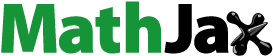
Abstract
This study developed a hybrid four-dimensional (4D) ensemble–variational (4DEnVar) radar data assimilation (DA) system for the Weather Research and Forecasting model. The 4DEnVar approach incorporated ensemble covariances at multiple time levels to assimilate observations distributed in the assimilation windows. By approximating the evolution of the background error using 4D ensemble covariance, use of the tangent linear and adjoint models was avoided. The impact of 4DEnVar radar DA on convective-scale analyses and forecasts was examined through comparison with 3DVar and 3DEnVar methods for the case of a squall line that occurred over southeastern China. In comparison with the other methods, 4DEnVar produced both smaller root mean square innovations for radar reflectivity and radial velocity and better analysis of the vertical structure of reflectivity. The corresponding relative humidity and vertical wind in convective regions were strengthened. Ultimately, 4DEnVar produced a substantially improved forecast, including improved quantitative precipitation and reflectivity forecast skill, and better representation of the squall line in terms of both areal coverage and intensity. In contrast, 3DEnVar improved the analysis and forecast modestly in comparison with 3DVar. Furthermore, sensitivity experiments indicated that a moderate assimilation window and a stronger ensemble weighting factor used in 4DEnVar could produce superior forecast results. The wind, temperature and water vapor were also improved by 4DEnVar, with the largest bias reduction for water vapor at low and middle levels. The improvements of 4DEnVar were further verified and shown effective using a mesocale convective system case and a local convection case.
1. Introduction
Owing to its high spatial and temporal resolutions, Doppler radar is a valuable source of data used widely to initialize convection-allowing numerical models with advanced data assimilation (DA) methods (Xiao et al., Citation2005; Tong and Xue, Citation2005; Sun et al. Citation2014). Among the various existing DA methods, three-dimensional variational DA (3DVar) is the simplest and least computationally expensive. It is used in a number of real-time applications of radar DA (Gao et al., Citation2004; Hu et al., Citation2006; Hu and Xue, Citation2007; Schenkman et al., Citation2011; Xie et al., Citation2011), e.g., the Advanced Regional Prediction System 3DVar system implemented in the Hazardous Weather Testbed Spring Forecasting Experiments (Clark et al., Citation2012) and the Weather Research and Forecasting (WRF) 3DVar radar DA system run at the Korea Meteorology Administration (KMA; Xiao et al., Citation2008). However, the background error covariance (BEC) of 3DVar is assumed static and isotropic, which might vary substantially depending on the actual convective-scale flows during radar DA.
The more advanced 4DVar method can implicitly develop the flow-dependent BEC by incorporating the tangent linear model into the radar DA procedure; however, the BEC at the beginning of the DA window is usually assumed static and not propagated from one DA window to another (Sun and Crook, Citation1997, Citation1998; Sun and Wang, Citation2013; Wang et al., Citation2013a). The ensemble Kalman filter (EnKF), which is another DA method that shares many advantages of 4DVar, can estimate the flow-dependent BEC using a group of ensemble forecasts. The capabilities of EnKF in the assimilation of radar observations for convective storms have been shown by many previous related studies (Snyder and Zhang, Citation2003; Dowell et al., Citation2004; Tong and Xue, Citation2005; Xue et al., Citation2006, Putnam et al., Citation2014). Nevertheless, despite encouraging earlier results, the performance of EnKF remains severely affected by the sampling and model errors, which can often lead to the rank deficiency problem.
To overcome the respective shortcomings of both variational and EnKF methods, a hybrid method that blends the advanced features of the ensemble-based and variational methods has been proposed by Hamill and Snyder (Citation2000) and by Lorenc (Citation2003). The hybrid algorithm incorporates the ensemble BEC into the cost function during variational DA, and it has been proven more robust than EnKF when the ensemble size is small or the model errors are large (Wang et al. Citation2009). The latter formulation, which introduces the flow-dependent BEC by augmenting extended control variables, is more flexible and more easily implemented in existing variational frameworks. A number of earlier studies established the effectiveness of the hybrid approach based on the extended control variable method in providing initial conditions for large-scale and mesoscale numerical weather prediction models (e.g. Buehner, Citation2005; Wang et al., Citation2008a, Citation2008b; Zhang et al., Citation2013; Pan et al., Citation2014; Wu et al., Citation2017).
Several previous studies also demonstrated the superiority of the hybrid method in assimilating radar observations to initialize convective-allowing models (e.g. Gao et al., Citation2013; Gao and Stensrud, Citation2014; Gao et al., Citation2016; Wang and Wang, Citation2017; Kong et al., Citation2018). Gao et al. (Citation2013) were the first to assimilate simulated radar observations for an idealized supercell using a hybrid 3DVar and EnKF radar DA system for the Advanced Regional Prediction System model. Their results indicated that the hybrid method produced the best analyses for most variables when observations from two radars were assimilated. As suggested by Bowler et al. (Citation2013), this approach could be called the three-dimensional ensemble–variational DA (3DEnVar) method. Recently, Wang and Wang (Citation2017) proposed a new reflectivity assimilation scheme for the Gridpoint Statistical Interpolation 3DEnVar by directly adding reflectivity as a state variable to avoid spuriously small and large hydrometeor increments in the analysis. Wang et al. (Citation2019) developed and tested a real-time, dual-resolution hybrid Warn-on-Forecast analysis and forecast system, and found that the reflectivity pattern and the updraft helicity tracks could be predicted accurately. In the above studies, the hybrid 3DEnVar method was used, in which the temporal evolution of the error covariance within the assimilation window is not considered.
As a natural extension of 3DEnVar, the four-dimensional ensemble–variational DA (4DEnVar) method incorporates 4D ensemble covariances spanning multiple time periods for fitting the model trajectory to the observations distributed within a finite assimilation window. In 4DEnVar, the temporal evolution of the error covariance is approximated efficiently using the covariances of ensemble perturbations at discrete times, which avoids use of the tangent linear model of the forecast model. The potential of the 4DEnVar approach has been demonstrated using simple models and simulated observations (Kleist et al. Citation2009; Buehner et al. Citation2010a, Citation2010b; Wang and Lei, Citation2014; Lorenc et al., Citation2015), e.g., in the DA system of the Canadian operational global numerical weather prediction model (Buehner et al., Citation2010b), and the U.S. Navy’s operational ensemble forecasting system (Bishop and Hodyss, Citation2011).
Despite these encouraging results, the studies of convective-scale application of 4DEnVar to assimilate radar observation have been limited. The purpose of this study is to develop a 4DEnVar radar DA system, and explore its potential for assimilating radar observations to initialize the WRF model, as well as the impact of such a system on the prediction of severe convective events. This system adopted velocity components as new control variables and an indirect scheme to assimilate radar reflectivity (Wang et al., Citation2013b; Gao et al., Citation2018). Several experiments were conducted to investigate both the impact of assimilating radar observations using the 4DEnVar method and the sensitivity of the 4DEnVar approach to two key parameters. These experiments were conducted for a case study of a severe squall line that occurred over southeastern China. To further demonstrate the effectiveness of this hybrid 4DEnVar method, the forecast skills from a mesocale convective system (MCS) case and a local convection case were determined.
The remainder of this paper is organized as follows. The details of the WRF 4DEnVar systems are described in Section 2. Section 3 provides an overview of the squall line case study, and Section 4 presents the configurations of the experiments. Section 5 compares the analysis and forecast results, and our conclusions and a discussion are given in Section 6.
2. Method
2.1. General description of the WRF 4DEnVar system
The flow-dependent ensemble BECs validated at multiple time levels spanning from 0 to are incorporated into 3DVar to assimilate observations within a DA window by augmenting the extended control variable method (Lorenc, Citation2003). The cost function of 4DEnVar (
) that contains the background term (
), ensemble term (
), and observation term (
), can be expressed as:
(1)
(1)
Here, represents the observation innovation vector, where
and
are the observation, analysis variable, and observation operator, respectively, at time period
is the linearized version of
and
is the observation error covariance matrix;
and
denote the static BEC and the related analysis increment, respectively;
is the ensemble covariance localization matrix; and
and
are the weighting coefficients for the static and flow-dependent BECs, which satisfied
The total increment
can be calculated as follows:
(2)
(2)
Here, vector is the perturbation of the i-th ensemble member normalized by
where
is the ensemble size; vectors
are the extended control variables for each ensemble member, and symbol
denotes scalar multiplication; In contrast, 4DVar calculates the increment at time
using the equation
where
represents the tangent linear model, and
denotes the analysis increment to be optimized using the adjoint model. Unlike 4DVar, 4DEnVar employs 4D ensemble covariances to estimate efficiently the time evolution of the error covariance (EquationEq. (2)
(2)
(2) ), which means use of the tangent linear and adjoint models can be avoided.
2.2. Indirect reflectivity assimilation scheme
Wang et al. (Citation2013b) proposed that direct assimilation of reflectivity suffers from linearization errors attributable to the observation operator. Therefore, an indirect reflectivity scheme was adopted that assimilated the hydrometer mixing ratios (), which included the rainwater mixing ratio (
), snow mixing ratio (
), and graupel mixing ratio (
) retrieved from reflectivity. The cost function associated with these hydrometer mixing ratios can be expressed as follows:
(3)
(3)
In EquationEq. (3)(3)
(3) , the first and second terms represent the background and observation terms for
where
and
are the background and observation error and covariances, respectively. As suggested by Wang et al. (Citation2013b), only the horizontal correlation within
is considered, and the corresponding variance and length scales are set to 4.0 g kg−1 and 10.5 km, respectively, and
is set to
Except for these hydrometer mixing ratios, the other control variables used in this study include velocity components
and
temperature
surface pressure
and pseudo-relative humidity
It was found that the use of the stream function
and unbalanced velocity potential
as momentum control variables, posed difficulties in fitting analyses to the high-resolution observations during the variational minimization process. Therefore, this study directly adopted
and
as momentum control variables to improve the degree of fitting and precipitation prediction, as suggested by Sun et al. (Citation2016).
3. Case study overview
A severe squall line that produced reports of frequent lightning, strong winds, and large hail in southeastern China on 14 June 2009 was selected for detailed and qualitative evaluation of the 4DEnVar method. The evolution of the squall line is illustrated using radar reflectivity observations in . At 0800 UTC, two convective clusters with maximum reflectivity of 65 dBZ had formed over Anhui and Jiangsu provinces. There were also some isolated convective cells over northern Henan, eastern Shandong, and Jiangsu provinces. At 0930 UTC, the convective clusters had developed into a linear convective system with length of 300 km and width of 150 km over the border between Jiangsu and Anhui provinces. The convective line intensified and moved southeastward with a pronounced rearward stratiform region. At 1130 UTC, the convective system was in the mature stage with a large area of reflectivity of >30 dBZ. During the southeastward shift and development, new convective cells generated and developed over Henan Province. By 1330 UTC, the convective system over Henan and Anhui provinces had strengthened and the stratiform region had expanded in area, whereas the squall line over Jiangsu Province had weakened. In addition, an MCS case and a local convection case that occurred over southeastern China were considered to demonstrate the improvements of 4DEnVar in quantitative reflectivity forecast skill.
4. Description of the experiments and verification techniques
In this study, seven experiments were conducted for the case of the squall line that occurred on 14 June 2009. These experiments are summarized in . The first three experiments involved radar DA experiments that assimilated both radar reflectivity and radial velocity using the 3DVar, 3DEnVar, and 4DEnVar approaches, respectively. The 4DEnVar experiment used a 30-min assimilation window with radar observations distributed every 5 min, and a 75% weighting value for the ensemble covariance. The other experiments (4DEnVar_L20, 4DEnVar_L40, 4DEnVar_W25, and 4DEnVar_W50) were the same as 4DEnVar but with different lengths of assimilation window (20 and 40 min) and different ensemble covariance weighting (25% and 50%). Thus, these 4DEnVar experiments were configured to investigate the sensitivity of 4DEnVar to both the length of the assimilation window and the ensemble covariance weighting. The construction of the static BEC for all the experiments adopted the National Meteorological Center method (Parrish and Derber, Citation1992). The differences of 24- and 12-h forecasts, initiated from 0.5° × 0.5° NCEP GFS data at 0000 and 1200 UTC every day during June 2009 over the inner domain, were used to construct the static BEC of control variables except for hydrometer mixing ratios. Only the horizontal correlation for the hydrometeor mixing ratios was considered, and the variance and length scale were set to 4.0 g kg−1 and 10.5 km, respectively, following Wang et al. (Citation2013b).
Table 1. List of experiments.
All experiments used a two-way, two-nested domain with the Advanced Research WRF (version 3.9.1) model. The outer (inner) domain consisted of 161 × 161 (501 × 501) horizontal grid points with horizontal grid spacing of 9 (3) km and 51 vertical grid levels stretching from the surface up to 50 hPa. The initial and lateral boundary conditions were obtained from the National Centers for Environmental Prediction Global Forecast System analyses and forecasts. shows the experimental domain and the locations of the eight weather radars used in the radar DA experiments. Both radar reflectivity and radial velocity data underwent quality control before assimilation, including the removal of ground clutter, despeckling, and velocity dealiasing (unfolding) (Brewster et al. Citation2005). The standard deviations of radial velocity and radar reflectivity observations were set to 2 m s−1 and 3 dBZ, respectively. All experiments used the same model physics that included the Rapid Update Cycle Land surface model (Smirnova et al., Citation1997), Yonsei University planetary boundary layer scheme (Hong et al., Citation2006), and Thompson microphysical scheme (Thompson et al., Citation2008).
Figure 2. Two model domains (black rectangle) superposed on terrain (unit: m, shaded). The black dots indicates the eight radars including Shangqiu radar (SQRD), Xuzhou radar (XZRD), Lianyungang radar (LYRD), Fuyang radar (FYRD), Yancheng radar (YCRD), Hefei radar (HFRD), Nanjing radar (NJRD), and Nantong radar (NTRD). The circles represent the maximum range of radars.

The 3DVar experiment, which involved a 6-h spin-up that started at 0000 UTC on 14 June 2009, was initialized from the National Centers for Environmental Prediction Global Forecast System. The radar data were assimilated during 0600–0900 UTC at a 1-h interval. For the other experiments, a 50-member ensemble was created at 0400 UTC by adding Gaussian, random, and smooth perturbations with standard deviations of 2 m s−1, 2 m s−1, 2 K, and 1 g kg−1 for the wind component,
wind component, perturbation potential temperature
and water vapor mixing ratio
respectively. Then, a 2-h ensemble forecast was conducted for the ensemble to sample the mesoscale structure, after which radar DA was conducted at a 1-h interval. The 3DEnVar experiment used only the ensemble covariance and the radar observations valid at the analysis time, whereas the 4DEnVar experiments used the ensemble covariance and radar observations at multiple time levels (at a 5-min interval) distributed within the assimilation window. For all experiments, a 6-h forecast was conducted, followed the radar DA procedure. In addition to the verification and detailed analysis based on the above case, to derive conclusions that are more generalized, the same 3DVar, 3DEnVar, and 4DEnVar experiments were conducted for an MCS case on 5 June 2009 and a local convection case on 23 June 2013.
Two common forecast statistics, i.e., the neighborhood-based fraction skill score (FSS; Roberts and Lean, Citation2008) and the BIAS (Mittermaier and Roberts, Citation2010), were used to assess quantitatively the performance of different experiments. The scores are calculated for forecast reflectivity and 1-h accumulated precipitation. The FSS score ranges from 0 to 1, with 1 signifying a perfect forecast and 0 signifying no skill. The BIAS score is above or below 1.0, when the forecast reflectivity or precipitation is higher or lower than the observation.
5. Results
5.1. 3DVar, 3DEnVar and 4DEnVar experiments
5.1.1. Analysis results
We first examined the analysis results of the 4DEnVar experiment relative to its counterpart 3DVar and 3DEnVar experiments. The root mean square innovations (RMSIs) of the analyzed fields for radar reflectivity and radial velocity calculated against the NJRD radar from 3DVar, 3DEnVar, and 4DEnVar are shown in . The RMSIs provide a measure of the overall model state in relation to the observations, and the calculations were limited to areas where the observed reflectivity was >10 dBZ. The RMSIs for all experiments generally decreased with each subsequent analysis–forecast cycle. Compared with 3DVar, the 3DEnVar and 4DEnVar experiments produced lower RMSIs in both radar reflectivity and radial velocity within the observational area of the NJRD radar, which was sufficiently close to observe the squall line. The error reduction was especially evident for the second assimilation cycle. This result is consistent with other hybrid studies showing that introduction of ensemble-estimated covariance into the variational method benefits the analysis (Gao et al., Citation2016; Kong et al., Citation2018). In comparison with the results of 3DEnVar, the RMSIs of 4DEnVar were smaller during all analysis and forecast cycles, especially for the radial velocity in the first three assimilation cycles. In addition, relative to the other experiments, 4DEnVar was found to produce lower RMSIs that were closer to the observation error standard deviations for both variables at the end of the assimilation cycle, which indicated that 4DEnVar provided a good estimate of these analysis variables.
Figure 3. Averaged root mean square innovations (RMSIs) for reflectivity (unit: dBZ) (a), and radial velocity (unit: m s−1) (b) for 3DVar (dashed gray curve), 3DEnVar (solid gray curve) and 4DEnVar (solid black curve) during the assimilation period. The x-axis is time (hour) starting at 0600 UTC 14 June 2009.

The analyzed vertical reflectivity along the line from 32.4°N, 116.1°E to 33.6°N, 120.9°E for each of the experiments is shown in at the end of the assimilation step. It can be seen that 3DVar almost missed the two strong convective cells observed at x = 100 and 300 km that extended up to 15 km. The analyzed reflectivity of both 3DEnVar and 4DEnVar in this region was less intense and smaller in extent than observed. However, it was most significant for 4DEnVar with more values >40 dBZ that matched the observations better. Differences among the experiments were also apparent both in the vertical structure of the relative humidity and in the vertical wind fields (). It is clear that 4DEnVar analyzed higher relative humidity and stronger updrafts in the convective regions, conductive to the development of convection.
Figure 4. Vertical cross sections of the radar reflectivity (unit: dBZ) (a–d), and relative humidity (unit: %, shaded) and vertical wind (unit: m s−1, vectors) (e–g) along line from 32.4°N, 116.1°E to 33.6°N, 120.9°E from observation (a), 3DVar (b, e), 3DEnVar (c, f), and 4DEnVar (d, g) at the end of the assimilation step. Solid black lines indicate regions of observed reflectivity exceeding 45 dBZ.

5.1.2. Reflectivity forecasts
In order to examine the different forecast results of the three experiments, shows the averaged FSS and BIAS scores for the 6-h forecasts from 3DVar, 3DEnVar, and 4DEnVar for radar reflectivity at the 25, 35, and 45 dBZ thresholds. Generally, the FSS decreased in all forecasts and the highest values were found for the 25-dBZ threshold (). The FSSs for 3DEnVar and 4DEnVar were substantially higher than for 3DVar for all thresholds during the entire forecast period. Meanwhile, it was found that the overall performance of 4DEnVar was better than that of 3DEnVar, especially for the 45-dBZ threshold between the second and fourth hours. In contrast, 3DVar showed a rapid decrease of the FSS in the first three hours for the 45-dBZ threshold, indicating low forecast skill for intense reflectivity cores. It can be seen from e that 4DEnVar had a slightly high initial BIAS for both the 25- and 35-dBZ thresholds, although the scores diminished to around 1.0 for the final three hours of the forecast. Generally, 3DVar (3DEnVar) produced large underestimation (overestimation) in the final (initial) three hours of the forecast. Although 4DEnVar had high BIAS in the initial two hours, it generally outperformed both 3DVar and 3DEnVar for the 45-dBZ threshold (). The high biases in the initial two hours in 4DEnVar may be likely caused by the overpredicted convection in the early part of the forecasts.
Figure 5. FSS (a–c) and BIAS (d–f) of the 3DVar (dashed gray curve), 3DEnVar (solid gray curve) and 4DEnVar (solid black curve) for reflectivity thresholds of 25, 35 and 45 dBZ. The x-axis is the time starting at 0900 UTC 14 June 2009.

The spatial distributions of forecast radar reflectivity at 1000 UTC 14 June 2009 as well as the observations are presented in . Observations indicated a strong and long squall line over Anhui and Jiangsu provinces that comprised a leading convective line and trailing stratiform precipitation. These features were captured poorly by 3DVar, which failed to distinguish the convective line over Anhui Province. It also underestimated the intensity of the observed intense convection and overestimated the extent of the moderate convection over Jiangsu Province. Although the general shape of the convection system over Anhui and Jiangsu provinces in 3DEnVar presents a reasonable match with the observations, the area of convection with reflectivity of 30–45 dBZ was overpredicted considerably. The leading convective line ahead of the stratiform region was not as well organized as in the observations. There were also some spurious areas of convection in northern Henan and southeastern Shandong provinces in both 3DVar and 3DEnVar. In comparison, 4DEnVar presented an improved forecast with reduced spurious convection in southeastern Shandong Province, as well as a more realistic representation of the leading convective line and trailing stratiform precipitation. To reveal the vertical structure of reflectivity, cross sections along line AB in of the observed and forecast reflectivities from the three experiments at the same time are shown in . In the region X = 200–300 km, Y = 0–15 km, it can be seen that a strong convective region with maximum reflectivity of 57 dBZ was captured well by 4DEnVar. In comparison, the same convective region from 3DVar and 3DEnVar was much weaker. In addition, a region of moderate convection, corresponding to the stratiform precipitation observed at 0–8 km height, was captured by both 3DEnVar and 4DEnVar but not by 3DVar.
Figure 6. Radar reflectivity (unit: dBZ) from observation (a), 3DVar (b), 3DEnVar (c) and 4DEnVar (d) at 1000 UTC 14 June 2009.

Figure 7. Vertical cross sections of the radar reflectivity (unit: dBZ) along line AB in from observation (a), 3DVar (b), 3DEnVar (c) and 4DEnVar (d) at 1000 UTC 14 June 2009.

The observed squall line became fully developed and reached maturity at 1100 UTC. A bow-shaped convective line formed and some new convective cells were produced continuously over Henan, Anhui, and Shandong provinces (). The forecast from 3DVar was similarly poor as that of the previous forecast time. The bow-shaped convective line was almost missing, and the convection over Henan and Jiangsu provinces was too weak. Comparatively, 3DEnVar showed improvement over 3DVar by producing more convection over Anhui and Henan provinces. Although the squall line was broader than observed, the bow-shaped convective line and the new convective cells generated behind the original were reproduced successfully in 4DEnVar. Furthermore, it captured accurately the two areas of strong convection over Henan Province. The corresponding vertical cross sections of reflectivity are shown in . In the region X = 50–200 km, Y = 0–12 km, 3DVar was unable to forecast the moderate convection (30–45 dBZ), consistent with the pattern shown in . However, this was improved by both 3DEnVar and 4DEnVar but with larger underestimation by the former. The observed region of intense convection at 200–300 km in the horizontal axis, which coincided with the convective line, was clearly identified in 4DEnVar, whereas it was underestimated by both 3DVar and 3DEnVar. At 1300 UTC, the squall line began to weaken and the direction of the convective line was changed in comparison with that of the previous hour, indicating the beginning of its dissipating stage. However, the convective system behind the squall line had strengthened by this stage (). Compared with both 3DVar and 3DEnVar, 4DEnVar produced an improved leading convective line, as well as a stronger convective system over the provinces of Henan and Anhui, which were closer to the observations.
5.1.3. Precipitation, wind, temperature, and humidity forecasts
Precipitation is another important variable in convective forecasting. We further compared the forecast results against 1-h accumulated precipitation for three thresholds to verify the quantitative precipitation forecast skill using the quantitative precipitation estimated product (Shen et al., Citation2014). shows that 4DEnVar performed best at the 1-mm threshold, i.e., the FSS with an initial score of around 0.78 decreased to approximately 0.60 in the final forecast hour. Overall, 3DVar had the lowest FSS, averaging around 0.10 (0.20) less than 3DEnVar (4DEnVar). When the precipitation threshold was increased, all experiments exhibited lower FSSs, and the lowest value, which was approximately 0.20, was produced by 3DVar for the 5.0-mm threshold for the final three hours. The FSSs for 3DEnVar were markedly higher than for 3DVar, especially for the 2.5-mm threshold in the third hour. Overall, 4DEnVar produced the highest FSSs of all the experiments throughout the forecast period. As shown in , the BIAS values of 4DEnVar were between those of 3DVar and 3DEnVar, and they were closest to 1.0 for the 1.0- and 2.5-mm thresholds. For the 5.0-mm threshold, both 3DVar and 3DEnVar underestimated the precipitation, especially the former. This underestimation was reduced substantially in 4DEnVar, and the BIAS values were close to 1.0 between the second and fourth hours.
Figure 11. FSSs (a–c) and BIAS (d–f) of the 3DVar (dashed gray curve), 3DEnVar (solid gray curve) and 4DEnVar (solid black curve) for precipitation threshold of 1.0, 2.5 and 5 mm. The x-axis is the time starting at 0900 UTC 14 June 2009.

The improvements in 4DEnVar throughout the forecast period were also seen in terms of the spatial distribution of the forecast 6-h accumulated precipitation. Two strong precipitation bands were observed over Henan, Anhui, and Jiangsu provinces (). In 3DVar, only some isolated precipitation cores were simulated over Henan Province, and the intensity of the precipitation band located over Anhui and Jiangsu provinces was underestimated. In addition, some precipitation in eastern Anhui Province was missed. The underprediction is confirmed by the low BIAS values shown in . Although 3DEnVar captured the precipitation centers, it underestimated the heavy precipitation >12.8 mm (>25.6 mm) over the border of Henan (Jiangsu) and Anhui provinces. Overall, 4DEnVar produced the best forecast of the major precipitation band in terms of both intensity and location, except for a slightly larger spatial extent.
Figure 12. Six-hour accumulated precipitation (unit: mm) from observation (a), 3DVar (b), 3DEnVar (c) and 4DEnVar (d) initial at 0900 UTC 14 June 2009.

The forecast horizontal wind components (), temperature (
) and water vapor field (
) were also verified against sounding observations in the inner domain. shows the averaged biases (observation minus forecast) of
and
forecasts at different height levels. For
4DEnVar outperformed both 3DVar and 3DEnVar, with biases reduction by 0.8–1.5 m s−1 at low and middle levels. For
4DEnVar was slightly better than 3DEnVar at most levels, while 3DVar was poorer than 4DEnVar at most levels, especially for ∼900 hPa and ∼300 hPa. The
biases from 3DEnVar (3DVar) were significantly reduced by 4DEnVar below 850 hPa, where their bias differences could be ∼0.4 °C (1.0 °C). The reduction of biases for
was greater than other variables. The biases of 3DEnVar were generally between 3DVar and 4DEnVar and were closer to 4DEnVar above 500 hPa. Overall, 4DEnVar gave the smallest biases on average for wind, temperature and water vapor at most levels.
5.2. 4DEnVar Sensitivity experiments
The assimilation window length that determines the number of radar observations to influence the analysis may have significant impact on the performance of 4DEnVar. To investigate the sensitivity of 4DEnVar to the length of the assimilation window, the three experiments (i.e., 4DEnVar_L20, 4DEnVar_L30, and 4DEnVar_L40) were conducted using the same ensemble weighting factor of 75% but for which the assimilation window was increased from 20 to 30 and 40 min, respectively. Most ensemble variontional DA approaches were sensitive to the relative weight of ensemble covariance and static covariance (Gao and Stensrud, Citation2014). Therefore, we also performed three experiments with an ensemble weighing factor of 25% (4DEnVar_W25), 50% (4DEnVar_W50), and 75% (4DEnVar_W75) with the 30-min assimilation window (see ).
The averaged FSSs and BIAS values for radar reflectivity from the first three experiments are compared in . When the window length was increased or decreased from 30 min, the performance of 4DEnVar was worse for all thresholds throughout the forecast hours. Specifically, 4DEnVar_L30 showed consistently higher FSSs than 4DEnVar_L40, especially for the 45-dBZ threshold, whereas 4DEnVar_L20 produced a slightly degraded FSS in comparison with 4DEnVar_L40. The averaged BIAS values from 4DEnVar_L30 were smaller than from both 4DEnVar_L20 and 4DEnVar_L40 for the 25- and 35-dBZ thresholds, and the differences between 4DEnVar_L30 and 4DEnVar_L20 were greater than between 4DEnVar_L30 and 4DEnVar_L40. For the 45-dBZ threshold, 4DEnVar_L30 occasionally underperformed slightly in comparison with 4DEnVar_L20 and 4DEnVar_L40; however, for most hours it performed better with BIAS values close to 1.0. It is evident that 4DEnVar benefited most with a moderate assimilation window length, which allows a reasonable number of radar observations to influence analysis.
Figure 14. FSS (a–c) and BIAS (d–f) of the 4DEnVar_L20 (dashed gray curve), 4DEnVar_L30 (solid black curve) and 4DEnVar_L40 (solid gray curve) for reflectivity thresholds of 25, 35 and 45 dBZ. The x-axis is the time starting at 0900 UTC 14 June 2009.

The averaged FSSs and BIAS values at different thresholds for these three experiments (i.e., 4DEnVar_W25, 4DEnVar_W50, and 4DEnVar_W75) are shown in . It can be seen that the FSSs of 4DEnVar_W75 were generally the highest among all the 4DEnVar experiments, whereas the FSSs of 4DEnVar_W25 were the lowest and worse than those of 4DEnVar_W50 (). It was also found that the three experiments with larger ensemble weighting had larger BIAS values that were closer to 1.0, as illustrated in . For the 25- and 35-dBZ thresholds, the BIAS values in all experiments decreased gradually, although those of 4DEnVar_W75 indicated better results in most forecast hours. For the 45-dBZ threshold, although there was significantly overestimation in all experiments in the first forecast hour, the BIAS values of 4DEnVar_W75 were closest to 1.0 in the final three forecast hours. The BIAS values from 4DEnVar_W50 also showed some improvement over 4DEnVar_W25 for all thresholds in all forecast hours, except the first hour. It is shown that better performances were obtained by the 4DEnVar experiment with a larger ensemble weighting, indicating the advantage of incorporating flow-dependent ensemble covariance into the variational analysis.
5.3. The MCS case and local convection case
The analyses presented in the previous section demonstrated the improved forecast skill of the 4DEnVar algorithm relative to 3DVar and 3DEnVar. To verify whether the improvements found in relation to the original single case are generalized, analyses of two further convective cases that occurred over southeastern China were conducted using the same configurations. The MCS case formed at 0500 UTC 5 June 2009, moved eastward, and then dissipated around 1500 UTC 5 June 2009. The local convection case began at 0000 UTC 23 June 2013. It developed at 0230 UTC, and then weakened at 1400 UTC on 23 June 2013. For these cases, a 6-h forecast was conducted after a 3-h continuous radar DA at a 1-h interval for the three experiments.
shows the averaged FSSs and BIAS values for each experiment for the two cases using 25, 35, and 45 dBZ reflectivity thresholds. It can be seen that 3DEnVar showed consistently higher FSSs than 3DVar and that further improvements were obtained by 4DEnVar for all thresholds for the entire period of forecast. Although the BIAS values for both 3DEnVar and 4DEnVar were above the optimal value of 1.0 for the 25- and 35-dBZ thresholds in the initial two forecast hours, the lower values of 4DEnVar suggest that 4DEnVar exhibited substantially less overestimation than 3DEnVar. For the highest threshold, 4DEnVar had the highest BIAS value that was close to 1.0, whereas 3DEnVar produced substantial underestimation in the final four forecast hours. In contrast, the BIAS values of 3DVar were below the optimal value of approximately 1.0 for all thresholds throughout much of the forecast period, except for the first forecast hour.
6. Conclusions and discussion
In this study, a hybrid 4DEnVar DA system within the WRF model was developed and applied to convective-scale assimilation of radar data. The algorithm uses ensemble covariances at multiple time levels to estimate the background error evolution within the assimilation window and thus the distributed multiple observations are assimilated. Three experiments were performed to investigate the impact of assimilating radar observations using the 4DEnVar method on the analysis and forecast results relative to the 3DEnVar and 3DVar approaches. The system was applied first to the case of a squall line that occurred over southeastern China on 14 June 2009, and then it was applied to an MCS case and a local convection case to obtain conclusions that could be considered more reliable.
The analysis indicated that 4DEnVar outperformed 3DVar based on average RMSI statistics for both reflectivity and radial velocity. Moreover, 4DEnVar was also slightly better than 3DEnVar, especially for the second assimilation cycle. The vertical structure of reflectivity from 4DEnVar was also better analyzed, and the corresponding relative humidity (updraft) was larger (stronger) than in the other experiments, which better supported the convective system development than other methods. The improved convective-scale initial conditions of 4DEnVar led to better forecast results. The quantitative forecast reflectivity was shown improved significantly in terms of both the FSS and the BIAS for the three thresholds of 25, 30, and 45 dBZ. It was found that 4DEnVar reproduced the observed squall line structure in both horizontal and vertical directions more accurately than the other methods, especially for the leading convective line in comparison with 3DVar. In contrast, 3DEnVar showed modest improvement over 3DVar for all thresholds and all forecast hours, but not nearly as marked as that of 4DEnVar. It underestimated the intensity of strong convection but overestimated the areal extent of the moderate convection of 30–45 dBZ. The positive impact of the hybrid method could also be seen in the forecast skill for 1.0-, 2.5-, and 5.0-mm precipitation thresholds, with those of 4DEnVar producing the largest FSSs and BIAS values closest to 1.0. It was found that 4DEnVar successfully predicted the observed 6-h accumulated precipitation pattern, particularly for heavy precipitation, whereas that of 3DEnVar and 3DVar was weaker and smaller. Verification against sounding observations in the inner domain indicated that 4DEnVar outperformed the 3DEnVar and 3DVar for all standard variables at all levels, and the bias reduction for water vapor was the largest.
The effects of the length of the assimilation window and the ensemble covariance weighting factor adopted in the 4DEnVar system were examined through several sensitivity experiments. Assimilation window lengths of 20, 30, and 40 min were tested for assimilating radar observations. The 30-min experiment was found to produce the best forecast skill. It was also found that the 4DEnVar approach with ensemble weighting of 75% performed best when a 50-member ensemble was used. Moreover, the 4DEnVar technique was applied to an MCS case and a local convection case, and the forecast results confirmed the effective improvement. Despite these encouraging results, the 4DEnVar radar DA system that we have established still has scope for further improvement. It is worth noting that the forecast reflectivity and precipitation were overestimated in the early part of the forecasts. More efforts are needed in the future to reduce the excessive moisture caused by evaporation below the lifting condensation level with both stratiform and convective clouds to improve the forecast results. Adding additional observations (e.g., satellite and conventional data) to reduce the mesoscale and large-scale errors, and provide a more accurate synoptic-scale environment for the convective forecasting will be considered. In addition, tests on dual-resolution will be conducted in the future to reduce computational costs.
Disclosure statement
No potential conflict of interest was reported by the authors.
Additional information
Funding
References
- Bishop, C. H. and Hodyss, D. 2011. Adaptive ensemble covariance localization in ensemble 4D-VAR state estimation. Mon. Weather Rev 139, 1241–1255. doi:https://doi.org/10.1175/2010MWR3403.1
- Bowler, N. E., Flowerdew, J. and Pring, S. R. 2013. Tests of different flavours of EnKF on a simple model. Q. J. R. Meteorol. Soc. 139, 1505–1519. doi:https://doi.org/10.1002/qj.2055
- Brewster, K. A., Hu, M., Xue, M. and Gao, J. 2005. Efficient assimilation of radar data at high resolution for short range numerical weather prediction. In: World Weather Res. Progr. Symp. Nowcasting Very Short-Range Forecast (CDROM 3.06). Toulouse, France: World Meteorological Organization.
- Buehner, M. 2005. Ensemble-derived stationary and flow-dependent background-error covariances: Evaluation in a quasi-operational NWP setting. Q J. R Meteorol. Soc. 131, 1013–1043. doi:https://doi.org/10.1256/qj.04.15
- Buehner, M., Houtekamer, P. L., Charette, C., Mitchell, H. L. and He, B. 2010a. Intercomparison of variational data assimilation and the ensemble Kalman filter for global deterministic NWP. Part I: Description and single-observation experiments. Mon. Weather Rev. 138, 1550–1566. doi:https://doi.org/10.1175/2009MWR3157.1
- Buehner, M., Houtekamer, P. L., Charette, C., Mitchell, H. L. and He, B. 2010b. Intercomparison of variational data assimilation and the ensemble Kalman filter for global deterministic NWP. Part II: One-Month experiments with real observations. Mon. Weather Rev. 138, 1567–1586. doi:https://doi.org/10.1175/2009MWR3158.1
- Clark, A. J., Weiss, S. J., Kain, J. S., Jirak, I. L., Coniglio, M. and co-authors. 2012. An overview of the 2010 Hazardous Weather Testbed experimental forecast program spring experiment. Bull. Amer. Meteor. Soc. 93, 55–74. doi:https://doi.org/10.1175/BAMS-D-11-00040.1
- Dowell, D. C., Zhang, F., Wicker, L. J., Snyder, C. and Crook, N. A. 2004. Wind and Temperature retrievals in the 17 May 1981 Arcadia, Oklahoma, supercell: Ensemble Kalman filter experiments. Mon. Wea. Rev. 132, 1982–2005. doi:https://doi.org/10.1175/1520-0493(2004)132<1982:WATRIT>2.0.CO;2
- Gao, J. and Stensrud, D. J. 2014. Some observing system simulation experiments with a hybrid 3DEnVAR system for storm-scale radar data assimilation. Mon. Wea. Rev. 142, 3326–3346. doi:https://doi.org/10.1175/MWR-D-14-00025.1
- Gao, J., Fu, C., Stensrud, D. J. and Kain, J. S. 2016. OSSEs for an ensemble 3DVAR data assimilation system with radar observations of convective storms. J. Atmos. Sci. 73, 2403–2426. doi:https://doi.org/10.1175/JAS-D-15-0311.1
- Gao, J., Xue, M., Brewster, K. and Droegemeier, K. K. 2004. A three-dimensional variational data analysis method with recursive filter for Doppler radars. J. Atmos. Oceanic Technol. 21, 457–469. doi:https://doi.org/10.1175/1520-0426(2004)021<0457:ATVDAM>2.0.CO;2
- Gao, J., Xue, M. and Stensrud, D. J. 2013. The development of a hybrid EnKF-3DVAR algorithm for storm-scale data assimilation. Adv. Meteorol. 2013, 1–12. doi:https://doi.org/10.1155/2013/512656
- Gao, S., Sun, J., Min, J., Zhang, Y. and Ying, Z. 2018. A scheme to assimilate “no rain” observations from Doppler radar. Weather Forecast 33, 71–88. doi:https://doi.org/10.1175/WAF-D-17-0108.1
- Hamill, T. M. and Snyder, C. 2000. A hybrid ensemble Kalman filter-3D variational analysis scheme. Mon. Wea. Rev. 128, 2905–2919. doi:https://doi.org/10.1175/1520-0493(2000)128<2905:AHEKFV>2.0.CO;2
- Hong, S. Y., Noh, Y. and Dudhia, J. 2006. A new vertical diffusion package with an explicit treatment of entrainment processes. Mon. Weather Rev. 134, 2318–2341. doi:https://doi.org/10.1175/MWR3199.1
- Hu, M. and Xue, M. 2007. Impact of configurations of rapid intermittent assimilation of WSR-88D radar data for the 8 May 2003 Oklahoma City tornadic thunderstorm case. Mon. Weather Rev. 135, 507–525. doi:https://doi.org/10.1175/MWR3313.1
- Hu, M., Xue, M., Gao, J. and Brewster, K. 2006. 3DVAR and cloud analysis with WSR-88D level-II data for the prediction of the Fort Worth, Texas, tornadic thunderstorms. Part II: Impact of radial velocity analysis via 3DVAR. Mon. Weather Rev. 134, 699–721. doi:https://doi.org/10.1175/MWR3093.1
- Kleist, D. T., Parrish, D. F., Derber, J. C., Treadon, R., Wu, W. S. and co-authors. 2009. Introduction of the GSI into the NCEP global data assimilation system. Weather Forecast 24, 1691–1705. doi:https://doi.org/10.1175/2009WAF2222201.1
- Kong, R., Xue, M. and Liu, C. 2018. Development of a hybrid En3DVar data assimilation system and comparisons with 3DVar and EnKF for radar data assimilation with observing system simulation experiments. Mon. Weather Rev. 146, 175–198. doi:https://doi.org/10.1175/MWR-D-17-0164.1
- Lorenc, A. C. 2003. The potential of the ensemble Kalman filter for NWP–A comparison with 4D-Var. Q. J. R. Meteorol. Soc. 129, 3183–3203. doi:https://doi.org/10.1256/qj.02.132
- Lorenc, A. C., Bowler, N. E., Clayton, A. M., Pring, S. R. and Fairbairn, D. 2015. Comparison of hybrid-4DEnVar and hybrid-4DVar data assimilation methods for global NWP. Mon. Weather Rev. 143, 212–229. doi:https://doi.org/10.1175/MWR-D-14-00195.1
- Mittermaier, M. and Roberts, N. 2010. Intercomparison of spatial forecast verification methods: Identifying skillful spatial scales using the fractions skill score. Weather Forecast 25, 343–354. doi:https://doi.org/10.1175/2009WAF2222260.1
- Pan, Y., Zhu, K., Xue, M., Wang, X., Hu, M. and co-authors. 2014. A GSI-based coupled EnSRF-En3DVar hybrid data assimilation system for the operational rapid refresh model: Tests at a reduced resolution. Mon. Weather Rev. 142, 3756–3780. doi:https://doi.org/10.1175/MWR-D-13-00242.1
- Parrish, D. F. and Derber, J. C. 1992. The National Meteorological Center's spectral statistical-interpolation analysis system. Mon. Wea. Rev. 120, 1747–−1763. doi:https://doi.org/10.1175/1520-0493(1992)120<1747:TNMCSS>2.0.CO;2
- Putnam, B. J., Xue, M., Jung, Y., Snook, N. and Zhang, G. 2014. The analysis and prediction of microphysical states and polarimetric radar variables in a mesoscale convective system using double-moment microphysics, multinetwork radar data, and the ensemble Kalman filter. Mon. Weather Rev. 142, 141–162. doi:https://doi.org/10.1175/MWR-D-13-00042.1
- Roberts, N. M. and Lean, H. W. 2008. Scale-selective verification of rainfall accumulations from high-resolution forecasts of convective events. Mon. Weather Rev. 136, 78–97. doi:https://doi.org/10.1175/2007MWR2123.1
- Schenkman, A. D., Xue, M., Shapiro, A., Brewster, K. and Gao, J. 2011. Impact of CASA radar and Oklahoma Mesonet data assimilation on the analysis and prediction of tornadic mesovortices in an MCS. Mon. Weather Rev. 139, 3422–3445. doi:https://doi.org/10.1175/MWR-D-10-05051.1
- Shen, Y., Zhao, P., Pan, Y. and Yu, J. 2014. A high spatiotemporal gauge-satellite merged precipitation analysis over China. J. Geophys. Res. Atmos. 119, 3063–3075. doi:https://doi.org/10.1002/2013JD020686
- Smirnova, T. G., Brown, J. M. and Benjamin, S. G. 1997. Performance of different soil model configurations in simulating ground surface temperature and surface fluxes. Mon. Wea. Rev. 125, 1870–1884. doi:https://doi.org/10.1175/1520-0493(1997)125<1870:PODSMC>2.0.CO;2
- Snyder, C. and Zhang, F. 2003. Assimilation of simulated Doppler radar observations with an ensemble Kalman filter. Mon. Weather Rev. 131, 1663–1677. doi:https://doi.org/10.1175//2555.1
- Sun, J. and Crook, N. A. 1997. Dynamical and microphysical retrieval from Doppler radar observations using a cloud model and its adjoint. Part I: Model development and simulated data experiments. J. Atmos. Sci. 54, 1642–1661. doi:https://doi.org/10.1175/1520-0469(1997)054<1642:DAMRFD>2.0.CO;2
- Sun, J. and Crook, N. A. 1998. Dynamical and microphysical retrieval from Doppler radar observations using a cloud model and its adjoint. Part II: Retrieval experiments of an observed Florida convective storm. J. Atmos. Sci. 55, 835–852. doi:https://doi.org/10.1175/1520-0469(1998)055<0835:DAMRFD>2.0.CO;2
- Sun, J. and Wang, H. 2013. Radar data assimilation with WRF 4D-Var. Part II: Comparison with 3D-Var for a squall line over the U.S. great plains. Mon. Weather Rev. 141, 2245–2264. doi:https://doi.org/10.1175/MWR-D-12-00169.1
- Sun, J., Wang, H., Tong, W., Zhang, Y., Lin, C.-Y. and co-authors. 2016. Comparison of the Impacts of momentum control variables on high-Resolution variational data assimilation and precipitation forecasting. Mon. Weather Rev. 144, 149–169. doi:https://doi.org/10.1175/MWR-D-14-00205.1
- Sun, J., Xue, M., Wilson, J. W., Zawadzki, I., Ballard, S. P. and co-authors. 2014. Use of NWP for nowcasting convective precipitation: Recent progress and challenges. Bull. Am. Meteorol. Soc. 95, 409–426. doi:https://doi.org/10.1175/BAMS-D-11-00263.1
- Thompson, G., Field, P. R., Rasmussen, R. M. and Hall, W. D. 2008. Explicit forecasts of winter precipitation using an improved bulk microphysics scheme. Part II: Implementation of a new snow parameterization. Mon. Weather Rev. 136, 5095–5115. doi:https://doi.org/10.1175/2008MWR2387.1
- Tong, M. and Xue, M. 2005. Ensemble Kalman filter assimilation of Doppler radar data with a compressible nonhydrostatic model: OSS experiments. Mon. Weather Rev. 133, 1789–1807. doi:https://doi.org/10.1175/MWR2898.1
- Wang, H., Sun, J., Fan, S. and Huang, X. Y. 2013b. Indirect assimilation of radar reflectivity with WRF 3D-Var and its impact on prediction of four summertime convective events. J. Appl. Meteorol. Climatol. 52, 889–902. doi:https://doi.org/10.1175/JAMC-D-12-0120.1
- Wang, H., Sun, J., Zhang, X., Huang, X. Y. and Auligné, T. 2013a. Radar Data assimilation with WRF 4D-Var. Part I: System development and preliminary testing. Mon. Weather Rev. 141, 2224–2244. doi:https://doi.org/10.1175/MWR-D-12-00168.1
- Wang, X., Barker, D. M., Snyder, C. and Hamill, T. M. 2008a. A hybrid ETKF-3DVAR data assimilation scheme for the WRF model. Part I: Observation system simulation experiment. Mon. Weather Rev. 136, 5116–5131. doi:https://doi.org/10.1175/2008MWR2444.1
- Wang, X., Barker, D. M., Snyder, C. and Hamill, T. M. 2008b. A hybrid ETKF-3DVAR data assimilation scheme for the WRF model. Part II: Real observing experiments. Mon. Weather Rev. 136, 5132–5147. doi:https://doi.org/10.1175/2008MWR2445.1
- Wang, X. and Lei, T. 2014. GSI-based four-dimensional ensemble–variational (4DEnsVar) data assimilation: Formulation and single-resolution experiments with real data for NCEP global forecast system. Mon. Wea. Rev. 142, 3303–3325. doi:https://doi.org/10.1175/MWR-D-13-00303.1
- Wang, X., Hamill, T. M., Whitaker, J. S. and Bishop, C. H. 2009. A comparison of the hybrid and EnSRF analysis schemes in the presence of model errors due to unresolved scales. Mon. Weather Rev. 137, 3219–3232. doi:https://doi.org/10.1175/2009MWR2923.1
- Wang, Y. and Wang, X. 2017. Direct assimilation of radar reflectivity without tangent linear and adjoint of the nonlinear observation operator in the GSI-based EnVar system: Methodology and experiment with the 8 May 2003 Oklahoma City tornadic supercell. Mon. Weather Rev. 145, 1447–1471. doi:https://doi.org/10.1175/MWR-D-16-0231.1
- Wang, Y., Gao, J., Skinner, P. S., Knopfmeier, K., Jones, T. and co-authors. 2019. Test of a weather-adaptive dual-resolution hybrid warn-on-forecast analysis and forecast system for several severe weather events. Weather Forecast 34, 1807–1827. doi:https://doi.org/10.1175/WAF-D-19-0071.1
- Wu, W. S., Parrish, D. F., Rogers, E. and Lin, Y. 2017. Regional ensemble–variational data assimilation using global ensemble forecasts. Weather Forecast 32, 83–96. doi:https://doi.org/10.1175/WAF-D-16-0045.1
- Xiao, Q., Kuo, Y. H., Sun, J., Lee, W. C., Lim, E. and co-authors. 2005. Assimilation of Doppler radar observations with a regional 3DVAR system: Impact of Doppler velocities on forecasts of a heavy rainfall case. J. Appl. Meteorol. 44, 768–788. doi:https://doi.org/10.1175/JAM2248.1
- Xiao, Q., Lim, E., Won, D. J., Sun, J., Lee, W. C. and co-authors. 2008. Doppler radar data assimilation in KMS’s operational forecasting. Bull. Am. Meteorol. Soc. 89, 39–44. doi:https://doi.org/10.1175/BAMS-89-1-39
- Xie, Y., Koch, S., McGinley, J., Albers, S., Bieringer, P. E. and co-authors. 2011. A space-time multiscale analysis system: A sequential variational analysis approach. Mon. Weather Rev. 139, 1224–1240. doi:https://doi.org/10.1175/2010MWR3338.1
- Xue, M., Tong, M. and Droegemeier, K. K. 2006. An OSSE framework based on the ensemble square root Kalman filter for evaluating the impact of data from radar networks on thunderstorm analysis and forecasting. J. Atmos. Ocean. Technol. 23, 46–66. doi:https://doi.org/10.1175/JTECH1835.1
- Zhang, F., Zhang, M. and Poterjoy, J. 2013. E3DVar: Coupling an ensemble Kalman filter with three-dimensional variational data assimilation in a limited-area weather prediction model and comparison to E4DVar. Mon. Weather Rev. 141, 900–917. doi:https://doi.org/10.1175/MWR-D-12-00075.1