slightly better fit the measurements during the second half of night in summer (not shown). Moreover, the temperature profile for very stably stratified boundary layers is slightly better depicted by COSMO-REA6 than by CCLM-oF-SN and CCLM-oF. It is not clear if the stratification statistics changes slightly only as a result of a better representation of nighttime weather conditions or sustained change in near-surface stability due to the assimilation of the 2 m temperature observations. However, the model physics, i.e. soil parameterization and turbulence parameterization, mainly control the stability and further the vertical profiles, and this is shared for COSMO-REA6, CCLM-oF-SN and CCLM-oF. Therefore, no or only minor improvements can be achieved by assimilation (regarding vertical gradients), as reflected by the results in . Regarding the distribution of wind gradients, we even observe slightly worse results for COSMO-REA6.
5.5. Representation of short-term variability
5.5.1. Temporal coherence of mean wind
The characteristics of the errors and the model’s accuracy are analyzed for the wind profiles with the bias-corrected, relative root mean square error (RMSErbc), as suggested by Drechsel et al. (Citation2012). Thus, we minimize the problem that the error calculation is affected by the logarithmic wind profile, which consequently provides larger errors in the upper levels than in the near-surface levels. Moreover, the intercomparison is harmonized by subtracting a systematic long-term bias over the years 2006 and 2007 from the model’s results, because this bias can easily be corrected. The bias is defined as the two-year mean difference between model and observations, separetely for all flux towers and levels.
RMSErbc is determined based on the hourly frequency for all towers and by separating the two seasons ‘MJJAS’ and ‘NDJFM’ (). The error is 5 to 6% larger in the summer season than in the winter season, for all reanalyses and hindcasts. For better understanding, the data were separated according to large-scale weather patterns to evaluate the analysis in light of atmospheric dynamics, as described in Appendix B. It turns out that periods with lower wind speeds are associated with a higher normalized error measure RMSErbc (). Considering only the frequent weather patterns, situations with a high pressure ridge, low over Central Europe and trough over Western Europe dominate the summer season (approximately 40%) and are associated with a large RMSErbc (). In contrast, patterns of western cyclonal and northwestern cyclonal and trough features over Central Europe dominate the winter season (approximately 45%) and are associated with small RMSErbc values. Therefore, it might be concluded that the time series of wind is better depicted during strong synoptic forcing in winter than in those situations, where the time series is strongly influenced by the radiation-driven development of the PBL, small-scale topographic features and convective activities.
Table 6. RMSErbc at each tower for hourly data in ‘MJJA’ (summer) and ’NDJFM’ (winter). The heights are grouped for the near-surface, above 100 m and in between.
In addition to the seasonal dependency, the vertical dependency and the magnitude of the error are small for flat and homogeneous topography but large for the complex terrain at Karlsruhe, where the flow interacts with treetops. This result is consistent with the study of Drechsel et al. (Citation2012), who found the smallest errors with respect to offshore locations.
Because the total model error is influenced mainly by errors in the correlation for the subdaily frequencies considered here (Niermann et al., Citation2019), it is expected that the assimilation of atmospheric data is very important for keeping the atmospheric variability as realistic as possible and the RMSErbc values small. Hence, the experiment without any assimilation offers the worst accuracy. The error is 11 to 13% larger than for UE-UKMO. Although UE-SMHI has more degrees of freedom to adapt to the observations than UE-UKMO, the error is nearly similar to that of UE-UKMO. As already discussed, 3D-Var does not allow for a time-evolving analysis, and it suffers from a comparatively unbalanced state introduced by the observational increments. The latter induces large errors for the lead time of 1 hour for all towers and in particular at the upper levels (not shown). COSMO-REA6 offers an accuracy comparable to that of UE-UKMO. There are some advantages at near-surface levels, but they diminish at higher levels. The reason is the combination of a continuous assimilation of near-surface winds (compare the scores of the COSMO models in ) and the doubled horizontal resolution, which better reflects the surface characteristics.
Although the effort of the CCLM-oF-SN is moderate compared to that of 4D-Var, 3D-Var and COSMO-REA6, its performance is approximately only 5% worse than that of UE-UKMO and COSMO-REA6. We conclude that the assimilation or nudging of atmospheric dynamics, at least in the middle and upper troposphere, is a crucial point for obtaining also a variability at flux towers that is as realistic as possible.
5.5.2. Distribution and detection of ramp events
Considering the transition problems and shortcomings in stability discussed in Sections 5.1 and 5.4, we analyze the performance of the reanalysis experiments regarding short-term changes in the wind speed. The ramp analysis (Section 4.5) is referred to as a tool to investigate the frequency distribution of variability in generated power for thresholds ranging between and
The corresponding shows the cumulative distribution function of ramp rates for two different temporal reference periods: (1) only the times 00, 06, 12 and 18 UTC are analyzed with a window length of six hours (subfigures a and b, all towers), and (2) all times are analyzed with a window length of 2 hours (subfigure c, 2 towers). The up-ramps and down-ramps are not distinguished because only minor differences occur between both.
Fig. 10. Cumulative frequency distribution of 6-hourly ramp events depending on the relative ramp rate for Billwerder at 110 m height and Karlsruhe at 140 m height (a) as well as for Cabauw at 140 m height and Falkenberg at 98 m height (b). (c) is the same as (b) but for 2-hourly ramps.

In addition to some deviations for the occurrence of moderate ramps, the COSMO models quite reasonably resolve the distribution in ramps considering step changes within six hours and within two hours. The absolute number of ramps is most convincing for CCLM-oF-SN and COSMO-REA6, whereas an overestimation is apparent for CCLM-oF (). The results for 3D-Var and 4D-Var are worse. Regarding the 6-hourly weak ramps, the relative likelihood of occurrence is often overestimated by UE-SMHI, but the absolute number of step changes with is systematically underestimated (). Consequently, the magnitude of all simulated ramps with at least 20% change is too weak (). It is unclear why the 3D-Var analysis increments preclude suitable ramp statistics, but as indicated by our analysis (), a seamless development of the PBL within the model is somehow suppressed and disturbed.
Regarding the 2-hourly weak ramps, UE-UKMO overestimates the likelihood of occurrence at all locations. In contrast, the simulated moderate ramps with are only half of the observed ramps (not shown), which is unique to the UKMO reanalysis. A further analysis reveals that the number of ramps is sufficiently near the analysis window but that too few ramp events appear in the forecast simulation in between (not shown). Moreover, the magnitude of the ramps with
is heavily underestimated in the forecast simulation – the wind speed variability appears to be dampened. This might be associated with the issues related to the diurnal cycle and the spin-up (Section 5.1).
The performance regarding the timing of weak ramp events is shown in (upper panel). The focus is on the 6-hourly ramps to prevent transition problems in the UERRA reanalyses. The success ratio and the probability of detection are considerably higher than in , (Yang et al., Citation2013), but the time frame differs, and the predictive skill is expected to be much higher in the assimilation products than in the forecast products. The best skill in ramp detection with a small amount of false alarms (SUC) is offered by the 4D-Var experiment, which is followed by that of the 3D-Var experiment with an overwhelming performance for the magnitudes of the hits (, dPhit). Regarding the lower levels, COSMO-REA6 also offers a comparable accuracy. This is consistent with the RMSErbc analysis, where the error was comparably small near the surface for COSMO-REA6. Furthermore, the accuracy in POD decreases for UE-UKMO and UE-SMHI and is approximately 10 to 15% smaller than that of SUC at levels above 100 m height. This is underpinned also by the statistics for the moderate ramps (not shown). The reason is the aforementioned underestimation of ramp events. Thus, many events are missed. In contrast, the ratio between false alarms and misses is more balanced for the COSMO models, and the difference between SUC and POD decreases.
Fig. 11. Success ratio (SUC) and probability of detection (POD) of ramps for different measurement locations and reanalysis products. The scores are shown for small ramps defined as the percentage change between 15 and 25% and regarding the analysis times 00, 06, 12 and 18 UTC (window length equals 6 hours). The color code is the same as in and .

The skill scores POD and SUC highlight an improved performance of sophisticated reanalysis compared to pure hindcasts. Comparing the reference UE-UKMO with a success ratio of 0.52 (mean over all locations, weak and moderate ramps), the accuracy is decreased by a factor of approximately 1.03 for UE-SMHI, 1.09 for COSMO-REA6, 1.35 for CCLM-oF-SN and 1.73 for CCLM-oF. The probability of detection is 0.48 for UE-UKMO and decreases by a factor of 1.02 for COSMO-REA6, 1.20 for UE-SMHI and CCLM-oF-SN and 1.45 for CCLM-oF. Considering also the 2-hourly ramps, COSMO-REA6 (SUC: 0.27, POD: 0.20) still outperforms the simulation without any assimilation (0.17, 0.12), as well as the simulation with large-scale nudging (0.22, 0.15).
6. Conclusions and outlook
To recall the objective of our work, we aimed to analyze and evaluate in detail the quality of regional reanalyses and hindcasts with respect to the lower planetary boundary layer and related sub-daily variability. The intention is to further use the atmospheric data for energy system models, with the focus on wind energy. In the evaluation framework data from most recent regional reanalysis projects were considered, i.e. UERRA-UKMO, UERRA-SMHI and COSMO-REA6. The evaluation was complemented by data from regional hindcasts using the same model system as COSMO-REA6, but with lateral boundary forcing (CCLM-oF) and with additionally spectral nudging (CCLM-oF-SN). The hindcasts were produced to better separate between different influencing factors affecting the model’s quality.
The quality of the model results was determined to be strongly dependent on both the complete model system, including assimilation method, resolution and physical parameterization, as well as on the performance measure. Considering the measure RMSE dominated by the temporal correlation on sub-daily time scales, smaller errors were found for the reanalyses than for the hindcasts, in particular the one without spectral nudging (CCLM-oF). Considering the distribution and the mean bias of wind speed at different levels, the performance of the hindcasts (in particular CCLM-oF-SN) was comparable to that of the UERRA reanalyses. This is the result of two overlapping effects: The reanalyses assimilate a lot of observational data, but the hindcasts offer a two times higher resolution and thus a better representation of the local topographic features. Here, COSMO-REA6 takes the advantage of elaborated assimilation as well as resolution and offers the smallest errors. Considering the distribution of ramp rates, the hindcasts offered the most convincing results.
Due to the experiments done with the COSMO setup (COSMO-REA6, CCLM-oF, CCLM-oF-SN), we were able to better analyze the impact of assimilation on model quality independent from other influencing factors. Indeed, the largest errors in the distribution of wind speed, mean bias and RMSErbc appear with simple downscaling. CCLM-oF is clearly outperformed by spectral nudging (CCLM-oF-SN) in nearly all aspects. Moreover, COSMO-REA6 is noticeably better than CCLM-oF-SN regarding the mean bias of wind and the temperature (not shown here, but we refer the comparison of 3D-Var and spectral nudging done by Zhang et al., Citation2017) thanks to continuous nudging of observations within the PBL. However, one key issue we found with the COSMO experiments is that the quality of the model results is limited, due in particular to the parameterizations of the physical processes. Deficits regarding temperature and wind profiles for stably stratified layers as well as shortcomings in diurnal cycles of different stabilities are not reduced by the sophisticated assimilation used in COSMO-REA6. The quality of the reanalysis is coupled with that of the PBL scheme. The results for stably stratified layers show that UE-SMHI with its comparably high vertical resolution may be the best choice for physically reasonable and consistent feed-in calculations. The results for unstable conditions indicated that the UKMO model overestimates superadiabatic lapse rates associated with excessively weak turbulent mixing.
The main advantage of the reanalysis setups was detected by a joint analysis of ramps and contingency tables, which turns out to be a extremely helpful tool for long-term simulations. The simulated ramps of the reanalysis offered a better timing than the simulated ramps of the hindcasts. A substantially better performance is achieved by 3D-Var and 4D-Var assimilation than by CCLM-oF and even CCLM-oF-SN. Despite some imbalances between missing ramp events and false alarms, UE-UKMO offers the best performance and should be preferred if high quality feed-in simulations are required with strongly varying atmospheric conditions.
The diurnal cycle of frequency distributions of different stability classes were for the most part well represented in the reanalyses and hindcasts. All of them were analyzed to overestimate unstable conditions at noon in winter and summer. The diurnal cycle of PBL wind is best depicted and most realistic for the hindcasts, where the seamless integration allows the model to freely develop its own physics. Although the near-surface development of wind speed is still somehow convincing in the UERRA reanalysis, weaknesses were identified for the levels above because of transition problems from analysis to forecast. As the model analysis is pushed to observations, a spin-up is detected for UE-SMHI in the subsequent forecast at a one hour lead time with a negative impact on the PBL development and also the high-frequency ramp distribution. These spin-up problems were reduced with UE-UKMO (4D-Var technique). COSMO-REA6 was shown to almost overcome the transition problem by continuous nudging, but the influence on directional shear and turbulence should be better investigated. However, a better analysis of the daily cycle of UERRA reanalysis might be achieved, if a forecast with longer lead times is used (12 to 36 hours) or if the hourly data of the 4D-Var system along the complete 6-hour assimilation window is used.
As the local wind characteristics and the model errors (RMSErbc > 20%) are highly dependent on the representation of topographic features, it is expected that a suitable intelligent error correction of reanalysis or hindcast data (e.g. Staffell and Pfenninger, Citation2016; González-Aparicio et al., Citation2017), dynamical adaptation (Žagar and Rakovec, Citation1999; Žagar et al., Citation2006) or large-eddy simulations could improve the model results. Without such post-processing, we have shown in this paper that the specific application should be clear prior usage of hindcast and reanalysis data: If the interest is in a small mean wind speed bias at Falkenberg, one would prefer data from COSMO-REA6, but if the interest is in the feed-in of wind power during nocturnal, stable weather conditions, one would prefer data from UERRA-SMHI. The strength of spectral nudging is the consistent and seamless development of friction-induced ageostrophy in the PBL (preventing unwanted modes, Hong and Chang, Citation2012), independence from temporally varying quality of onsite observations and only a moderate effort.
Regarding model examination, a more detailed data analysis for quantification of further potential impacts, as soil processes and atmosphere-land interactions, is desired for future studies. It is definitely worth extending the PBL analysis to wind direction, specific humidity and energy fluxes. Moreover, one might think of a further filter for atmospheric stability, e.g. a distinction by cloud cover or rain rate. A first examination suggests that model deficiencies could still be better isolated than they are now (inversion situations under overcast conditions). Moreover, a further investigation of radio soundings and remote-sensing data near flux towers could help to determine the impact of those errors originating from dynamics in the upper PBL and above.
All the conclusions drawn are valid for the flux towers investigated here. The statements may differ for regions characterized by fully different climate and atmospheric regimes. It would be worthwhile to include other flux towers and the marine measurement towers FINO1, FINO2 and FINO3, but up to now the profile data are not completely checked for quality and reliability. A much larger database is apparently operated by wind companies, but the access is restricted. However, most important for our investigations is that long-term climate simulations are analyzed by more detailed multivariate studies of atmospheric profiles, keeping in mind the model’s limitations due to horizontal and vertical meshes.
zela_a_1804294_sm9893.pdf
Download PDF (1.1 MB)Acknowledgments
We thank the site operators at the MOL-RAO belonging to the German Weather Service, who delivered data from tower site Falkenberg. We thank to the weather mast group of the Meteorological Institute at the University of Hamburg, who delivered data from tower site Hamburg. We thank the group associated with the Cabauw tower and the related CESAR data base. We thank the IMK-TRO for providing Karlsruhe tower data. Without the extremely helpful comments and insight of the providers of observational data and reanalysis data, this paper would not exist. Furthermore, we thank Semjon Schimanke for carefully checking parts of the manuscript. In particular, we thank the reviewers for their incredible effort to improve the quality of the paper. The research work was funded by the Federal Ministry for Economic Affairs and Energy (ID: 0324006A). The authors acknowledge support from the Deutsche Klimarechenzentrum and the CLM community.
Disclosure statement
No potential conflict of interest was reported by the authors.
Supplementary material
Supplemental data for this article can be accessed here.
References
- Baldauf, M., Seifert, A., Förstner, J., Majewski, D., Raschendorfer, M. and co-authors. 2011. Operational convective-scale numerical weather prediction with the COSMO model: description and sensitivities. Mon. Weather Rev. 139, 3887–3905. doi:https://doi.org/10.1175/MWR-D-10-05013.1
- Barthlott, C., Kalthoff, N. and Fiedler, F. 2003. Influence of high-frequency radiation on turbulence measurements on a 200 m tower. Meteorol. Z. 12, 67–71. doi:https://doi.org/10.1127/0941-2948/2003/0012-0067
- Bengtsson, L., Andrae, U., Aspelien, T., Batrak, Y., Calvo, J. and co-authors. 2017. The HARMONIE–AROME model configuration in the ALADIN–HIRLAM NWP system. Mon. Weather Rev. 145, 1919–1935. doi:https://doi.org/10.1175/MWR-D-16-0417.1
- Best, M. J., Pryor, M., Clark, D. B., Rooney, G. G., Essery, R. L. H. and co-authors. 2011. The joint UK land environment simulator (JULES), model description – Part 1: energy and water fluxes. Geosci. Model Dev. 4, 677–699. doi:https://doi.org/10.5194/gmd-4-677-2011
- Beyrich, F., Herzog, H.-J. and Neisser, J. 2002. The LITFASS project of DWD and the LITFASS-98 experiment: the project strategy and the experimental setup. Theor. Appl. Climatol. 73, 3–18. doi:https://doi.org/10.1007/s00704-002-0690-8
- Bianco, L., Djalalova, I. V., Wilczak, J. M., Cline, J., Calvert, S. and co-authors. 2016. A wind energy ramp tool and metric for measuring the skill of numerical weather prediction models. Weather Forecast. 31, 1137–1156. doi:https://doi.org/10.1175/WAF-D-15-0144.1
- Bollmeyer, C., Keller, J. D., Ohlwein, C., Wahl, S., Crewell, S. and co-authors. 2015. Towards a high-resolution regional reanalysis for the European CORDEX domain. Q. J. R. Meteorol. Soc. 141, 1–15. 2486. doi:https://doi.org/10.1002/qj.2486
- Borsche, M., Kaiser-Weiss, A. K. and Kaspar, F. 2016. Wind speed variability between 10 and 116m height from the regional reanalysis COSMO-REA6 compared to wind mast measurements over Northern Germany and the Netherlands. Adv. Sci. Res. 13, 151–161. doi:https://doi.org/10.5194/asr-13-151-2016
- Brümmer, B., Lange, I. and Konow, H. 2012. Atmospheric boundary layer measurements at the 280 m high Hamburg weather mast 1995-2011: mean annual and diurnal cycles. Meteorol. Z. 21, 319–335. doi:https://doi.org/10.1127/0941-2948/2012/0338
- Brümmer, B. and Schultze, M. 2015. Analysis of a 7-year low-level temperature inversion data set measured at the 280 m high Hamburg weather mast. Meteorol. Z. 24, 481–494. doi:https://doi.org/10.1127/metz/2015/0669
- Bubnov, R., Hello, G., Bénard, P. and Geleyn, J.-F. 1995. Integration of the fully elastic equations cast in the hydrostatic pressure terrain-following coordinate in the framework of the ARPEGE/ALADIN NWP system. Mon. Weather Rev. 123, 515–535. doi:https://doi.org/10.1175/1520-0493(1995)123<0515:IOTFEE>2.0.CO;2
- Cannon, D., Brayshaw, D., Methven, J., Coker, P. and Lenaghan, D. 2015. Using reanalysis data to quantify extreme wind power generation statistics: a 33 year case study in Great Britain. Renew. Energy 75, 767–778. doi:https://doi.org/10.1016/j.renene.2014.10.024
- Clark, D. B., Mercado, L. M., Sitch, S., Jones, C. D., Gedney, N. and co-authors. 2011. The joint UK land environment simulator (JULES), model description – Part 2: carbon fluxes and vegetation dynamics. Geosci. Model Dev. 4, 701–722. doi:https://doi.org/10.5194/gmd-4-701-2011
- Cuxart, J., Bougeault, P. and Redelsperger, J.-L. 2000. A turbulence scheme allowing for mesoscale and large-eddy simulations. Q. J. R. Meteorol. Soc. 126, 1–30. doi:https://doi.org/10.1002/qj.49712656202
- Cuxart, J., Holtslag, A. A. M., Beare, R. J., Bazile, E., Beljaars, A. and co-authors. 2006. Single-column model intercomparison for a stably stratified atmospheric boundary layer. Boundary-Layer Meteorol. 118, 273–303. doi:https://doi.org/10.1007/s10546-005-3780-1
- Dahlgren, P., Landelius, T., Kållberg, P. and Gollvik, S. 2016. A high-resolution regional reanalysis for Europe. Part 1: three-dimensional reanalysis with the regional HIgh-Resolution Limited-Area Model (HIRLAM). Q. J. R. Meteorol. Soc. 142, 2119–2131. doi:https://doi.org/10.1002/qj.2807
- de Rooy, W. and de Vries, H. 2017. Harmonie Verification and Evaluation. Technical Report. Availabe from HIRLAM-A Programme c/o Onvlee, KNMI, P.P. Box. 201, 3730 AE. 79 pp.
- Dee, D. P., Uppala, S. M., Simmons, A. J., Berrisford, P., Poli, P. and co-authors. 2011. The ERA-Interim reanalysis: configuration and performance of the data assimilation system. Q. J. R. Meteorol. Soc. 137, 553–597. doi:https://doi.org/10.1002/qj.828
- Dickinson, R. E. 1984. Modeling evapotranspiration for three-dimensional global climate models. Climate Processes and Climate Sensitivity 58–72. doi:https://doi.org/10.1029/gm029p0058
- Drechsel, S., Mayr, G. J., Messner, J. W., Stauffer, R., Drechsel, S. and co-authors. 2012. Wind speeds at heights crucial for wind energy: measurements and verification of forecasts. J. Appl. Meteorol. Climatol. 51, 1602–1617. doi:https://doi.org/10.1175/JAMC-D-11-0247.1
- Dürr, B. 2004. The greenhouse effect in the Alps - by models and observations. Ph.D. thesis.
- Emeis, S., Münkel, C., Vogt, S., Müller, W. J. and Schäfer, K. 2004. Atmospheric boundary-layer structure from simultaneous SODAR, RASS, and ceilometer measurements. Atmos. Environ. 38, 273–286. 054. doi:https://doi.org/10.1016/j.atmosenv.2003.09.054
- Frank, C., Pospichal, B., Wahl, S., Keller, J. D., Hense, A. and co-authors. 2020. The added value of high resolution regional reanalyses for wind power applications. Renew. Energy 148, 1094–1109. doi:https://doi.org/10.1016/j.renene.2019.09.138
- Frank, C. W., Wahl, S., Keller, J. D., Pospichal, B., Hense, A. and co-authors. 2018. Bias correction of a novel European reanalysis data set for solar energy applications. Sol. Energy 164, 12–24. doi:https://doi.org/10.1016/j.solener.2018.02.012
- Gelaro, R., McCarty, W., Suárez, M. J., Todling, R., Molod, A. and co-authors. 2017. The modern-era retrospective analysis for research and applications, Version 2 (MERRA-2). J. Clim. 30, 5419–5454. doi:https://doi.org/10.1175/JCLI-D-16-0758.1
- Geyer, B. 2014. High-resolution atmospheric reconstruction for Europe 1948–2012: coastDat2. Earth Syst. Sci. Data 6, 147–164. doi:https://doi.org/10.5194/essd-6-147-2014
- Geyer, B. 2017. coastDat-3_COSMO-CLM_ERAi. World Data Center for Climate (WDCC) at DKRZ, last access at Aug 2018. Online at: http://cera-www.dkrz.de/WDCC/ui/Compact. jsp?acronym=coastDat-3{\_}COSMO-CLM{\_}ERAi.
- González-Aparicio, I., Monforti, F., Volker, P., Zucker, A., Careri, F. and co-authors. 2017. Simulating European wind power generation applying statistical downscaling to reanalysis data. Appl. Energy 199, 155–168. doi:https://doi.org/10.1016/j.apenergy.2017.04.066
- Gupta, H. V., Kling, H., Yilmaz, K. K. and Martinez, G. F. 2009. Decomposition of the mean squared error and NSE performance criteria: implications for improving hydrological modelling. J. Hydrol. 377, 80–91. doi:https://doi.org/10.1016/j.jhydrol.2009.08.003
- Heide, D., Greiner, M., von Bremen, L. and Hoffmann, C. 2011. Reduced storage and balancing needs in a fully renewable European power system with excess wind and solar power generation. Renew. Energy 36, 2515–2523. doi:https://doi.org/10.1016/j.renene.2011.02.009
- Helbig, N., Löwe, H., Lehning, M., Helbig, N., Löwe, H. and co-authors. 2009. Radiosity approach for the shortwave surface radiation balance in complex terrain. J. Atmos. Sci. 66, 2900–2912. doi:https://doi.org/10.1175/2009JAS2940.1
- Hong, S.-Y. and Chang, E.-C. 2012. Spectral nudging sensitivity experiments in a regional climate model. Asia-Pacific J. Atmos. Sci. 48, 345–355. doi:https://doi.org/10.1007/s13143-012-0033-3
- Hoyer, S. and Hamman, J. J. 2017. xarray: N-D labeled arrays and datasets in Python. J. Open Res. Softw. 5. doi:https://doi.org/10.5334/jors.148
- Jacob, M. 2013. Beeinflussung Von Windmessungen an Einem Rohrmast Durch Die Maststruktur (Bachelor Thesis). Technical Report, Department MIN, University of Hamburg, 35 pp.
- James, P. M. 2007. An objective classification method for Hess and Brezowsky Grosswetterlagen over. Theor. Appl. Climatol. 88, 17–42. doi:https://doi.org/10.1007/s00704-006-0239-3
- Kaiser-Weiss, A. K., Kaspar, F., Heene, V., Borsche, M., Tan, D. G. H. and co-authors. 2015. Comparison of regional and global reanalysis near-surface winds with station observations over Germany. Adv. Sci. Res. 12, 187–198. doi:https://doi.org/10.5194/asr-12-187-2015
- Kaldemeyer, C., Boysen, C. and Tuschy, I. 2016. Compressed air energy storage in the German energy system – status quo & perspectives. Energy Procedia 99, 298–313. doi:https://doi.org/10.1016/j.egypro.2016.10.120
- Kalthoff, N. and Vogel, B. 1992. Counter-current and channelling effect under stable stratification in the area of Karlsruhe. Theor. Appl. Climatol. 45, 113–126. doi:https://doi.org/10.1007/BF00866400
- Konow, H. M. 2015. Tall wind profiles in heterogeneous terrain. Ph.D. thesis.
- Landelius, T., Dahlgren, P., Gollvik, S., Jansson, A. and Olsson, E. 2016. A high-resolution regional reanalysis for Europe. Part 2: 2D analysis of surface temperature, precipitation and wind. Q. J. R. Meteorol. Soc. 142, 2132–2142. doi:https://doi.org/10.1002/qj.2813
- Li, D., von Storch, H. and Geyer, B. 2016. Testing reanalyses in constraining dynamical downscaling. J. Meteorol. Soc. Jpn. 94A, 47–68. doi:https://doi.org/10.2151/jmsj.2015-044
- Lorenc, A. C. and Rawlins, F. 2005. Why does 4D-Var beat 3D-Var? Q. J. R. Meteorol. Soc. 131, 3247–3257. doi:https://doi.org/10.1256/qj.05.85
- Mohan, M. and Siddiqui, T. 1998. Analysis of various schemes for the estimation of atmospheric stability classification. Atmos. Environ. 32, 3775–3781. doi:https://doi.org/10.1016/S1352-2310(98)00109-5
- Monin, A. and Obukhov, 1954. Basic laws of turbulent mixing in the surface layer of the atmosphere. Contrib. Geophys. Inst. Acad. Sci. USSR 151, 163–187.
- Neisser, J., Adam, W., Beyrich, F., Leiterer, U. and Steinhagen, H. 2002. Atmospheric boundary layer monitoring at the Meteorological Observatory Lindenberg as a part of the “Lindenberg Column”: facilities and selected results. Meteorol. Z. 11, 241–253. doi:https://doi.org/10.1127/0941-2948/2002/0011-0241
- Niermann, D., Borsche, M., Kaiser-Weiss, A. and Kaspar, F. 2019. Evaluating renewable energy relevant parameters of COSMO-REA6 by comparing against station observations, satellites and other reanalyses. Meteorol. Z. 28, 347–360. doi:https://doi.org/10.1127/metz/2019/0945
- Noilhan, J., Planton, S., Noilhan, J. and Planton, S. 1989. A simple parameterization of land surface processes for meteorological models. Mon. Weather Rev. 117, 536–549. doi:https://doi.org/10.1175/1520-0493(1989)117<0536:ASPOLS>2.0.CO;2
- Pfenninger, S. and Staffell, I. 2016. Long-term patterns of European PV output using 30 years of validated hourly reanalysis and satellite data. Energy 114, 1251–1265. 2016.08.060. doi:https://doi.org/10.1016/j.energy.2016.08.060
- Rabin, J., Delon, J. and Gousseau, Y. 2008. Circular earth mover’s distance for the comparison of local features, In: 2008 19th International Conference on Pattern Recognition, IEEE, Tampa, Florida, 1–4,
- Randles, C. A., Da Silva, A. M., Buchard, V., Colarco, P. R., Darmenov, A. and co-authors. 2017. The MERRA-2 aerosol reanalysis, 1980 - onward, Part I: system description and data assimilation evaluation. J. Clim. 30, 6823–6850. 1175/JCLI-D-16-0609.1. doi:https://doi.org/10.1175/JCLI-D-16-0609.1
- Rawlins, F., Ballard, S. P., Bovis, K. J., Clayton, A. M., Li, D. and co-authors. 2007. The Met Office global four-dimensional variational data assimilation scheme. Q. J. R. Meteorol. Soc. 133, 347–362. doi:https://doi.org/10.1002/qj.32
- Rienecker, M. M., Suarez, M. J., Gelaro, R., Todling, R., Bacmeister, J. and co-authors. 2011. MERRA: NASA’s modern-era retrospective analysis for research and applications. J. Clim. 24, 3624–3648. doi:https://doi.org/10.1175/JCLI-D-11-00015.1
- Schraff, C. and Hess, R. 2003. A Description of the Non-Hydrostatic Regional Model LM - Part III: Data Assimilation. Technical Report, Deutscher Wetterdienst, Offenbach, Germany.
- Schubert-Frisius, M., Feser, F., von Storch, H. and Rast, S. 2017. Optimal spectral nudging for global dynamic downscaling. Mon. Weather Rev. 145, 909–927. doi:https://doi.org/10.1175/MWR-D-16-0036.1
- Sevlian, R. and Rajagopal, R. 2013. Detection and statistics of wind power ramps. IEEE Trans. Power Syst. 28, 3610–3620. doi:https://doi.org/10.1109/TPWRS.2013.2266378
- Sharp, E., Dodds, P., Barrett, M. and Spataru, C. 2015. Evaluating the accuracy of CFSR reanalysis hourly wind speed forecasts for the UK, using in situ measurements and geographical information. Renew. Energy 77, 527–538. doi:https://doi.org/10.1016/j.renene.2014.12.025
- Staffell, I. and Pfenninger, S. 2016. Using bias-corrected reanalysis to simulate current and future wind power output. Energy 114, 1224–1239. doi:https://doi.org/10.1016/j.energy.2016.08.068
- Stull, R. B. 1988. An Introduction to Boundary Layer Meteorology. Springer, Dordrecht, 670 pp.
- Tammelin, B., Vihma, T., Atlaskin, E., Badger, J., Fortelius, C. and co-authors. 2011. Production of the Finnish Wind Atlas. Wind Energ. 16, 19–35. doi:https://doi.org/10.1002/we.517
- Unden, P. 2018. Uncertainties in Ensembles of Regional Reanalyses (UERRA) - Final Report. Technical Report, SVERIGES METEOROLOGISKA OCH HYDROLOGISKA INSTITUT.
- Urraca, R., Huld, T., Gracia-Amillo, A., Martinez-de Pison, F. J., Kaspar, F. and co-authors. 2018. Evaluation of global horizontal irradiance estimates from ERA5 and COSMO-REA6 reanalyses using ground and satellite-based data. Sol. Energy 164, 339–354. doi:https://doi.org/10.1016/j.solener.2018.02.059
- Van de Wiel, B. J. H., Moene, A. F., Steeneveld, G. J., Baas, P., Bosveld, F. C. and co-authors. 2010. A conceptual view on inertial oscillations and nocturnal low-level jets. J. Atmos. Sci. 67, 2679–2689. doi:https://doi.org/10.1175/2010JAS3289.1
- Van Ulden, A. P. and Wieringa, J. 1996. Atmospheric boundary layer research at Cabauw. Boundary-Layer Meteorol. 78, 39–69. doi:https://doi.org/10.1007/BF00122486
- Verkaik, J. W. and Holtslag, A. A. M. 2007. Wind profiles, momentum fluxes and roughness lengths at Cabauw revisited. Boundary-Layer Meteorol. 122, 701–719. doi:https://doi.org/10.1007/s10546-006-9121-1
- von Storch, H., Langenberg, H., Feser, F., von Storch, H., Langenberg, H. and co-authors. 2000. A spectral nudging technique for dynamical downscaling purposes. Mon. Weather Rev. 128, 3664–3673. doi:https://doi.org/10.1175/1520-0493(2000)128<3664:ASNTFD>2.0.CO;2
- Walters, D., Boutle, I., Brooks, M., Melvin, T., Stratton, R. and co-authors. 2017. The met office unified model global atmosphere 6.0/6.1 and jules global land 6.0/6.1 configurations. Geosci. Model Dev. 10, 1487–1520. doi:https://doi.org/10.5194/gmd-10-1487-2017
- Wenzel, A., Kalthoff, N. and Horlacher, V. 1997. On the profiles of wind velocity in the roughness sublayer above a coniferous forest. Boundary Layer Meteorol. 84, 219–230. 1000444911103. doi:https://doi.org/10.1023/A:1000444911103
- Wohland, J., Reyers, M., Märker, C. and Witthaut, D. 2018. Natural wind variability triggered drop in German redispatch volume and costs from 2015 to 2016. PLoS One. 13, e0190707. doi:https://doi.org/10.1371/journal.pone.0190707
- Wood, N., Staniforth, A., White, A., Allen, T., Diamantakis, M. and co-authors. 2014. An inherently mass-conserving semi-implicit semi-Lagrangian discretization of the deep-atmosphere global non-hydrostatic equations. Q. J. R. Meteorol. Soc. 140, 1505–1520. doi:https://doi.org/10.1002/qj.2235
- Yang, Q., Berg, L. K., Pekour, M., Fast, J. D., Newsom, R. K. and co-authors. 2013. Evaluation of WRF-predicted near-hub-height winds and ramp events over a Pacific Northwest site with complex terrain. J. Appl. Meteorol. Climatol. 52, 1753–1763. doi:https://doi.org/10.1175/JAMC-D-12-0267.1
- Yang, Y., Uddstrom, M. and Duncan, M. 2011. Effects of short spin-up periods on soil moisture simulation and the causes over New Zealand. J. Geophys. Res. 116.
- Žagar, M. and Rakovec, J. 1999. Small-scale surface wind prediction using dynamic adaptation. Tellus A 51, 489–504. doi:https://doi.org/10.3402/tellusa.v51i4.14051
- Žagar, N., Žagar, M., Cedilnik, J., Gregorič, G. and Rakovec, J. 2006. Validation of mesoscale low-level winds obtained by dynamical downscaling of ERA40 over complex terrain. Tellus A Dyn. Meteorol. Oceanogr. 58, 445–455. doi:https://doi.org/10.1111/j.1600-0870.2006.00186.x
- Zerrahn, A. and Schill, W.-P. 2017. Long-run power storage requirements for high shares of renewables: review and a new model. Renew. Sustain. Energy Rev. 79, 1518–1534. doi:https://doi.org/10.1016/j.rser.2016.11.098
- Zhang, Q., Pan, Y., Wang, S., Xu, J. and Tang, J. 2017. High-resolution regional reanalysis in China: evaluation of 1 year period experiments. J. Geophys. Res. Atmos. 122, 10801–10819. doi:https://doi.org/10.1002/2017JD027476
Appendix A.
Description of tower measurements
Appendix A.1.
The Hamburg weather mast
The measurement tower in Hamburg is a steel tube mast with a height of 300 m and a diameter of 2 m. The measurements are taken with sensors mounted on booms (one at each level) oriented to the south. The distance between the measurement device and the midpoint of the mast is nearly 6 m. For the near-surface measurements, a 12 m wind mast located WNW of the tall tower at a distance of 170 m is also operated. The instrumentation is nearly the same for the period 2000–2016. Turbulence-based measurements started in 2000 using USAT devices from METEK (USA-1, frequency of 20 Hz), which were replaced by the next generation of METEK’s USA devices in 2013 and complemented by an extra device at a height of 280 m added in mid-2010. Additionally, temperature and relative humidity were measured within a ventilated and radiation-protected tube (using a platinum resistance sensor and a humicap, respectively). More details can be found in Brümmer et al. (Citation2012) and on the related webpage http://wettermast.uni-hamburg.de.
The orography near the tower is flat (mean sea level), and 2 km to the northeast, the Geest slope is present with a difference of up to 50 m in height. The land surfaces are characterized by sparse-dense villages, mainly to the south; widespread industrial sites with low buildings mainly to the west and northwest; and wasteland and rinsing surfaces mainly to the northeast and east. The area far to the southeast is dominated mainly by agricultural areas. According to the environmental conditions, the data are subdivided into wind sector groups (east, south, west and north). The related roughness at the Hamburg Weather mast was estimated by Konow (Citation2015) applying the MOST theory under nearly neutral conditions (see ).
The wind blowing from the northern sector is influenced by the mast shadowing effect. Jacob (Citation2013) quantified the impact of the mast with parallel SODAR observations and identified the sector from nearly 320∘ to 50∘ as being influenced. He proposed a correction for the mean wind speed to recover a gapless climatology of the wind speed observations, which is also applied in our analyses.
We were provided data with a frequency of every 10 min (averages). The original raw data were converted into ‘netcdf’ format to make the evaluation as easy as possible. During that process, the time series is checked for missing data, outliers and physical robustness (yearly cycle and plausible range for variables). Regarding the wind speed and direction, we store the uncorrected data stream, a data stream masking the northern sector and a data stream containing mast-corrected wind speeds.
Appendix A.2.
Falkenberg
The 100 m tower at Falkenberg is a lattice construction with a rectangular cross section (1.2 m side length). Wind sensors are mounted on three booms (length: 1.5 m) at each measuring level oriented approximately to the south, west and north. The wind observations are influenced by the lattice construction. To overcome this issue, the final wind data are derived by taking the measurement at that boom, which is at most offering an unperturbed flow.
The standard instrumentation consists of cup anemometers and wind vanes. The temperature and humidity are measured in aspirated radiation shields by platinum resistance sensors and humicap polymer sensors, respectively. Additionally, high-frequency wind measurement devices (USATs) were installed for experimental setups and have now been continuously operated at heights of 40 and 90 m since 2008. Near-surface measurements are conducted at a 10 m wind mast located approximately 70 m south-southeast of the tall tower. The lattice mast has a triangular shape with 1.5 m booms oriented toward the southwest. More details can be found in Neisser et al. (Citation2002) and Beyrich et al. (Citation2002).
The orography near the tower has only a 5 m height difference over 1 km2. The onsite vegetation is short grass, which is regularly mowed to keep its height below 20 cm. The environment is characterized by grassland and agricultural fields. A small village is located 600 m to the southeast, and a small forest is located 1 km to the west. The onsite roughness at Falkenberg shows, on average, only minor directional variability. The onsite roughness length, derived during neutral conditions and near the grass-covered ground, varies between 0.01 and 0.03 m only. However, observations above 10 m are also influenced by the cropland, which mostly induces a higher roughness length.
Data with a frequency of every 10 min (averages) were provided and used for evaluation.
Appendix A.3.
Cabauw
This 213 m tower is a solid cylinder with a diameter of 2 m. Because of flow distortion, wind sensors are mounted on two (north and southwest) of the overall three booms, which extend 9.4 m beyond the surface of the cylinder at each measurement level. The anemometer that is best exposed is used for the data record. The standard instrumentation for wind measurements consists of propeller vanes. The temperature and humidity are observed using a thermocouple/platinum resistance sensor and a humicap, respectively. For the near-surface winds below 20 m, additional flow distortion is present, and two auxiliary masts operated to the southeast and northwest of the main tower. More details can be found in Verkaik and Holtslag (Citation2007).
The tower is located on flat terrain with the North Sea at a distance of 50 km. The onsite surface consists mainly of short grass with small villages, rows of trees along streets and bushes. The closer environment shows, only for the southwest sector, more or less undisturbed flow conditions with nearly no obstacles. The northern sector is characterized by a line of trees and houses forming a windbreak-like obstacle, and pasture is present farther away. A village is situated to the east, and roads, orchards, riverbeds and dikes are located to the southeast. Considering the complex topography, Verkaik and Holtslag (Citation2007) found a dependency of the roughness length on the method used, wind direction and effective fetch (height above ground) ().
Data with a frequency of every 10 min (30 min averages) were downloaded from the CESAR data archive.
Appendix A.4.
Karlsruhe
This 200 m tower is operated by the Institute for Meteorology and Climate Research (IMK-TRO) and is a lattice construction. The measurement devices are cup anemometers, wind vanes and resistance thermometers. To avoid the shadowing effect, wind measurements are taken at two sides of the tower (west and east), and the sensor that is exposed most is designed to provide an unperturbed signal. For further details, the reader is referred to Barthlott et al. (Citation2003) and Wenzel et al. (Citation1997).
The tower is located in the eastern part of the wide Rhine valley (30 km) and 110 m above MSL. The hills of the Kraichgau appear 10 km to the east with a mean hill height of 250 m (60 m lower than the top of the tower). The onsite surface is mainly coniferous forest (trees with a 30 m height located 10 m apart from each other) with an extension of 10 km to the south and 8 km along the west-east axis. Consequently, the near-surface wind defined at a 10 m height has to be extracted with care. Kalthoff and Vogel (Citation1992) utilized the wind measurements at 40 m above ground as near-surface winds. The commonly used zero-plane displacement within the Monin-Obukhov similarity theory was determined by Wenzel et al. (Citation1997) to be 20 m. They discussed the challenging task of experimentally determining the roughness length at the tower site.
We were provided data with a frequency of every 10 min (averages). Just like the Hamburg flux tower, the data were converted into ‘netcdf’ format, and the time series were rechecked for inconsistencies. According to Wenzel et al. (Citation1997), the time series was aggregated from 10 min values to 30 min values.
Appendix B.
Dependency of errors to GWLs
The data over 2 years were separated for the so-called Grosswetterlagen (GWLs) of Hess and Brezowsky. Twenty-nine types of weather patterns were considered, and a related automatic detection was introduced as described by James (Citation2007). The German Weather Service has provided the resulting classification for each day starting in 1881. Thus, the atmospheric data of the flux towers (here, the wind speed and the RMSE values of the model experiments) were calculated with respect to each day and then associated with the 29 GWLs is done. The corresponding statistics were derived for the two flux towers farthest from each other and are shown in Fig. S1.
Appendix C.
Sensitivity to vertical interpolation
The calculation for vertical gradients and thus stability is dependent on the vertical interpolation used to reconstruct the model data. As the vertical profiles of temperature and wind speed might be very nonlinear near the surface, an impact on the statistics presented in this paper is expected, i.e. UERRA products support us with values at the 2 m and 15 m levels only, with none at 10 m. We performed an additional analysis of the statistics on daily cycles of stratification using not only linear reconstruction but also two other methods for vertical interpolation: quadratic polynomials and cubic splines. The results are shown for the flux tower Billwerder in Fig. S2. The COSMO-related models do not show a visible impact of the interpolation method (not shown). Regarding UE-UKMO, the stratification statistics are slightly modified at noon in winter with respect to neutral and unstable stratification. A redistribution takes place because higher order polynomials alter the interpolation to emphasize the value of the level at 15 m for the reconstruction of the 10 m value. If the absolute value of the gradient is decreased and furthermore located near the transition zone between the ‘vus’ and ‘ns’ cases, neutral conditions are favored. In addition, a weak sensitivity for interpolation appears at evening in summer. The reason for the redistribution between stable and very stable cases is very likely the same as before: a decrease in the absolute value of the gradient favors stable stratifications.
Just like the stratification, the temperature gradient is also only slightly sensitive to interpolation (Fig. S3). Therefore, we conclude that the interpolation does not affect the main conclusions drawn in this paper.