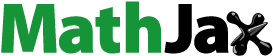
Abstract
In this paper, we consider a flux-limited Keller–Segel model derived in [Citation16, Citation18] in a one-dimensional bounded domain and give a refined asymptotic result of the spiky steady state by using the Sturm oscillation theorem in a more meticulous way based on the existence result of spiky steady state in [Citation4], showing a more accurate characterization of the cell aggregation phenomenon.
1. Introduction
Chemotaxis is a fundamental cellular reaction for survival, which describes the directional movements towards or away from the chemical stimuli. It is a fundamental mechanism in many important biological phenomenon such as the healing of wound [Citation17], pattern formation [Citation2, Citation14] and so on. Mathematical models of chemotaxis were widely studied in recent years and the model proposed by Keller and Segel [Citation9, Citation10] was well known and received widespread acceptance. A lot of outstanding work has been done on the Keller–Segel system and its variants to study the aggregation and wave propagation of cells, two important phenomena in chemotaxis, and we refer the reader to [Citation5, Citation7, Citation8] for details. About the wave propagation phenomenon, we refer the reader to [Citation12] and [Citation19], which are the papers for the traveling waves and the periodic waves generated from the Keller–Segel model.
In this paper, we focus on the flux-limited Keller–Segel (FLKS) system, which reads as follows: (1)
(1) where
represents the density of cells and
the concentration of chemical substance at time t and position x; ψ is the function to describe the chemical response mechanism, which is so-called the chemotactic sensitivity function with the chemotactic coefficient
. ψ is assumed to be a smooth function and a typical form of ψ is
. The assumption of limited flux here means that there exists a positive constant
such that
and
. So when the cells are in the large gradient environment (
is large), the chemotactic flux,
, is bounded to model velocity saturation.
The earliest relevant work about the FLKS system is in [Citation15], where Patlak first used the kinetic theory to express the chemotactic velocity in term of the average of velocities and run times of individual cells. Later, this approach was boosted and developed by Alt et al. [Citation1, Citation13] and so on. The derivation of the kinetic chemotaxis model in previous work gives the motivation to study the FLKS system. One can see details of the derivation of the FLKS system in [Citation16, Citation18].
In the FLKS system, since the global existence in time t of the solutions of (Equation1(1)
(1) ) with flux limited assumption is proven in [Citation4, Citation6], blow-up will not happen in finite time. In [Citation16], the large-time behaviour of the FLKS system in
was studied with a fixed initial mass
, denoted as m, for
. From [Citation16], we know that the existence of positive non-constant radial steady states depends on the dimension n and the initial mass m, that is, for n = 2, radial symmetric solutions exist if and only if
while there is no positive non-constant radial steady state for any m>0 when n>2. For any bounded domain
,
, the FLKS system with Neumann boundary conditions and mass constraint has positive non-constant radial steady states when the chemotactic coefficient χ is large enough (see [Citation20]), where the mass constraint means that
which comes from the integration of (Equation1
(1)
(1) ) with Neumann boundary conditions. In [Citation20], the existence of radial spiky steady states is proved, that is, when χ tends to be infinity, the non-constant radial steady states found in [Citation20] tends to be like spikes, where finding spiky steady states is another way in mathematics to describe the aggregation phenomenon of chemotaxis models except blow-up.
In this paper, we consider the one-dimensional steady states of the FLKS system (Equation1(1)
(1) ) with the mass constraint and Neumann boundary conditions in a bounded domain as follows:
(2)
(2) where α, M and L are fixed positive constants. We denote ML as the total mass of cells and denote
for convenience. Hence
satisfying
(3)
(3) The flux limited situation
and the smoothness of the function ϕ imply that there must exist a constant
such that
. Without loss of generality, we assume
in the following for simplicity.
The existence of monotone solution of (Equation2(2)
(2) ) is proved in [Citation4] for
where
is a constant depends on L, α, M and ϕ. As χ goes to infinity, it is proved that the decreasing solution ρ concentrates at x = 0, that is
in the sense of distribution, and the decreasing solution S converges to an explicit function uniformly on
, by using Helly's compactness theorem and the Sturm oscillation theorem (see Theorem 5.4 in [Citation4]), which implies the existence of the spiky steady state and hence the aggregation of cells. In this paper, we use the Sturm oscillation theorem in a more meticulous way to give a refined asymptotic result, that is, to give a more accurate characterization of the cell aggregation.
2. A priori estimate
Here we give the priori estimate first.
Lemma 2.1
If is the positive classical solution of (Equation2
(2)
(2) ), we have
(4)
(4)
(5)
(5) and
(6)
(6)
Proof.
We use the Green's function to give the lower bound of S. For any fixed , let
be the Green's function satisfying
Observe that G can be explicitly given by
Since
,
, we have that for any
,
Now we give the upper bound of v. Recall
Since
and
, we can rewrite
as
for
. Because the solution is positive, that is,
on
, we have
where the first inequality comes from the second equality in (Equation1
(1)
(1) ). The mean value theorem of integration implies that there exists some
such that
. Similarly, S can be estimated by
By the equation in the first line of (Equation2
(2)
(2) ) and the boundary condition, we have
which implies that
Take
for some
. Then
that is
3. Refined asymptotic
Recalling in [Citation4], the results that as ,
in the sense of distribution and
uniformly on
were proved. These results are true in our paper, and we give a refined asymptotic result below with a method coming from [Citation3]. Although both the functions ρ and S depend on the parameter χ, for convenience, in the following we still use the notations
and
other than
and
.
Theorem 3.1
For the solutions of (Equation2(2)
(2) ) in Theorem 5.4 in [Citation4], we have a further result, which is
(7)
(7) and
(8)
(8)
Remark 3.1
Comparing Equation (53) in [4] and Equation (Equation2(2)
(2) ) in our paper, we denote
. Observe that if the assumptions in (54) in [Citation4] are satisfied, that is,
,
and
, then our assumptions in (Equation3
(3)
(3) ) are satisfied by the facts
and
. So the assumptions of function ϕ in our paper give more relaxed restrictions of the FLKS system (Equation2
(2)
(2) ) than the one, system (53) and (56), in [Citation4]. Actually, using the same methods in [Citation4] and our paper, we can also obtain the results that as
,
in the sense of distribution and
uniformly on
and the result in Theorem 3.1 for system (Equation2
(2)
(2) ) with ϕ defined in (Equation3
(3)
(3) ).
Remark 3.2
From (Equation7(7)
(7) ), we can see that as
, the function
tends to be 0 with the order
on
and tends to be infinity with the order χ at the point 0. So we have
for
and
as
and Theorem 3.1 gives more detailed description of the profile of
when χ is large, that is, how the profile of ρ tends to be like a spike at the point 0 as
. Denote
and
, it is easy to check that
, and we can see the change of the profile of
clearly by (Equation8
(8)
(8) ) when χ is large.
Proof.
We will use Sturm's oscillation theorem in a more meticulous way to get our result. Recall for
. Using Sturm's oscillation theorem by a comparison between
and
, and using the fact that
on
for fixed
, we obtain that
for
. By (Equation5
(5)
(5) ) and the fact that ρ is decreasing, we have
for
and hence
where
is well defined because
is
smooth. Thus we have
which implies that
(9)
(9) Recall that
. Thus with Lemma 2.1 we have
Integrating the above equation over
, we obtain
which is
Since
is uniformly bounded for all large χ, we can assume that there exists some constant B such that
after passing to a subsequence as
. Then we take
and
Thus
and
Since S and
are uniformly bounded for all
,
is uniformly bounded. So we can assume
locally uniformly on
after further passing to a subsequence as
. For any fixed point
, since
as
,
as
, which implies that
By the integration of
, we have
which is
Now we need to find the value of B. Observe that
By (Equation9
(9)
(9) ), we know
which is,
.
Since the limit is unique, we have
By the monotonicity of
, we have
(10)
(10) Then the Lebesgue's dominated convergence theorem gives us
Recall that
for some positive constant C and
. Then we have
by (Equation10
(10)
(10) ) and the fact that
converges to
as
. Thus we prove (Equation7
(7)
(7) ).
By the representation of S via Green's function, which is
then we have
With a same discussion of the estimate of
in the proof of Theorem 3.2 in [Citation11], we have (Equation8
(8)
(8) ) and we finish this proof.
Disclosure statement
No potential conflict of interest was reported by the author(s).
Additional information
Funding
References
- W. Alt, Biased random walk model for chemotaxis and related diffusion approximation, J. Math. Biol. 9 (1980), pp. 147–177.
- E. Budrene and H. Berg, Complex patterns formed by motile cells of Escherichia coli, Nature 349 (1991), pp. 630–633.
- X. Chen, J. Hao, X. Wang, Y. Wu, and Y. Zhang, Stability of spiky solution of Keller–Segel's minimal chemotaxis model, J. Differ. Equ. 257 (2014), pp. 3102–3134.
- A. Chertock, A. Kurganov, X. Wang, and Y. Wu, On a chemotaxis model with saturated chemotactic flux, Kinet. Relat. Models 5 (2012), pp. 51–95.
- T. Hillen and K. Painter, A user's guide to PDE models for chemotaxis, J. Math. Biol. 58 (2009), pp. 183–217.
- T. Hillen, K. Painter, and C. Schmeiser, Global existence for chemotaxis with finite sampling radius, Discrete Contin. Dyn. Syst. Ser. B. 7 (2007), pp. 125–144.
- D. Horstmann, From 1970 until present: The Keller–Segel model in chemotaxis and its consequences I, Jahresber. DMV 105 (2003), pp. 103–165.
- D. Horstmann, From 1970 until present: The Keller–Segel model in chemotaxis and its consequences II, Jahresber. DMV 106 (2004), pp. 51–69.
- E. Keller and L. Segel, Initiation of slime mold aggregation viewed as an instability, J. Theor. Biol.26 (1970), pp. 399–415.
- E. Keller and L. Segel, Models for chemotaxis, J. Theor. Biol. 30 (1971), pp. 225–234.
- H. Li, Spiky steady states of a chemotaxis system with singular sensitivity, J. Dyn. Differ. Equ. 30 (2018), pp. 1775–1795.
- P. Liu, J.P. Shi, and Z.A. Wang, Pattern formation of the attraction-repulsion Keller–Segel system, Discrete Contin. Dyn. Syst. Ser. B 18 (2013), pp. 2597–2625.
- H. Othmer, S.R. Dunbar, and W. Alt, Models of dispersal in biological systems, J. Math. Biol. 26 (1988), pp. 263–298.
- K.J. Painter, P.K. Maini, and H.G. Othmer, Stripe formation in juvenile pomacanthus explained by a generalized turing mechanism with chemotaxis, Proc. Natl. Acad. Sci. 96 (1999), pp. 5549–5554.
- C.S. Patlak, Random walk with persistence and external bias, Bull. Math. Biophys. 15 (1953), pp. 311–338.
- B. Perthame, N. Vauchelet, and Z. Wang, The flux limited Keller–Segel system; properties and derivation from kinetic equations, Rev. Mat. Iberoam. 36 (2020), pp. 357–386.
- G.J. Petter, H.M. Byrne, D.L.S. Mcelwain, and J. Norbury, A model of wound healing and angiogenesis in soft tissue, Math. Biosci. 136 (1996), pp. 35–63.
- J. Saragosti, V. Calvez, N. Bournaveas, A. Buguin, P. Silberzan, and B. Perthame, Mathematical description of bacterial traveling pulses, PLoS Comput. Biol. 6 (8) (2010), Article ID e1000890.
- Z.A. Wang, Mathematics of traveling waves in chemotaxis, Discrete Contin. Dyn. Syst. Ser. B. 18 (2013), pp. 601–641.
- Z.A. Wang and X. Xu, Radial spiky steady states of a flux-limited Keller–Segel model: Existence, asymptotics and stability, Stud. Appl. Math. 148 (2022), pp. 1251–1273.