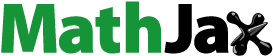
ABSTRACT
The aim of this study was to investigate the effects of different recovery power outputs on the reconstitution of W′ and to develop a dynamic bi-exponential model of W′ during depletion and reconstitution. Ten trained cyclists (mass 71.7 ± 8.4 kg; V̇O2max 60.0 ± 6.3 ml·kg−1·min−1) completed three incremental ramps (20 W·min−1) to the limit of tolerance on each of six occasions with recovery durations of 30 and 240 s. Recovery power outputs varied between 50 W (LOW); 60% of critical power (CP) (MOD) and 85% of CP (HVY). W′ reconstitution was measured following each recovery and fitted to a bi-exponential model. Amplitude and time constant (τ) parameters were then determined via regression analysis accounting for relative intensity and duration to produce a dynamic model of W′. W′ reconstitution slowed disproportionately as recovery power output increased (p < 0.001) and increased with recovery duration (p < 0.001). The amplitudes of each recovery component were strongly correlated to W′ reconstitution after 240 s at HVY (r = 0.95), whilst τ parameters were found to be related to the fractional difference between recovery power and CP. The predictive capacity of the resultant model was assessed against experimental data with no differences found between predicted and experimental values of W′ reconstitution (p > 0.05). The dynamic bi-exponential model of W′ accounting for varying recovery intensities closely described W′ kinetics in trained cyclists facilitating real-time decisions about pacing and tactics during competition. The model can be customised for individuals from known CP and W′ and a single additional test session.
Highlights
A dynamic bi-exponential model of W′ accounting for both varying power output and duration.
Individual customisation of the model can be achieved with a single specific test session.
W′ reconstitution slows disproportionally with increasing intensity after repeated bouts.
KEYWORDS:
Introduction
Cycle races are physically demanding events, yet cyclists’ physiological abilities alone are seldom enough to facilitate victory. Accordingly, race tactics are a crucial component in “mass start” races where decisive moves may be characterised by repeated short high-intensity efforts preceding a sustained high-intensity effort (Abbiss et al., Citation2013), or other stochastic races such as criteriums where short lapped circuits with technical corners elicit repeated high-intensity efforts to remain in contention (Etxebarria et al., Citation2019). Such high-intensity efforts are typically within the severe exercise intensity domain, that is above the threshold at which a metabolic steady state can be maintained (Poole et al., Citation2016). Critical power (CP) estimates the threshold separating the severe exercise domain from the lower heavy domain, where a metabolic steady state is achievable (Jones et al., Citation2019), whereas W′ reflects a finite volume of work available above CP. Together these parameters form the 2-parameter CP model (Monod & Scherrer, Citation1965) which can describe performance within the severe intensity domain. However, this model does not account for the reconstitution of W′ which is assumed to occur when power falls below CP (Chidnok et al., Citation2013). Hence, there is a need for an intermittent model that can account for continuously varying expenditure and reconstitution of W′ and facilitate real-time monitoring of fatigue progression (Jones & Vanhatalo, Citation2017) to fashion race tactics.
W′ was initially considered to be an anaerobic work capacity reliant upon anaerobic glycolysis, stored glycogen, ATP and phosphocreatine (PCr) (Moritani et al., Citation1981), but has been shown to be at least partly dependent upon the accumulation of fatiguing metabolites such as inorganic phosphate, hydrogen ions and adenosine diphosphate (Chidnok et al., Citation2013; Johnson et al., Citation2014) alongside the depletion of substrates such as PCr (Chidnok et al., Citation2013). Moreover, since the precise physiological constituents of W′ are not fully understood, this necessitates that the modelling of W′ recovery is based on experimental observations of performance where W′ is repeatedly depleted and reconstituted. Using this approach, Skiba, Chidnok, Vanhatalo, Jones (Skiba et al., Citation2012; Skiba & Clarke, Citation2021) derived a mono-exponential model of W′ balance from an intermittent exercise protocol based on a 60/30 s (Equationequation 1(1)
(1) ), where W′bal = balance of W′; W′exp = W′ expended; t-u = recovery duration. The W′ recovery time constant (τ) was established as Equationequation 2
(2)
(2) using non-linear regression from the experimental group data, where. DCP = difference between CP and recovery power output.
(1)
(1)
(2)
(2) Whilst successfully validated against similar exercise protocols (Skiba et al., Citation2014; Skiba et al., Citation2014), it was found that τ differed markedly between participants, hence should be determined on an individual basis (Bartram et al., Citation2018; Caen et al., Citation2019; Chorley et al., Citation2019). The integration model was dependent upon the mean power output during recoveries being known, consequently W′ balance could only be resolved retrospectively. To address this problem, a differential form of the model was produced (Skiba et al., Citation2015), whereby the W′ balance could be evaluated dynamically by separating the expenditure and reconstitution phases (equations 3a-3b). Furthermore, the differential equation replaces the time constant with a variable derived from CP and W′ aiding its simplicity but removing the customisations previously recommended by fitting τ for the individual.
(3a)
(3a)
(3b)
(3b)
Both model forms were based on a mono-exponential reconstitution of W′ which was found to underestimate the observed W′ reconstitution over shorter time frames (≲2 min) (Caen et al., Citation2021; Chorley et al., Citation2019; Chorley et al., Citation2022) and overestimate W′ reconstitution over longer time frames ( 5 min) (Caen et al., Citation2021; Chorley et al., Citation2022). Subsequently, two independent studies investigated bi-exponential modelling of W′ reconstitution following full depletion (Caen et al., Citation2021; Chorley et al., Citation2022). Both found the two-component model an excellent fit to observed W′ reconstitution kinetics for durations ranging from 15 s to 15 min (Caen et al., Citation2021; Chorley et al., Citation2022). The two-component model may reflect different physiological mechanisms as the τ of the two components are unrelated within individuals (Chorley et al., Citation2022). For example, the fast component (FC) responsible for 30-40% of W′ reconstitution within the first 30 s when recovery power output is in the moderate intensity domain (Caen et al., Citation2021; Chorley et al., Citation2022) has a similar τ to PCr restoration (van den Broek et al., Citation2007). The bi-exponential reconstitution model (Equationequation 4
(3b)
(3b) ) has extra complexity compared to the mono-exponential models requiring the computation of the amplitude of the fast and slow components (SC) together with their respective τ values. Whilst these parameters were found to be highly variable (Caen et al., Citation2021; Chorley et al., Citation2022), individualised estimations for these parameters could be made from experimental trials comprising recovery durations of 30 and 240 s (equations 5a-5d) following full depletion of W′ (Chorley et al., Citation2022) (where W′30rec and W′240rec are W′ reconstitution after 30 and 240 s in the respective recovery periods).
(4)
(4)
(5a)
(5a)
(5b)
(5b)
(5c)
(5c)
(5d)
(5d)
(5e)
(5e)
Although the bi-exponential models provided a framework for W′ reconstitution kinetics, the models were only verified with recovery power outputs in the moderate intensity domain. It is known that reconstitution of W′ does not occur above CP (Chidnok et al., Citation2012), and that faster W′ reconstitution occurs with lower recovery intensities (Lievens et al., Citation2021; Skiba et al., Citation2012), but the influence of differing power outputs on the bi-exponential model parameters has yet to be quantified. As such, a functional bi-exponential model that can be applied within cycle sport remains elusive. Furthermore, a fatiguing effect has been observed following repeated bouts to exhaustion which manifests as a slowing of the reconstitution of W′ (Chorley et al., Citation2019; Chorley et al., Citation2022). Whilst PCr restoration has been linked to the fast phase of W′ reconsitution (Chorley et al., Citation2022), it has been shown to be inhibited by increased levels of hydrogen ions (van den Broek et al., Citation2007) which remain elevated after 4 mins of rest following severe exercise (Parolin et al., Citation1999), thus potentially impacting the slow phase of W′ reconstitution. Such a slowing of PCr restoration has been observed during intermittent severe intensity as the session progresses (Chidnok et al., Citation2013), but is not yet accounted for by current intermittent models of W′ reconstitution.
An individualised dynamic model of W′ could be used in real-time to influence race tactics by providing instantaneous feedback to athletes of their current balance of W′, allowing them to judge their ability to sustain severe intensity efforts. To do so would also require the minimisation of the test burden for athletes, such that the estimation of model parameters would be possible from a small number (ideally one) of additional test sessions. Therefore, the main aim of this study was to investigate the effects of varying recovery power outputs (of 30 and 240 s durations) on the parameters of the bi-exponential model of W′ reconstitution at intensities within the heavy and moderate intensity domains, and to produce a dynamic bi-exponential model of W′ reconstitution accounting for recovery power and duration. Secondary aims were to derive an individualised model estimation from a single test session and to quantify the fatiguing effects of repeated efforts on W′ reconstitution kinetics.
Methods
Participants
Ten trained cyclists (male = 9; female = 1; age: 43.6 ± 11.9 y; body mass 71.7 ± 8.4 kg; stature 176.3 ± 6.0 cm; body fat 18.3 ± 8.5%; V̇O2max 60.0 ± 6.3 ml·kg−1·min−1) volunteered to participate in this study and provided written informed consent. All participants were healthy, competitive amateur cyclists with a regular weekly training volume of 9.3 ± 3.0 h·week−1. Following institutional ethical approval, laboratory testing sessions were undertaken at the end of their racing seasons.
Experimental design
Within a 4-week period, participants undertook a baseline ramp all-out test (a) to determine CP and W′ followed by six sessions consisting of a repeated W′ depletion and reconstitution task (Chorley et al., Citation2019) with recovery durations fixed at 30 and 240 s and reversed in order at 240 s and 30 s. Recovery phases were completed at power outputs of 50 W (LOW); 60% of CP (MOD) and 85% of CP (HVY). Trial order was randomised and balanced such that each combination of recovery power output condition and recovery duration (30 and 240 s) was undertaken as both a first and second recovery period (b). Participants were asked to arrive 3 h post-prandial, having avoided strenuous exercise and alcohol in the preceding 24 h, and caffeine in the preceding 4 h. A minimum of 48 h was required between visits. Sessions were undertaken in an air-conditioned laboratory at similar times of day (± 1.5 h).
Figure 1. Testing protocol examples of (a) baseline test protocol to determine critical power following the expenditure of W′ and provide a familiarisation prior to (b) the experimental protocol with recovery phases varied in duration and intensity. W′ is equal to the area above CP (in Joules) during the initial ramp phase, and the amount of W′ reconstituted during the recovery phases is equal to the area above CP in the subsequent ramp phases.

All exercise sessions were performed seated on an electronically braked cycle ergometer (Lode Excalibur Sport, Lode BV, Groningen, Netherlands) fitted to each participant. Breath-by-breath pulmonary gas exchange was sampled throughout to determine O2 and CO2 concentrations and volumes using an on-line gas analyser (Quark CPET, Cosmed, Rome, Italy) calibrated with gases of known concentrations and volumes prior to each test. Strong verbal encouragement was provided throughout the tests. Changes in cycle ergometer test phases were automated with pre-programmed conditions.
Procedures
Baseline ramp all-out test
Participants were measured for stature, body mass and body composition (BodPod, Cosmed, Rome, Italy). Prior to the start of the baseline ramp all-out test, the ergometer’s linear mode resistance was set based on recent race and training data, and the participant’s preferred cadence, to allow for an accurate determination of CP. The test commenced with a warm-up at 100 W and after 5 min this transitioned to an incremental ramp at 20 W·min−1 to the limit of tolerance (deemed to be when cadence fell below 60 r·min−1). At this point power output was reduced to 30 W above predicted CP to utilise any remaining W′ (Chorley et al., Citation2019) until cadence fell below 50 r·min−1 and W′ was deemed expended. The ergometer then switched to linear mode (at a fixed resistance calculated for each participant to elicit their preferred cadence at their estimated CP) for two minutes of all-out cycling. Time to completion of this phase was withheld from participants to minimise the adoption of pacing. For familiarisation purposes only, the test continued with a 50 W recovery for two minutes at 85% of predicted CP, before commencing a second 20 W·min−1 ramp at CP +30W (to reduce errors associated with the intra-day variability of CP (Chorley et al., Citation2019), until cadence fell below 60 r·min−1 at which point power output was once again reduced to CP +30 W until cadence fell below 50 r·min−1 (a).
W′ depletion and reconstitution trials
The depletion and reconstitution trials comprised a repeated ramp test (b) to determine the amount of W′ reconstituted following 30 and 240 s of active recovery. Like the baseline test, these tests commenced with a 5 min warm-up at 100 W before transitioning to a smooth ramp at 20 W·min−1 to the limit of tolerance (as defined above), following which power output was reduced to CP +30 W (determined by the baseline test) until cadence fell below 50 r·min−1 and W′ was deemed to be expended. The first recovery period (duration of 30 s or 240 s) was then followed by a second smooth ramp starting at CP +30 W and a rate of 20 W·min−1 to the limit of tolerance and step-down to CP +30 W. A second recovery period of the alternate duration to the first (240 s or 30 s) was followed by a third and final ramp and step-down, again starting at CP +30 W.
Data processing
Errant breaths differing by ≥ 4 SD from the local mean were removed from the gas exchange data, which was interpolated and aligned with power output to produce second-by-second data using a custom Microsoft Excel (v. 2110) spreadsheet. CP was calculated as the mean power output of the last 30 s of the linear phase of the baseline test (Murgatroyd et al., Citation2014). Gas exchange threshold (GET) was determined using the V-slope method (Beaver et al., Citation1986) and corrected for the V̇O2 mean response time using data from the baseline test, and used to verify recovery intensities domains (Bergstrom et al., Citation2013) with the MOD intensity being below the GET, and HVY intensity being between GET and CP for each individual participant. Individual V̇O2max was calculated as the highest rolling 30-s average across all tests (Day et al., Citation2003). W′ was calculated as the mean work done above CP during the first ramp and step-down over the series of tests, whilst W′ reconstitution was the amount of work done above CP in the ramp and step-down phases following its respective recovery period, expressed as percentage of W′.
Model fitting
Bi-exponential model parameters (Equationequation 4(3b)
(3b) ) were fitted to the experimental data with OriginPro 2020b (Originlab Corp., Northampton, MA, USA). FCamp derived from equations 5a-5e was overestimated (see Results), consequently fitting was achieved for each participant’s data by sharing FCamp across LOW, MOD and HVY conditions for the first recovery period, τFC and τSC were individually fitted for each data set (Chorley et al., Citation2022). The sum of FCamp and SCamp was constrained to 100%, and to prevent non-physiological values τFC and τSC were bounded as ≥8 s and ≤5000 s, respectively (Jones & Vanhatalo, Citation2017; Skiba et al., Citation2012). Pearson’s product-moment correlations were used to assess potential predictors of model parameters from experimental values of W′ reconstitution, and FCamp was subsequently estimated by linear regression. Estimations of τFC and τSC were derived from non-linear least squares regression in relation to the fractional difference between recovery power and CP (%DCP) using the Solver add-in for Microsoft Excel and estimations of W′ following the second recovery period were derived from multiple linear regression in relation to the reconstitution following the first recovery period and %DCP using Microsoft Solver to match actual W′ reconstitution. Derived equations were retrofitted into the bi-exponential model framework (Equationequation 4
(3b)
(3b) ) for recovery and W′ depletion (Equationequation 3
(3a)
(3a) a) to produce a dynamic model for W′ balance.
Statistical analysis
Descriptive statistics (mean ± SD) were calculated for all dependent variables and checked for normality using the Shapiro–Wilk test. Three-way repeated measures ANOVA (recovery power output x recovery duration x recovery period) were used to assess differences in W′ reconstitution. Sphericity was checked with Mauchly’s test and accounted for where necessary using the Greenhouse-Geisser adjustment. A priori paired sample t-tests were used to compare differences in the means of W′ reconstitution at LOW, MOD and HVY within recovery periods, and at each corresponding intensity and duration between recovery periods. Effect sizes were calculated using Cohen’s d. Paired sample t-tests were used to test the veracity of the bi-exponential model parameters in estimating W′ reconstitution compared to actual W′ reconstitution. Statistical significance was set at p < 0.05. All statistical analyses were performed using SPSS v.27 (IBM Corp., Armonk, NY, US).
Results
Baseline participant measurements were CP 293 ± 37 W; W′ 11.0 ± 2.9 kJ; GET 223 ± 26 W; PPO 371 ± 40 W, of which the contribution to W′ from the CP + 30 W step down was 929 ± 667 J.
W′ reconstitution
The experimental procedures produced a range of W′ reconstitution ranging from 63.1 ± 15.2% to 20.6 ± 9.0% and are detailed in . Significant main effects of recovery power output (p < 0.001), recovery duration (p < 0.001) and recovery period (p = 0.03) were observed, with W′ reconstitution being higher at lower power outputs, after longer recovery durations, and within the first recovery period. Additionally, a significant (p = 0.001) recovery duration x power output interaction effect was evident, with the enhanced magnitude of W′ reconstitution at 240 s versus 30 s being greater at lower recovery power outputs, whilst an interaction between recovery period x duration approached significance (p = 0.06). Mean W′ reconstitution measurements and effect sizes of increasing recovery intensities are shown in . W′ reconstitution was reduced during the second 240 s recovery period at HVY when compared to the same duration and intensity during first recovery period (p < 0.01; ES = 1.3). No significant differences were found at other corresponding datapoints whilst W′ reconstitution of all corresponding time-points following the first and second recovery periods were highly correlated (r > 0.67; p < 0.04).
Table 1. Mean ± SD W′ reconstitution as a percentage of W′ (absolute values in parenthesis), after 30 and 240 s at LOW (50 W), MOD (60% of CP) and HVY (85% of CP) following both first and second recovery periods.
Model assessments
Bi-exponential model parameters calculated using equations 5a-5e were used to predict fractions of W′ reconstitution. Analysis revealed that the model performed better for the LOW recovery condition but as recovery intensity increased to the HVY condition, the model tended to overestimate W′ reconstitution (a).
Figure 2. (a) Actual versus predicted values of W′ reconstitution derived from the prediction equations for amplitude and time constants. MOD and HVY predictions of time constants maintained the amplitude values derived from LOW. (b) Actual versus predicted mean ± SD values of W′ reconstitution derived from the new prediction equations for FCamp and time constants. Solid bars are actual measurements, shaded columns are predicted values. * p < 0.05 indicating actual and predicted values are different.

Model fitting
Individual fitting demonstrated large inter-individual variations in the bi-exponential model parameters fitted to measured values (). The FCamp parameter was very strongly correlated to the fraction of W′ reconstitution after 240 s of recovery in the HVY condition (r = 0.949; p < 0.001), with linear regression yielding Equationequation 6(5a)
(5a) a. Non-linear regression equations for the parameter of τFC and τSC produced equations 6b and 6c, respectively.
(6a)
(6a)
(6b)
(6b)
(6c)
(6c)
Table 2. Individual fitted parameters of bi-exponential recovery to W′ reconstitution during the first recovery period.
Linear regression yielded Equationequation 7(5b)
(5b) which described the decline in W′ reconstitution following the second recovery period in comparison to that of the first recovery period.
(7)
(7) No differences were found in the individualised model fits using equations Equation6
(5a)
(5a) and Equation7
(5b)
(5b) and measured values of W′ reconstitution (b). During the first recovery period a maximum bias of 1.2% was found at 30 s of the MOD recovery condition, whilst the maximum bias of 3.7% in second recovery period was found at 240 s of the MOD recovery condition.
Discussion
By investigating the effects of different recovery power outputs on the reconstitution of W′ this study has yielded a new dynamic bi-exponential model of W′ accounting for both variable recovery power and duration, that can be individually parameterised from known CP and W′ and a single additional test session. Bi-exponential models of W′ reconstitution kinetics have proved to be a superior fit of W′ reconstitution kinetics when compared to mono-exponential models at moderate intensities (Caen et al., Citation2021; Chorley et al., Citation2022) following exhaustive exercise. The present findings demonstrate that increasing recovery intensity slows the W′ reconstitution kinetics concurring with previous experimental data and mono-exponential models (Morton & Billat, Citation2004; Skiba et al., Citation2012). W′ reconstitution was subject to the interaction effect between recovery duration and intensity which presented as a disproportionate slowing of W′ reconstitution over time, which was particularly evident in the HVY condition. Despite the clear effects of recovery intensity, duration and recovery period on W′ reconstitution, large variations in the experimental measurements demonstrated markedly different individual W′ reconstitution kinetics, corroborating findings previously reported in homogeneous groups (Bartram et al., Citation2018; Chorley et al., Citation2022) and necessitating the need for individual customisations of W′ reconstitution models.
The use of a bi-exponential model of W′ reconstitution was first proposed by Skiba, Chidnok, Vanhatalo, Jones (Skiba et al., Citation2012) and has since been shown to be an excellent fit when recovery periods have been undertaken at a low to moderate intensities (Caen et al., Citation2021; Chorley et al., Citation2022). The ensuing prediction equations based on 30 and 240 s recovery durations (Chorley et al., Citation2022) performed well against actual measurements in the present study within the moderate intensity domain but reconstitution of W′ was overestimated as recovery intensities increased (i.e. heavy domain) on account of the FCamp parameter being inflated due to the fitting at lower recovery intensities. Fitting of the FCamp parameter across the intensity range of the present study revealed a lower FCamp than estimated from the prediction equation (5a) which is based solely on a recovery intensity of 50 W. Moreover, the W′ reconstituted after 4 min at 85% of CP proved to be an excellent predictor (r = 0.95) of FCamp. It appears that the recovery in the heavy intensity domain permits a large portion of the fast component to be reconstituted after 4 min with only a small (≈ 5%) contribution of the slow component, thus isolating the FCamp parameter, possibly reflecting a near complete reconstitution of PCr stores and low levels of metabolite clearance. The subsequent non-linear regression equations of τFC and τSC reflect the continuous non-linear relationship with W′ reconstitution and recovery power output inherent within the mono-exponential models of W′ (Skiba et al., Citation2012; Skiba et al., Citation2014). It has been suggested that the non-linear reconstitution of W′ may be differentiated within intensity domains (Lievens et al., Citation2021) and may have a lower limit of power output beyond which no further speeding of W′ is achieved (Skiba et al., Citation2012), however the existence of such inflection points would require investigations including many more power outputs than employed in the studies to date.
Whilst the fitting of experimental data to determine the amplitude and time constants undoubtedly leads to the most accurate individual model, the number of tests required makes this impractical for cyclists, hence a compromise model is required balancing accuracy with practicality. The regression equations determined from the present study successfully accounted for power and duration when retrofit into the bi-exponential framework with no statistical biases between measured and estimated values (although these have yet to be validated in additional data sets). Anthropometric and physiological variables collated during this study, including those previously correlated with W′ reconstitution (Chorley et al., Citation2020) were tested against the experimental data but failed to improve the model fit, hence the individual fit of the equations is based on the single measurement of W′ reconstituted (240 s at 85% of CP) and %DCP. Whilst simply applying the mean experimental FCamp of 36% to the model may offer an alternative to undertaking a specific test session, the large inter-individual variations in FCamp (SD = 11.0%) suggests the prudence of incorporating the additional customisation. Whilst FCamp has previously been shown to be unrelated to any prior known anthropometric or physiological measurement (Chorley et al., Citation2022), the fractional difference between CP and recovery power was found to enable estimations of τFC and τSC, as was the case in the τ regression equation previously produced for mono-exponential models of W′ reconstitution (Bartram et al., Citation2018; Skiba et al., Citation2012).
Measures of aerobic fitness such as V̇O2max and CP have been linked to faster recovery of W′ (Caen et al., Citation2021; Chorley et al., Citation2022) explaining the inclusion of DCP within W′ reconstitution models. Indeed, Skiba, Chidnok, Vanhatalo, Jones (Skiba et al., Citation2012) suggested this was related to an oxidative reserve and the ability to regenerate PCr, which is itself an oxidative process (Haseler et al., Citation1999). There is strong evidence that PCr stores are an important contributor to W′ (Chidnok et al., Citation2013b; Jones et al., Citation2008) with its recovery being linked to the fast component of W′ reconstitution (Caen et al., Citation2021). It has been suggested that the recovery of PCr is itself a bi-exponential process (Harris et al., Citation1976; Nevill et al., Citation1997) with similar fast component τ values of 15 s following high intensity exercise (Forbes et al., Citation2009) to that observed in the present study. It is, however, unlikely, that the bi-exponential model even with the added complexity of the multi-component terms for τFC and τSC reflects the intricacies of the underlying physiology of W′ reconstitution. This physiology is thought to incorporate the hitherto unquantified interactions of fatiguing metabolites (Chidnok et al., Citation2013b), muscle fibre phenotype (Lievens et al., Citation2020), V̇O2 kinetics (Caen et al., Citation2021), and an element of central fatigue (Hureau et al., Citation2018) in addition to the replenishment of the high energy stores such as PCr.
The presented model of W′ reconstitution can be paired with a W′ depletion equation to provide a full dynamic model of W′. To account for bi-exponential reconstitution, however, depletion of W′ also be apportioned between the fast and slow components, hence an assumption is necessary that the depletion is concurrent and proportional to the amount of W′ remaining in each component, such that the full dynamic model in absolute terms can be given as equations 8a and 8b.
(8a)
(8a)
(8b)
(8b) And:
The slowing of W′ reconstitution following a repeated bout in the present study was similar to previous findings (Chorley et al., Citation2019), with little slowing of W′ reconstitution from the first to the second 30 s recoveries, whilst the slowing was more evident after the 240 s recoveries, suggesting different underlying physiological mechanisms between the fast and slow components. The present study extended those findings to demonstrate that W′ reconstitution slowed disproportionally with increasing recovery intensity following repeated bouts of exercise and has been quantified with Equationequation 7(5b)
(5b) . However, whilst this slowing of W′ reconstitution was related to the amount of W′ reconstituted following the initial bout and recovery intensity, it could not be apportioned to the fast and slow components with any degree of predictability, thus preventing a continuous equation incorporating a slowing of τFC and τSC from being derived. Furthermore, the application of this fatiguing effect on further repeats remains unknown, whilst the effects of repeated partial depletion of W′ on W′ reconstitution kinetics during multiple bouts of exercise have yet to be investigated. As such, it would be premature to incorporate the slowing effect into the dynamic model without further experimental data.
Limitations
The measurements of W′ determined in the present study are towards the lower ranges reported in previous studies (Bergstrom et al., Citation2014; Murgatroyd & Wylde, Citation2011), possibly due to most of the participants being time-triallist and triathletes. Whilst the absolute values of W′ are poorly correlated to the model parameters, hence unlikely to affect the reconstitution model per se, it supports the need for individualisation of the model as suggested.
To date, all models of W′ reconstitution have been developed from exhaustive exercise (Caen et al., Citation2021; Chorley et al., Citation2022; Skiba et al., Citation2012; Skiba et al., Citation2014), yet a dynamic model of W′ must account for partial depletion and reconstitution as typically encountered during cycle races. Due to the separate fast and slow components of the bi-exponential model, it must be assumed that W′ is depleted proportionally across the two components relative to their individual balances, and that W′ reconstitution follows the same kinetic trajectory as when following full depletion, albeit from a different starting point. Investigations involving partial depletion of W′ have been previously studied (Lievens et al., Citation2021; Sreedhara et al., Citation2020), however these have yet to validate kinetic models of W′ reconstitution, and this remains one avenue for future research, which may lead to refinements and improvements in the application of the proposed model.
Conclusions
This study delivers a full dynamic model of W′ accounting for power and duration during the expenditure and reconstitution of W′ above and below CP. The model can be adapted into an iterative function, to provide second-by-second information to practitioners for training purposes. Furthermore, it can be easily integrated into on-cycle computers to show instantaneous estimations of a cyclist’s W′ that can be used to influence real-time decisions about pacing strategy, such as deciding the power output and duration of the next “attack” or knowing when to abandon such a move before leaving themselves vulnerable. The single additional test session in addition to the prior known parameters of CP and W′ allows the individual customisation of the model to be achieved with minimal impact on training regimes. Further refinement of the W′ reconstitution model should be encouraged to improve its validity in differing scenarios, and whilst the slowing of W′ can be estimated following a repeated exhaustive bout, but further work is required to incorporate this into the reconstitution model.
Author contributions
AC conceived and designed research. AC conducted experiments. AC, SM and KL analysed and interpreted the data, and contributed to the manuscript. All authors read and approved the manuscript.
Availability of data and material
Data is available upon request from the corresponding author.
Consent to participate
Informed consent to participate was obtained from all individual participants included in the study.
Consent for publication
Informed consent to publish was obtained from all individual participants included in the study.
Acknowledgements
The authors would like to thank all the participants of the study.
Disclosure statement
No potential conflict of interest was reported by the author(s).
Additional information
Funding
References
- Abbiss, C. R., Menaspa, P., Villerius, V., & Martin, D. T. (2013). Distribution of power output when establishing a breakaway in cycling. International Journal of Sports Physiology and Performance, 8(4), 452–455. https://doi.org/10.1123/ijspp.8.4.452
- Bartram, J. C., Thewlis, D., Martin, D. T., & Norton, K. I. (2018). Accuracy of W′ recovery kinetics in high performance cyclists—modeling intermittent work capacity. International Journal of Sports Physiology and Performance, 13(6), 724–728. https://doi.org/10.1123/ijspp.2017-0034
- Beaver, W. L., Wasserman, K., & Whipp, B. J. (1986). A new method for detecting anaerobic threshold by gas-exchange. Journal of Applied Physiology (1985), 60(6), 2020–2027. https://doi.org/10.1152/jappl.1986.60.6.2020
- Bergstrom, H. C., Housh, T. J., Zuniga, J. M., Traylor, D. A., Camic, C. L., Lewis Jr, R. W., Schmidt, R. J., & Johnson, G. O. (2013). The relationships among critical power determined from a 3-min all-out test, respiratory compensation point, gas exchange threshold, and ventilatory threshold. Research Quarterly for Exercise and Sport, 84(2), 232–238. https://doi.org/10.1080/02701367.2013.784723
- Bergstrom, H. C., Housh, T. J., Zuniga, J. M., Traylor, D. A., Lewis Jr, R. W., Camic, C. L., Schmidt, R. J., & Johnson, G. O. (2014). Differences among estimates of critical power and anaerobic work capacity derived from five mathematical models and the three-minute all-out test. Journal of Strength and Conditioning Research, 28(3), 592–600. https://doi.org/10.1519/JSC.0b013e31829b576d
- Caen, K., Bourgois, G., Dauwe, C., Blancquaert, L., Vermeire, K., Lievens, E., … Boone, J. (2021). W’ recovery kinetics following exhaustion: A two-phase exponential process influenced by aerobic fitness. Medicine & Science in Sports & Exercise, 1911–1921. https://doi.org/10.1249/MSS.0000000000002673
- Caen, K., Bourgois, J. G., Bourgois, G., Van Der Stede, T., Vermeire, K., & Boone, J. (2019). The reconstitution of W′ depends on both work and recovery characteristics. Medicine & Science in Sports & Exercise, 51(8), 1745–1751. https://doi.org/10.1249/MSS.0000000000001968
- Chidnok, W., Dimenna, F. J., Bailey, S. J., Vanhatalo, A., Morton, R. H., Wilkerson, D. P., & Jones, A. M. (2012). Exercise tolerance in intermittent cycling. Medicine & Science in Sports & Exercise, 44(5), 966–976. https://doi.org/10.1249/MSS.0b013e31823ea28a
- Chidnok, W., DiMenna, F. J., Fulford, J., Bailey, S. J., Skiba, P. F., Vanhatalo, A., & Jones, A. M. (2013a). Muscle metabolic responses during high-intensity intermittent exercise measured by31P-MRS: Relationship to the critical power concept. American Journal of Physiology-Regulatory, Integrative and Comparative Physiology, 305(9), R1085–R1092. https://doi.org/10.1152/ajpregu.00406.2013
- Chidnok, W., Fulford, J., Bailey, S. J., DiMenna, F. J., Skiba, P. F., Vanhatalo, A., … Jones, A. M. (2013b). Muscle metabolic determinants of exercise tolerance following exhaustion: Relationship to the “critical power”. Journal of Applied Physiology (1985), 115(2), 243–250. https://doi.org/10.1152/japplphysiol.00334.2013
- Chorley, A., Bott, R. P., Marwood, S., & Lamb, K. L. (2019). Slowing the reconstitution of W’ in recovery with repeated bouts of maximal exercise. International Journal of Sports Physiology and Performance, 14(2), 149–155. https://doi.org/10.1123/ijspp.2018-0256
- Chorley, A., Bott, R. P., Marwood, S., & Lamb, K. L. (2020). Physiological and anthropometric determinants of critical power, W’ and the reconstitution of W′ in trained and untrained male cyclists. European Journal of Applied Physiology, 120(11), 2349–2359. https://doi.org/10.1007/s00421-020-04459-6
- Chorley, A., Bott, R. P., Marwood, S., & Lamb, K. L. (2022). Bi-exponential modelling of W’ reconstitution kinetics in trained cyclists. European Journal of Applied Physiology, 122(3), 677–689. https://doi.org/10.1007/s00421-021-04874-3
- Day, J. R., Rossiter, H. B., Coats, E. M., Skasick, A., & Whipp, B. J. (2003). The maximally attainable VO2 during exercise in humans: The peak vs. maximum Issue. Journal of Applied Physiology (1985), 95(5), 1901–1907. https://doi.org/10.1152/japplphysiol.00024.2003
- Etxebarria, N., Ingham, S. A., Ferguson, R. A., Bentley, D. J., & Pyne, D. B. (2019). Sprinting after having sprinted: Prior high-intensity stochastic cycling impairs the winning strike for gold. Frontiers in Physiology, 10. https://doi.org/10.3389/fphys.2019.00100
- Forbes, S. C., Paganini, A. T., Slade, J. M., Towse, T. F., & Meyer, R. A. (2009). Phosphocreatine recovery kinetics following low- and high-intensity exercise in human triceps surae and rat posterior hindlimb muscles. American Journal of Physiology-Regulatory, Integrative and Comparative Physiology, 296(1), R161–R170. https://doi.org/10.1152/ajpregu.90704.2008
- Harris, R. C., Edwards, R. H., Hultman, E., Nordesjo, L. O., Nylind, B., & Sahlin, K. (1976). The time course of phosphorylcreatine resynthesis during recovery of the quadriceps muscle in man. Pflügers Archiv, 367(2), 137–142. https://doi.org/10.1007/BF00585149
- Haseler, L. J., Hogan, M. C., & Richardson, R. S. (1999). Skeletal muscle phosphocreatine recovery in exercise-trained humans is dependent on O2 availability. Journal of Applied Physiology (1985), 86(6), 2013–2018. https://doi.org/10.1152/jappl.1999.86.6.2013
- Hureau, T. J., Romer, L. M., & Amann, M. (2018). The ‘sensory tolerance limit': A hypothetical construct determining exercise performance? European Journal of Sport Science, 18(1), 13–24. https://doi.org/10.1080/17461391.2016.1252428
- Johnson, M. A., Mills, D. E., Brown, P. I., & Sharpe, G. R. (2014). Prior upper body exercise reduces cycling work capacity but not critical power. Medicine & Science in Sports & Exercise, 46(4), 802–808. https://doi.org/10.1249/MSS.0000000000000159
- Jones, A. M., Burnley, M., Black, M. I., Poole, D. C., & Vanhatalo, A. (2019). The maximal metabolic steady state: Redefining the ‘gold standard’. Physiological Reports, 7(10), e14098. https://doi.org/10.14814/phy2.14098
- Jones, A. M., & Vanhatalo, A. (2017). The ‘critical power’ concept: Applications to sports performance with a focus on intermittent high-intensity exercise. Sports Medicine, 47(S1), 65–78. https://doi.org/10.1007/s40279-017-0688-0
- Jones, A. M., Wilkerson, D. P., DiMenna, F., Fulford, J., & Poole, D. C. (2008). Muscle metabolic responses to exercise above and below the “critical power” assessed using31P-MRS. American Journal of Physiology-Regulatory, Integrative and Comparative Physiology, 294(2), R585–R593. https://doi.org/10.1152/ajpregu.00731.2007
- Lievens, E., Klass, M., Bex, T., & Derave, W. (2020). Muscle fiber typology substantially influences time to recover from high-intensity exercise. Journal of Applied Physiology (1985), 128(3), 648–659. https://doi.org/10.1152/japplphysiol.00636.2019
- Lievens, M., Caen, K., Bourgois, J. G., Vermeire, K., & Boone, J. (2021). W’ reconstitution accelerates more with decreasing intensity in the heavy versus the moderate intensity domain. Medicine & Science in Sports & Exercise, 53(6), 1276–1284. https://doi.org/10.1249/MSS.0000000000002574
- Monod, H., & Scherrer, J. (1965). The work capacity of a synergic muscular group. Ergonomics, 8(3), 329–338. https://doi.org/10.1080/00140136508930810
- Moritani, T., Nagata, A., Devries, H. A., & Muro, M. (1981). Critical power as a measure of physical work capacity and anaerobic threshold. Ergonomics, 24(5), 339–350. https://doi.org/10.1080/00140138108924856
- Morton, R. H., & Billat, L. V. (2004). The critical power model for intermittent exercise. European Journal of Applied Physiology, 91(2-3), 303–307. https://doi.org/10.1007/s00421-003-0987-z
- Murgatroyd, S. R., & Wylde, L. A. (2011). The power-duration relationship of high-intensity exercise: From mathematical parameters to physiological mechanisms. The Journal of Physiology, 589(10), 2443–2445. https://doi.org/10.1113/jphysiol.2011.209346
- Murgatroyd, S. R., Wylde, L. A., Cannon, D. T., Ward, S. A., & Rossiter, H. B. (2014). A ‘ramp-sprint’ protocol to characterise indices of aerobic function and exercise intensity domains in a single laboratory test. European Journal of Applied Physiology, 114(9), 1863–1874. doi:10.1007/s00421-014-2908-8
- Nevill, A. M., Jones, D. A., McIntyre, D., Bogdanis, G. C., & Nevill, M. E. (1997). A model for phosphocreatine resynthesis. Journal of Applied Physiology (1985), 82(1), 329–335. https://doi.org/10.1152/jappl.1997.82.1.329
- Parolin, M. L., Chesley, A., Matsos, M. P., Spriet, L. L., Jones, N. L., & Heigenhauser, G. J. (1999). Regulation of skeletal muscle glycogen phosphorylase and PDH during maximal intermittent exercise. American Journal of Physiology-Endocrinology and Metabolism, 277(5), E890–E900. https://doi.org/10.1152/ajpendo.1999.277.5.E890
- Poole, D. C., Burnley, M., Vanhatalo, A., Rossiter, H. B., & Jones, A. M. (2016). Critical power. Medicine & Science in Sports & Exercise, 48(11), 2320–2334. https://doi.org/10.1249/MSS.0000000000000939
- Skiba, P. F., Chidnok, W., Vanhatalo, A., & Jones, A. M. (2012). Modeling the expenditure and reconstitution of work capacity above critical power. Medicine & Science in Sports & Exercise, 44(8), 1526–1532. https://doi.org/10.1249/MSS.0b013e3182517a80
- Skiba, P. F., Clarke, D., Vanhatalo, A., & Jones, A. M. (2014a). Validation of a novel intermittent W’ model for cycling using field data. International Journal of Sports Physiology and Performance, 9(6), 900–904. https://doi.org/10.1123/ijspp.2013-0471
- Skiba, P. F., & Clarke, D. C. (2021). The W’ balance model: Mathematical and methodological considerations. International Journal of Sports Physiology and Performance, 16(11), 1561–1572. https://doi.org/10.1123/ijspp.2021-0205
- Skiba, P. F., Fulford, J., Clarke, D. C., Vanhatalo, A., & Jones, A. M. (2015). Intramuscular determinants of the ability to recover work capacity above critical power. European Journal of Applied Physiology, 115(4), 703–713. https://doi.org/10.1007/s00421-014-3050-3
- Skiba, P. F., Jackman, S., Clarke, D., Vanhatalo, A., & Jones, A. M. (2014b). Effect of work and recovery durations on W′ reconstitution during intermittent exercise. Medicine & Science in Sports & Exercise, 46(7), 1433–1440. https://doi.org/10.1249/MSS.0000000000000226
- Sreedhara, V. S. M., Ashtiani, F., Mocko, G. M., Vahidi, A., & Hutchison, R. E. (2020). Modeling the recovery of W′ in the moderate to heavy exercise intensity domain. Medicine & Science in Sports & Exercise, 52(12), 2646–2654. https://doi.org/10.1249/MSS.0000000000002425
- van den Broek, N. M., De Feyter, H. M., Graaf, L. D., Nicolay, K., & Prompers, J. J. (2007). Intersubject differences in the effect of acidosis on phosphocreatine recovery kinetics in muscle after exercise are due to differences in proton efflux rates. American Journal of Physiology-Cell Physiology, 293(1), C228–C237. https://doi.org/10.1152/ajpcell.00023.2007