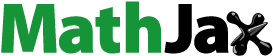
Abstract
A stochastic mathematical model is proposed to study how environmental heterogeneity and the augmentation of mosquitoes with bacteria affect the outcomes of dengue disease. The existence and uniqueness of the positive solutions of the system are studied. Then the V-geometrically ergodicity and stochastic ultimate boundedness are investigated. Further, threshold conditions for successful population replacement are derived and the existence of a unique ergodic steady-state distribution of the system is explored. The results show that the ratio of infected to uninfected mosquitoes has a great influence on population replacement. Moreover, environmental noise plays a significant role in control of dengue fever.
1. Introduction
Every year many people are infected with mosquito-borne diseases including dengue fever and dengue haemorrhagic fever, caused by dengue viruses. Dengue infections are one of the main reasons for illness in the tropics and subtropics [Citation21]. Due to the lack of licensed vaccines or drugs, the most effective way to treat dengue is to control its mosquito vectors, but traditional insecticide spraying not only pollutes the environment but also causes insecticide resistance [Citation14]. Many studies have demonstrated that releases of mosquitoes carrying the endosymbiotic bacteria Wolbachia provide a novel method to control dengue [Citation4, Citation19]. This succeeds because cytoplasmic incompatibility (CI) is induced in the mosquitoes with Wolbachia, which causes early embryonic death when uninfected females mate with infected males, but this does not affect infected females, resulting in widespread distribution of the bacteria in nature [Citation6, Citation16].
Based on the mechanisms of CI, two strategies including population suppression and population replacement have been proposed to control mosquitoes [Citation5], with population suppression being realized by releasing -infected males inundatively and population replacement being achieved when
-infected mosquitoes are released inoculatively [Citation22, Citation34]. Recently, researchers in many laboratories around the world are trying to control dengue virus by releasing
-infected mosquitoes with some success [Citation17, Citation33]. Many different types of mathematical models have been proposed to study the transmission dynamics between wild and
-infected mosquitoes, including discrete time models [Citation11, Citation13], continuous time models [Citation10, Citation20] and impulsive differential equation models [Citation37, Citation38]. For example, Haygood and Turelli established a discrete model to analyse the impact of host population subdivision on the evolution of CI-causing bacteria strains in specific host species, its results showed that in the subdivided host population with local density regulation, the strain evolution tended to be stronger CI [Citation13]. Farkas and Hinow introduced and studied some differential equation models of population dynamics of
infection, and they found that under the condition of mutual compatibility, strains with higher transmission efficiency or lower infection mortality are superior to competitors [Citation10]. Zhang et al. proposed the birth-pulse model of
transmission through mosquito population and reached the conclusion that population eradication can be achieved only when the parameters lie in a specific regions and the initial density of non-infection is low enough, regardless of the infection ratio [Citation37].
However, wild mosquitoes and the released -infected mosquitoes in nature are inevitably influenced by environmental fluctuations including temperature, wind, rainfall, oxygen and so on [Citation18, Citation32]. These environmental factors affect the breeding, growth and development of mosquitoes: (1) the larval indices in the wet season is greater than that in the dry season [Citation7, Citation31], (2) higher temperature will hasten mosquitoes development [Citation8]. Relevant research indicates that the synergistic effect of between temperature and precipitation may have a significant impact on mosquitoes ecology and mosquito-borne diseases [Citation1]. Indeed, the population dynamics of dengue vector mosquitoes are strongly linked with temperature and rainfall fluctuations [Citation36]. The significant changes that this fluctuation causes to the mosquito population, stochastic differential equations with white noises provide a more realistic description.
Therefore, in this paper, we propose a mathematical model comprising a system of stochastic differential equations, governing the evolution of mosquitoes with white noises, then derive threshold conditions for population replacement and study the ergodic steady-state distribution of the system.
2. Model formation and preliminaries
Throughout this paper, the total population of mosquitoes is denoted by and we assume that the mosquito population is infected by a single strain of
. The transmission can only be passed from infected females to their offspring, but transmission is imperfect with probability
.
can be subdivided into four subpopulations, namely uninfected females,
, infected females,
, uninfected males,
, and infected males,
. b and d are the density dependent birth rate and death rate for the mosquito population, respectively. f is the proportion of females in the offspring. When an infected male mates with an uninfected female, zygotic death of offspring caused by CI usually occurs with a probability
. Although CI has a beneficial effect on infected females, a fitness cost effect D of mosquitoes infected with
is assumed to be non-zero with D>0 being fitness cost and D<0 being fitness benefit, and the sign of D depends on the mosquito species and
strains. Then the model with overlapping generations can be described as follows:
(1)
(1) In reality, the proportion of infected males that mate with infected females is usually the same as in uninfected populations, i.e. we have
, so after one or two generations, the ratio of males to females is identical in both cases. However, modifications may be considered if the sex ratios change [Citation15]. Therefore, the entire infected (
) and uninfected (
) populations are introduced to simplify system (Equation1
(1)
(1) ) [Citation10, Citation20], with suitable parameters the model is rescaled to
(2)
(2) Many studies have focused on the model (Equation2
(2)
(2) ) [Citation10, Citation20, Citation39], not only providing threshold conditions for the existence and stability of all possible equilibria but also discussing the biological significance regarding mosquito population replacement. But the effects of environmental heterogeneity on the dynamics of
spread have been ignored. All organisms in natural habitats are constrained by fluctuations of many environmental factors such as temperature, nutrition, oxygen, pH and so on [Citation32], and mosquitoes are no exception. Hu and co-authors constructed a mathematical model to study how random switches in birth rates affect the dynamics of
spread [Citation18]. Hence, we introduce white noise to study the influences of stochastic perturbations and assume that they are directly proportional to the entire infected
and uninfected
populations. Some authors (see [Citation23, Citation24]) have pointed out that this assumption is reasonable and well justified biologically. Because the specific
can not only be successfully transmitted in mosquito populations but also act like a vaccine to stop mosquitoes from replicating and spreading dengue virus, augmentation of mosquitoes with
bacteria has been used to realize the aims of population replacement, and to prevent the occurrence of diseases, such as dengue disease [Citation17, Citation34]. Therefore, we proposed a stochastic system (Equation2
(2)
(2) ) with control to model the effects of environmental fluctuations and the augmentation of mosquitoes, and system (Equation2
(2)
(2) ) is modified as
(3)
(3) where θ is the quantity of mosquitoes infected with
being continuously released.
and
denote independent Brownian motion defined in a complete probability space
,
and
are the intensities of the noise on the entire infected
and uninfected
populations, respectively.
Throughout the paper, is denoted as a complete probability space with filtration
and satisfies: (a) right continuous and (b)
involves all
-null sets. The independent Brownian motion
is defined on this probability space. Assume that
is an
-measurable
-valued random variable, where
. Define functions
and
such that they are Borel measurable. Consider the following Itô-type stochastic differential equation
(4)
(4) and the equivalent system of (Equation4
(4)
(4) ) is
(5)
(5) Let
be the transition probability, and
for any
,
and
.
Then we give some important definitions as follows [Citation12, Citation25, Citation26, Citation35].
Definition 2.1
[Citation26]
Let be a solution of (Equation3
(3)
(3) ) provided
{X(t)} is continuous and
-adapted;
and
;
for any
(Equation4
(4)
(4) ) holds with probability 1.
Definition 2.2
[Citation12, Citation25]
Let be a solution of SDE (Equation3
(3)
(3) ):
if
, then
becomes extinctive;
if
, then
becomes weakly persistent;
if for any
, there are two constants
and
such that
Lemma 2.1
[Citation35]
Let if there are constants
and
such that
satisfies
for any
,
is also a constant, then
; if there are constants
and
such that
satisfies
for any
, then
.
3. Main results
3.1. Properties of the solutions
For any given initial conditions, a unique global solution of system (Equation3(3)
(3) ) exists if its coefficients satisfy the linear growth condition and local Lipschitz condition [Citation26]. Now, we show that the solution of system (Equation3
(3)
(3) ) is positive and global by using methods of Lyapunov analysis [Citation9].
Theorem 3.1
Let be a solution of SDE (Equation3
(3)
(3) ) with initial condition
, then
is unique for any
and it further remains in
with probability 1, namely
for any
almost surely.
Proof.
Let and
, applying Itô's formula to system (Equation3
(3)
(3) ) yields
(6)
(6) where initial conditions are
and
. Note that the coefficients of system (Equation6
(6)
(6) ) are locally Lipschitz continuous, there is a blow up time
such that system (Equation6
(6)
(6) ) exists with a unique local solution
on
. It follows from Itô's formula that
is just the unique local solution of system (Equation3
(3)
(3) ) on
. To show the solution
is global we only need to prove
. Let
big enough for
and
lying within the interval
. For any integer
, define the stopping time as
Let
be empty set, and set
.
increases as
. Let
, clearly,
. For any
, if
a.s., then we have
and
. Therefore, what we only need to do is to show
. Otherwise, there exists with two constants T>0 and
so that
. Consequently, there is an integer
such that
(7)
(7) Define a
-function
as
and
is positive for
. Making use of Itô's formula, then
where
where C is a positive constant and independent of I, U and t. Thus
Set
, then we obtain
calculating the mathematical expectation of the above inequality yields
(8)
(8) For any
, let
. From (Equation7
(7)
(7) ) we have
. For any
, there is at least one
or
equalling n or
, thereby,
Combinations of (Equation7
(7)
(7) ) and (Equation8
(8)
(8) ) lead to
with
denoting as the indicator function of
. Once
, we obtain
which is a contradiction. Therefore,
. This completes the proof.
Theorem 3.1 indicates that any solutions of SDE (Equation3(3)
(3) ) will finally remain in a compact set of
. In the following, we prove that the solutions of SDE (Equation3
(3)
(3) ) satisfy another important property, i.e. the Markov process
is V-geometrically ergodic.
Theorem 3.2
Let initial condition , if
and
, then Markov process
is V-geometrically ergodic.
Proof.
Note that N = I + U, define a function such that
as
for
, where
(9)
(9) Applying Itô's formula yields
(10)
(10) where
Hence the Lyapunov condition holds, for details, see [Citation28].
Note that when and
, then SDE (Equation3
(3)
(3) ) is uniformly elliptic [Citation3]. Athreya et al. pointed out that there is a jointly continuous function defined as
. For all
,
is strictly positive so that for all measure sets A we obtain
For any w>0, there is a positive constant
such that
. Thus for any measurable set A, we have
where Leb is Lebesgue measure and
. So the Minirization condition holds. This completes the proof.
Theorem 3.3
For any initial value , the solution
of system (Equation3
(3)
(3) ) is stochastically ultimately bounded and permanent.
Proof.
Clearly, N = I + U, define a Lyapunov function and choose a small enough ξ such that
. Making use of Itô's formula, it follows from (Equation10
(10)
(10) ) that we obtain
Integrating the above equation from 0 to t and taking mathematical expectation, then
Thus
From Markov inequality, choose a positive constant
large enough so that
,
Consequently,
In view of
, so
It follows from the definitions that system (Equation3
(3)
(3) ) is stochastically ultimately bounded and permanent. This ends the proof.
3.2. Population replacement
One of the feasible measures to prevent dengue diseases from spreading is to achieve population replacement, by means of releasing mosquitoes with . This section will focus on the conditions for population replacement. To this end, for simplicity we denote
Note that
, then we have
, which means that
. Based on this fact, we have the following main results.
Theorem 3.4
Theorem 3.4 If , then the total uninfected mosquitoes become extinct.
Proof.
According to system (Equation3(3)
(3) ), defining a Lyapunov function
, applying Itô's formula to the second equation of system (Equation3
(3)
(3) ) and integrating the above equation from 0 to t we obtain
(11)
(11) where
. Because of
and the strong law of large numbers for local martingales we obtain
(12)
(12) When
, taking the superior limit of equation (Equation11
(11)
(11) ) and by using L'Hospital's rule we have
So
, which indicates that the total uninfected mosquitoes become extinct. This completes the proof.
Remark 3.1
Notice that the threshold conditions of Theorem 4 determine the outcomes of population replacement, it implies that the ratio of infected to uninfected mosquitoes and environmental noise play significant roles in control of dengue fever.
For simplicity, denoteFigure 1. Extinction of the uninfected mosquitoes. (a) ; (b)
. All other parameter values were fixed as: q = 0.2,
, b = 0.02, d = 0.02, D = 0.01,
and initial value

Since is very sensitive to key parameters, it is critical to conduct sensitivity analysis. In Figure , two cases for
and k are discussed respectively. If fixing parameter values as shown in Figure (a), it can be found that
increases with the increase of k. For
, a small value of k can ensure that
, which indicates that uninfected mosquitoes are extinct. For
, it can be observed that
, implying uninfected mosquitoes continue to exist. If fixing parameter values as shown in Figure (b), it is noted that
decreases from
to
when
increases. Obviously, for k = 0.1,
reaches
faster. In general, the smaller k and the larger
will hasten to the extinction of uninfected mosquitoes.
Figure 2. These plots show that sensitivity of on
. (a) We set
and
; (b) We set k = 0.1 and k = 0.8, and all other parameter values were fixed as:
, b = 0.1, q = 0.2.

Through the above discussion, it is not difficult to find that increasing θ (i.e. the release of -infected mosquitoes) can increase k (i.e. the ratio of
-infected mosquitoes and uninfected mosquitoes). Because a small k can ensure the rapid extinction of mosquitoes, it suggests that when using the ‘mosquito control’ strategy, it is necessary to choose an appropriate threshold for the release of
-infected mosquitoes.
3.3. Stationary distribution and ergodicity for the system
In this section, we explore the existence of a unique ergodic steady-state distribution of the system (Equation6(6)
(6) ). If f is a bounded function on
, defining
, then we only need to show that the following two properties hold true [Citation27, Citation40],
there exists a bounded domain
with regular boundary Γ such that its closure
, and a non-negative
function
exists such that for any
, LV is negative;
for any bounded domain
there is a positive constant ζ such that the diffusion matrix for system (Equation3
(3)
(3) ) given by
satisfies
for all
, and
Theorem 3.5
If
(13)
(13) then system (Equation3
(3)
(3) ) has a unique ergodic stationary distribution.
Proof.
Let
where
is a sufficiently small constant. Take
such that
then by use of Itô's formula on V yields
where
where M is an upper bound which satisfies
and
due to the solution
of system (Equation3
(3)
(3) ) being stochastically ultimately bounded.
It is easy to obtain that
where
Case 1. If
, then
If
, then
Case 2. If
, then
If
, then
In conclusion, when
or
or
or
, we can get
. Hence, choose
sufficiently small and let
, then
which means that the condition (1) holds true. Furthermore,
and thus condition (2) has also been satisfied. Therefore, the system (Equation3
(3)
(3) ) has a unique ergodic stationary distribution (Figure ). This completes the proof.
Figure 3. Stationary distribution of deterministic model and stochastic model: (a) we set initial values as ; (b) we set initial values as
. The initial values of the solution illustrated by the black line were fixed as
, and all other parameters were fixed as:
, b = 0.1, d = 0.1, D = 0.01,
, q = 0.2, q = 0.3.

4. Conclusion
Many researchers are designing programmes to release -infected mosquitoes to control dengue virus, a promising strategy that has attracted the attention of many mathematical researchers. Given that mosquitoes in natural habitats are inevitably affected by environmental fluctuations [Citation18, Citation32, Citation36], we developed a mathematical model comprising a system of stochastic differential equations, governing the evolution of mosquitoes with white noise.
We first prove the existence and uniqueness of the positive solutions of the proposed system. Then we study the V-geometrical ergodicity and stochastic ultimately boundedness of the system. Further, threshold conditions for successful population replacement are derived, and it is shown that the system has a unique ergodic steady-state distribution. The results show that the ratio of infected to uninfected mosquitoes has a great influence on population replacement. Moreover, environmental noise plays a significant role in control of dengue fever.
The highlights are listed as follows: (1) the proposed model considers not only the influence of white noise but also introduces the continuously released -infected mosquitoes; (2) the threshold conditions for the extinction of uninfected mosquitoes and the system's stationary distribution are obtained; (3) biologically, by increasing the release of
-infected mosquitoes, resulting in an increase in the ratio of
-infected mosquitoes and uninfected mosquitoes, and then reaching a suitable value, which can promote the rapid extinction of uninfected mosquitoes.
In fact, some studies have shown that different strains have different biological characteristics, which are different in reducing the incidence rate of dengue fever [Citation2, Citation29, Citation30]. For example, the
Au strain has a high virus blocking rate, while the
Mel strain has only a moderate level, but both strains have high maternal transmission rates. In addition, the loss of
infection is lower in the
Au strain and higher in the
Mel strain. Finally, the
Au strain does not exhibit cytoplasmic incompatibility, while
Mel exhibits cytoplasmic incompatibility. Therefore, further research on the impact of environmental factors on the effectiveness of different
strains may add new perspectives, which will also become our future research direction.
Acknowledgments
The author is very grateful to the anonymous referee for a careful reading, helpful suggestions and valuable comments which led to the improvement of the manuscript.
Disclosure statement
No potential conflict of interest was reported by the author(s).
Additional information
Funding
References
- E.F. Andongma, S.A. Forchu, B.T. Andongma, and B.K. Gana, Impact of environmental changes on mosquitoes and disease transmission, J. Nat. Sci. Res. 10 (2020), pp. 35–40.
- T.H. Ant, C.S. Herd, V. Geoghegan, A.A. Hoffmann, and S.P. Sinkins, The Wolbachia strain wAu provides highly efficient virus transmission blocking in Aedes aegypti, Plos Pathog. 14(1) (2018), pp. e1006815.
- A. Athreya, T. Kolba, and J.C. Mattingly, Propagating Lyapunov functions to prove noise-induced stabilization, Electron. J. Probab. 17(96) (2012), pp. 1–38.
- B.J. Beaty, Genetic manipulation of vectors: a potential novel approach for control of vector-borne diseases, Proc. Natl. Acad. Sci. 97(19) (2000), pp. 10295–10297.
- J.J. Bull and M. Turelli, Wolbachia versus dengue: evolutionary forecasts, Evol. Med. Public Health. 2013(1) (2013), pp. 197–207.
- E. Caspari and G. Watson, On thee volutionary importance of cytoplasmic sterility in mosquitoes, Evolution 13(4) (1959), pp. 568.
- Q. Cheng, Q. Jing, R.C. Spear, J.M. Marshall, Z. Yang, and P. Gong, Climate and the timing of imported cases as determinants of the dengue outbreak in Guangzhou 2014: evidence from a mathematical model, PLoS Negl. Trop. Dis. 10(2) (2016), pp. e0004417.
- J. Couret and M.Q. Benedict, A meta-analysis of the factors influencing development rate variation in Aedes aegypti (Diptera: Culicidae), BMC Ecol. 14(1) (2014), pp. 3.
- D. Dalal, D. Greenhalgh, and X. Mao, A stochastic model of AIDs and condom use, J. Math. Anal. Appl. 325(1) (2007), pp. 36–53.
- J.Z. Farkas and P. Hinow, Structured and unstructured continuous models for Wolbachia infections, Bull. Math. Biol. 72(8) (2010), pp. 2067–2088.
- P.E.M. Fine, On the dynamics of symbiote-dependent cytoplasmic incompatibility in culicine mosquitoes, J. Invert. Path. 31(1) (1978), pp. 10–18.
- T.G. Hallam and Z.E. Ma, Persistence in population models with demographic fluctuations, J. Math. Biol. 24(3) (1986), pp. 327–339.
- R. Haygood and M. Turelli, Evolution of incompatibility-inducing microbes in subdivided host populations, Evolution 63(2) (2009), pp. 432–447.
- J. Hemingway and H. Ranson, Insecticide resistance in insect vectors of human disease, Annu. Rev. Entomol. 45(1) (2000), pp. 371–391.
- W.A. Hickey and G.B. Craig, Genetic distortion of sex ratio in a mosquito Aedes aegypti, Genetics53(6) (1966), pp. 1177–1196.
- A.A. Hoffmann, M. Turelli, and L.G. Harshman, Factors affecting the distribution of cytoplasmic incompatibility in Drosophila simulans, Genetics 126(4) (1990), pp. 933–948.
- A.A. Hoffmann, B.L. Montgomery, J. Popovici, I. Iturbe-Ormaetxe, P.H. Johnson, F. Muzzi, M.Greenfield, M. Durkan, Y.S. Leong, Y. Dong, and H. Cook, Successful establishment of Wolbachia in Aedes populations to suppress dengue transmission, Nature 476(7361) (2011), pp. 454–457.
- L.C. Hu, M.G. Huang, M.X. Tang, J.S. Yu, and B. Zheng, Wolbachia spread dynamics in stochastic environments, Theor. Popul. Biol. 106 (2015), pp. 32–44.
- S. James, C.P. Simmons, and A.A. James, Ecology: mosquito trials, Sciences 334(6057) (2011), pp. 771–772.
- M.J. Keeling, F.M. Jiggins, and J.M. Read, The invasion and coexistence of competing Wolbachia strains, Heredity 91(4) (2003), pp. 382–388.
- J.L. Kyle and E. Harris, Global spread and persistence of dengue, Annu. Rev. Microbiol. 62(1) (2008), pp. 71–92.
- H. Laven, Eradication of culex pipiens fatigans through cytoplasmic incompatibility, Nature 216(5113) (1967), pp. 383–384.
- M. Liu and C. Bai, Analysis of a stochastic tri-trophic food-chain model with harvesting, J. Math. Biol. 73(3) (2016), pp. 597–625.
- M. Liu and M. Fan, Permanence of stochastic Lotka–Volterra systems, J. Nonlinear Sci. 27(2) (2017), pp. 425–452.
- Z. Ma and T.G. Hallam, Effects of parameter fluctuations on community survival, Math Biosci. 86(1) (1987), pp. 35–49.
- X. Mao, G. Marion, and E. Renshaw, Environmental Brownian noise suppresses explosions in populations dynamics, Stoch. Process Appl. 97(1) (2002), pp. 95–110.
- X. Mao, Stationary distribution of stochastic population systems, Syst Contol Lett. 60(6) (2011), pp. 398–405.
- S.P. Meyn and R.L. Tweedie, Stability of Markovian processes III: Foster–Lyapunov criteria for continuous-time processes, Adv. Appl. Probab. (1993), pp. 518–548.
- M.Z. Ndii, N. Anggriani, J.J. Messakh, and B.S. Djahi, Estimating the reproduction number and designing the integrated strategies against dengue estimating the reproduction number and designing the integrated strategies against dengue, Res. Phys. 10 (2021), pp. 1–10.
- S.T. Ogunlade, A.I. Adekunle, M.T. Meehan, D.P. Rojas, and E.S. McBryde, Modeling the potential of wAuWolbachia strain invasion in mosquitoes to control aedesborne arboviral infections, Sci. Rep. 10(1) (2020), pp. 16812.
- W. Preechaporn, M. Jaroensutasinee, and K. Jaroensutasinee, Albopictus in three topographical areas, Control 1 (2007), pp. 23–27.
- E. Renshaw, Modelling Biological Population in Space and Time, Cambridge University Press, Cambridge, 1995.
- A. Utarini, C. Indriani, R.A. Ahmad, W. Tantowijoyo, E. Arguni, M.R. Ansari, E. Supriyati, D.S.Wardana, Y. Meitika, I. Ernesia, and I. Nurhayati, Efficacy of Wolbachia-Infected mosquito deployments for the control of dengue, N. Engl. J. Med. 384 (2021), pp. 2177–2186.
- T. Walker, P.H. Johnson, and L.A. Moreira, The wMel Wolbachia strain blocks dengue and invades caged Aedes aegypti populations, Nature 476 (2011), pp. 450–453.
- K. Wang, Stochastic Biomathematical Models, Science Press, Beijing, 2010. (in Chinese).
- X. Wang, S. Tang, J. Wu, Y. Xiao, and R.A. Cheke, A combination of climatic conditions determines major within-season dengue outbreaks in Guangdong province, Parasit. Vectors. 12(1) (2019), pp. 45.
- X. Zhang, S. Tang, and R.A. Cheke, Birth-pulse models of Wolbachia-induced cytoplasmic incompatibility in mosquitoes for dengue virus control, Nonlinear Anal-Real. 22 (2015), pp. 236–258.
- X. Zhang, S. Tang, R.A. Cheke, and H. Zhu, Modeling the effects of augmentation strategies on the control of dengue fever with an impulsive differential equation, Bull. Math. Biol. 78(10) (2016), pp. 1968–2010.
- X. Zhang, S. Tang, and R.A. Cheke, Models to assess how best to replace dengue virus vectors with Wolbachia-infected mosquito populations, Math. Bioci. 269 (2015), pp. 164–177.
- Y. Zhao, S. Yuan, and T. Zhang, The stationary distribution and ergodicity of a stochastic phytoplankton allelopathy model under regime switching, Commun. Nonlinear Sci. Numer. Simul. 37 (2016), pp. 131–142.