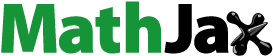
Abstract
We study an avascular spherical solid tumour model with cell physiological age and resource constraints in vivo. We divide the tumour cells into three components: proliferating cells, quiescent cells and dead cells in necrotic core. We assume that the division rate of proliferating cells is nonlinear due to the nutritional and spatial constraints. The proportion of newborn tumour cells entering directly into quiescent state is considered, since this proportion can respond to the therapeutic effect of drug. We establish a nonlinear age-structured tumour cell population model. We investigate the existence and uniqueness of the model solution and explore the local and global stabilities of the tumour-free steady state. The existence and local stability of the tumour steady state are studied. Finally, some numerical simulations are performed to verify the theoretical results and to investigate the effects of different parameters on the model.
MATHEMATICS SUBJECT CLASSIFICATION:
1. Introduction
Tumor growth model originated from the empirical equation was first proposed by Gompertz in 1825 to simulate tumour growth [Citation1], it remains highly relevant in a wide range of biomedical fields due to its good fit and simplicity with experimental data [Citation2,Citation3]. Later, a large number of papers aimed at understanding and developing the mechanisms of Gompertz's model equation [Citation4–7]. Over the next numerous years, a large number of mathematical models were proposed and studied for tumours in vivo or in vitro [Citation8–13]. In which Gyllenberg and Webb's model divided cancer cell populations into proliferating and quiescent cells, which greatly contributed to the development of mathematical models of tumours [Citation11]. Single-population tumour models provide a concise description of tumour growth, while multiple-population tumour models provide a more detailed description of the different physiological behaviours of different types of subpopulations within a tumour population.
Population age has been widely considered in various fields of bio-mathematics. Population models that consider age structure better reflects the real world, and corresponding models such as demographic models [Citation14,Citation15], epidemic models [Citation16–18], microscopic virus models [Citation19,Citation20] and cell population models [Citation8,Citation21] are presented. In the cell population model, cells are divided by age to represent the period of cell cycle. In 2002, Dyson et al. [Citation22] took the age structure into account in a linear two-component cell model, and he divided cell populations into proliferative and quiescent states to describe the different proliferative behaviours of cells. His model well characterized the early exponential growth of the cells and demonstrated that the final age distribution tended to be consistent.
Solid tumours tend to exhibit different growth rates in vascular and avascular growth environments. Initially, solid tumours in the avascular environment usually exhibit exponential growth based on the relative abundance of nutrients and space, followed by the development of a linear growth stage due to areas of quiescent cell development and core necrosis [Citation23–25], In the middle to later stage avascular tumour growth exhibits nonlinear proliferation. Ward et al. [Citation26] constructed a model of avascular tumour growth and investigated the exponential (initial) and linear (intermediate) stages of growth. Based on the improvement of Gyllenberg and Webb's model [Citation11] on the assumption of immediate clearance of dead cells, Alzahrani et al. [Citation27] further extended the model to a three-compartment form, after which the above model was further improved by considering a resource-constrained form [Citation28], and by studying the steady state of the tumour, the relationship between tumour size and the clearance rate of dead cells at positive steady state was found. Their work takes into account the prevalence of necrotic nuclear properties in advanced avascular tumours. Necrotic cores are mostly found in advanced avascular tumours, and the multilayer solid tumour model is more suitable for middle to later stage spherical tumour growth with avascular and resource-limited environment in vivo.
If solid tumour cells growing in an avascular environment do not produce enough vascular growth signalling protein (e.g. angiopoietin), then the tumour will only grow to the maximum size supported by the avascular environment and will not enter a vascular state. In avascular multicellular tumour spheroids, proliferating cells usually enter a quiescent state and stop dividing due to resource and space constraints, and eventually quiescent cells die and form necrotic cores inside the tumour. Avascular tumours do not immediately remove the dead cells from the necrotic cores, forming multilayered solid tumour structures. The quiescent layer and necrotic core tend to be common in the later stages of avascular multicellular tumour spheroids [Citation29]. Quiescent cells usually increase with tumour size, usually in a nonlinear fashion. The proliferation rate of tumour proliferating cells tends to exhibit nonlinear characteristics in the middle and late stages of avascular spheroid tumours. Liu et al. [Citation30–32] considered nonlinear proliferation functions in age-structured tumour models and analysed the stability of tumour models with nonlinear proliferation functions. Due to the nonlinear effects of resource limitation on cell populations in an avascular tumour environment, nonlinear models can better describe tumour growth in this situation. We consider nonlinear proliferation functions to the cell population model as in [Citation22,Citation33,Citation34] and consider necrotic cores in tumour cells to represent the multilayered structure of solid spherical tumours in vivo. The physiological age change of tumour cells may differ from the real time change, and we emphasize the evolution speed κ of tumour cells in the cell cycle ( tumour cell age/time) [Citation22,Citation30,Citation33–35]. The ratio of physiological age to time is different for different tumour cells. For a certain class of tumours, benign tumours may have a slower physiological age growth and thus have a smaller value of κ. Cycle-specific drugs are the most commonly used anti-cancer drugs, and we describe the therapeutic effect of cycle-specific drugs in terms of the proportion ℓ of newborn tumour cells entering the quiescent state [Citation30,Citation34].
The rest of this paper is structured as follows. In Section 2, we present a three-component tumour model with nonlinear age-structured proliferation functions. In Section 3, we rewrite the model and establish conditions for the existence, uniqueness and boundedness of the model. The existence and stability of tumour-free stable state is discussed in Section 4. The existence and local stability of the tumour steady state is studied in Section 5. In Section 6, we verify the results with numerical simulations and analyse the effects of parameter changes on the subpopulation size of the tumour. We give a short discussion at the end of the paper.
2. Multilayer age-structured tumour model
We construct a three-layer solid tumour model with nonlinear age-structured proliferation functions. We divide the tumour cells into three components being proliferating cells, quiescent cells and dead cells in necrotic cores not removed in time. Due to the three-layer structure of the avascular multicellular tumour spheroids in the late phase, we assume that death proliferating cells in the outer layer is cleared immediately, while dead quiescent cells enter the necrotic core and are cleared at a clearance rate d. The key developments in this model are based on previous research including [Citation8,Citation22,Citation30,Citation34]. Proliferating cells exhibit different physiological behaviours at different ages, whereas quiescent cells are not sensitive to their age stage, so in our model we only consider the age structure of proliferating cells.
The ratio of the change in physiological age of proliferating cells to the real time change is assumed to be a constant value κ, i.e. . If
, it means that the physiological age a change is twice the real time t change, which may indicate more active tumour cells growth. We define the nonlinear division rate of proliferating tumour cells as
, which is related to the age of proliferating tumour cells a and the total number of tumour cells
. The proliferating cell division rate
decreases as the population size
increases. Tumor cells need time to prepare substances required for cell proliferation, meaning that cell proliferation behaviour is correlated with cell age a. For the relatively limited amount of material in the avascular tumour environment, the larger the total number of tumour cells N, the less material is available for one tumour cell proliferation, so an increase for total number of tumour cells will lead to a decrease for cell proliferation function. We also assume that the age of the newly proliferating cells is 0. The newborn cells enter the proliferative phase again in proportion
and enter the quiescent phase directly in proportion ℓ with the age of 0 [Citation8,Citation33,Citation35]. This parameter ℓ reflects the effect of the therapeutic dose of drugs such as erlotinib [Citation36], which may affects the proportion of newborn cancer cells entering into quiescence [Citation34].
Since proliferating cells exhibit different physiological states at different ages, we consider the age-based change in the death rate of proliferating cells defined as at age a and the death rate of quiescent cells defined as a constant
. The constant d>0 is defined as the clearance rate of dead cells in necrotic care. As Liu [Citation30], we consider
as the cell age interval of cancer. We assume that due to spatial and nutritional constraints there is no quiescent cells entry into proliferating cells, but has age-based transition from proliferating cells to quiescent cells. This transfer rate is defined as
at age a with
.
The model adopts the following three-compartment system
(1)
(1) with boundary and initial conditions
(2)
(2) where
are numbers of quiescent and dead cells at time t,
is the age-specific density of proliferating cell population at time t with age a and age range of
.
represents the total number of proliferating cells physiological age from
to
at time t.
is the total number of cells at time t including proliferating ones, quiescent ones and dead ones.
For the rest of this this paper, we let the parameters are positive and assumption (H1) hold.
(H1) ,
,
, where
.
The nonnegative assumption on the age-specific parameters and ϑ satisfies the biological significance. (H1) guarantees the existence and uniqueness of the model solutions.
Following this, we first do some preparatory work and prove some fundamental properties of the model.
3. Model transformation and fundamental properties
In this section, we rewrite the model to a convenient form to prove the existence, uniqueness and boundedness of the solution of the model.
3.1. Model transformation
Let
(3)
(3) The total number of proliferation cells at time t is
. In this way we make the proliferation cells, the quiescent cells and the dead cells have the same structures. According to the biological significance of
we know that
when
. Similarly, we assume that Q and D satisfy
and
when
.
Based on (Equation3(3)
(3) ), we rewrite system (Equation1
(1)
(1) )–( Equation2
(2)
(2) ) to be
(4)
(4) with initial conditions
(5)
(5) and boundary conditions
(6)
(6) Obviously, the existence, uniqueness and boundedness of solutions between system (Equation1
(1)
(1) )–( Equation2
(2)
(2) ) and new system (Equation4
(4)
(4) )–( Equation6
(6)
(6) ) are consistent. We then investigate the existence, uniqueness and boundedness of solutions of system (Equation4
(4)
(4) )–( Equation6
(6)
(6) ).
3.2. Existence and uniqueness of system solutions
We study the fundamental properties of system (Equation4(4)
(4) )–( Equation6
(6)
(6) ) including the existence and uniqueness of the system solutions. Define a Banach space
with norm
for
, where
is the norm in
and
is the transpose of the vector ν. Consider the initial-boundary value problem of system (Equation4
(4)
(4) )–( Equation6
(6)
(6) ) as an abstract Cauchy problem on the Banach space X.
Let A be a linear operator defined by
(7)
(7) The domain
is given as follows
(8)
(8) where
denotes the set of absolutely continuous functions on interval
,
is positive cone of
,
(9)
(9) We define a nonlinear operator
as
(10)
(10) Based on assumption (H1), we can know that the operator F is Lipschitz continuous.
Let . The system (Equation4
(4)
(4) )–( Equation6
(6)
(6) ) can be considered as a semilinear Cauchy problem in Banach space X as follows
(11)
(11) where
. Using the same method as [Citation37], the following theorem can be obtained.
Theorem 3.1
For any initial value , there exists a unique mild solution
in
for the abstract Cauchy problem (Equation11
(11)
(11) ), and the positive invariant set of the semiflow
is
.
3.3. Boundedness of the model
Boundedness of the system is important for studying tumour models. In the following, we discuss the boundedness of system (Equation4(4)
(4) )–( Equation6
(6)
(6) ). We make the following assumptions about the nonlinear age-structured proliferation function.
(H2) Assume that the nonlinear proliferation rate has the separated variable form
.
satisfies
,
is continuously differentiable in
. In addition,
and
when
, where
is a positive constant.
satisfies
with a positive constant
.
Based on assumption (H2) we can get the following theorem.
Theorem 3.2
System (Equation4(4)
(4) )–( Equation6
(6)
(6) ) is ultimately bounded if assumptions (H1) and (H2) are satisfied.
Proof.
Let be the total number of all living cancer cells. From system (Equation4
(4)
(4) )–( Equation6
(6)
(6) ) we can know that
(12)
(12) where
. From assumption (H2) we get
(13)
(13) Let
, therefore,
(14)
(14) Similarly, for t large enough, we have
(15)
(15) In addition,
(16)
(16) Therefore,
, M is a positive constant. It is easy to see system (Equation4
(4)
(4) )–( Equation6
(6)
(6) ) is bounded if assumptions (H1) and (H2) are satisfied.
4. Existence and global stability of tumour-free steady state
In this section, we study the local stability of the tumour-free steady state by using a linear system and then study the global stability of the tumour-free steady state under two different conditions.
The steady state of system (Equation4
(4)
(4) )–( Equation6
(6)
(6) ) needs to satisfy the time-independent system
(17)
(17) with initial conditions
(18)
(18) where
(19)
(19) with
. The existence of tumour cell subpopulations is consistent with the initial value (Equation18
(18)
(18) ) when equation (Equation17
(17)
(17) )–( Equation18
(18)
(18) ) is satisfied. This means that either all three cell subpopulations are present or not present at the steady state. The system (Equation17
(17)
(17) )–( Equation18
(18)
(18) ) has a tumour-free stable state
and no boundary stable states.
4.1. Local stability of the tumour-free steady state
To discuss the local stability of the tumour-free steady state, we hypothesize that
(H3) is differentiable for N.
Based on assumption (H3), we can derive the tumour-free steady state linear system as
(20)
(20) with the boundary conditions
(21)
(21) Assume that the separating variable forms of the solutions are
(22)
(22) Then, we get
(23)
(23) Solving the first equation of (Equation23
(23)
(23) ) and substitute it into the forth equation, we get
(24)
(24) where
and
. Because of
(
leads to the tumour-free steady state), from (Equation24
(24)
(24) ) we get the following characteristic equation:
(25)
(25) Define the right-hand side of Equation (Equation25
(25)
(25) ) by
. It is not difficult to see that
is a continuous monotonically decreasing function with respect to
and satisfies
. Thus Equation (Equation25
(25)
(25) ) has and only has a unique real root
. Moreover, for the special case
we have
If condition
(26)
(26) is satisfied, we can obtain from the monotonicity of
that all the root real parts of
are less than 0 when Equation (Equation25
(25)
(25) ) holds.
Thus for the tumour-free steady state, the following theorem can be obtained.
Theorem 4.1
Let (H1) and (H3) hold. Moreover, if Equation (Equation26(26)
(26) ) is satisfied, then the tumour-free steady state
is locally asymptotically stable.
4.2. Global stability of tumour-free steady state
Here, we discuss the global stability of the tumour-free stable state under two different assumptions. We first make the following assumption.
(H4) Assume with
. Moreover,
is continuously differentiable and
in
.
Remark 1
The nonlinear proliferation function is related to the age a and the total number of tumour cells N. Assume that
has a separable form
, where
is the proliferation function of tumour cells with respect to age a when there is no restriction from the number of tumour cells N.
expresses the restriction on proliferation by the total number of tumours N, and the larger the N, the smaller the proliferation ability. Hence, we assume that
.
If the assumption (H4) is satisfied, the following theorem can be obtained.
Theorem 4.2
If assumptions (H1) and (H4) hold and
(27)
(27) is satisfied, the tumour-free stable state
is globally asymptotically stable.
Proof.
From system (Equation4(4)
(4) )–( Equation6
(6)
(6) ), we have
(28)
(28) Rewrite System (Equation28
(28)
(28) ) as a Cauchy problem for the Banach space
as follows.
(29)
(29) where the linear operator
is defined as
and
.
Construct linear comparison equations
(30)
(30) Let
and
be a integral solution of systems (Equation29
(29)
(29) ) and (Equation30
(30)
(30) ), respectively. When assumption (H4) holds, applying the comparability principle we get
(31)
(31) for all
. For linear problem (Equation30
(30)
(30) ), assume that
(32)
(32) The linear abstract problem (Equation30
(30)
(30) ) becomes
(33)
(33) where
,
and
are defined in (Equation19
(19)
(19) ). Solving the first equation of Equation (Equation33
(33)
(33) ) and substituting it to the second equation yields
(34)
(34) where
(
leads to the tumour-free steady state). From Equation (Equation34
(34)
(34) ) we get the following characteristic equation:
(35)
(35) If condition (Equation27
(27)
(27) ) is satisfied, then all roots of Equation (Equation35
(35)
(35) ) only have negative real parts.
Therefore, we get
(36)
(36) Then, for t large enough, we get
And hence
In the same way, we get
Let
, then we obtain
End of this proof.
When we choose assumption (H2), the global stability of the tumour-free steady state is also obtained under specific conditions.
Theorem 4.3
When assumptions (H1) and (H2) are true, and condition
(37)
(37) is satisfied, the tumour-free stable state
is globally asymptotically stable, where
and M is the ultimate upper bound of the system (Equation4
(4)
(4) )–( Equation6
(6)
(6) ).
Proof.
Transform the system (Equation4(4)
(4) )–( Equation6
(6)
(6) ) into the Cauchy problem in Banach space
as
(38)
(38) Define the linear operator H from X to X as
(39)
(39) and
is given as
. Similar to the approach in Theorem 4.2, we construct the linear abstract equations
(40)
(40) Let the integral solutions of system (Equation38
(38)
(38) ) and system (Equation41
(41)
(41) ) be
and
, respectively, and using the principle of comparability, we can know that
(41)
(41) Define the operator C from
to X as
Let the eigenvector of the operator H + C be defined as ω. The characteristic equation satisfied by system (Equation41
(41)
(41) ) can be obtained as follows.
(42)
(42) If condition (Equation37
(37)
(37) ) is satisfied, we can obtain that all eigenvectors ω have only negative real parts. Hence, we get
where
. That is
Since
,
and
are all nonnegative, we have
This proof is completed.
We proved the existence and local stability of the tumour-free steady state. Conditions for the global stability of the tumour-free steady state were obtained under different assumptions. From the conditions that need to be satisfied in Theorems 4.2 and 4.3, we know that condition (Equation27(27)
(27) ) is stronger, and when Equation (Equation27
(27)
(27) ) holds, Equations (Equation20
(20)
(20) ) and (Equation37
(37)
(37) ) also hold. Theorems 4.2 and 4.3 prove the global asymptotic stability of the tumour-free stable state under different assumptions. Assumption (H2) has more requirements relative to assumption (H4) that is, assumption (H2) is more strongly conditioned, and assumption (H4) is necessarily satisfied when assumption (H2) is satisfied. The interval between the two theorems lies in the difference in the assumptions and the strength of the threshold conditions.
5. Existence and local stability of the tumour steady state
In this section, we first discuss the existence of the positive steady state of system (Equation4(4)
(4) )–( Equation6
(6)
(6) ) and then study the local stability of it.
5.1. Existence of the tumour steady state
Define the tumour stable state of system (Equation4(4)
(4) )–( Equation6
(6)
(6) ) as
.
should satisfy the time-independent system (Equation17
(17)
(17) )–( Equation18
(18)
(18) ). A simple calculation of the time-independent system (Equation17
(17)
(17) )–( Equation18
(18)
(18) ) yields
(43)
(43) where
. Substituting the second equation of (Equation18
(18)
(18) ) and Equation (Equation43
(43)
(43) ) into the integral solution of the second equation of (Equation17
(17)
(17) ) yields
(44)
(44) In the same way, we get
(45)
(45) From Equations (Equation43
(43)
(43) )–( Equation45
(45)
(45) ) we can know that the existence of positive solutions of
,
and
depend on
. Thus the existence of tumour steady state for system (Equation4
(4)
(4) )–( Equation6
(6)
(6) ) is consistent with the existence of the positive solution of Equation (Equation43
(43)
(43) ). By using Equation (Equation43
(43)
(43) ) and the boundary condition
can be represented as
(46)
(46) where
(47)
(47) Equation (Equation46
(46)
(46) ) has a unique non-zero solution when
. The biological significance of
is that at tumour steady state a tumour cell produces an average of one tumour cell during its entire life cycle.
Theorem 5.1
Let assumption (H1) and N>0 hold, then
(48)
(48) is a sufficient and necessary condition for the existence of the tumour steady state. When in this case, the tumour steady state
can be represented by N in the form of (Equation43
(43)
(43) )–( Equation45
(45)
(45) ) with
(49)
(49)
Proof.
Combining Equations (Equation43(43)
(43) )–( Equation45
(45)
(45) ) we can deduce that
(50)
(50) Then, it can be seen that Equation (Equation49
(49)
(49) ) holds. In order to complete the proof, it can be sufficiently showed that the combination (Equation43
(43)
(43) )–( Equation45
(45)
(45) ) and (Equation48
(48)
(48) ) is equivalent to Equation (Equation46
(46)
(46) ). The analysis in this section just examines it is right. This proof is completed.
5.2. Local stability of the tumour steady state
To study the tumour steady state of the system we enhanced the assumption (H3) as follows.
(H5) is differentiable relative to N, and the partial derivative
of
with respect to N is bounded in the interval
.
The tumour steady state local perturbation form is defined as
, i.e.
Let
, where
. It can be seen that
is a constant and depend on the steady state. Then,
Based on basic system (Equation4
(4)
(4) )–( Equation6
(6)
(6) ), the perturbed form of the tumour steady state needs to satisfy
(51)
(51) where
and
The local properties are determined by the lower order terms, and we neglect the higher order terms in system (Equation48
(48)
(48) ) to obtain the following linear system.
(52)
(52) Based on assumption (Equation23
(23)
(23) ), we can obtain
(53)
(53) where
Let
. Solving the integral solution of system (Equation53
(53)
(53) ), we get
(54)
(54) The first equation of (Equation51
(51)
(51) ) requires the expression of
. Substituting the three equations of (Equation54
(54)
(54) ) into the expression for
yields
In the same way, substituting
,
and
into the above equation, we get
where
Let
We get
Then, substituting
into the expression of
and
. We get
(55)
(55) and
(56)
(56) Then we get characteristic equation:
(57)
(57) Finally, if Equation (Equation57
(57)
(57) ) is satisfied, the system solution of the form (Equation22
(22)
(22) ) exists. If all the characteristic roots satisfying Equation (Equation57
(57)
(57) ) have only negative real parts, the perturbation form
near the tumour steady state
tends to
as time tends to positive infinity. The following theorem is obtained.
Theorem 5.2
Let (H1), (H5) and (Equation48(48)
(48) ) be satisfied. In addition, if all solutions
of Equation (Equation57
(57)
(57) ) have negative real parts, the tumour steady state
of system (Equation4
(4)
(4) )–( Equation6
(6)
(6) ) is locally asymptotically stable.
Remark 2
We determine the local stability of the tumour steady state by analysing the sign of the eigenvalue of the characteristic Equation (Equation57(57)
(57) ) that satisfies the tumour steady state perturbation form [Citation38]. Based on our assumptions in this paper, if no solution
of (Equation57
(57)
(57) ) with
, the tumour steady state of the system (Equation4
(4)
(4) )–( Equation6
(6)
(6) ) will be locally asymptotically stable. The expression of (Equation57
(57)
(57) ) does not lead to the monotonicity of
and it is more difficult to get a mathematical criterion for determining the sign of
. But, in Section 6, the existence and stability of the tumour steady state is discussed by using numerical simulations.
6. Numerical simulations
We conduct some numerical simulations to illustrate the local and global stabilities of the trivial steady state as well as the existence and local stability of the positive steady state
. There are four key parameters: the proportion ℓ of newly born tumour cells entering the quiescent state, the evolution speed κ, the dead rate µ, dead cell clearance rate d should be more concerned. Different cells have different maximum survival times, and in the numerical simulations we chose
hours as the maximum tumour survival age and applied it to all examples. The age at which tumour cell proliferation begins is
hours.
For the nonlinear age-structured tumour proliferation functions we assume a separation variable of the form , where the age-structured proliferation function takes
(58)
(58) in the interval
and the restriction term takes
for all
(see [Citation30,Citation34]), where
is a variance constant. Assuming that the mortality rate differs before and after the age at which the tumour begins to proliferate, the mortality rate of the proliferating cells is select as a function as follows.
(59)
(59) for all
. Let the quiescent cells death rate be
. Let
(60)
(60) where
[Citation30].
is the maximum age of transformation from proliferating cells to quiescent cells. The mitotic interval is generally divided into
phase, S phase and
phase, but only in
phase, the transformation from proliferating cells to quiescent cells occurs. The duration of
phase varies from cell to cell in different environments. Billy et al. [Citation39] characterized the cell cycle at the single cell level in proliferating cell populations and differentiated
phase cells based on fluorescence image modelling techniques. Basse et al. [Citation40] used flow cytometry to analyse the DNA content of human melanoma cells at multiple time points and gave the proportion of
phase cells. For convenience, we take
hours with the maximum cell survival age as
hours. The forms of b, Ψ, µ and ϑ are based on biological significance and the assumptions of this article.
We study the effect of parameters on the conditions for determining the local stability of the tumour-free steady state, and investigate the global stability of the tumour-free steady state under suitable parameters. Take [Citation41], the initial functions
,
[Citation35] and
[Citation27]. We first study the effect of the proportion ℓ on the condition (Equation26
(26)
(26) ) in Theorem 4.1 for determining the local stability of the tumour-free steady state at different values of κ. Let
The tumour-free steady state
is locally stable when J<1.
Figure (a) shows the effect of the proportion ℓ on J at three different κ values. Note that J<1 always holds for . The effect of κ and ℓ on J is illustrated in Figure (b).
It is known from Figure that for larger values of κ a larger value of ℓ is required to ensure the local stability condition J<1 for the tumour-free steady state. This means that a larger dose of the drug erlotinib may prevent newborn cells from entering the proliferative state. It is interesting to note that when the cell evolution speed κ is less than 0.8717, any proportion ℓ in can contribute the inequality J<1, i.e. the extinction of the tumour cells.
Figure 1. (a) Effect of the parameter ℓ on the threshold condition J for stable tumour-free steady state at three different values of κ. (b) The effect of parameters ℓ and κ on J. (
).

Here we study the effect of µ on J.
Figure (a) shows the effect of the parameter µ on J for different values of κ. Figure (b) shows the effect of parameters µ and ℓ on J for different values of κ. From Figure (a ,b) we can conclude that in order to guarantee the condition J<1 with a larger κ, a larger µ and a larger ℓ are required. It can be seen from Figure that the mortality rate µ is smaller in order of magnitude and has a greater effect on J, meaning that the system is more sensitive to the parameter µ.
Now, we study the global stability of the trivial steady state . The initial value functions are chosen to be
and
in all cases without special instructions. From the previous results (Theorems 4.2 and 4.3) we can know that the global stability of the tumour steady state is independent of clearance rate d. From condition (Equation27
(27)
(27) ) in Theorem 4.2 and choosing parameter values
,
,
respectively, we can obtain
It is easy to see that these parameter values satisfy the global stability condition of
. The age distribution of the proliferating cells in Figure (a) shows that the number of proliferating cells reaches 0 in less than 500 hours, and Figure (b) shows this change from the number of proliferating cells at different ages. Figure (c) plots the changes in the number of the three tumour cell subpopulations P, Q and D as well as the changes in the total number of total cells N. Figure (d) shows the effect of different initial value functions on the total number of cells N when condition (Equation27
(27)
(27) ) is satisfied. It can be seen that the change in the initial value functions is only relevant for the time to reach the tumour-free steady state of the system.
Figure 2. (a) The effect of the parameter µ on J for κ taking three different values. (b) The effect of parameters ℓ and µ on J when κ takes three different values.

Figure 3. (a) Age distribution of proliferating tumour cells . (b) Trends in proliferating cells at six different ages. (c) The time series of three types of cells P, Q, D and the total number N. (d) The time series of N under four different initial value functions. The parameters of these four figures take values of
,
and
, which satisfy the global stability condition (Equation27
(27)
(27) ) of Theorem 4.2 for the tumour-free steady state.

Choosing a uniform upper bound on the total number of cells and by condition (Equation37
(37)
(37) ) in Theorem 4.3 we define
If we choose the parameter values
and
, by calculation we can get
, which satisfies condition (Equation37
(37)
(37) ).
Similar to Figures and plots the global stability of the tumour steady state under a superior condition. Condition (Equation37
(37)
(37) ) requires a lower death rate µ and a longer time t to reach the trivial steady state
than condition (Equation27
(27)
(27) ). It can be seen that
is needed to satisfy
if
and
, yet only
is needed to satisfy
. Numerical simulations are used to show again that condition (Equation27
(27)
(27) ) is stronger than condition (Equation37
(37)
(37) ).
Figure 4. (a) The age distribution of proliferating cells . (b) The time series of
at six fixed ages corresponding to Theorem 4.3. (c) The time series of four types of cells P, Q, D and the total number N. (d) The time series of the total number N under four different initial values. Where parameters
,
, and
are taken. These four figures show the global stability of the trivial steady state
under conditions of Theorem 4.3.

Next we discuss the existence and local stability of the tumour steady state. The values of the parameters are taken as and
. With these parameter values, the tumour steady state existence condition
is satisfied when N reaches
.
Figures describe the changes in the number of subpopulations in the tumour steady state from different perspectives. The initial value functions in Figures and is chosen as ,
and
. Figure (a) demonstrates the age distribution of proliferating tumour cells
under conditions that satisfy the existence of tumour steady state. Figure (b) shows the trends of proliferating cells at different ages. Figure (c) shows the cross-sections of Figure (a) at different times, i.e. the age distribution of proliferating cells at different times. It can be seen that the age distribution of proliferating cells tends to be consistent with increasing time. Figure (a) shows the time series of three types of cells P, Q, D and the total number N. From Figure (a) we can see that the number of the dead cells D is much lower than the number of other cells, so we show the time series of the number of the death cells D in Figure (b) alone.
Figure 5. (a) Age distribution of proliferating cells in tumour steady state. (b) Trends in proliferating cells at different ages. (c) Age distribution of proliferating cells at six different time points.

Figure 6. (a) The time series of three types of cells P, Q, D and the total number N. (b) The time series of the number of the death cells D.

Figure 7. (a) Effect of different initial value functions on proliferating cells of age 0. (b) Time series of N under different initial value functions.

From (Equation43(43)
(43) )–( Equation45
(45)
(45) ) we can know that steady state system (Equation17
(17)
(17) )–( Equation18
(18)
(18) ) depends continuously on the number of the newborn proliferating cells with age zero. We study the effect of different initial value functions on the total number of cells and the number of proliferating cells at age 0.
It can be seen from Figure (a ,b) that even though the initial value functions are different, the total number of cells N and the proliferating cells with age 0 tend to be the same for a long enough time, which indicates the stability of the tumour steady state. It shows that the initial value functions have no effect on the steady state of the system at a long enough time.
Figures and show the effect of parameters κ, ℓ and d on the number of P, Q, D and N at sufficiently long time points t = 4500 hours under the same mortality rate , respectively. Figure , we can know that the system is stable when the time is taken as 4500 hours. From Figure (a) we can observe that all tumour subpopulations P, Q, D and the total number of tumour cells N in the steady state increase with increasing κ. This may indicate that larger solid spherical tumours have a greater rate of cell evolution κ. Figure (b) shows the number of cells P, Q, D and N strictly decreases with the increase in the parameter ℓ.
Figure 8. (a) The effect of increasing the parameter κ at a sufficiently large time point on P, Q, D and N. (b) The effect of increasing the parameter ℓ on P, Q, D and N at the same time. (
,
and d = 0.01).

Figure 9. (a)–(b) The effect of the increase of d on P, Q, D and N when the system reaches the steady state (). The parameter values are chosen as
,
and
.

The tumour steady state is transformed into the tumour-free steady state if ℓ is large enough. We can also learn that is satisfied when
,
, d = 0.001,
,
, i.e. system (Equation4
(4)
(4) )–( Equation6
(6)
(6) ) has a globally stable tumour-free steady state. From Figure (a ,b) we can obtain that with the increase of the clearance rate d, Q and P will initially increase, while D and N will initially decrease. The increase of the clearance rate d initially had a significant effect on the number of dead cells D (see Figure (b)). When the clearance rate d>0.7, the change of the number of three kind cells P,Q,D and the total cells' number N will be very small.
We know from numerical simulations that controlling solid tumour growth requires a smaller κ, as well as a larger ℓ. This means that controlling tumour growth requires a smaller rate of cell evolution and a larger proportion of newly born tumour cells that allowed to enter the quiescent state. The initial value functions affect the arrival time to the steady state, but have no effect on the system when the time is long enough. The dead cell clearance rate d does not have a significant impact on the system than κ and ℓ, while D is still sensitive to the initial change in parameter d.
7. Discussion
We analysed a multilayer age-structured tumour model with nonlinear proliferation functions and necrotic cores. First, we discussed some fundamental results such as the existence, uniqueness (Theorem 3.2) and boundedness (Theorem 3.2) of solutions of the model. Then we obtained local stability conditions (Theorem 4.1) for the tumour-free steady state under some assumptions. The global stability of the tumour-free steady state is proved under two different assumptions (Theorems 4.2 and 4.3) using the comparability principle. The existence (Theorem 5.1) and local stability (Theorem 5.2) of the tumour steady state have also been demonstrated. Numerical simulations visualize the effect of parameter changes on the threshold conditions of the tumour-free steady state and analyse the effect of initial values and parameter changes on the system.
Our three-component model and mathematical theoretical results can provide some suggestions on these tumours with avascular growth. A greater proportion ℓ and a greater death rate µ can reduce tumour size and even eliminate tumours. A smaller evolution speed κ contributes to tumour control. Moreover, The death cell clearance rate d does not affect the extinction of the tumour population. Compared with the results in article [Citation28], the dead cell clearance rate d has an effect on the tumour size in the positive steady state, but the effect of this is very small.
In this paper, the selection of the parameters is based on literature but not distortion. If supported by experimental data, we may estimate the parameter values, and hence can greatly improve the application of this paper. This will be an important work we will pursue in the future.
This model does not consider the spatial factors and the bidirectional transformation of the proliferating and quiescent states. Considering spatial factors and bidirectional transfer into the model is very challenging and meaningful problem. In addition, it would be interesting and worthwhile to consider the four stages of the proliferating cell cycle and tumour immunity into future work.
Disclosure statement
No potential conflict of interest was reported by the author(s).
Additional information
Funding
References
- G Benjamin. On the nature of the function expressive of the law of human mortality, and on a new mode of determining the value of life contingencies. Proc R Soc Lond. 1825;115:513–583.
- AK Laird. Dynamics of tumor growth. Br J Cancer. 1964;18:490–502. doi: 10.1038/bjc.1964.55
- L Norton, R Simon, HD Brereton, et al. Predicting the course of Gompertzian growth. Nature. 1976;264:542–545. doi: 10.1038/264542a0
- CL Frenzen, JD Murray. A cell kinetics justification for Gompertz equation. SIAM J Appl Math. 1986;46:614–629. doi: 10.1137/0146042
- F Kozusko, Z Bajzer. Combining Gompertzian growth and cell population dynamics. Math Biosci. 2003;185:153–167. doi: 10.1016/S0025-5564(03)00094-4
- M Marusic, Z Bajzer, S Vuk-Pavlovic, et al. Tumor growth in vivo and as multicellular spheroids compared by mathematical models. Bull Math Biol. 1994;56:617–631.
- M Marusic, S Vuk-Pavlovic. Prediction power of mathematical models for tumor growth. J Biol Syst. 1993;1:69–78. doi: 10.1142/S0218339093000069
- BP Ayati, GF Webb, RA Anderson. Computational methods and results for structured multiscale models of tumor invasion. SIAM Multiscale Model Simul. 2006;5:1–20. doi: 10.1137/050629215
- P Bi, S Ruan, X Zhang. Periodic and chaotic oscillations in a tumor and immune system interaction model with three delays. Chaos. 2014;24:023–101. doi: 10.1063/1.4870363
- JA Florian , JL Eiseman, RS Parker. Accounting for quiescent cells in tumour growth and cancer treatment. IEE Proc Syst Biol. 2005;152:185–192. doi: 10.1049/ip-syb:20050041
- M Gyllenberg, GF Webb. Quiescence as an explanation of Gompertzian tumor growth. Growth Dev Aging. 1989;53:25–33.
- CJ Thalhauser, T Sankar, MC Preul, et al. Explicit separation of growth and motility in a new tumor cord model. Bullet Math Biol. 2009;71:585–601. doi: 10.1007/s11538-008-9372-8
- D Zhu, S Ruan, D Liu. Stable periodic oscillations in a two-stage cancer model of tumor and immune system interactions. Math Biosci Eng. 2012;9:347–368. doi: 10.3934/mbe.2012.9.347
- SN Busenberg, KP Hadeler. Demography and epidemics. Math Biosci. 1990;101:63–74. doi: 10.1016/0025-5564(90)90102-5
- H Inaba. Age-structured population dynamics in demograhpy and epidemiology. Singapore: Springer; 2017.
- H Inaba. Mathematical analysis of an age-structured SIR epidemic model with vertical transmission. Discrete Contin Dyn Syst Ser B. 2006;6:69–96.
- H Inaba. Age-structured homogeneous epidemic systems with application to the MSEIR epidemic model. J Math Biol. 2007;54:101–146. doi: 10.1007/s00285-006-0033-y
- RYM Massoukou, A Traore. An age-structured model for the transmission dynamics of hepatitis B: asymptotic analysis. Turk J Math. 2017;41:436–460. doi: 10.3906/mat-1412-50
- CJ Browne, SS Pilyugin. Global analysis of age-structured within-host virus model. Discrete Contin Dyn Syst Ser B. 2013;18:1999–2017.
- D Xiao, S Ruan, Y Yang. Global stability of an age-structured virus dynamics model with Beddington–DeAngelis infection function. Math Biosci Eng. 2015;12:859–877. doi: 10.3934/mbe
- J Wang, J Lang, X Zou. Analysis of an age structured HIV infection model with virus-to-cell infection and cell-to-cell transmission. Nonlinear Anal RealWorld Appl. 2017;34:75–96. doi: 10.1016/j.nonrwa.2016.08.001
- J Dyson, R Villella-Bressan, GF Webb. Asynchronous exponential growth in an age structured population of proliferating and quiescent cells. Math Biosci. 2002;177-178:73–83. doi: 10.1016/S0025-5564(01)00097-9
- J Carlsson. A proliferation gradient in three-dimensional colonies of cultured human glioma cells. Int J Cancer. 1977;20:129–136. doi: 10.1002/ijc.v20:1
- AD Congar, MC Ziskin. Growth of mammalian multicellular tumour spheroids. Cancer Res. 1983;43:558–560.
- J Folkman, M Hochberg. Self-regulation of growth in three dimensions. Exp Med. 1973;138:745–753. doi: 10.1084/jem.138.4.745
- JP Ward, JR King. Mathematical modelling of avascular-tumour growth. IMA J Math Appl Med Biol. 1997;14:39–69. doi: 10.1093/imammb/14.1.39
- EO Alzahrani, A Asiri, MM El-Dessoky, et al. Quiescence as an explanation of Gompertzian tumor growth revisited. Math Biosci. 2014;254:76–82. doi: 10.1016/j.mbs.2014.06.009
- EO Alzahrani, Y Kuang. Nutrient limitations as an explanation of Gompertzian tumor growth. Discrete Contin Dyn Syst Ser B. 2016;21:357–372. doi: 10.3934/dcdsb
- HE Skipper. Kinetics of mammary tumor cell growth and implications for therapy. Cancer. 1971;28:1479–1499. doi: 10.1002/(ISSN)1097-0142
- Z Liu, J Chen, J Pang, et al. Modeling and analysis of a nonlinear age-structured model for tumor cell populations with quiescence. J Nonlinear Sci. 2018;28:1763–1791. doi: 10.1007/s00332-018-9463-0
- Z Liu, C Guo, H Li, et al. Analysis of a nonlinear age-structured tumor cell population model. Nonlinear Dyn. 2019;98:283–300. doi: 10.1007/s11071-019-05190-4
- Z Liu, C Guo, J Yang, et al. Steady states analysis of a nonlinear age-structured tumor cell population model with quiescence and bidirectional transition. Acta Appl Math. 2020;169:455–474. doi: 10.1007/s10440-019-00306-9
- O Arino, E Sanchez, GF Webb. Necessary and sufficient conditions for asynchronous exponential growth in age structured cell populations with quiescence. J Math Anal Appl. 1997;215:499–513. doi: 10.1006/jmaa.1997.5654
- P Gabriel, SP Garbett, V Quaranta, et al. The contribution of age structure to cell population responses to targeted therapeutics. J Theor Biol. 2012;311:19–27. doi: 10.1016/j.jtbi.2012.07.001
- FB Brikci, J Clairambault, B Ribba, et al. An age-and-cyclin-structured cell population model for healthy and tumoral tissues. J Math Biol. 2008;57:91–110. doi: 10.1007/s00285-007-0147-x
- DR Tyson, SP Garbett, PL Frick, et al. Fractional proliferation: a method to deconvolve cell population dynamics from single-cell data. Nat Methods. 2012;9:923–928. doi: 10.1038/nmeth.2138
- H Inaba. A semigroup approach to the strong ergodic theorem of the multi-state stable population process. Math Popul Stud. 1988;1:49–77. doi: 10.1080/08898488809525260
- ME Gurtin, RC Maccamy. Non-linear age-dependent population dynamics. Arch Ration Arch Ration Mech Anal. 1974;54:281–300. doi: 10.1007/BF00250793
- F Billy, J Clairambaultt, O Fercoq, et al. Synchronisation and control of proliferation in cycling cell population models with age structure. Math Comput Simul. 2014;96:66–94. doi: 10.1016/j.matcom.2012.03.005
- B Basse, BC Baguley, ES Marshall, et al. A mathematical model for analysis of the cell cycle in cell lines derived from human tumors. J Math Biol. 2003;47:295–312. doi: 10.1007/s00285-003-0203-0
- L Spinelli, A Torricelli, P Ubezio, et al. Modelling the balance between quiescence and cell death in normal and tumour cell populations. Math Biosci. 2006;202:349–370. doi: 10.1016/j.mbs.2006.03.016