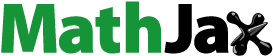
Abstract
The study of winter seasonal predictability with the climate model INM-CM5-0 is presented. Initial conditions were produced using ERA-Interim reanalysis data for atmosphere, SODA3.4.2 reanalysis data for ocean and the bias-correction algorithm. The seasonal 5-month re-forecasts consisting of 10 ensemble members with small initial condition perturbations for each year over the 35-yr period are conducted. A comparison of the multiyear mean winter averaged anomaly correlation for basic variables in several regions with similar results of SLAV model was conducted. An increase in the anomaly correlation for the years with El Niño and La Niña events was shown. The predictability of NAO and PNA indices was studied. INM-CM5-0 provides very high skill in predicting the winter NAO (correlation coefficient of 0.71 with ERA-Interim reanalysis and 0.68 with instrumental CRU data for 1991–2010). It was shown, that the stratospheric variability provides a significant contribution, although potentially is not the only cause of model high skill in NAO index predictability. Correlation coefficients for PNA index in December-February is 0.60. In the years of the most pronounced El Niño the values of PNA index have significantly positive values, and for La Niña years they are noticeably less than zero.
1. Introduction
Coupled atmospheric and oceanic general circulation models developed in the Institute of Numerical Mathematics Russian Academy of Sciences (INM RAS) were utilized for climate simulations only. However, modern model requirements suggest seamless approach (Hoskins, Citation2013), which means high predictive skills at various timescales from day to season and decade. The unified framework helps to make forecasts at various timescales, but also to unravel the main sources of errors responsible for the failure of models to adequately capture major modes (Brown et al., Citation2012). Nevertheless, moving to a seamless approach is not trivial and introduces a lot of challenges. Сoupled models for seasonal forecasts were built at the European Center for Medium-Range Weather Forecasts ECMWF (System 4 – Molteni et al. (Citation2011), SEAS5 – Stockdale et al. (Citation2018)) at the National Environmental Forecasting Centers NCEP (Global Forecast System – GFS), and other weather prediction centers.
In order to study INM-CM5-0 capability to simulate anomalies at seasonal timescale, a series of 5-months model experiments, started from bias-corrected initial states responding to November 1 of 1980–2014, were performed. The results of hindcasts were analyzed by means of assessing the predictability of the basic climatic patterns of the Northern Hemisphere.
First, anomaly correlations for basic fields are studied. Then, predictability of global teleconnections – North Atlantic Oscillation (NAO) and Pacific North American (PNA) pattern that exert an influence on winter temperatures, air pressure and precipitation over the Eurasia and United States (Leathers et al., Citation1991; Polonsky et al., Citation2004) is considered. Finally, the predictability of winter high latitude stratospheric state and its influence on the model skill is evaluated.
2. Model and design of seasonal hindcasts
In most world weather and climate centers, seasonal weather forecasts are made using the coupled models of atmosphere and ocean general circulation. The similar family of coupled models named INMCM was developed in the INM RAS. INMCM has several configurations. This study is based on the experiments carried out with the model version INM-CM5-0 with atmospheric resolution in longitude and latitude, 73 vertical levels with a model top at 0.2 hPa and oceanic resolution
with 40 vertical levels. Model is participated in Coupled Model Intercomparison Project, Phase 6 (CMIP6). Model description and results of present day climate simulation can be found in Volodin et al. (Citation2017). Simulation of climate changes in historical period 1850–2014 is presented in Volodin and Gritsun (Citation2018).
In reforecasts, Northern Hemisphere winter conditions for November–March are modelled. November 1 was initial data for all reforecasts. Winter seasons from 1980–1981 to 2014–2015 are considered. Initial conditions for the hindcasts are generated applying the bias correction algorithm in order to avoid model climate drift and to improve the accuracy of reforecasts.
In the INMCM retrospective seasonal forecasts the ocean is initialized from Simple Ocean Data Assimilation (SODA) 3.4.2 using monthly mean values of practical salinity, potential temperature, sea level, ice mass and ice thickness, ice concentration in five gradations of thickness for Octobers and Novembers of 1980–2014 years.
Ice concentration data are summarized for all gradations, since there is the only sea ice gradation in the INM RAS model. Model and reanalysis climatologies are calculated using data from model historical experiment previously carried out for the period of 1980–2014 according to the CMIP6 protocol. Data for November 1 are calculated as the arithmetic average of monthly means for October and November. After that, the difference between model and reanalysis climatologies was added to reanalysis data for November 1 of considered year in 1980–2014, converted to model units and interpolated to the model grid. The final values of the variables here and after by the example of 1980, taken as the initial state for the model 1980–1981 experiments are obtained using the technique of model bias eliminating:
(1)
(1)
Here is the model climatology of November 1 for the period 1980–2014,
are the values of the reanalysis variables for November 1 of 1980,
is the reanalysis climatology of November 1 for the period 1980–2014.
Negative values of sea ice compactness were replaced by zero. Ice thickness in the case of nonzero ice compactness was restricted by interval of 0.01 cm–1800 cm. The second point was unrealistically large values of the salinity anomaly calculated from reanalysis data, which reached the value of 5 PSU in the Black Sea and some other inland water basins. It was decided to set the limiting absolute value of the salinity anomaly to zero in the Black Sea and to 1 PSU in other similar cases.
Atmosphere initial conditions were obtained from ERA-Interim reanalysis. We used data of soil temperature and humidity, surface pressure, skin temperature, air temperature and specific humidity, snow depth, U- and V-components of the wind speed taken from the ERA – Interim reanalysis for 00:00 November 1 1980–2014 years. To compile a set of initial data for experiments, similar procedure of subtraction the difference of reanalysis and model climatologies, with the exception for soil moisture and soil temperature were converted to model horizons, and snow depth, soil temperature and humidity were calculated using a modified EquationEq. (2)(2)
(2) to bring the values of physical quantities to the same range:
(2)
(2)
where
is the standard deviation of the model variables for the 1st of November 1980–2014,
is the standard deviation of the reanalysis variables for the 1st of November 1980–2014, the remaining notation coincides with the notation in EquationEq. (1)
(1)
(1) .
The INMCM seasonal 5-month re-forecast consists of 10 ensemble members initialized on the November 1 for each year over the 35-yr period 1980–2014. Small initial condition perturbations are applied to air temperature and wind speed to represent uncertainty in the initial state and increase ensemble spread. Ensemble member 1 is initialized from unperturbed initial conditions.
In the next section, we will analyze the results of model experiments.
3. Skill assessment of seasonal hindcasts
3.1. Anomaly correlation
To evaluate the model skill in basic variables, we compared results obtained for INM-CM5-0 with similar results for SLAV semi-Lagrangian model of general atmospheric circulation (Tolstykh et al., Citation2010) that is used for routine seasonal forecasts in Russian Hydrometcenter.
shows the anomaly correlations for multiyear mean (1980–2014) DJF-averaged fields of 2-m air temperature, air temperature at 850 hPa, geopotentials of isobaric surfaces 200 hPa and 500 hPa, precipitation rate and sea-level pressure in several climatic zones. Correlation coefficients were calculated using the GPCP v.2.3 reanalysis monthly mean precipitation rate and ERA – Interim reanalysis data for all other weather fields interpolated to the model grid. The results obtained for INM-CM5-0 climate model are in good agreement with SLAV. The global anomaly correlations for 2-m air temperature field, 200 hPa and 500 hPa geopotentials exceed the same results for the SLAV model and for 850 hPa air temperature field correlation is the same in both models. INM-CM5-0 has a better predictive skill for 2-m air temperature and geopotential of 200 hPa in the Northern extratropics, and an equal result for the correlation of 500 hPa geopotential field and precipitation rate in this region, although in other regions the predictability of precipitation is lower than for SLAV. It should be noted that the precipitation forecasting is a complex issue and remains poor in many models (Kiktev et al., Citation2018). In the tropics INM-CM5-0 climate model better describes the 850 hPa air temperature. Moreover, it can be seen that for the most fields correlation coefficients have the highest values in the tropics, because of Niño phenomena providing higher seasonal predictability in the region.
Table 1. The correlation coefficients of 2-m air temperature anomalies (), anomalies of air temperature at 850 hPa (
), geopotential anomalies of the isobaric surface 200 hPa (
), geopotential anomalies of the isobaric surface 500 hPa (
), anomalies of precipitation rate (
), sea-level pressure anomalies (
) for four regions: globally, in the tropics (20°S–20°N), Northern extratropics (20°N–90°N), Southern extratropics (90°S N–20°S).
The anomaly correlation for the 2-m air temperature in the tropics during the winter seasons of 1980–2014 is presented in the . The ONI (Oceanic Niño Index) is used to identify El Niño and La Niña phenomena. ONI is calculated as a three consecutive months average anomaly of ocean surface temperature (SST) in the Niño region 3.4. If the anomaly is positive and exceeds 0.5°, then we have El Niño, while in the case of negative anomalies with absolute value above 0.5° we have La Niña. Depending on the absolute value of ONI, phenomena are classified into weak (0.5°–0.9°), moderate (1.0°–1.4°), strong (1.5°–1.9°) and very strong (more than 2.0°). It can be seen that correlation coefficients have higher values in El Niño and La Niña years, and in many cases the values are higher the more pronounced phenomena are.
Table 2. Anomaly correlation for 2-m air temperature anomalies () in the tropics (20°S–20°N) for each winter season beginning in December 1980–2014, and the average value for all winter seasons.
Correlation coefficients of INM-CM5-0 and ERA-Interim reanalysis monthly mean air temperature and U-component of the wind speed averaged along the circle of latitudes, from 60°N to 88°N for air temperature and from 60°N to 70°N for wind speed at different heights are presented in and , respectively. The largest values of correlation are observed in both cases mainly in the first two months, with the exception of the stratosphere, where results of experiments have much better agreement with observations at 1–10 hPa in December-February for air temperature.
Fig. 1. Correlation coefficients of INM-CM5-0 and ERA-Interim reanalysis monthly mean air temperature averaged along the circle of latitudes and from 60°N to 88°N at different heights.

Fig. 2. Correlation coefficients of INM-CM5-0 and ERA-Interim reanalysis monthly mean U-component of the wind speed averaged along the circle of latitudes and from 60°N to 70°N at different heights.

shows the correlation coefficients of INM-CM5-0 and ERA-Interim reanalysis monthly mean geopotential field averaged along the circle of latitudes and from 60°N to 88°N at different heights. As can be seen from the figure, the region of high correlations spreads downward during November–December, that means the influence of the stratosphere on the lower atmospheric layers. Downward propagation of high correlations can lead to high correlation in NAO index discussed below at least partially. In the air temperature, relatively high correlation regions remain in the upper stratosphere throughout all winter season because of a noticeable trend in temperature, about minus 1 K per 10 years, because of ozone decrease and CO2 increase. This leads also to relatively high correlation in geopotential above 5 hPa during whole winter. Correlation in wind decreases to near zero values at the end of the season because wind depends on gradient of temperature rather than on temperature itself, but trend in temperature gradient induced by anthropogenic forcing is not very strong.
3.2. On the predictability of the North Atlantic Oscillation (NAO) Index
North Atlantic Oscillation is the reason of the most notable fluctuations in the Northern Hemisphere climate, which is reliable for the total variability of the large-scale oceanic and atmospheric fields in the North Atlantic and surrounding continental regions (Barnston and Livezey, Citation1987; Hurrell, Citation1995; Polonsky et al., Citation2004). Midlatitude westerly transport of relatively humid and warm air from the North Atlantic to Europe, depends on the pressure gradient between the Azores and Southwest Iceland (Polonsky et al., Citation2004). This pressure gradient fluctuation is defined as North Atlantic Oscillation.
NAO index is the measure of North Atlantic Oscillation. There are different approaches of NAO index calculation. We calculate NAO index following (Hurrell and Deser, Citation2009) as the time series of the leading Empirical Orthogonal Function (EOF) of sea level pressure anomalies over the Atlantic sector 20°–80°N, 90°W–40°E. Namely, NAO index was computed as the winter months average projection coefficient of ensemble mean monthly model sea level pressure anomalies to the first EOF of monthly reanalysis sea level pressure anomalies over the Atlantic sector. The results obtained for the INM RAS climate model for the period of 1980–2014 are presented in . In order to assess the skill of the INM RAS model in winter NAO, the indices for ERA-Interim reanalysis and instrumental CRU data (Jones et al., Citation1997) were also calculated.
Fig. 4. Time series of DJF NAO index. Maximum and minimum of the INM-CM5-0 10-member ensemble are shown as a blue dashed lines. INM-CM5-0 ensemble averaged NAO index is plotted in red. The ERA-Interim and instrumental CRU NAO indices are shown in black and green, respectively.

The temporal correlation coefficients of the INM-CM5-0 NAO indices and both, ERA-Interim reanalysis and instrumental CRU data NAO indices, were found. For the INM-CM5-0 the correlation coefficient reaches the value of 0.71 (interval 0.39–0.88 for 95% confidence level) with ERA-Interim reanalysis and 0.68 with instrumental CRU data for 1991–2010, whereas all other seasonal forecast systems and coupled climate models show lower results. Baker et al. (Citation2018) showed that correlation coefficient for NAO index for any individual present day forecast system is not so high. The highest correlation coefficient about 0.6 was found for MetOffice GloSea5-GA3 system for 25 ensemble members. Correlation coefficient can reach 0.70 for multimodel ensemble only.
Therefore, an important issue is to examine the causes of such high model NAO predictability skill. There are several factors that potentially affect the winter NAO index: North Pacific sea surface temperature anomalies, autumn–early winter Eurasian snow cover anomalies (Wu et al., Citation2011), Arctic sea ice anomalies, quasi-biennial oscillation (QBO) in the equatorial stratosphere, anomalies in the Atlantic ocean state, both in tropical and middle latitudes. In the first four cases effect mainly occurs through the excitation of stratosphere anomalies and their downward propagation. The analysis of probable sources of NAO predictability for the INM RAS climate model showed small correlation of NAO index with the temperature both in the extratropical Pacific Ocean and in the tropic latitudes. Correlation of model ensemble mean DJF NAO index with the equatorial QBO index is 0.24 only for 35 seasons. It is also insignificant as well as in the real climate system.
Correlation of autumn snow and NAO index during next winter is widely discussed in the literature (see, for example, Wu et al. Citation2011; Kim et al. Citation2013; Orsolini et al. Citation2016; Wegmann et al. Citation2020). In the model ensemble, there is correlation of initial snow depth and NAO index durnig next winter. Regression of snow depth prescribed for November 1 onto ensemble mean DJF NAO index can be seen in .
Fig. 5. Regression of initial snow water equivalent thickness (cm) prescribed for Nov. 1 on DJF NAO index.

Low snow amount in the most part of Siberia corresponds with high NAO index, as commonly known. Additional model ensemble runs were performed to study the response of DJF NAO index to initial snow depth. In the first series, initial state of snow depth for November 1 was prescribed as mean over data for November 1 of 1980–2014 plus anomaly presented in . In the second series, mean state minus anomaly in was prescribed for snow. Other prognostic variables, including atmospheric, oceanic and soil state were prescribed as mean for 35 years in the same way for the first and the second series. Ten ensemble members were run in both series. Runs show that there is no confidence difference between two model run series in DJF NAO index. This means that initial snow depth is probably an indicator only of some atmospheric processes that defines future DJF NAO index rather than forcing for winter NAO. Analysis of initial sea ice showed that correlation of November Arctic sea ice compactness and DJF NAO is weak in the model. So, further studies are needed to explain high predictability of NAO index.
3.3. Predictability of the PNA index
The position of mean midtropospheric stationary waves over North America is fixed by orographic forcing of the Rocky Mountains, Tibetan Plateau and the land-sea temperature contrasts along the eastern coasts of Asia and North America (Held, Citation1983; Chen and Trenberth, Citation1988a, Citation1988b). The normal PNA pattern features an atmospheric pressure ridge over western North America and a trough over eastern North America. Changes in the cyclones and anticyclones position and strength lead to the jet stream variation. The PNA index is an important predictor of North American climate, which represents an intensity and location departure of coming from the east of Asia tropospheric flow over the continent from the mean value (Rodionov and Assel, Citation2001). An amplification of the PNA stationary wave determines positive PNA index, whereas the damping means negative PNA index (Leathers and Palecki, Citation1992).
The PNA index is strongly correlated with monthly temperatures in many US climatic divisions, with the centers of highest correlation in the Pacific Northwest and the Southeast (Leathers et al., Citation1991). Correlations between the PNA index and precipitation are weaker and less extensive than they are for temperature, but large coherent regions of moderately high correlations are observed across the nation (Leathers et al., Citation1991).
Positive values of PNA index indicate positive temperature anomaly on the west coast of North America and negative temperature anomaly across south-eastern and south-central part of USA. Increased precipitation is observed on the west coast of Alaska and United States, the Gulf of Mexico north coast and Florida. Droughts are associated with the United States Midwest, the Canadian Prairies and the Pacific Northwest. Completely opposite situation characterizes the case of negative PNA index. The positive phase of PNA pattern tends to appear in the El Niño years, while negative phase is associated more often with La Niña episodes.
The PNA index was calculated using the (Wallace and Gutzler, Citation1981) formulae adopted for the INM RAS climate model grid:
where
is the normalized 500 mb geopotential height anomaly found using the standard deviation and 1980–2015 period monthly means,
is the number of a month from January to December,
is the number of a year in 1980–2014 period,
is the longitude and
is the latitude. The result of calculations is presented in . It is seen that the reanalysis PNA index varies in the 10-member ensemble spread of model data almost during the whole period of 1980–2015, while the values of PNA index calculated using ensemble averaged 500 mb geopotential height anomaly significantly exceed the maximum limit of the range in 1997–1998 winter season and diverge more from the reanalysis curve than the ensemble mean PNA value.
Fig. 6. Time series of DJF PNA index. Maximum and minimum of the INMCM 10-member ensemble are shown as a blue dashed lines, ensemble mean is presented as a blue solid line. PNA index calculated using ensemble averaged 500 mb geopotential height anomaly is plotted in red. The ERA-Interim PNA index is shown in black.

In order to estimate the ability of the INM RAS climate model to forecast the value of PNA index, the correlation coefficients of the model and ERA-Interim reanalysis PNA indices for each month from November to February of the period 1980–2015 were computed. summarizes obtained results. Correlation coefficients decrease with the growth of month number, since the shift between model prediction and reanalysis rises during the model run. The DJF-month averages correlation equals 0.60 (interval 0.33–0.78 for 95% confidence level). Correlation coefficient for seasonal mean is higher than that one calculated for each winter month separately as the random fluctuations are averaged, and the contribution of the model response to El Niño event increases.
Table 3. Table summarizing the correlation coefficients of the model and ERA-Interim reanalysis PNA indices for DJF-month averages and for each month from November to February of the period 1980–2015.
shows model and reanalysis PNA indices for winter months of the years with the strongest El Niño and La Niña events during the period of 1980–2014. Actually, in the years of the most pronounced El Niño the values of PNA index are noticeably greater than zero, and for La Niña years they are significantly negative.
Table 4. Model and reanalysis PNA indices for winter months of the years with the strongest El Niño and La Niña events during the period of 1980–2014.
3.4. Stratospheric anomalies prediction skill
The model ability of large-scale processes (e.g. sudden stratospheric warming event) forecasting, might be an important source of seasonal predictability since due to the global teleconnections they determine the future development of the climate situation throughout the Northern Hemisphere. In particular, the hypothesis whether high skill in predicting the winter NAO can be explained by the stratospheric anomalies prediction skill should be checked.
The first empirical orthogonal function (EOF) calculated using monthly mean air temperature of the Northern Hemisphere at different heights averaged for the zone from 60° to 88° N and along the circle of latitudes for ERA-Interim reanalysis data during winter seasons of 1980–2015 is presented in . The ordinal number of a month was taken as a spatial coordinate that allowed computing EOFs characterizing the intraannual variability of the selected parameter. The EOF represents downward propagation of temperature anomalies in polar stratosphere. It corresponds to the polar night jet oscillation (PJO) studied in Kuroda and Kodera (Citation2001). Their representing regression of polar temperature on PJO index looks similar to our . In Kuroda and Kodera (Citation2001) and Kuroda and Kodera (Citation2004) it was shown that downward propagation of stratospheric anomaly leads to anomaly in AO index.
Fig. 7. The first EOF of monthly mean air temperature calculated from ERA-Interim reanalysis data (1980–2015) in the Northern Hemisphere at the pressure levels from 1000 to 1 hPa averaged for the latitudinal zone from 60° to 88° N.

In Vorobyeva and Volodin (Citation2018) using an ensemble of the model experiments starting on the December 1 it was found that it is possible to predict the positive projection to the first mode of monthly mean air temperature during December–March in the years with a large positive anomaly of the zonal mean meridional heat flux in December. This hypothesis is used for the bias-corrected seasonal hindcasts based on the INM-CM5-0 model since the projection of monthly mean air temperature anomaly to the first EOF of reanalysis data was accepted as the main index characterizing the dynamics of the stratosphere. To examine the model stratospheric anomalies prediction skill the ensemble averaged projections for winter seasons during the period of 1980–2015 were compared with similar projections calculated for reanalysis data. The result of calculations is shown in . The correlation coefficient for the time series of INM-CM5-0 and ERA-Interim projections performs the value of 0.47 (interval 0.16–0.69 for 95% confidence level), which is less than for NAO index. Therefore, the stratospheric variability provides a significant contribution, although potentially is not the only cause of model high skill in seasonal NAO index prediction, which is also likely due to initial state of the troposphere.
Fig. 8. The projection coefficient of the ensemble averaged monthly mean air temperature anomaly to the first EOF of reanalysis data for each winter season during the period of 1980–2014 for the INM-CM5-0 (shown in red) and a similar projection for reanalysis (plotted in green). Monthly mean air temperature was averaged along the circle of latitudes and for the zone from 60° to 88° N.

To review the findings from the years with maximum model stratospheric anomalies of both signs were chosen. The winter season of 1986–1987 is considered for the largest positive air temperature anomaly and the winter season of 1988–1989 regards to the largest negative anomaly. As it can be seen from the and , INM-CM5-0 air temperature anomaly during November–March of these years reaches smaller values than for ERA-Interim. Nevertheless, the vertical distributions of air temperature anomaly have similar features, such as the same order of alternating extremes and the change of anomaly signs in the same time.
Fig. 9. The vertical distribution of air temperature anomaly for: a) INM-CM5-0 ensemble averaged data; b) ERA-Interim reanalysis during the period of November 1986–March 1987. Anomalies are averaged along the circle of latitudes and for the zone from 60° to 88° N.

Fig. 10. The vertical distribution of air temperature anomaly for: a) INM-CM5-0 ensemble averaged data; b) ERA-Interim reanalysis during the period of November 1988–March 1989. Anomalies are averaged along the circle of latitudes and for the zone from 60° to 88° N.

Model data shows that stratospheric signal of El Niño is not very strong. During 110 El Niño seasons we have 76 major sudden stratospheric warmings (SSWs) defined according to WMO critreia (McInturff, Citation1978) in individual model forecast runs. During 120 La Niña seasons there are 83 major SSWs, and during 120 seasons with neutral conditions model produced 93 major SSWs. This means that there is no much difference in major SSW statistics between El Niño, La Niña and neutral years. Analysis of ensemble of historical runs with INM-CM5-0 showed that winter stratospheric response to El Niño is maximum in February-March, and polar stratosphere is 1–2 K warmer in El Niño years than in La Niña years. So, El Niño can potentially play some role in explanation of model performance in simulation of observed stratospheric anomalies, but some other mechanisms should play significant role.
4. Conclusion
This paper describes research on INM-CM5-0 seasonal predictability skill in basic fields and key patterns – North Atlantic Oscillation, Pacific North American pattern and stratospheric variability.
The anomaly correlations for multiyear mean (1980–2014) DJF-averaged fields of 2-m air temperature, air temperature at 850 hPa, geopotentials of isobaric surfaces 200 hPa and 500 hPa, precipitation rate and sea-level pressure in several regions (globally, in the tropics (20° S–20° N), Northern Extratropics (20° N–90° N) and Southern Extratropics (90° S–20° S)) were calculated. The results are compared with similar results of the SLAV model developed for routine seasonal forecasts. Similarity is shown. Moreover, anomaly correlations for some variables have greater values than for SLAV. Anomaly correlation for precipitation is low. An analysis of the anomaly correlation for 2 m air temperature in the tropics for each winter season showed an increase in the correlation during the years with El Niño and La Niña events.
The temporal correlation coefficient of the INM-CM5-0 NAO index attained a value of 0.71 with ERA-Interim reanalysis and 0.68 with instrumental CRU data. It was shown, that the correlation coefficient for the time series of INM-CM5-0 and ERA-Interim projections of monthly mean air temperature anomaly to the first EOF of reanalysis data performs the value of 0.47, that means the stratospheric variability provides a significant contribution, although potentially is not the only cause of model high skill in NAO index predictability. Analysis of major SSW occurrence during El Niño, La Niña seasons and seasons with neutral conditions allows to conclude that ENSO events can potentially play some role in explanation of model performance in simulation of model stratospheric anomalies, but some other mechanisms should play significant role. Initial data of temperature both in the extratropical Pacific Ocean and in the tropic latitudes as well as November equatorial QBO index, Arctic sea ice compactness and snow depth demonstrate weak correlation with DJF NAO index.
The correlation coefficients of the INM RAS climate model and ERA-Interim reanalysis PNA indices for each month from November to February of the period 1980–2015 were computed and showed acceptable results. As was expected, in the years of the most pronounced El Niño the values of PNA index are significantly positive, and for La Niña years they are noticeably less than zero.
The obtained results allow to expect the INMCM tolerable seasonal prediction skill, which examination and development is necessary to expand in a future study.
Disclosure statement
No potential conflict of interest was reported by the authors.
Additional information
Funding
References
- Baker, L. H., Shaffrey, L. C., Sutton, R. T., Weisheimer, A. and Scaife, A. A. 2018. An intercomparison of skill and overconfidence/underconfidence of the wintertime North Atlantic Oscillation in multimodel seasonal forecasts. Geophys. Res. Lett. 45, 7808–7817. doi:https://doi.org/10.1029/2018GL078838
- Barnston, A. G. and Livezey, R. F. 1987. Classification, seasonality and persistence of low frequency atmospheric circulation patterns. Mon. Wea. Rev. 115, 1083–1126. doi:https://doi.org/10.1175/1520-0493(1987)115<1083:CSAPOL>2.0.CO;2
- Brown, A., Milton, S., Cullen, M., Golding, B., Mitchell, J. and co-authors. 2012. Unified modeling and prediction of weather and climate: A 25-year journey B. Am. Meteorol. Soc. 93, 1865–1877. doi:https://doi.org/10.1175/BAMS-D-12-00018.1
- Chen, S. C. and Trenberth, K. E. 1988a. Orographically forced planetary waves in the Northern Hemisphere winter: Steady state model with wave-coupled lower boundary formation. J. Atmos. Sci. 45, 657–680. doi:https://doi.org/10.1175/1520-0469(1988)045<0657:OFPWIT>2.0.CO;2
- Chen, S. C. and Trenberth, K. E. 1988b. Forced planetary waves in the Northern Hemisphere winter: Wave-coupled and thermal forcings. J. Atmos. Sci. 45, 682–704. doi:https://doi.org/10.1175/1520-0469(1988)045<0682:FPWITN>2.0.CO;2
- Held, I. M. 1983. Stationary and quasi-stationary eddies in the extratropical stratosphere: Theory. In: Large-scale dynamical processess in the atmosphere (eds. B. J. Hoskins and R. P. Pearce). New York: Academic Press, pp. 127–268.
- Hoskins, B. 2013. The potential for skill across the range of the seamless weather-climate prediction problem: A stimulus for our science.Q. J. R. Meteorol. Soc. 139, 573–584. doi:https://doi.org/10.1002/qj.1991
- Hurrell, J. W. 1995. Decadal trends in the North Atlantic oscillation: Regional temperatures and precipitation. Science 269, 676–679. doi:https://doi.org/10.1126/science.269.5224.676
- Hurrell, J. W. and Deser, C. 2009. North Atlantic climate variability: The role of the North Atlantic Oscillation. J. Mar. Syst. 78, 28–41. doi:https://doi.org/10.1016/j.jmarsys.2008.11.026
- Jones, P. D., Jónsson, T. and Wheeler, D. 1997. Extension to the North Atlantic Oscillation using early instrumental pressure observations from Gibraltar and South-West Iceland. Int. J. Climatol. 17, 1433–1450. doi:https://doi.org/10.1002/(SICI)1097-0088(19971115)17:13<1433::AID-JOC203>3.0.CO;2-P
- Kiktev, D. B., Tolstykh, M. A., Zaripov, R. B., Kruglova, E. N., Kulikova, I. A. and co-authors. 2018. Results of joint operational testing of technology of detailed ensemble long-range forecasts based on global models of Hydrometcenter of Russia and Voeikov Main Geophysical Observatory. Gidrometeorologicheskie Issledovaniya i Prognozy [Hydrometeorological Research and Forecasting] 367, 116–133. [in Russ.].
- Kim, Y., Kim, K. and Kim, B. 2013. Physical mechanisms of European snow cover variability and its relationship to the NAO. Clim. Dyn. 40, 1657–1669. doi:https://doi.org/10.1007/s00382-012-1365-5
- Kuroda, Y. and Kodera, K. 2001. Variability of the polar-night jet in the northern and southern hemispheres. J. Geophys. Res. 106, 20703–20713. doi:https://doi.org/10.1029/2001JD900226
- Kuroda, Y. and Kodera, K. 2004. Role of the polar-night jet oscillation on the formation of Arctic Oscillation in the Northern Hemisphere winter. J. Reophys. Res. 109, D11112.
- Leathers, D. J. and Palecki, M. A. 1992. The Pacific/North American teleconnection pattern and United States climate. Part II. Temporal characteristics and index specification. J. Climate 5, 707–716. doi:https://doi.org/10.1175/1520-0442(1992)005<0707:TPATPA>2.0.CO;2
- Leathers, D. J., Yarnal, B. and Palecki, M. A. 1991. The Pacific/North American teleconnection pattern and United States climate. Part I: Regional temperature and precipitation associations. J. Clim. 4, 517–528. doi:https://doi.org/10.1175/1520-0442(1991)004<0517:TPATPA>2.0.CO;2
- McInturff, R. M., Ed. 1978. Stratospheric warmings: Synoptic, dynamic and general-circulation aspects. NASA Reference Publ. NASA-RP-1017, 174 pp. Online at: http://ntrs.nasa.gov/archive/nasa/casi.ntrs.nasa.gov/19780010687.pdf
- Molteni, F., Stockdale, T., Balmaseda, M. et al. 2011. The new ECMWF seasonal forecast system (System 4). ECMWF Tech. Memoran. 656, 49.
- Orsolini, Y. J., Senan, R., Vitart, F., Balsamo, G., Weisheimer, A. and co-authors. 2016. Influence of the Eurasian snow on the negative Noteh Atlantic oscillation in subseasonal forecasts of the cold winter 2009/2010. Clim. Dyn. 47, 1325–1334. doi:https://doi.org/10.1007/s00382-015-2903-8
- Polonsky, A. B., Basharin, D. V., Voskresenskaya, E. N. et al. 2004. Relationship between the North Atlantic Oscillation, Euro-Asian climate anomalies and Pacific variability. Pac. Oceanogr. 2, 52–66.
- Rodionov, S. and Assel, R. 2001. A new look at the Pacific/North American Index. Geophys. Res. Lett. 28, 1519–1522. doi:https://doi.org/10.1029/2000GL012185
- Stockdale, T., Johnson, S., Ferranti, L. et al. 2018. ECMWF`s new long-range forecasting system SEAS5. ECMWF Newslett. 154, 15–20.
- Tolstykh, M. A., Kiktev, D. B., Zaripov, R. B., Zaichenko, Y. and Shashkin, V. V. 2010. Simulation of the seasonal atmospheric circulation with the new version of the semi-Lagrangian atmospheric model. Izv. Atmos. Ocean. Phys. 46, 133–143. doi:https://doi.org/10.1134/S0001433810020015
- Volodin, E. M. and Gritsun, A. S. 2018. Simulation of observed climate changes in 1850–2014 with climate model INM-CM5. Earth Syst. Dynam. 9, 1235–1242. doi:https://doi.org/10.5194/esd-9-1235-2018
- Volodin, E. M., Mortikov, E. V., Kostrykin, S. V., Galin, V. Y., Lykossov, V. N. and co-authors. 2017. Simulation of the present day climate with the climate model INMCM5. Clim. Dyn. 49, 3715–3734. doi:https://doi.org/10.1007/s00382-017-3539-7
- Vorobyeva, V. V. and Volodin, E. M. 2018. Investigation of the structure and predictability of the first mode of stratospheric variability based on the INM RAS climate model. Russ. Meteorol. Hydrol. 43, 737–742. doi:https://doi.org/10.3103/S1068373918110043
- Wallace, J. M. and Gutzler, D. S. 1981. Teleconnections in the geopotential height field during the Northern Hemisphere winter. Mon. Wea. Rev. 109, 784–812. doi:https://doi.org/10.1175/1520-0493(1981)109<0784:TITGHF>2.0.CO;2
- Wegmann, M., Rohrer, M., Santolaria-Otin, M. and Lohmann, G. 2020. Eurasian autumn snow link to winter North Atlantic oscillation is strongest for Arctic warming periods. Earth Syst. Dynam. 11, 509–524. doi:https://doi.org/10.5194/esd-11-509-2020
- Wu, Q., Hu, H. and Zhang, L. 2011. Observed influences of autumn–early winter Eurasian snow cover anomalies on the hemispheric PNA-like variability in winter. J.Clim. 24, 2017–2023. doi:https://doi.org/10.1175/2011JCLI4236.1