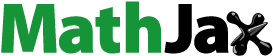
Abstract
Here, we investigate a mathematical model to assess the impact of disinfectants in controlling diseases that spread in the population via direct contacts with the infected persons and also due to bacteria present in the environment. We find that the disease-free and endemic equilibria of the system are related via a transcritical bifurcation whose direction is forward. Our numerical results show that controlling the transmissions of disease through direct contacts and bacteria present in the environment can help in reducing the disease prevalence. Moreover, fostering the recovery rate and the death rate of bacteria play significant roles in disease eradication. Our numerical observations convey that reducing the bacterial density at the source discharged by the infected population through the use of chemicals has prominent effect in disease control. Overall, our findings manifest that the disinfectants of high quality can completely control the bacterial density and the disease outbreak.
1. Introduction
The utmost challenging issue for the survival of human lives apart from war, starvation and natural disasters is the spread of infectious diseases around the world. The outbreak of these diseases not only enhances the mortality rate but also requires an enormous amount of wealth to prevent them [Citation14]. Therefore, it is essential to find the means to control the prevalence of diseases. Infectious diseases can be transmitted through the air (air-borne transmission), water (water-borne transmission), and the direct contact with the infected persons and also indirectly via the intermediate objects known as vectors/carriers. Bacterial diseases such as cholera and typhoid still remain as a major public health problem in developing countries, where outbreaks continue to occur due to poor sanitation and personal hygiene [Citation8, Citation15]. Pathogenes of bacterial diseases, like cholera (V. cholerae), typhoid (Salmonella typhi), diarrhoea and dysentery (Shigella) spread through contaminated food and water or from an infected person to a healthy one as a result of poor hygiene. Some individuals produce growth substrates surrounding the bacterial cells, thereby provide a very conducive environment to the bacteria, resulting in the fast spread of the diseases. The clinically infected animals/humans harbour and shed bacteria in the environment, and act as a source of infection to susceptible humans through direct contact or indirectly through fecal-contaminated environments [Citation13]. Globally, more than 600 million people living in urban areas are still facing a lack of basic sanitation, and the total urban population is expanding by around 80 million per year [Citation49], making it a challenge to keep up with sanitation infrastructure requirements. Consumption of polluted drinking water is estimated to cause more than five hundred thousand diarrhoeal deaths every year [Citation48]. According to WHO, every year there are 1.3–4.0 million cases of cholera and 21,000–143,000 deaths worldwide [Citation3]. Therefore, it has become desirable to wipe out pathogenic bacteria from the environment by some means to control the prevalence of diseases. Pathogenic bacteria, such as E. coli pathogenic strains, Campylobacter, V. cholera , Salmonella, and Shigella are treated by using disinfectants (chlorine, chloramine, ozone, hydrogen peroxide with UV and chemical coagulation), thereby preventing waterborne diseases.
The study of various infectious diseases has been carried out in the past few decades by considering the direct contact of susceptible with infectives, without taking into account the role of bacteria present in the environment [Citation4, Citation14, Citation16, Citation17]. However, there are certain diseases such as tuberculosis and typhoid fever which spread via direct contacts with infectives as well as by the bacteria present in the environment [Citation9, Citation12, Citation34–36, Citation51]. Therefore, it is salient to study the dynamics of such diseases so that proper control strategies can be applied. Mathematical modelling is a useful methodology for evaluating the hypotheses that underlie disease processes, which can be applied to assess the impact of control efforts and predict future trends. Several kinds of mathematical models have been proposed to understand the bacterial transmission and their control. Singh et al. [Citation41] have assumed that disease spreads in the population due to the direct contact between susceptible and infected individuals as well as due to the bacteria transported by the carriers, like flies and ticks from the environment to the water and edibles of human population. They have considered that the density of carrier population follows logistic growth and human-related activities make the environment conducive for the growth of carrier population. They found that the disease can be controlled by controlling the growth rate of density of carrier population; however the immigration of population to susceptible class and human-related activities make the disease endemic. This model has been generalized by Misra et al. [Citation27, Citation28] for cholera and typhoid diseases by incorporating the fact that the density of carrier population in the environment can be controlled using the chemicals like DDT and chlorine. Authors have concluded that the use of chemicals not only control the carrier population but also reduces the number of infected individuals in the community.
Notably, in the aforementioned studies, the density of bacteria has not been considered explicitly, and the authors have assumed that the bacteria of the disease are present in the environment. Shukla et al. [Citation39] have incorporated the density of bacteria explicitly as a dynamic variable in the modelling phenomenon. Here, authors have assumed that the susceptible individuals contract the infection due to direct contacts with infected individuals and indirectly either through the bacteria transported by the carriers to the edibles or due to the movement of susceptible individuals in the bacteria filled environment. The findings showed that the growth rate of bacteria in the environment due to discharge of excreta of infected individuals causes challenges to control the spread of the disease. A mathematical model for the control of cholera disease using chemicals that reduce the density of bacteria in the aquatic environment was analysed by Misra and Singh [Citation32]. Authors have assumed here a bilinear interaction between susceptible individuals to the density of bacteria, and that the rate of use of chemicals in the aquatic environment is proportional to the density of bacteria in the aquatic environment with some time lag. Shukla et al. [Citation40] have studied the effect of sanitation effort on the spread of bacterial diseases, and found that if sanitation effort is suitably applied to the human habitat, the density of the bacteria declines which in turn reduces the number of infected population. Musa et al. [Citation33] incorporated public health education programmes (that include house-to-house enlightenment, media coverage, reports, etc.) to assess its impact and provide effective strategies to combat typhoid fever in the endemic areas. They obtained wavelet results to show the temporal patterns of typhoid fever outbreaks in Taiwan.
Media coverage is also a key effective approach for controlling the spread of infectious diseases because it raises public awareness of disease transmission modes, which leads to behavioural changes in people's attitudes about disease transmission. Some studies have been conducted to control the spread of infectious diseases with the impact of media [Citation10, Citation11, Citation20, Citation21, Citation29–31, Citation37, Citation38, Citation44]. In particular, Dubey et al. [Citation10] have studied the joint effects of awareness programmes through media and treatment of disease in infected population. They have concluded that the optimal treatment together with awareness can help in the total eradication of infectious disease from the population. Misra et al. [Citation29] have developed a mathematical model to study the effect of TV and social media advertisements to control the infectious diseases. They observed that more TV and social media advertisements cause destabilization of the system and induce oscillations through Hopf-bifurcation. Recently, Lata et al. [Citation19] have studied a mathematical model for the water-borne diseases, like cholera by incorporating TV and social media advertisements as a dynamic variable. They have assumed that aware individuals adapt some precautionary measures not to contract the infection and they also make some effectual efforts, like chlorination of water, etc., to reduce the density of bacteria in the aquatic environment. The outcomes of this study showed that awareness among the people due to TV and social media advertisements, and chlorination of water made by aware individuals have substantial impact on the control of water-borne diseases. Majumder et al. [Citation23] investigated the impact of saturated treatments on HIV-TB dual epidemic as a consequence of COVID-19. They have explored the joint effects of awareness and treatment by analysing an optimal control problem. Massard et al. [Citation26] investigated a multi-strain epidemic model for COVID-19 by considering the infected as well as asymptomatic cases. The study reveals that the beta/gamma variants could have led to a larger number of infections in both symptomatic and asymptomatic people. The findings of Sun et al. [Citation42] suggest that stamping out the infected individuals and blocking the epidemic zones play significant roles in the prevention and control of the foot-and-mouth diseases. Ma et al. [Citation22] observed that by enhancing the effective coverage of mask use in public, the cumulative confirmed cases can be reduced to a great extent. A 10 year systematic review of mathematical models for dengue fever epidemiology by Aguiar et al. [Citation2] provide insights on general features to be considered to model the aspects of real-world public health problems, such as the current epidemiological scenario (COVID-19 pandemic) we are living in. The study of Asamoah et al. [Citation5] demonstrated that in the absence of vaccination, practising physical or social distancing protocols is the most cost-saving and most effective control intervention in Saudi Arabia.
Besides the aforementioned studies, it is crucial to reduce the density of bacteria in the environment for the eradication of the disease from the community. To achieve this goal, i.e. eradication of disease, in this paper, we formulate and analyse a mathematical model to control the density of bacteria in the environment using chemicals. Here, we assume that a part of the chemical is used to treat the excreta of infected human population, i.e. reducing the density of bacteria at source and the remaining part of the chemical is used to kill the bacteria in the environment.
We arrange the remaining portions of this paper in the following way. In the next section, we propose our epidemic model. In the following section, the model is analysed; positivity and boundedness of the system's solutions are shown; equilibria are obtained and their stability properties are discussed; an explicit expression for the basic reproduction number is derived by using the next-generation matrix method; the existence of transcritical bifurcation between the disease-free and endemic equilibria is shown. The simulation results are carried out in Section 4 to extrapolate the analytical findings and explore the role of disinfectants in controlling the disease. The paper ends with important observations of this study in Section 5.
2. The mathematical model
Here, we propose a mathematical model for the diseases which spread among the human population due to direct contact of the susceptible persons with the infected ones and also through the bacteria of these diseases present in the environment. To this end, we classify the total human population of the considered region into three disjoint groups: susceptible individuals (S), infected individuals (I) and the recovered individuals (R). Let the density of bacteria present in the environment of the considered domain be B. Also, we assume that amount of disinfectants is being used to control the excessive bacterial growth at the hot spots of the infected individuals.
Table summarizes all the considered dynamical variables for the model to be investigated here. In the formulation of the model, the following ecological/epidemiological assumptions are made.
Table 1. Descriptions of variables used in the model system (Equation1(1)
(1) ).
All the new immigrants are susceptible and join the group at a constant rate Λ. The population is homogeneously mixed and disease spreads via the direct contact of susceptible with infected individuals, and also through bacteria present in the environment.
All the susceptible individuals living in the habitat are affected by the bacterial populations. Besides its self-growth, the density of bacteria in the environment increases as the number of infected individuals increases.
Disinfectants/sanitizers potentially suppress the density of bacteria at source (medical hospitals, infected hot spots) discharged by infected individuals, and are introduced at a rate proportional to density of bacteria present in the environment.
The bacterial populations at source discharged by infected individuals could be minimized to a limited extent by the use of disinfectants/sanitizer due to their limited effectiveness and also the cost involved for the purchase.
The concentration of chemicals reduces due to its uptake by bacteria present in the environment apart from its natural depletion.
The concentration of disinfectants/sanitizers reduces due to their use to control the density of bacteria at source discharged by infected individuals.
The infected individuals recover from infection through proper treatment and subsequently join the recovered class.
The individuals in the recovered class lose their immunity with passage of time and become susceptible again.
There is natural mortality in each human cohort, whereas infected individuals experience disease induced death additionally.
Considering the above assumptions all together, a schematic diagram representing the problem is depicted in Figure , and the model equations are obtained as follows:
(1)
(1)
Let
,
,
,
and
be the initial conditions for solving the above set of differential equations.
Figure 1. Schematic diagram of model system (Equation1(1)
(1) ).

In model (Equation1(1)
(1) ), we have assumed that the density of bacteria increases in the environment due to the self-growth and discharge by the infected individuals (mainly excreta of infectives). The chemical disinfectants are used to control the bacteria in the environment. A part of the chemical disinfectants (i.e.
) is assumed to be used for treating the excreta of infectives (i.e. the control of bacteria at source) and the remaining is used to reduce the bacteria in the environment. As the chemical disinfectants have the limited effect on the treatment of excreta (i.e. all the bacteria present in the excreta cannot be killed in the single application of chemical disinfectants), so we have considered its limited impact
. Further, it is worthy to note that the coefficient of decay in the density of bacteria present in the environment due to uptake of chemicals (i.e.
) should be greater than one as a small amount of chemical disinfectants may kill a large amount of bacteria. Further, the per-capita natural death rate of bacteria will be greater than its self growth rate, i.e.
. Apparently, if
, then there is outburst of the bacterial population in the environment. Thus, to limit the net growth rate of bacteria in the environment, we must impose the condition
in system (Equation1
(1)
(1) ). Also, it may be noted that
. For epidemiological reasons, all the parameters involved in system (Equation1
(1)
(1) ) are considered to be positive, and are described in Table .
Table 2. Descriptions of parameters involved in system (Equation1(1)
(1) ).
At first, we show that the model system (Equation1(1)
(1) ) is well-possed. In this regard, we present the following theorem [Citation43].
Theorem 2.1
Let be the cone of non-negative vectors in
. Let
defined by
where
be locally Lipschitz and satisfy
whenever
and
. Then, for every
, there exists a unique solution of
,
with values in
, which is defined on some interval
, b>0. If
, then
Proof.
To apply this theorem for system (Equation1(1)
(1) ), we have to show that
is locally Lipschitz and
. If
, then the condition
is trivially satisfied. As the right hand of system (Equation1
(1)
(1) ) is continuously differentiable on
, so the locally Lipschitz condition trivially holds. Let
, i.e.
. Taking
as an example, we have
Notably the partial derivative of F exists and is bounded in
, i.e. F is locally Lipschitz. This implies that there exists a unique solution which is defined on
, b>0. Assume that
, then
This contradicts the boundedness of
. Thus,
, and hence there exists a unique, non-negative solution on
.
Now, using the fact that N = S + I + R, we reduce system (Equation1(1)
(1) ) to the following equivalent form:
(2)
(2)
Now onwards, we study the dynamics of system (Equation2
(2)
(2) ) in detail.
3. Mathematical analysis of system (2)
3.1. Positivity and boundedness of solutions
Theorem 3.1
If , system (Equation2
(2)
(2) ) has a unique non-negative solution. The region of attraction for all solutions of model (Equation2
(2)
(2) ) initiating in the positive orthant is given by the following set:
With respect to model system (Equation2
(2)
(2) ), the region Ω is compact and positively invariant. Further, it is closed and bounded in the positive cone of the five-dimensional space.
Proof.
We can rewrite system (Equation1(1)
(1) ) in the following form:
where
and
with
The vector
is positive. Notably, all the off-diagonal entries of the matrix
are non-negative. Therefore, the matrix
is Metzler for every
. Thus, system (Equation1
(1)
(1) ) is positively invariant in
[Citation1]. Hence, all the solution trajectories of system (Equation1
(1)
(1) ) that originate from initial states in
, confine therein forever.
From the third equation of system (Equation2(2)
(2) ), we have
Using the standard comparison theorem [Citation18], we have
If
then the above inequality gives
Hence, for any larger values of t,
Now, we have
At N−I−R = 0, we have
. This implies that
. Therefore,
for any large time. Further, from the fourth equation of system (Equation2
(2)
(2) ), as
, we get
for any large value of t. From the theory of differential inequality [Citation18], we obtain
This gives,
for large value of t.
Furthermore, from the fifth equation of system (Equation2(2)
(2) ), as
for large value of t, we get
From the theory of differential inequality [Citation18], we obtain
This yields that
for large time. Thus, we find that all solutions of system (Equation2
(2)
(2) ) enter into the region Ω, indicating that this region is an attracting set.
3.2. Disease-free equilibrium and its stability
The disease-free equilibrium corresponding to model system (Equation2(2)
(2) ) can be obtained by equating the right-hand side of system (Equation2
(2)
(2) ) to zero, when none of people in the considered domain is affected by the disease. For system (Equation2
(2)
(2) ), the disease-free equilibrium is
. Apparently, the equilibrium
always exists in the system. Now, we will establish the local stability of the equilibrium
in terms of the basic reproduction number (
), a potential measure that determines whether a specific disease can invade a population or not.
3.2.1. Basic reproduction number
In order to obtain an expression for the basic reproduction number () associated with system (Equation2
(2)
(2) ), we use the approach of the next generation matrix [Citation47]. To this, we find the transmission (new infection terms) and transition terms in the infected subsystem of system (Equation2
(2)
(2) ) as follows:
Thus, the transmission (new infection) and transition matrices corresponding to the equilibrium
are respectively obtained as
It has been well established that the basic reproduction number of an epidemic model is the spectral radius of the next generation matrix
, i.e.
. Thus, for model system (Equation2
(2)
(2) ), we get
(3)
(3)
Remark 3.1
Note that the expression in (Equation3(3)
(3) ) stands for the basic reproduction number associated with the model system (Equation2
(2)
(2) ) and represents the average number of secondary infections produced by a single infected person in a group of completely susceptible people. From the expression of
, the impacts of disease transmission through the direct contacts of susceptible with the infected individuals and with bacteria present in the environment are clearly visible. It expresses the facts that the disease spreads in the community through the direct as well as indirect routes of transmission. It is also apparent from the expression of
that reducing the net growth rate of bacteria in the environment can help in controlling the prevalence of the disease.
Following [Citation47], the local stability of the disease-free equilibrium of system (Equation2
(2)
(2) ) is stated in the following theorem.
Theorem 3.2
The disease-free equilibrium is always feasible. It is locally asymptotically stable if
and unstable if
.
Proof.
It can be easily proved by evaluating the Jacobian matrix of the system (Equation2(2)
(2) ) at the disease-free equilibrium
and finding the corresponding eigenvalues.
Regarding global stability of the disease-free equilibrium , we have the following theorem.
Theorem 3.3
The disease-free equilibrium of model system (Equation2
(2)
(2) ) is globally asymptotically stable in the region
of I−B plane if
.
Proof.
We obtain the following subsystem from the system (Equation2(2)
(2) ):
Consider,
and
. Since
for all I, B>0, therefore
Clearly,
does not change its sign and also it is not identically zero in the positive quadrant of the I−B plane. Thus, by the Bendixson–Dulac criterion [Citation50], system (Equation2
(2)
(2) ) has no limit cycle in the positive quadrant of the I−B plane. As disease-free equilibrium
is locally asymptotically stable whenever
, so it will be globally asymptotically stable in the region
of the I−B plane for
. A similar procedure can be used to prove the global stability of the disease-free equilibrium
in the other planes.
3.3. Endemic equilibrium and its stability
Let be an endemic equilibrium of the epidemic model (Equation2
(2)
(2) ), whose components are positive solutions of the system's equilibrium equations. From the second equilibrium equation of system (Equation2
(2)
(2) ), we have
(4)
(4)
From the third equilibrium equation of system (Equation2
(2)
(2) ), we get
(5)
(5)
Next, from the fifth equilibrium equation of system (Equation2
(2)
(2) ), we have
(6)
(6)
Using Equation (Equation6
(6)
(6) ) in the fourth equilibrium equation of system (Equation2
(2)
(2) ), we have
(7)
(7)
Note that the left-hand side (LHS) of the above equation is a decreasing whereas the right-hand side (RHS) is an increasing function of B. Thus, if there is a positive solution of Equation (Equation7
(7)
(7) ), then it is unique in the interval
. Let
from above, then the RHS of Equation (Equation7
(7)
(7) ) becomes zero whereas the LHS will be positive and finite for
This means, LHS>RHS. If
from below, then the RHS of Equation (Equation7
(7)
(7) ) becomes
whereas the LHS is positive and finite. In this case, LHS<RHS. Therefore, there exists a unique positive solution of Equation (Equation7
(7)
(7) ), say
in the interval
. We may rewrite Equation (Equation7
(7)
(7) ) as
(8)
(8)
where
On differentiating Equation (Equation8
(8)
(8) ) with respect to I, we get
Notably,
provided
. We can conclude from the above that
This yields that
Thus, we find that
and
. Notably,
Thus, for a positive value of S, we must have
Further, by using Equations (Equation4
(4)
(4) ), (Equation5
(5)
(5) ) and (Equation8
(8)
(8) ) in the first equilibrium equation of system (Equation2
(2)
(2) ), and noting that
is an appropriate positive solution of Equation (Equation7
(7)
(7) ), we get
(9)
(9)
The following properties of Equation (Equation9
(9)
(9) ) can be easily observed.
, which is positive provided
.
.
∀
.
Thus, there is a unique positive root (say ) of the equation
in the interval
whenever
. Putting
in Equations (Equation4
(4)
(4) )–(Equation7
(7)
(7) ), we can obtain the positive values of
,
,
and
. Thus, the equilibrium
exists in the system provided
and
.
Regarding local stability of the endemic equilibrium , we have the following theorem.
Theorem 3.4
The endemic equilibrium , if feasible, is locally asymptotically stable.
Proof.
The Jacobian matrix J at the endemic equilibrium becomes,
where
For the matrix
, we get the following characteristic equation:
(10)
(10)
If
,
is a root of Equation (Equation10
(10)
(10) ), then
Note that
Similarly, we can show that
Thus, the
of Equation (Equation10
(10)
(10) )
This shows that for
, Equation (Equation10
(10)
(10) ) does not possess any root with positive real part. Note that the inequality
holds provided
Obviously,
and
. Thus,
The fourth equilibrium equation of system (Equation2
(2)
(2) ) yields that
. This together with the above equation implies
. Thus, the
of Equation (Equation10
(10)
(10) )
The above discussions imply that Equation (Equation10
(10)
(10) ) does not have any solution with positive real part. Therefore, it can be concluded that the endemic equilibrium
, if exists, is locally asymptotically stable.
3.4. Direction of bifurcation at 

Theorem 3.2 indicates that there is an exchange of stability between equilibria and
at the critical value
. Thus, there is a possibility of transcritical bifurcation at
. To determine the nature of bifurcation, involving equilibrium
at
, we employ central manifold theory [Citation7].
Without loss of generality, we introduce the new variables ,
,
,
and
. With these, system (Equation2
(2)
(2) ) gets the following form:
(11)
(11)
Note that at
,
. The disease-free equilibrium associated with system (Equation11
(11)
(11) ) is
The linearized matrix corresponding to system (Equation11
(11)
(11) ) around the equilibrium
at
is obtained as
The above matrix has a simple eigenvalue zero and all other eigenvalues are negative at
.
If is the right eigenvector corresponding to the zero eigenvalue at
, then
Similarly, from the matrix
, we obtain a left eigenvector
(associated with the zero eigenvalue) with components as
Now, we compute the following quantities:
(12)
(12)
to get,
(13)
(13)
Now, we present the following theorem.
Theorem 3.5
Consider model system (Equation2(2)
(2) ), and let a and b be as in (Equation13
(13)
(13) ), where a<0 and b>0. The local dynamics of system (Equation2
(2)
(2) ) around the equilibrium
can be stated as: when
with
, the equilibrium
is locally asymptotically stable, and there exists a negative unstable equilibrium
. On the other hand, for
with
, the equilibrium
is unstable and there exists a positive locally asymptotically stable equilibrium
. This signifies that at
, system (Equation2
(2)
(2) ) showcases a supercritical (forward) bifurcation.
Proof.
The proof follows from Theorem 4.1 p. 373 and Remark 1 p. 375 in [Citation7].
The next theorem is for the global asymptotic stability of the endemic equilibrium .
Theorem 3.6
The endemic equilibrium , if feasible, is globally asymptotically stable in the region of attraction Ω provided,
(14)
(14)
(15)
(15)
Proof.
To establish the global stability of the endemic equilibrium , we consider a positive definite function as follows:
(16)
(16)
Here,
's
are some positive constants that will be determined later.
The time derivative of G along the solution of system (Equation2(2)
(2) ), for
and
is obtained as
Clearly, following inequalities ensure the negative definite of the derivative
inside the region Ω:
(17)
(17)
(18)
(18)
(19)
(19)
(20)
(20)
From inequalities (Equation17
(17)
(17) ) and (Equation18
(18)
(18) ), a positive value of
can be chosen if the inequality (Equation14
(14)
(14) ) holds. Also, from inequalities (Equation19
(19)
(19) ) and (Equation20
(20)
(20) ), we obtain the positive value of
in view of condition (Equation15
(15)
(15) ). Thus, the derivative
will be negative definite inside the region of attraction Ω if both the conditions (Equation14
(14)
(14) ) and (Equation15
(15)
(15) ) hold good.
4. Numerical simulations
Now, to support the analytically obtained results in the previous section and to get a better understanding of the behavior of system (Equation1(1)
(1) ), we perform some numerical simulations. Unless it is mentioned in the text, the parameter values utilized for the numerical observations of system (Equation1
(1)
(1) ) are the same as provided in Table .
Table 3. Values of parameters used for numerical simulations of system (Equation1(1)
(1) ).
4.1. Sensitivity analysis
With respect to some epidemiologically important model parameters, we calculate the basic reproduction number's normalized forward sensitivity indices following the method described in [Citation25]. The ratio of the relative change in a variable to the relative change in a parameter is given by the normalized forward sensitivity index of that variable to the parameter. For instance, the normalized forward sensitivity index of a variable γ that relies differentiably on a parameter δ is defined by . Figure depicts the normalized forward sensitivity indices of the basic reproduction number
with respect to some of the model parameters. The figure demonstrates that the value of
grows with an increment in the value of either of the parameters Λ, β, η,
and s as each of them has positive index with
. Apparently, the figure depicts that the model parameters L, ν and
have negative indices with
. So, increments in these model parameters can lead to a decline in the value of
. The figure also shows that the sensitivity indices of
with respect to the parameters Λ and ν are
and
, respectively. This represents the fact that 1% rise in the value of Λ (ν) will result in 1% increment (decrement) in the value of
. Importantly, a lesser value of
is preferable for control of the disease. This suggests to prevent increment in the values of parameters that have positive indices with
whereas parameters having negative indices with
should be encouraged. Hence, the policymakers must focus on the prevention strategies that reduce (enhance) the values of parameters having positive (negative) sensitivity indices with the basic reproduction number.
Figure 2. The normalized forward sensitivity indices of the basic reproduction number () with respect to the model parameters Λ, β, η, L, ν,
,
and s. Here, the values of parameters are chosen from Table .

It is worthy to note that the parameter values used for simulations of the mathematical models may have some errors as they are determined from experiments. To tame this uncertainty in the selection of model parameters, we perform global sensitivity analysis in system (Equation1(1)
(1) ) by implementing Latin hypercube sampling (LHS) and partial rank correlation coefficients (PRCCs) [Citation6, Citation24]. Note that LHS permits to differ several model parameters simultaneously in an efficient way, whereas PRCC correlates the model input parameters with the response function by giving values between
and 1. Here, we have taken the response function as the infected population (I). Notably, the signs of PRCCs indicate the type of correlations between the input parameters and the output variable while the values represent the strength of their influences. We run 1000 simulations per LHS by letting uniform distributions for each of the input parameter. The baseline values of parameters are taken from Table with
deviations, and the PRCC values are plotted as bar graphs in Figure . From the figure, we see that the model parameters Λ, β, η,
and s have positive correlations with the infected population in the epidemic region. The figure also depicts that the infected population is negatively correlated with the parameters L, ν and
. All the parameters with significant correlations with the infected population are marked by
on the respective bars. The sensitivity results indicate that boosting up the parameters with negative PRCCs with the infected population can help in reducing the epidemic burden. Besides, controlling the parameters with positive PRCC values can play an important role in the disease eradication.
Figure 3. The uncertainty of the model (Equation1(1)
(1) ) on infected individuals (I). Baseline values of parameters are the same as in Table except
. Significant parameters are marked by
(p-value
).

4.2. Impacts of some key parameters on disease control
We further explore the effects of the parameters , s and ν on the basic reproduction number (
). By varying
and ν simultaneously, we plot
as contour line in Figure . It is apparent from the figure that if the difference between
and s increases, the basic reproduction number decreases. We can see from the figure that for the lower values of
, the basic reproduction number (
) is greater than unity. However, if the difference
is above a certain value,
becomes less than unity, where the endemic equilibrium loses its feasibility and the disease-free equilibrium becomes feasible. It is also clear from the figure that for smaller values of ν, the value of
is higher and greater than one. But,
becomes less than unity after a certain range of ν. Moreover, we see from the figure that simultaneous increments in the values of ν and the difference
decrease the value of
, and push it back to a value smaller than one after certain ranges of these two. Notably, the disease will persist among the population for lower values of ν and
, but if their values increase,
becomes less than unity, and the endemicity will be lost in the population. Thus, it can be concluded from the figure that the prevalence of disease can be reduced by increasing the recovery rate of infected population and the decay rate of bacteria in the epidemic region. Importantly, attention must be paid on the reduction of the self growth rate of bacteria.
Figure 4. Contour plots of the basic reproduction number () as a functions of
and ν. Rest of the parameters are at the same values as in Table . In the figure, dashed green line stands for
. (For interpretation of the references to color in this figure legend, the reader is referred to the web version of this article.)

Now, we show the presence of forward bifurcation in system (Equation1(1)
(1) ). In view of expression (Equation3
(3)
(3) ), we first write the model parameter β in term of the basic reproduction number (
). Then, we vary
in the interval [0.01,2.5], and plot the equilibrium number of infected population in system (Equation1
(1)
(1) ), see Figure . In the figure, the blue line denotes the stable disease-free equilibrium (
), the red line represents unstable disease-free equilibrium (
) and the magenta line stands for the stable endemic equilibrium (
). The figure demonstrates that for
, system (Equation1
(1)
(1) ) settles at the stable disease-free steady state. But, as
exceeds the unit value, the endemic equilibrium
bifurcates into the positive quadrant and showcases stable configuration whereas the disease-free equilibrium loses its stability. This indicates that the disease-free and endemic equilibria of system (Equation1
(1)
(1) ) are interlinked via a transcritical bifurcation. This manifests that the epidemic will persist (or die out) in the population whenever
(or
). Thus, Figure numerically illustrates the analytical findings of Theorem 3.2.
Figure 5. Bifurcation diagram of system (Equation1(1)
(1) ) with respect to
. Parameters are at the same values as in Table except
, d = 0.004,
and
. Here, blue, red and magenta colours, respectively, denote the stable disease-free equilibrium (
), unstable disease-free equilibrium (
) and the stable endemic equilibrium (
). (For interpretation of the references to color in this figure legend, the reader is referred to the web version of this article.)

In Figures and , we simultaneously vary two parameters in model (Equation1(1)
(1) ), and see their impacts on the infected population and bacterial density in the epidemic region. Here, the pairs of parameters are taken as
,
,
and
. Indeed, these model parameters play a significant role in either spread or control, of the disease. In the figures, the two surfaces represent the maximum and minimum values of the variables on the simultaneous variations of these parameters. If two surfaces are visible in the pictures, then fluctuations occur in the equilibrium values of the populations while when the two surfaces collide, a stable situation is attained. The impacts of direct and indirect ways of transmissions of disease on the population levels are apparent from Figure . We can see in Figure (a) that for lower values of β, the equilibrium values of both infected and bacterial populations become zero. But, their equilibrium values increase as the value of β increases. Additionally, we see that after a certain value of β, the infected population attains a plateau. The parameter η possesses similar effect on the persistence of disease in the population (see Figure (b)). The figure depicts that up to a certain range of η, the disease wipes out from the population. However, the disease persists if the value of η exceeds a fixed value. The number of infected persons increases up to a certain range of η, and it attains a plateau as η surpasses a certain value. Figure (a) shows that by adding more disinfectants for the control of bacteria, the number of infected population can be reduced. Further, it shows that the number of infected persons rapidly decreases with an increase in the growth rate of chemicals due to increased bacterial density in the environment. Indeed, if the chemicals are being used at a high rate, then the bacteria population dies out from the environment and the disease will be under control. An increased growth rate of bacteria is dangerous for the human population because it causes a rapid incline in the number of infectives. This behaviour can be seen in Figure (b). Overall, Figures and demonstrate that the increased disease transmission rates due to direct and indirect routes lead to an accelerated growth in the epidemic size. But, in that case, controlling the bacterial population through the use of chemicals can play a pivotal role in the disease eradication.
Figure 6. Surface plots of the infective population (first column) and bacterial density (second column) with respect to (a) β and , and (b) η and
. Other parameters are at the same values as in Table .

Figure 7. Surface plots of the infective population (first column) and bacterial density (second column) with respect to (a) ϕ and , and (b)
and k. Other parameters are at the same values as in Table .

Now, we see the role of disinfectants in the control of disease in the population. To this, we plot the infective population (I) and the density of bacteria (B) for four different combinations of and
, Figure (a). It can be seen from the figure that the infective population and bacteria population attain high equilibrium values when
. But, for
and
, the epidemic curve gets reduced. We see further decrements in the infective population and the bacterial density for
and
. Moreover, we observe that for
and
, the infection wipes out from the community and the bacteria disappear from the environment. The figure showcases that the efficacy of chemicals to reduce the density of bacteria discharged by infected individuals plays a major role in the control of bacterial density and elimination of the disease. Finally, we see the impacts of chemicals in reducing the density of bacteria at the source discharged by the infected individuals. A variation plot for the infected individuals (I) and the bacterial density (B) in Figure (b) show that the disease persists in the population for
. However, the number of infectives and the bacterial density fall on increasing the values of k and
to 0.5 and 0.0001, respectively. A comparatively more decrease in the infected population can be seen from the figure on increasing the value of
to 0.01. Importantly, the figure shows that both the infective and bacteria populations can be completely controlled for
. Thus, one can say that the disease can be totally controlled by enhancing the death of bacteria through the use of disinfectants.
Figure 8. Variations in the infective population (first column) and bacterial density (second column) with respect to time for different combinations of (a) and
, and (b) k and
. Rest of the parameters are at the same values as in Table except
.

Figure illustrates the global stability of the disease-free equilibrium in two different spaces. For this figure, we have chosen
, d = 0.004,
and
while the values of other parameters are taken from Table . For this parametric set-up, we obtain the value of basic reproduction number as
. In Figure (a), we observe that the solution trajectories of system (Equation1
(1)
(1) ) starting from four distinct values converge to the components of the disease-free equilibrium
in I−R−B space. Likewise, Figure (b) demonstrates the global stability of the disease-free equilibrium
in
space. The figures suggest that for such parametric values, the disease can be wiped out from the population for a longer period of time.
Figure 9. Figure illustrating the global asymptotic stability of disease-free equilibrium in (a) I−R−B and (b)
spaces. Parameters are at the same values as in Table except
, d = 0.004,
and
. Rest of the parameters are at the same values as in Table .

For the endemic equilibrium , the global stability is illustrated in Figure . For this figure, we take the values of parameters from Table for which
. Figure (a) clearly demonstrates that solution trajectories of system (Equation1
(1)
(1) ) starting within the region of attraction Ω converge to the components of the equilibrium
in
space. Similarly, we observe in Figure (b) that the solution trajectories of system (Equation1
(1)
(1) ) originating in the region Ω ultimately converge to the components of the endemic equilibrium
in I−R−B space. The global stability of the equilibrium point
can also be shown in other spaces using phase diagrams. Thus, the analytical result of Theorem 3.6 is justified in Figure . Thus, one can say that for such values of model parameters, the disease persists among the population.
5. Conclusion
Here, we have explored the impact of chemicals in controlling the diseases that spread in the population due to direct contacts between infective and the susceptible individuals, and also via bacteria present in the environment. The model equations described the time evolutions of susceptible human population, infected human population, recovered human population, bacteria present in the environment and chemical concentration of disinfectants/sanitizers. Mathematically, we showed that the proposed model exhibits disease-free and endemic equilibria which are connected via a forward bifurcation. We observed that for , system (Equation2
(2)
(2) ) exhibits a globally stable disease-free equilibrium. On the other hand, a unique endemic equilibrium exists in the system for
. Moreover, sufficient conditions are derived under which the endemic equilibrium is globally asymptotically stable. These results advise to focus on some control strategies that lower the epidemic threshold below unity. This can be achieved by controlling the rate of immigration into the epidemic region and transmissions of disease due to direct/indirect contacts of the susceptible individuals with the infected ones and the bacteria. Importantly, accelerating the recovery rate of infected individuals and the death rate of bacteria present in the environment can be effective ways for controlling the disease.
We have implemented some sophisticated simulation techniques to discover the influences of some epidemiologically important model parameters on the disease dynamics. Our sensitivity results showed that the parameters Λ, β, η, and s have positive correlations with the infected individuals. Thus, increments in the values of these parameters can boost up the disease prevalence. On the other hand, the parameters L, ν and
have negative influences on the infective cases. These results indicate that controlling the transmission rates of disease due to the contacts between susceptibles and infectives, and via bacteria present in the environment can help in reducing the disease prevalence. At the same time, fostering the rate of recovery and the natural death rate of bacteria can play significant roles in disease eradication. Additionally, we found that controlling the bacterial population at the sources discharged by the infected persons significantly reduces the epidemic size. Our simulation results showed the importance of disinfectants in the control of disease among the human population. The results evoked that the disinfectants of high efficacy can help in controlling the bacterial density and thereby eradicate the disease from the region of epidemic outbreak. Overall, the findings of this study suggest that using disinfectants of high quality can play a crucial role in the control of bacterial diseases in the human population.
Acknowledgments
All the authors express their gratitude to the associate editor and the reviewers whose comments and suggestions have helped in improving this paper.
Disclosure statement
No potential conflict of interest was reported by the author(s).
Additional information
Funding
References
- A. Abate, A. Tiwari, and S. Sastry, Box invariance in biologically-inspired dynamical systems, Automatica 45(7) (2009), pp. 1601–1610.
- M. Aguiar, V. Anam, K.B. Blyuss, C.D.S. Estadilla, B.V. Guerrero, D. Knopoff, B.W. Kooi, A.K. Srivastav, V. Steindorf, and N. Stollenwerk, Mathematical models for dengue fever epidemiology: A 10-year systematic review, Phys. Life Rev. 40 (2022), pp. 65–92. doi:10.1016/j.plrev.2022.02.001
- M. Ali, A.R. Nelson, A.L. Lopez, and D.A. Sack, Updated global burden of cholera in endemic countries, PLoS Negl. Trop. Dis. 9(6) (2015), p. e0003832.
- R.M. Anderson and R.M. May, Population biology of infectious diseases: Part I, Nature 280(5721) (1979), pp. 361–367.
- J.K.K. Asamoah, E. Okyere, A. Abidemi, S.E. Moore, G.Q. Sun, Z. Jin, E. Acheampong, and J.F. Gordon, Optimal control and comprehensive cost-effectiveness analysis for COVID-19, Results Phys. 33 (2022), p. 105177.
- S.M. Blower and H. Dowlatabadi, Sensitivity and uncertainty analysis of complex models of disease transmission: An HIV model, as an example, Int. Stat. Rev. 62(2) (1994), pp. 229–243.
- C. Castillo-Chavez and B. Song, Dynamical models of tuberculosis and their applications, Math. Biosci. Engrg. 1(2) (2004), pp. 361–404.
- Cholera: Scourge of the poor. Available at http://www.infoplease.com/cig/dangerous-diseases-epidemics/cholera-scourge-poor.html, 2013.
- J. Cui, Z. Wu, and X. Zhou, Mathematical analysis of a cholera model with vaccination, J. Appl. Math. 2014 (2014), p. 324767.
- B. Dubey, P. Dubey, and U.S. Dubey, Role of media and treatment on an SIR model, Nonlinear Anal. Model. Control 21(2) (2016), pp. 185–200.
- S. Funk, E. Gilad, C. Watkins, and V.A.A. Jansen, The spread of awareness and its impact on epidemic outbreaks, Proc. Natl. Acad. Sci. 106(16) (2009), pp. 6872–6877.
- M. Ghosh, P. Chandra, P. Sinha, and J.B. Shukla, Modelling the spread of bacterial disease: effect of service providers from an environmentally degraded region, Appl. Math. Comput. 160(3) (2005), pp. 615–647.
- S. Gopinath, S. Carden, and D. Monack, Shedding light on salmonella carriers, Trends Microbiol. 20(7) (2012), pp. 320–327.
- H.W. Hethcote, The mathematics of infectious diseases, SIAM Review 42(4) (2000), pp. 599–653.
- S.D. Hove-Musekwa, F. Nyabadza, C. Chiyaka, P. Das, A. Tripathi, and Z. Mukandavire, Modelling and analysis of the effects of malnutrition in the spread of cholera, Math. Comput. Model 53(9–10) (2011), pp. 1583–1595.
- M.J. Keeling and L. Danon, Mathematical modelling of infectious diseases, Br. Med. Bull 92(1) (2009), pp. 33–42.
- I.Z. Kiss, J. Cassell, M. Recker, and P.L. Simon, The impact of information transmission on epidemic outbreaks, Math. Biosci. 225(1) (2010), pp. 1–10.
- V. Lakshmikantham, S. Leela, and A.A. Martynyuk, Stability Analysis of Nonlinear Systems, M. Dekker, New York, 1989.
- K. Lata, A.K. Misra, and Y. Takeuchi, Modeling the effectiveness of TV and social media advertisements on the dynamics of water-borne diseases, Int. J. Biomath. 15(02) (2022), p. 2150069.
- Y. Liu and J.A. Cui, The impact of media coverage on the dynamics of infectious disease, Int. J. Biomath. 01(01) (2008), pp. 65–74.
- X. Lu, S. Wang, S. Liu, and J. Li, An SEI infection model incorporating media impact, Math. Biosci. Eng. 14(5/6) (2017), pp. 1317–1335.
- X. Ma, X.F. Luo, L. Li, Y. Li, and G.Q. Sun, The influence of mask use on the spread of COVID-19 during pandemic in New York city, Results Phys. 34 (2022), pp. 105224.
- M. Majumder, P.K. Tiwari, and S. Pal, Impact of saturated treatments on HIV-TB dual epidemic as a consequence of COVID-19: Optimal control with awareness and treatment, Nonlinear Dyn. 109(1) (2022), pp. 143–176. doi:10.1007/s11071-022-07395-6
- S. Marino, I.B. Hogue, C.J. Ray, and D.E. Kirschner, A methodology for performing global uncertainty and sensitivity analysis in systems biology, J. Theor. Biol. 254(1) (2008), pp. 178–196.
- M. Martcheva, An Introduction to Mathematical Epidemiology, Springer, New York, 2015.
- M. Massard, R. Eftimie, A. Perasso, and B. Saussereau, A multi-strain epidemic model for COVID-19 with infected and asymptomatic cases: application to French data, J. Theor. Biol. 545 (2022), pp. 1–17.
- A.K. Misra, S.N. Mishra, A.L. Pathak, P. Misra, and R. Naresh, Modeling the effect of time delay in controlling the carrier dependent infectious disease – cholera, Appl. Math. Comput. 218(23) (2012), pp. 11547–11557.
- A.K. Misra, S.N. Mishra, A.L. Pathak, P.K. Srivastava, and P. Chandra, A mathematical model for the control of carrier-dependent infectious diseases with direct transmission and time delay, Chaos Solit. Fract. 57 (2013), pp. 41–53.
- A.K. Misra, R.K. Rai, and Y. Takeuchi, Modeling the control of infectious diseases: Effects of TV and social media advertisements, Math. Biosci. Eng. 15(6) (2018), pp. 1315–1343.
- A.K. Misra and R.K. Rai, A mathematical model for the control of infectious diseases: Effects of TV and radio advertisements, Int. J. Bifurcat. Chaos 28(03) (2018), pp. 1850037.
- A.K. Misra and R.K. Rai, Impacts of TV and radio advertisements on the dynamics of an infectious disease: A modeling study, Math. Meth. Appl. Sci. 42(4) (2019), pp. 1262–1282.
- A.K. Misra and V. Singh, A delay mathematical model for the spread and control of water-borne diseases, J. Theor. Biol. 301 (2012), pp. 49–56.
- S.S. Musa, S. Zhao, N. Hussaini, S. Usaini, and D. He, Dynamics analysis of typhoid fever with public health education programs and final epidemic size relation, Results Appl. Math. 10 (2021), pp. 100153.
- A. Mwasa and J.M. Tchuenche, Mathematical analysis of a cholera model with public health interventions, Biosystems 105(3) (2011), pp. 190–200.
- R. Naresh, S. Pandey, and J.B. Shukla, Modeling the cumulative effect of ecological factors in the habitat on the spread of tuberculosis, Int. J. Biomath. 02(03) (2009), pp. 339–355.
- R.K. Rai, A.K. Misra, and Y. Takeuchi, Modeling the impact of sanitation and awareness on the spread of infectious diseases, Math. Biosci. Eng. 16(2) (2019), pp. 667–700.
- R.K. Rai, P.K. Tiwari, Y. Kang, and A.K. Misra, Modeling the effect of literacy and social media advertisements on the dynamics of infectious diseases, Math. Biosci. Eng. 17(5) (2020), pp. 5812–5848.
- R.K. Rai, S. Khajanchi, P.K. Tiwari, E. Venturino, and A.K. Misra, Impact of social media advertisements on the transmission dynamics of COVID-19 pandemic in India, J. Appl. Math. Comput. 68 (2022), pp. 19–44.
- J.B. Shukla, V. Singh, and A.K. Misra, Modeling the spread of an infectious disease with bacteria and carriers in the environment, Nonlinear Anal. Real World Appl. 12(5) (2011), pp. 2541–2551.
- J.B. Shukla, R. Naresh, S.R. Verma, and M. Agarwal, Modeling the effect of sanitation in a human habitat to control the spread of bacterial diseases, Model. Earth Syst. Environ. 6(1) (2020), pp. 39–49.
- S. Singh, P. Chandra, and J.B. Shukla, Modeling and analysis of the spread of carrier dependent infectious diseases with environmental effects, J. Biol. Syst. 11(03) (2003), pp. 325–335.
- G.Q. Sun, H.T. Zhang, L.L. Chang, Z. Jin, H. Wang, and S. Ruan, On the dynamics of a diffusive foot-and-mouth disease model with nonlocal infections, SIAM J. Appl. Math. 82(4) (2022), pp. 1587–1610.
- H.R. Thieme, Mathematics in population biology, Princeton University Press, Princeton, 2018.
- P.K. Tiwari, R.K. Rai, S. Khajanchi, R.K. Gupta, and A.K. Misra, Dynamics of coronavirus pandemic: Effects of community awareness and global information campaigns, Eur. Phys. J. Plus. 136(10) (2021), pp. 994.
- P.K. Tiwari, R.K. Rai, A.K. Misra, and J. Chattopadhyay, Dynamics of infectious diseases: Local versus global awareness, Int. J. Bifurcat. Chaos 31(07) (2021), pp. 2150102.
- P.K. Tiwari, R.K. Rai, R.K. Gupta, M. Martcheva, and A.K. Misra, Modeling the control of bacterial disease by social media advertisements: Effects of awareness and sanitation, J. Biol. Syst. 30(01) (2022), pp. 51–92.
- P. van den Driessche and J. Watmough, Reproduction numbers and sub-threshold endemic equilibria for compartmental models of disease transmission, Math. Biosci. 180(1-2) (2002), pp. 29–48.
- World Health Statistics 2018, Monitoring health for the SDGs (sustainable development goals), World Health Organization, Geneva, 2018.
- WHO/UNICEF, JMP Sanitation Data 2019. Available at https://washdata.org/data/household/.
- S. Wiggins, Introduction to Applied Nonlinear Dynamical Systems and Chaos, Springer, New York, 2003.
- Y. Zhao, M. Li, and S. Yuan, Analysis of transmission and control of tuberculosis in mainland China, 2005–2016, based on the age-structure mathematical model, Int. J. Environ. Res. Public Health. 14(10) (2017), p. 1192.