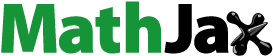
Abstract
HIV/AIDS-COVID-19 co-infection is a major public health concern especially in developing countries of the world. This paper presents HIV/AIDS-COVID-19 co-infection to investigate the impact of interventions on its transmission using ordinary differential equation. In the analysis of the model, the solutions are shown to be non-negative and bounded, using next-generation matrix approach the basic reproduction numbers are computed, sufficient conditions for stabilities of equilibrium points are established. The sensitivity analysis showed that transmission rates are the most sensitive parameters that have direct impact on the basic reproduction numbers and protection and treatment rates are more sensitive and have indirect impact to the basic reproduction numbers. Numerical simulations shown that some parameter effects on the transmission of single infections as well as co-infection, and applying the protection rates and treatment rates have effective roles to minimize and also to eradicate the HIV/AIDS-COVID-19 co-infection spreading in the community.
1. Introduction
Infectious diseases are clinically verified illnesses caused by pathogenic microbial agents’ such as viruses, bacteria, fungi, and parasites [Citation14]. COVID-19 and HIV/AIDS are most common infectious diseases caused by viruses [Citation12,Citation27].
The human immunodeficiency virus (HIV) discovered in 1981, has been among the leading life-affecting viruses expanding throughout nations in the world. Acquired immunodeficiency syndrome abbreviated as AIDS is one of the most community-affecting epidemics in history caused by HIV and it has been a global pandemic since 1981 [Citation28,Citation29,Citation12,Citation22,Citation18]. HIV/AIDs remain a significant global health problem that affects almost 70 million people worldwide and has been a significant cause of morbidity and mortality [Citation12,Citation2,Citation21]. It mainly attacks human white blood cells and is transmitted through sexual intercourse, needle sharing, and direct contact with blood or other body fluids containing the virus and from mother to child during childbirth [Citation19,Citation29,Citation22].
COVID-19 a communicable disease caused by the novel coronavirus (SARS-CoV-2) was discovered for the first time in the middle of December 2019 in China and it has been declared an extremely contagious acute respiratory disease [Citation31,Citation24,Citation26,Citation7,Citation20,Citation22,Citation27]. Since it has rapidly spread throughout nations in the world and causing major health concern the World Health Organization (WHO) declared it as a global pandemic on 11 March 2020 [Citation32,Citation3,Citation20]. It has a direct transmission from human being to human being with direct touch of contaminated materials and indirect transmission with inhalation of respiratory air droplets by coughing and sneezing [Citation23,Citation25,Citation9,Citation17]. The pandemic has an extra health system burden and a great negative impact on nation’s population and economies throughout the whole world [Citation16,Citation10,Citation26]. Recently, WHO approved vaccination, quarantine, isolation, using face masks, washing hands with alcohol, and social distancing are possible prevention and control strategies [Citation32,Citation23,Citation33,Citation10].
Different researchers have investigated that COVID-19 infection could be high in people living with other infections like TB, HIV and cholera who have compromised immunity [Citation24,Citation11,Citation26,Citation1,Citation22,Citation4]. HIV/AIDS infection is the main cause of individuals’ weak immunity and individuals living with HIV/AIDS are highly affected by opportunistic infections like TB, pneumonia and COVID-19 [Citation2,Citation28]. Even though there is no full evidence to justify the observation conclusively, individuals living with HIV/AIDS were more likely to have a positive medical result, and were almost 32% and 82% more likely to get hospital admitting and require ventilation, respectively, because of COVID-19 infection than those had negative HIV/AIDs result [Citation1,Citation22]. Different scholars have formulated and analysed mathematical models to investigate the transmission dynamics of infectious diseases using ordinary differential equations approach like [Citation31,Citation19,Citation6,Citation11,Citation32,Citation3,Citation23,Citation25,Citation16,Citation28,Citation29,Citation10,Citation17,Citation26,Citation7] and using fractional order derivative approach like [Citation1].
Mathematical modelling approaches have been crucial to provide basic frameworks in order to understand the transmission dynamics of infectious diseases [Citation26]. In the development of this paper, we have reviewed research papers that have been done on the transmission dynamics of different infectious diseases. Tchoumi et al. [Citation26] proposed and investigated the co-dynamics of malaria and COVID-19 co-dynamics: with optimal control strategies. The numerical simulation results verifies the theoretical optimal control analysis and illustrates that using malaria and COVID-19 protection measures concurrently can help mitigate there transmission compared with applying single infections protection measures. Teklu, Shewafera Wondimagegnhu, and Koya Purnachandra Rao, 2022 [Citation29] constructed and examined HIV/AIDS and pneumonia co-infection model with control measures such as pneumonia vaccination and treatments of pneumonia and HIV/AIDS infections. H.F. Huo and R. Chen [Citation12] formulated a stage structure HIV/AIDS model to study the transmission of HIV/AIDS with treatment. The model analyses show that ART at the asymptomatic initial stages of the HIV incidence or before-AIDS stage is effective to decrease its spreading rate. Omondi et al. (Citation2018) [Citation21], analysed a sex-structured community infection model and discuss male and female HIV infection trends with heterosexual activities. The analysis of the study deduced that ART treatment has a considerable impact on the HIV/AIDS spreading rate. Hezam et al. [Citation11], formulated a mathematical model for cholera and COVID-19 co-infection which describes the transmission dynamics of COVID-19 and cholera in Yemen. The model analysis, examined four controlling measures such as social distancing, lockdown, the number of test kits to control the COVID-19 outbreak, and the number of susceptible individuals who can get CWTs for water purification. Anwar et al. [Citation33], constructed a mathematical model on COVID-19 with the isolation controlling measure on the COVID-19-infected individuals throughout the community. Ahmed et al. [Citation1] formulated and analysed HIV and COVID-19 co-infection model with ABC-fractional operator approach to investigate an epidemic prediction of a combined HIV-COVID-19 co-infection model. Numerical simulations were carried out to justify that the disease will stabilize at a later stage when enough protection strategies are taken. Ringa et al. [Citation22] developed and examined a mathematical model on HIV and COVID-19 co-infection dynamics with optimal control strategies. The results suggested that HIV prevention strategies can significantly reduce the burden of co-infections with COVID-19, while effective treatment of COVID-19 could reduce co-infections with opportunistic infections such as HIV/AIDS.
Researches show that HIV/AIDS and COVID-19 co-infection is a major public health concern especially in developing nations of the world. The main purpose of this paper is to investigate the impacts of COVID-19 protection, COVID-19 treatment, HIV prevention and HIV treatment prevention and controlling strategies simultaneously on the transmission dynamics of HIV/AIDS and COVID-19 co-infection in the community. Some researchers invested much effort in studying HIV/AIDS and COVID-19 co-infection, none of them has considered COVID-19 protection, COVID-19 treatment, HIV protection, and HIV treatment as prevention and control strategies simultaneously in a single model formulation. Based on the findings of different literatures we have realized the gaps and highly motivated to undertake this study and fill the gap we have mentioned above. The remaining part of the paper is organized in the following sequence; the model is constructed in section 2 and is analysed in section 3. Sensitivity analysis and numerical simulation, and discussions and conclusions of the study are carried out in sections 4, and 5, respectively.
2. Model formulation
Motivated by observation of different scholars epidemiological models in a real world situations have played a fundamental role in the better understanding of the transmission dynamics and controlling and preventive strategies against infectious diseases, in this paper, we have proposed a mathematical model with system of ordinary differential equations. The total human population at a given time which is denoted by
is classified into eight mutually exclusive classes of susceptible individuals denoted by
, COVID-9 protected individuals denoted by
, HIV protected individuals denoted by
, COVID-19 mono-infected individuals denoted by
, HIV mono-infected individuals denoted by
, HIV/AIDS and COVID-19 co-infected individuals denoted by
, COVID-19 recovered individuals denoted by
, and HIV/AIDS treated individuals denoted by
such that the total population is given by
The susceptible individuals acquires HIV/AIDS at the standard incidence rate given by
(1)
(1) where
is the modification parameter that increases infectivity and
is the HIV/AIDS transmission rate.
The susceptible individuals acquires COVID-19 at the standard incidence rate given by
(2)
(2) where
is the modification parameter that increases infectivity and
is the COVID-19 transmission rate.
To formulate the HIV/AIDS and COVID-19 co-infection model we have the following assumptions: ,
and
are portions of the recruited individuals entered the COVID-19 protected class, the HIV protected class, and the susceptible class respectively, the susceptible class is increased by individuals entered from COVID-19 recovered class in which those individuals lost their immunity by the rate
, human populations are homogeneous and variable, no HIV transmission from HIV treated class and no vertical transmission, there is no simultaneous dual-infection transmission, HIV/AIDS and COVID-19 co-infected individuals are treated with the rate
and entered to the HIV/AIDS treated class.
In this section using parameters given in Table , model variables in Table , and the model assumptions given, the schematic diagram for the transmission dynamics of HIV/AIDS and COVID co-infection is given in Figure .
Figure 1. Schematic diagram of HIV/AIDS and COVID-19 transmission dynamics with and
given in (1) and (2), respectively.

Table 1. Model parameters symbols and definitions.
Table 2. Biological meaning of variables.
Using Figure we derive the following system of non-linear differential equations of the co-infection
(3)
(3) With initial conditions
and
(4)
(4) The sum of all the differential equations in (3) is
(5)
(5)
2.1. Basic properties of the COVID-19 and HIV/AIDS co-infection model (3)
The model can be analysed by dealing with different quantitative and qualitative attributes. Since the system deals with human populations which cannot be negative, we need to show that all the state variables are always non-negative as well as the solutions of the system (3) remain positive with positive initial conditions (4) in the bounded region
(6)
(6) It is important to show that each state variable defined in Table with positive initial conditions (4) is non-negative for all-time
in the bounded region given in (6) and the model (3) is mathematically and epidemiologically meaning full.
Theorem 1
(Non-negativity of the model solutions): At the initial conditions (4) the solutions ,
,
,
,
and
of the system (3) are non-negative for all time
.
Proof: Assume ,
,
,
,
,
and
then for all t > 0, we have to prove that
(t) > 0,
,
> 0,
> 0,
> 0,
> 0, and
> 0.
Define: = sup
,
,
and
.
Since are continuous we deduce that
. If
= ∞, then positivity holds.
But, if 0 < <∞,
or
or
or
or
or
or
or
The first equation of the COVID-19 and HIV/AIDS co-infection model (3) gives
and applying the integrating factor method and after some calculations we have got
where
and by the definition of
the solution
hence
.
Again the second equation of the model (3) gives us and we have got
where
and from the definition of
then the solution
hence
.
Similarly we have the results hence
,
hence
,
hence
,
hence
,
hence
, and
hence
.
Thus, by the definition of
and hence all the solutions of the system (3) are non-negative which is the required prove of the theorem.
Theorem 2
(Boundedness of the model solutions): The region given by (6) is boundedin
.
Proof:
Since all the state variables are non-negative by Theorem 1, from (5) in the absence of infections we do have . By incorporating standard comparison theorem we have got
and integrating both sides gives
where
is some constant and after some steps of calculations we have got
which means all possible solutions of the system (3) with positive initial conditions given in (4) enter into the bounded region
.
3. Qualitative analysis of the models
Before we analysed the HIV/AIDS and COVID-19 co-infection model (3), we need to gain some background about the HIV/AIDS mono-infection and COVID-19 mono-infection transmission dynamics.
3.1. HIV/AIDS mono-infection model
The HIV/AIDS mono-infection model of the co-infection model (3) at is given by
(7)
(7) where the total HIV/AIDS mono-infection population is given by
with force of infection given by
, and initial conditions
,
,
, and
. In the region
, it is easy to show that the set
is positively invariant and a global attractor of all positive solutions of the HIV/AIDS mono-infected model (7). Hence, it is sufficient to consider the dynamics of model (7) in
as epidemiologically and mathematically well-posed.
3.1.1. Stability of the disease-free equilibrium point
The disease-free equilibrium (DFE) of the HIV/AIDS mono-infection model (7) is obtained by setting each equation of the system (7) to zero. Also, at the DFE, there are no infections, and treated. Thus, the disease free equilibrium point is .
The linear stability of the DFE is established using the van den Driesch and Warmouth next-generation matrix approach stated in [Citation30] on the model (7). The matrices
and
for the new infection terms and the remaining transfer terms are given by
and
The basic reproduction number of the HIV/AIDS mono-infection model (7) is the dominant eigenvalue of the next-generation matrix
given by
Since the basic reproduction number of HIV/AIDs denoted by
is defined as the expected number of secondary cases generated by one infected individual during its entire period of infectiousness in a fully susceptible population, the DFE
is locally asymptotically stable if
and unstable otherwise.
3.1.2. Existence and uniqueness of endemic equilibrium point
Now making the right-hand side of the dynamical system given in Equation (7) as zero we have determined that
Let us put
,
and substitute
in the HIV/AIDS force on infection we have determined that
and after some simplification we have derived the non-zero linear equation as
(8)
(8) where
and
if
since all parameters are positive.
Then we do have and hence
if and only if
.
Thus, based on the final result there is a unique positive endemic equilibrium for the HIV/AIDS sub-model given in Equation (7) if and only if
.
Lemma 1:
The HIV/AIDS mono-infection model given in Equation (7) has a unique endemic equilibrium solution if and only if .
3.1.3. DFE global asymptotic stability
Lemma 2:
(The Castillo-Chavez et al. criteria stated in [Citation5]), if the HIV/AIDS mono-infection model can be written as
where
be the components of non-infected individuals and
be the components of infected individuals including treated class and
denotes the DFE point of the dynamical system (7).
Assume (i) For ,
is globally asymptotically stable (GAS)
(ii) ,
for
where
is an M-matrix i.e. the off diagonal elements of
are non-negative and
is the region in which the system makes biological sense. Then the fixed point
is GAS equilibrium point of the system (7) whenever
.
Lemma 3:
The DFE point of the HIV/AIDS mono-infection model (7) is GAS if
and the two sufficient conditions given in Lemma 2 are satisfied.
Proof:
Here we are applying Lemma 2 on the HIV/AIDS mono-infection model (7) and we have got the following matrices
and
After some steps of calculations, we have determined that
Now since
we have got
and
, which satisfies condition (ii) of Lemma 2, thus, the DFE point
of the HIV/AIDS mono-infection model (7) is GAS if
.
Biologically, whenever the HIV/AIDS mono-infection disease dies out while the total population increases [Citation6].
3.2. COVID-19 mono-infection model
The COVID-19 mono-infection model of the system (3) at , is given by
(10)
(10) with the COVID-19 mono-infection infection rate (‘force of infection’) given by
(11)
(11) and with COVID-19 mono-infection initial conditions
,
total population
+
.
In the region , it is easy to show that the set
is positively invariant and a global attractor of all positive solutions of the COVID-19 mono-infection model (10). Hence, it is sufficient to consider the dynamics of model (10) in
as epidemiologically and mathematically well-posed.
3.2.1. Stability of DFE point
The disease-free equilibrium point of COVID-19 mono-infection model (10) denoted by is obtained by making the entire model equations equal to zero at
. Thus, the COVID-19 mono-infection model disease-free equilibrium point is
.
The basic reproduction number of COVID-19 mono-infection is the average number of new COVID-19 infection generated by a typically COVID-19 infectious individual in a community. Similarly, using the approach in [Citation30], we find COVID-19 reproduction number to be
.
Since the basic reproduction number of COVID-19 denoted by is defined as the expected number of secondary cases generated by one infected individual during its entire period of infectiousness in a fully susceptible population, the DFE
is locally asymptotically stable if
and unstable otherwise.
3.2.2. Existence and uniqueness of endemic equilibrium point
The endemic equilibrium point(s) of the COVID-19 mono-infection model given in Equation (10) is/are determined by making the right-hand side of the system zero and after some steps of computations we have got that
(12)
(12) where
,
and
.
Now substitute given in Equation (12) in Equation (11) we computed as
and gives as
(13)
(13) Rearranging (13) we have derived the non-zero linear equation
(14)
(14) where
and
if
since parameters have positive values.
From Equation (14) we do have
> 0 if and only if
since all parameters are positive.
Thus, the COVID-19 mono-infection model given in Equation (10) has a unique positive endemic equilibrium point only whenever .
Lemma 4:
The COVID-19 mono-infection model given in Equation (10) has a unique positive endemic equilibrium if and only if .
3.2.3. DFE point global asymptotic stability
Lemma 5:
The DFE point of the COVID-19 mono-infection model (10) is GAS if
whenever the two sufficient conditions given in Lemma 2 are satisfied.
Proof: Here we are applying Lemma 2 on the COVID-19 mono-infection model (10) and setting be the components of non-infected individuals and
be the components of infected individuals including recovery class. Then we have determined the following matrices
and
After some steps of calculations we have determined that
since
,
we have got
,
and
, thus, the DFE point
of the COVID-19 mono-infection model (10) is GAS if
.
Biologically, whenever the COVID-19 mono-infection disease dies out while the total population increases [Citation6].
3.3. COVID-19 and HIV/AIDS co-infection model
Having analysed the dynamics of the two mono-infection models, that is HIV/AIDS mono-infection model (7) and the COVID-19 mono-infection model (10) the main HIV/AIDS and COVID-19 co-infection model (3) is now considered and analysed in the bounded region given in Equation (6).
3.3.1. Stability of DFE point
The disease-free equilibrium point of the HIV/AIDS and COVID-19 Co-infection model (3) denoted by is obtained by making all the equations equal to zero providing that
. Thus, the HIV/AIDS and COVID-19 Co-infection model (3) DFE point is
Similarly, using the approach in [Citation30], the model (3) basic reproduction number denoted by
is to be determined as
The basic reproduction number of COVID-19 and HIV AIDS co-infected model is the dominant eigenvalue of the next-generation matrix
given by
where
,
represent the basic reproduction number for HIV/AIDS mono-infection model (7),
and
are the basic reproduction numbers for COVID-19 mono-infection model (10) and COVID-19 and HIV/AIDS co-infection model (3), respectively.
Since the basic reproduction number of HIV/AIDS and COVID-19 co-infection denoted by is defined as the expected number of secondary cases generated by one co-infected individual during its entire period of infectiousness in a fully susceptible population, the DFE
islocally asymptotically stable if
and unstable otherwise.
3.3.2. Endemic equilibrium of the model (3)
The endemic equilibrium point of the complete model (3) obtained by making each differential equation as zero is given by
Since the model is highly non-linear the explicit computation of the endemic equilibrium of the full model (3) in terms of model parameters is difficult analytically; however, based on the previous analyses of the HIV/AIDS and COVID-19 mono-infection models the model (3) endemic equilibrium point
exists whenever
and
i.e.
. We will give the stability explanation of
in our numerical simulation part.
3.3.3. Bifurcation analysis of the co-infection model
Let ,
,
,
,
,
,
, and
so that
+
,
+
,
.
Further, by using vector notation the full model (3) can be written in the form
with
, as follows
(15)
(15) with
where
and
where
then the method entails evaluating the Jacobian of the system (13) at the DFE
, denoted by
and this gives us
where
,
,
,
,
,
+
,
,
,
,
Without loss of generality, consider the case when and
, so that
. Furthermore, let
is chosen as a bifurcation parameter. Solving for
from
as
we have got the value
.
After solving the Jacobian of the system (13) at the DFE, with
, we obtained the eigenvalues as
< 0 or
or
or
+
if
or
or
or
or
Hence all eigenvalues are negative whenever . It follows that the Jacobian
of (13) at the DFE, with
, denoted by
, has a simple zero eigenvalue (with all other eigenvalues having negative real part). Hence, the Centre Manifold theory can be used to analyse the dynamics of the model (3). In particular, the Castillo-Chavez and Song theorem [Citation6], will be used to show that the model (3) undergoes forward bifurcation at
. Eigenvectors of
: For the case when
, the right eigenvectors of the Jacobian of (15) at
(denoted by
associated with the zero eigenvalue given by
.
The left eigenvectors associated with the zero eigenvalue at
satisfying
given by
After going through detailed computations and simplification, we have the following bifurcation coefficients
and
as
Thus
(16)
(16) Hence, it follows from in Castillo-Chavez and Song [Citation6] that the model (3) do not exhibits a backward bifurcation at
Thus, there is only a DFE point
of theCOVID-19 and HIV/AIDS co-infection model (3) exists which means there is no positive endemic equilibrium point whenever
.
Note: In sections 3.1.5 and 3.2.5 we have shown both the disease-free equilibriums of the HIV/AIDS mono-infection and COVID-19 mono-infection models are GAS whenever the corresponding basic reproduction numbers values are less than unity. Thus based on this result and Equation (16) the DFE point of the COVID-19 and HIV/AIDS co-infection model (3) is GAS if
4. Sensitivity and numerical analysis
To support some qualitative results in the previous sections, we have performed several sensitivity analyses and numerical simulations. To find relevant model parameters illustrated in Table , we have observed and reviewed different literatures that have been done by different scholars and we assume realistic values for the purpose of numerical illustration.
Table 3. Parameter values used for simulations.
4.1. Investigation of sensitivity analysis
Definition: The normalized forward sensitivity index of a variable, , that depends differentially on a parameter
is defined as SI
[Citation8,Citation15].
Sensitivity indices allow us to investigate the relative importance of different parameters in HIV/AIDS and COVID-19 co-infection transmission. The most sensitive parameter has a magnitude of the sensitivity index larger than that of all other parameters. We can calculate the sensitivity index in terms of basic reproduction numbers and
since
.
Using the baseline parameter values given in Table , we have derived Table and Table to show the sensitivity indices of the model parameters.
Table 4. Sensitivity indices of
Table 5. Sensitivity indices of
In this paper, with baseline parameter values given in Table we have computed implied that HIV/AIDS spreads in the community and also we have determined the indices in Table . And sensitivity analysis shows that the HIV/AIDS transmission rate
has the highest impact on the basic reproduction number of HIV/AIDS (
).
Similarly, using parameter values given in Table we have computed implied that COVID-19 is spreading throughout the community and also we have computed the sensitivity indices as shown in Table . And sensitivity analysis shows that the foremost sensitive positive parameter is the COVID-19 spreading rate
. Using Table and Table , biologically we can conclude that the most sensitive parameters to
and
which can be controlled through prevention and control measures are found to be the HIV/AIDS transmission rate
and the COVID-19 transmission rate
respectively.
Figure illustrates the sensitivity indices of the model parameters where the transmission rates and
are biologically the most sensitive parameters directly proportional to the basic reproduction numbers of HIV/AIDS and COVID-19 respectively. Similarly, the portion of recruitment rate entered to HIV protected group
, the portion of recruitment rate entered to COVID-19 protected group
, and the COVID-19 recovery rate
are the most sensitive parameters indirectly proportional to the basic reproduction numbers.
4.2. Numerical simulations
In this section, we conduct numerical solutions of the system (3). For numerical solutions of the proposed model, the initial conditions have non-negative values since the number of individuals in each compartment cannot be negative. All numerical computations were performed in MATLAB using the ode45 embedded Runge–Kutta numerical method. Now, we start to investigate the stability of equilibrium points, examine the influences of various parameters, and explore the impact of intervention strategies. For simulation purposes, we specified the non-negative initial values and baseline parameter values in Table .
4.2.1. Simulation of the model whenever 

The scenario in Figure illustrates the dynamical anticipation of the model (3) over time when the value of . This numerical evaluation confirms the theoretical proof in (3.3.1). The basic reproduction number is computed as
. Figure illustrated that after 20 days the solutions of the COVID-19 and HIV/AIDS co-infection dynamical system (3) are converging to the DFE point whenever
. Biologically it means that the outbreak of COVID-19 and HIV/AIDS co-infection throughout the community will be eradicated in the near futureto. Ultimately, the numerical simulation and the theoretical analysis in (3.3.1) are compatible.
4.2.2. Simulation of the model whenever 

Now, we turn to investigate the stability of the endemic equilibrium point of model (3) where In Figure all the numerical routines show that numerical solutions trajectories attract toward the endemic stable equilibrium of the system. Figure depicts the time series evaluation of model (3) with baseline parameter values given in Table . Now, we computed the basic reproduction number as
, which is greater than one. That means that the model (3) solutions converges to the endemic equilibrium point whenever
(Figure ).
4.2.3. Effect of HIV transmission on COVID-19 infection
Figure 5 illustrates the effect of HIV transmission rate on the number of HIV/AIDS and COVID-19 co-infectious population
The simulation result shows that increasing the value of
increases the number of HIV/AIDS and COVID-19 co-infectious individuals in the community. Consequently, increasing HIV transmission rate
from 0.00001 to 0.8 leads to a highly increase of HIV/AIDS and COVID-19 co-infection
.
4.2.4. Effect of COVID-19 transmission on HIV/AIDS infection
Figure illustrates the effect of COVID-19 transmission rate on the number of HIV/AIDS and COVID-19 co-infectious population
The simulation result shows that increasing the value of
increases the number of HIV/AIDS and COVID-19 co-infectious individuals in the community. Consequently, increasing COVID-19 transmission rate
from 0.00001 to 0.8 leads to a high increase of HIV/AIDS and COVID-19 co-infection
.
4.2.5. Effect of treatment on HIV/AIDS infection
Figure illustrates the effect of treatment rate on the number of HIV infectious population
. The result shows that increasing the value of
decreases the number of HIV/AIDS infectious individuals in the community. Consequently, increasing treatment intervention measure from 0.25 to 0.85 against HIV/AIDS highly decreases the number of HIV/AIDS infectious population
.
4.2.6. Effect of treatment on co-infected population
Figure illustrates the effect of treatment rate on the number of HIV/AIDS and COVID-19 co-infectious population
. The result shows that increasing the value of
decreases the number of HIV/AIDS and COVID-19 co-infectious individuals in the community. Consequently, increasing treatment intervention measure from 0.2 to 0.8 against HIV/AIDS and COVID-19 co-infection highly decreases the number of co- infectious population
.
4.2.7. Effect of 
on 

Figure depicts the transmission rate have the highest direct influence on
. The result shows that
increase the dissemination rate of HIV/AIDS in the community. Consequently, introducing intervention measures against HIV/AIDS dissemination is essential to reduce
value to less than 0.75.
4.2.8. Effect of 
on 

Figure depicts portion of recruitment rate entered to HIV protected group has the highest indirect influence on
. The result shows that
decrease the dissemination rate of HIV/AIDS in the community. Consequently, introducing the portion of recruitment rate
more than the value 0.608 makes the value of
below one.
4.2.9. Effect of 
on 

Figure depicts HIV/AIDS rate has fundamental indirect influence on
. The result shows that as
increases the dissemination rate of HIV/AIDS in the community decreases. Consequently, introducing the treatment rate
more than the value 0.898 makes the value of
below one.
4.2.10. Effect of 
on 

Figure investigated the impact of COVID-19 transmission rate on the COVID-19 basic reproduction number
. The figure reflects that whenever the value of
increases implies the COVID-19 basic reproduction number is going up, and the value of
leads that
Therefore public health policymakers shall concentrate on minimizing the values of COVID-19 transmission rate
to prevent and control COVID-19 expansion in the community. Biologically, it means that the COVID-19 infection increases whenever its transmission rate
increases and the infection will be eradicated from the community whenever
4.2.11. Effect of 
on 

Figure depicts portion of recruitment rate entered to COVID-19 protected group has the highest indirect influence on
. The result shows that
decrease the dissemination rate of COVID-19 in the community. Consequently, introducing the portion of recruitment rate
more than the value 0.898 makes the value of
below one.
4.2.12. Effect of 
on 

Figure depicts HIV/AIDS rate has fundamental indirect influence on
. The result shows that as the COVID-19 recovery rate
increases the dissemination rate of HIV/AIDS in the community decreases. Consequently, introducing the treatment rate
more than the value of 0.758 makes the value of
below one.
5. Discussions and conclusions
Recently, HIV/AIDS and COVID-19 co-infection has been reported in different literature as one of the deadly infectious diseases. It has been a common public health burden especially in developing nations of the world. Some possible control measures against HIV/AIDS and COVID-19 single infections which are considered in this study include protections and treatments. This paper presents the HIV/AIDS and COVID-19 co-infection to investigate the impact of protection measures and treatment on the HIV/AIDS and COVID-19 co-infection transmission dynamics using ordinary differential equation approach. Rigorous analysis of the model shows that, the model solutions are shown to be non-negative and bounded, using next-generation matrix approach the basic reproduction numbers are computed, sufficient conditions for the local and global stabilities of the models’ equilibrium points are determined, and each of the disease-free and endemic equilibrium points of the models are both locally and GAS whenever the corresponding basic reproduction number is below one.
The sensitivity analysis of the model shows that the most important parameters that impact the basic reproduction numbers of the model (hence, disease burden) are the HIV transmission rate, COVID-19 transmission rate, proportion of protections from HIV and COVID-19, respectively, COVID-19 recovery rate of individuals. This suggests that increasing protection rates, treatment rates and recovery rate will decrease HIV/AIDS and COVID-19 co-infection transmission in the community.
The theoretical results are verified by the numerical simulations and it can be summarized as: the complete co-infection model (3) solutions are converging to the DFE point whenever its basic reproduction number that means there is no co-existence of the DFE point together with positive endemic equilibrium point. The complete model (3) solutions are converging to its endemic equilibrium point whenever
Furthermore, the HIV/AIDS basic reproduction number
is decreasing whenever the HIV protection rate
increases, the basic reproduction numbers
and
of the COVID-19 and HIV/AIDS and COVID-19 co-infection models are decreased whenever portion of protection
of COVID-19 increases, the transmission rates increase the corresponding basic reproduction numbers also increase, increasing COVID-19 protection, HIV/AIDS protection, treatment and COVID-19 recovery rate leads to a decrease of the corresponding basic reproduction numbers. Increasing the HIV/AIDS transmission rate
has a direct impact on the HIV/AIDS basic reproduction number and also has a direct impact on the number of co-infectious population. Similarly, the COVID-19 transmission rate
has a direct impact on the COVID-19 basic reproduction number and also has a direct impact on the number of co-infectious population. Increasing the co-infection treatment rate
decreases the number of co-infected individuals. Since the results show that HIV/AIDS transmission has an impact on COVID-19 infected individuals, COVID-19 transmission has a great impact on HIV/AIDS infected individuals, and applying COVID-19 protection rate, HIV/AIDS protection rate, and treatment rates has an effective role to minimize and also to eradicate the HIV/AIDS and COVID-19 co-infection in the community. The stakeholders shall concentrate on maximizing these intervention mechanisms to prevent and control the co-infection in the community.
The study is preliminary and potential researcher can extend it in different ways, such as including the optimal control approach, stochastic approach, fractional order derivative approach, environmental impacts, age and spatial structure, HIV infection stages, validate the model by applying appropriate real infection data.
Disclosure statement
The authors declares no conflict of interest in the study.
Data availability
Data used to support the findings of this study are included in the article.
References
- I. Ahmed, E.F. Doungmo Goufo, A. Yusuf, P. Kumam, P. Chaipanya, and K. Nonlaopon, An epidemic prediction from analysis of a combined HIV-COVID-19 co-infection model via ABC-fractional operator. Alexandria Eng. J. 60(3) (2021), pp. 2979–2995.
- A. Babaei, H. Jafari, and A. Liya, Mathematical models of HIV/AIDS and drug addiction in prisons. Eur Phys J Plus 135(5) (2020), pp. 1–12.
- Y.J. Baek, T. Lee, Y. Cho, J.H. Hyun, M.H. Kim, Y. Sohn, J.H. Kim, et al., A mathematical model of COVID-19 transmission in a tertiary hospital and assessment of the effects of different intervention strategies. PloS one 15(10) (2020), pp. e0241169.
- S.R. Bandekar, and M. Ghosh, A co-infection model on TB-COVID-19 with optimal control and sensitivity analysis. Math. Comput. Simul. 200 (2022), pp. 1–31.
- C. Castillo-Chavez, S. Blower, P. van den Driessche, D. Kirschner, and A-A. Yakubu, Mathematical approaches for emerging and reemerging infectious diseases: models, methods, and theory, Springer Science & Business Media, 2002.
- C. Castillo-Chavez, and B. Song, Dynamical models of tuberculosis and their applications. Math Biosci Eng 1(2) (2004), pp. 361–404.
- T.-M. Chen, J. Rui, Q.-P. Wang, Z.-Y. Zhao, J.-A. Cui, and L. Yin, A mathematical model for simulating the phase-based transmissibility of a novel coronavirus. Infect. Dis. Poverty. 9(1) (2020), pp. 1–8.
- N. Chitnis, J.M. Hyman, and J.M. Cushing, Determining important parameters in the spread of malaria through the sensitivity analysis of a mathematical model. Bull. Math. Biol. 70(5) (2008), pp. 1272–1296.
- L. Cirrincione, F. Plescia, C. Ledda, V. Rapisarda, D. Martorana, R.E. Moldovan, K. Theodoridou, and E. Cannizzaro, COVID-19 pandemic: Prevention and protection measures to be adopted at the workplace. Sustainability 12(9) (2020), 3603.
- D.O. Daniel, Mathematical model for the transmission of Covid-19 with nonlinear forces of infection and the need for prevention measure in Nigeria. J. Infect. Dis. Epidem 6 (2021), pp. 158.
- I.M. Hezam, A. Foul, and A. Alrasheedi, A dynamic optimal control model for COVID-19 and cholera co-infection in Yemen. Adv. Differ. Equ. 1(2021) (2021), pp. 1–30.
- H.-F. Huo, and R. Chen, Stability of an HIV/AIDS treatment model with different stages. Discrete. Dyn. Nat. Soc. 2015 (2015), pp. 1–9.
- J. Lutera, D. Mbete, and S. Wangila, Co-infection model of HIV/AIDS-pneumonia on the effect of treatment at initial and final stages. IOSR J. Math. (IOSR-JM) 14(5) (2018), pp. 56–81.
- M. Martcheva, An Introduction to Mathematical Epidemiology. Vol. 61, Springer, New York, 2015.
- T.T. Mekonnen, Mathematical model analysis and numerical simulation for codynamics of meningitis and pneumonia infection with intervention. Sci. Rep. 12(1) (2022), pp. 1–22.
- J.Y. Mugisha, J. Ssebuliba, J.N. Nakakawa, C.R. Kikawa, and A. Ssematimba, Mathematical modeling of COVID-19 transmission dynamics in Uganda: Implications of complacency and early easing of lockdown. PloS one 16(2) (2021), pp. e0247456.
- S.S. Musa, I.A. Baba, A. Yusuf, T.A. Sulaiman, A.I. Aliyu, S. Zhao, and D. He, Transmission dynamics of SARS-CoV-2: A modeling analysis with high-and-moderate risk populations. Results Phys. 26 (2021), pp. 104290.
- J.K. Nthiiri, G.O. Lavi, and A. Mayonge, Mathematical model of pneumonia and HIV/AIDS coinfection in the presence of protection. Int. J. Math. Anal 9(42) (2015), pp. 2069–2085.
- A. Nwankwo, and D. Okuonghae, Mathematical analysis of the transmission dynamics of HIV syphilis co-infection in the presence of treatment for syphilis. Bull. Math. Biol. 80(3) (2018), pp. 437–492.
- A. Omame, N. Sene, I. Nometa, C.I. Nwakanma, E.U. Nwafor, N.O. Iheonu, and D. Okuonghae, Analysis of COVID-19 and comorbidity co-infection model with optimal control. Optim. Control Appl. Methods 42(6) (2021), pp. 1568–1590.
- E.O. Omondi, R.W. Mbogo, and L.S. Luboobi, Mathematical analysis of sex-structured population model of HIV infection in Kenya. Lett. Biomath. 5(1) (2018), pp. 174–194.
- N. Ringa, M.L. Diagne, H. Rwezaura, A. Omame, S.Y. Tchoumi, and J.M. Tchuenche, HIV and COVID-19 co-infection: A mathematical model and optimal control. Inform. Med. Unlocked 31, (2022), pp. 100978.
- P. Riyapan, S.E. Shuaib, and A. Intarasit, A mathematical model of COVID-19 pandemic: A case study of Bangkok, Thailand. Comput. Math. Methods. Med. 2021 (2021), pp. 1–11.
- P. Ssentongo, E.S. Heilbrunn, A.E. Ssentongo, S. Advani, V.M. Chinchilli, J.J. Nunez, and P. Du, Epidemiology and outcomes of COVID-19 in HIV-infected individuals: a systematic review and meta-analysis. Sci. Rep. 11(1) (2021), pp. 1–12.
- D. Sun, X. Long, and J. Liu, Modeling the COVID-19 epidemic with multi-population and control strategies in the United States. Front. Public. Health. 9 (2021), pp. 1–14.
- S.Y. Tchoumi, M.L. Diagne, H. Rwezaura, and J.M. Tchuenche, Malaria and COVID-19 co-dynamics: A mathematical model and optimal control. Appl. Math. Model. 99 (2021), pp. 294–327.
- S.W. Teklu, Mathematical analysis of the transmission dynamics of COVID-19 infection in the presence of intervention strategies. J. Biol. Dyn. 16(1) (2022), pp. 640–664.
- S.W. Teklu, and T.T. Mekonnen, HIV/AIDS-pneumonia co-infection model with treatment at each infection stage: Mathematical analysis and numerical simulation. J. Appl. Math. 2021 (2021), pp. 1–21.
- S.W. Teklu, and K.P. Rao, HIV/AIDS-Pneumonia codynamics model analysis with vaccination and treatment. Comput. Math. Methods. Med. 2022 (2022), pp. 1–20.
- P. Van den Driessche, and J. Watmough, Reproduction numbers and sub-threshold endemic equilibria for compartmental models of disease transmission. Math. Biosci. 180(1–2) (2002), pp. 29–48.
- I.M. Wangari, S. Sewe, G. Kimathi, M. Wainaina, V. Kitetu, and W. Kaluki, Mathematical modelling of COVID-19 transmission in Kenya: A model with reinfection transmission mechanism. Comput. Math. Methods. Med. 2021 (2021), pp. 1–18.
- H.M. Yang, L.P. Lombardi Junior, F.F. Morato Castro, and A.C. Yang, Mathematical modeling of the transmission of SARS-CoV-2, evaluating the impact of isolation in São Paulo State (Brazil) and lockdown in Spain associated with protective measures on the epidemic of Covid-19. Plos One 16(6) (2021), pp. 1–24.
- A. Zeb, E. Alzahrani, V.S. Erturk, and G. Zaman, Mathematical model for coronavirus disease 2019 (COVID-19) containing isolation class. BioMed Res. Int. 2020 (2020), pp. 1–7.