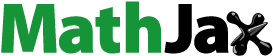
ABSTRACT
In recent decades, Digital transformation has significantly shifted human activities from physical space to cyber space. When users access the internet, uniform resource locator (URL) data are autogenerated. Using URLs, this study presents a novel framework for exploring cyber space structure from the perspectives of complex networks and activity fragmentation. Web domains within URL data are metaphorically regarded as ‘digital locations,’ and consecutive digital locations form ‘cyber trajectories.’ Human activities that occur at digital locations are semantically labeled and used to generate activity-based motifs. Motifs are defined as frequently occurring processes in cyber trajectories. Based on this, three network types are constructed: Global cyber human activity network, including all trajectories, and space-dependent and motif-dependent cyber human activity networks, focusing on specific regions and motifs. A case study conducted in Jilin, China, using approximately 4.3 gigabytes of URL data, revealed: 1) Cyber human activity patterns exhibit strong regularity and clustering of several types, with metropolitan regions favoring simpler patterns; 2) Cyber human activity networks demonstrate heavy-tailed and hierarchically polycentric structures; 3) The importance of websites in information dissemination increases super linearly along with their increased connectivity. This work deepens our understanding of cyber space functionality, offering insights into cyber information propagation.
1. Introduction
Human activity, geographic space, and the interactions between them have received great attention from experts in various fields for quite some time, and there have been many representative works, encompassing such topics as time geography (Hägerstrand Citation1989), space syntax (Hillier et al. Citation1976; Hillier and Hanson Citation1989) and the new sciences of city (Batty Citation2013). Among them, there has been plenty of research conducted to explore human activity in physical space, and such studies typically use the method of assessing human activity networks, since such networks enable individual trajectories to be modeled using graph theory. A collection of individuals moving in physical space is regarded as a human activity network that is oriented toward the network structure. Then, the application of complex network theory to these networks has enabled the statistical analysis of human activities in physical space and the evaluation of their properties. In addition, motifs have also been widely used to reveal heterogeneous properties among different activity patterns (Cao et al. Citation2019). In the context of human activities, motifs are defined as frequently occurring movements that originate from complex networks.
With the popularization of the internet and smart devices in recent decades (smartphones, intelligent sensors, etc.), human activities are no longer restricted to physical space. The digital transformation has been gradually and fundamentally shifting human activities from occurring in physical space to occurring in cyber space. Cyber human activity has been rapidly gaining attention since an increasing proportion of physical human activity, i.e. physical activities such as communication and shopping, has gradually been replaced by online activities. In addition to this type of replacement, the rapid development in information and communication technologies (ICTs) has also weakened the association between activity, place, and time and thus enabled a fragmentation of activity in both space and time (Alexander et al. Citation2011; Couclelis Citation2004). Activity fragmentation is a process where activities are disintegrated into separate smaller subtasks that can be performed at different times and locations (Couclelis Citation2003). In addition to activity fragmentation, fragmentation has also been applied in many other fields, such as cell biology and ecology (Fahrig Citation2019; Lodish et al. Citation2008). Although slight differences exist in the understanding of fragmentation in different fields, the basic definition is consistent among them. Generally, there are two types of activity fragmentation: temporal fragmentation and spatial fragmentation.
Previous studies have confirmed a strong association among activity fragmentation, transportation and ICT in physical space. For instance, the advent of ICT has enabled paid work to be conducted during unconventional times, such as late evenings and weekends, and at unconventional locations, such as homes and trains (Alexander et al. Citation2011), and this transformation has reduced travel demand, as it has afforded individuals greater flexibility in their travel decisions (Ben-Elia et al. Citation2014). As cyber space is shaped by various online human activities, such as browsing, sharing, and consuming (Zhang et al. Citation2022), the fragmentation of human activities also plays a crucial role in defining the characteristics of cyber space. For instance, leisure activities in cyber space can be dispersed across different websites, such as Netflix and Steam. This fragmentation between different websites can be a significant factor that influences information diffusion in cyber space. Additionally, the temporal fragmentation of human activity can also have an impact, especially in studies involving cyber human activity networks in which time is a determining factor. For example, the temporal fragmentation of online activities may indicate periods when individuals temporarily disconnect from cyber space, which influences the construction and analysis of cyber human activity networks.
However, the literature concerning human activity in cyber space, especially cyber human activity networks, is insufficient. Some studies have explored topics such as cyber visiting preferences (Zhang et al. Citation2022) and the correlation between physical and cyber human activity (Hazarie et al. Citation2020; Zhao et al. Citation2014). However, research on cyber human activity networks is scarce, and a comprehensive framework for the theoretical construction and analysis of such networks is lacking due to the scarcity of cyber datasets. Additionally, the impact of the temporal fragmentation of human activities on the construction of cyber human activity networks has been neglected in previous research. Moreover, previous studies have generally treated cyber space as a homogeneous entity, disregarding the potential heterogeneity across different cyber human activity patterns and different regions.
To address these gaps, this study is aimed at exploring the structures of cyber space from the perspective of cyber human activity networks, which are constructed and characterized on the basis of approximately 4.3 gigabytes of uniform resource locator (URL) records. The web domains in the URL data visited by users are treated as the metaphor indicating ‘digital locations.’ A digital trajectory of online users can be generated by connecting consecutive digital locations in chronological order. Then, based on the head/tail break method, the complete trajectory is split into segments using the average of the time intervals between users’ consecutive URL records, which represents the temporal fragmentation of cyber activities. After that, the interconnected digital locations form a cyber human activity network. In this work, we construct three kinds of networks, a global human activity network, which includes all trajectories, cyber human activity subnetworks across different cities, and multiple cyber activity subnetworks developed in accordance with activity-based motifs, which are abstracted from the activity labels assigned to the trajectories. Finally, these networks are explored from two perspectives, namely, complex network-driven measures and the scaling relations between such measures, to explore network structures in cyber space.
This study makes two significant contributions. First, we propose a comprehensive framework for defining, constructing, and analyzing the cyber human activity network through the use of massive URL data. This approach offers a novel perspective on exploring the structure of cyber space. Moreover, we consider the temporal fragmentation of cyber activities when building cyber human activity networks. Second, apart from presenting the characteristics of the entire cyber human activity network, we delve into the potential heterogeneity between different activity patterns and regions by employing activity-based motifs and examining multiple cyber activity subnetworks.
The remainder of this work is organized as follows. Section 2 reviews the related literature. Section 3 introduces the study area and URL data processing. Section 4 presents the proposed methodology framework, including the construction of activity-based motifs and the generation and characterization of cyber human activity networks. Section 5 presents an analysis of the findings, followed by a presentation of the conclusions and discussions in Section 6.
2. Literature review
Currently, it is obvious that cyber space has fundamentally changed nearly every aspect of human life. For instance, Alipay and WeChat pay have become mainstream payment methods, online shopping has gradually replaced traditional offline shopping, and even culture has changed, including such aspects as how we express and identify ourselves and how we socialize with others and gain information (Franklin Citation2015; Xu Citation2022). The enormous impact and radical changes caused by cyber space make it a necessary and urgent research target.
The fragmentation of activity is one of the most significant changes brought about by the development of information and communication technologies (ICTs). This concept was initially popular in ecology, which advanced vast literature concerning the fragmentation of forests and habitats (Fahrig Citation2019; Rutledge Citation2003). Fragmentation begins to appear in individual activities with the advancement of technology, which is noted by Couclelis (Citation1998). Couclelis (Citation1998) claims that this shift from traditional place-based perspectives to individual-based views, which is exemplified by activity fragmentation, can profoundly impact urban society. For instance, activity fragmentation can manifest as an alteration in place usage and an increase in the diversity of activity patterns, which influences travel demand and offers crucial insights for transportation policy-makers (Alexander et al. Citation2011).
Cyber space, which is shaped by human internet activities through diverse technologies, requires a consideration of the impact of activity fragmentation to properly explore it, particularly in the context of cyber human activity networks. Previous research on cyber space has mainly been conducted in the computer science field and focused on the physical topology of cyber space. These studies typically employ complex network theory to explore cyber networks, which are formed by fixed hyperlinks between websites (Pastor-Satorras, Vázquez, and Vespignani Citation2001). These findings have significantly advanced our understanding of cyber networks, which has benefitted the development of search algorithms (Adamic et al. Citation2001), routing (Floyd and Paxson Citation2001), and virus prevention (Pastor-Satorras and Vespignani Citation2001). Furthermore, certain studies have ventured into cyber space through the application of theoretical perspectives, such as the integration of cyber philosophy, cyber science, and the cyber information field to establish a foundational framework that can enable a more profound understanding of cyber-physical-social-thinking and cyber spatial objects (Kademi Citation2020; Kademi and Koltuksuz Citation2021). Then, building upon these theories, relevant models, such as the network cellular automata model, have been developed to characterize cyberspace (Kademi and Koltuksuz Citation2020). Human geographers have also delved into cyber space, with Gibson (Citation1984) describing its placelessness, timelessness, and disembodiment in his novel ‘Neuromancer.’ Recently, cyber geography has gained greater attention in light of rapid network technology advancements. However, fundamental challenges associated with cyber space, such as the absence of place, the insignificance of geographical distance, and the mapping of cyber space, remain unresolved (Batty Citation1993; Batty Citation1997; Benedikt Citation1991; Boos Citation2017; Dodge Citation2001; Huang Citation2001; Kwan Citation2001; Sui Citation2004). Therefore, a novel perspective is introduced in this study by proposing a framework for exploring cyber space structure through cyber human activity networks, which has received limited attention in the extant literature. Moreover, the construction of cyber human activity networks considers the fragmentation characteristic of human activity.
In physical space, human activity analysis has become a significant and interdisciplinary research area, as it assists in the uncovering of spatiotemporal patterns in individual and group movements (Cao et al. Citation2019; Tu et al. Citation2018). These movements can be represented by various graphs based on origin-destination (OD) matrices, making the study of human activity networks an effective approach (Parthasarathi Citation2014). Similarly, cyber space, which is another dimension of human activity, follows the same human activity logic as physical space (Hillier Citation2007). Investigating cyber space through human activity analysis is intriguing. However, cyber human activity networks remain relatively unexplored and lack a clear definition. In this study, a framework for defining and analyzing cyber human activity networks using domain trajectories is proposed. Additionally, given the temporal fragmentation of cyber access (Alexander, Ettema, and Dijst Citation2010; Ben-Elia et al. Citation2014), decomposing individual graphs into segments is crucial for a realistic simulation of cyber human activities.
Complex network theory, which has proven effective in various network analyses, such as computer networks, social networks, and biology (Albert and Barabási Citation2002; Dorogovtsev and Mendes Citation2003; Newman Citation2003), deals with networks comprising diverse nodes and edges (Newman Citation2018). Quantitative measures such as node degree, clustering coefficient, and centrality, along with properties such as scale-free and hierarchical characteristics, are commonly used to assess networks (Albert and Barabási Citation2002; Barthélemy Citation2004; Ravasz and Barabási Citation2003). Complex network theory has found wide applications in physical human activity networks: for instance, Tran and Draeger (Citation2021) used network centrality to enhance urban access, and Wang et al. (Citation2017) predicted traffic congestion using PageRank scores. Building upon complex network theory, our study is aimed at investigating the structure of cyber human activity networks and exploring the potential heterogeneity in different regions and cyber activity patterns through various motifs.
Motifs, which are derived from complex network theory, are commonly used to categorize human activities and reveal the variations in different activity patterns. In the context of human activity networks, motifs refer to frequently occurring structures and can be broadly classified into two types: location-based motifs (LBMs) and activity-based motifs (ABMs). LBMs entails sequences of stopover sites that are connected by human movements. For instance, if we examine an individual's daily activities, only including those movements between home and office, we can represent the corresponding LBM with two dots that symbolize home and office, connected by a two-way arrow indicating the movements between these locations. Conversely, ABMs involves sequences of human activities and the journeys between them. For example, a person's daily activities might include home, work, lunch at a restaurant, and returning home after work. The corresponding ABM can be represented with three dots denoting home, work, and recreation, linked by two-way arrows. Both LBMs and ABMs can be used to describe various activity patterns and explore complex human behaviors, each with a unique focus. These motifs find applications in various areas, such as assessing daily trip arrangements (Cao et al. Citation2019), understanding the relationship between motif counts and scaling parameters (Cao et al. Citation2021), and revealing connections between diverse motifs and regional functions (Liu et al. Citation2016; Shi et al. Citation2022). Surprisingly, limited research has delved into cyber human activity motifs, let alone examined the differences between them. Inspired by ABMs in physical space, our study proposes a similar approach for extracting ABMs in cyber space. For instance, if we consider an individual's cyber trajectory, including websites such as WeChat, YouTube, and WhatsApp, this can be abstracted into a motif containing two nodes representing communication and entertainment linked by two-way arrows. We not only extract ABMs in cyber space but also explore the structural variations in multiple cyber human activity subnetworks constructed through the use of cyber ABMs. Detailed procedures are outlined in Section 4 of this study.
3. Study area and datasets
3.1. Study area
The study area in this work is Jilin Province, China, as shown in . Jilin Province is located in northeastern China, bordering North Korea and Russia. Its total area is 191,202 km², with a population of approximately 24.07 million according to national statistics (The Seventh Census, 2020). Changchun is the capital city, and there are eight other secondary administrative regions in the province (right in ). According to government statistics, there has always been an enormous gap in economic and social development between the capital and other secondary administrative regions. Therefore, spatial heterogeneity analysis is also applied in the study.
3.2. Uniform resource locator (URL) data
HTTP URL data are used to construct cyber human activity networks. Initially, the generation process of URL data proceeds as follows: users’ smart mobile devices access the internet via signal communication with cellular towers. These towers, which are maintained by service providers, not only grant public network access but also monitor users’ internet usage. The metadata of users’ internet usage comprise URL data, including the entire data transmission chain involving ‘user devices-cellular towers-website services.’ The dataset used in this study is derived from China Unicom, which is one of China's three major mobile operators and uses a comprehensive user sign-in process prior to providing mobile network services. Moreover, according to official reports, by the end of 2016, China Unicom accounted for one-seventh of the mobile user market share. Based on the statistical principles of large datasets, a sampling ratio exceeding 10% is deemed representative. Therefore, the dataset from China Unicom is representative. To safeguard users’ privacy, the data provider has removed personal demographic attributes such as usernames, phone numbers, gender, age, and mobile phone brands. An example of the data used in the study is presented in , comprising five components: the unique identity of mobile phone users when accessing mobile internet (User ID, further masked in ), the time of visiting a website (Request Time), the domain of visited websites (URL, with data length varying greatly depending on the website domain), the types of websites (Activity Type, manually classified with detailed category contents shown in ), and the latitude and longitude of the cellular tower to which the user connects during their online activity (Location). The data used in the study were collected on Tuesday, December 20, 2016. In total, there are 87,098 internet users and 19,916,899 internet records based on the one-day data in the study area. On average, every internet user browses approximately 228 web domains a day. Further calculations were conducted to present the user proportions in different cities, as depicted in . It is evident that Changchun City has the highest user proportion, followed by Jilin City. Siping City ranks third, with the remaining six cities exhibiting comparable proportions. This distribution aligns with the population ranking from the 2015 Jilin Province census, which further confirms the representativeness of our dataset in a spatial context.
Figure 2. The user proportions in the nine secondary administrative regions of Jilin Province, as calculated from the URL dataset.

Table 1. The HTTP URL sample data.
Table 2. The detailed contents of each category.
4. Methodology
This section illustrates the framework used for this study, as presented in . This framework includes three main steps. The first step is to abstract the activity-based motifs considering the temporal fragmentation of cyber access. The second step is to generate the global cyber human activity network (NGlobal), space-dependent cyber human activity networks (NSpace), and motif-dependent cyber human activity networks (NMotif) through the use of activity-based motifs. The third step is to characterize the cyber human activity networks from two aspects: statistical measures and scaling relations.
4.1. Extraction of activity-based motifs
The process of abstracting the extensive uniform resource locator (URL) data into activity-based motifs can be broken down into two main steps, as shown in . First, the URL data are segmented into separate, noncontinuous internet records for each individual, considering fragmented internet access. Next, utilizing the activity labels assigned to each web domain, activity-based motifs are derived from the aggregation of abstractions of each segment, where nodes represent web domains and edges represent the movements between two web domains.
4.1.1. Temporal fragmentation of cyber access
First, the data were sorted according to the timestamp, and the internet records of each individual were cropped in chronological order, as shown in the right part of . Here, the black folded line represents a person’s physical human activities in a day, and the three red straight lines represent the periods when the person is connected to cyber space and conducts some online activities. The orange triangles represent a set of log-ins to the cyber space, and the purple triangles represent a set of log-offs. In this work, we apply a head/tail break to the time interval between consecutive internet records to split individual records into several segments corresponding to each period of cyber activity. As illustrated in , the time interval of consecutive records within the red line (Interval 1, Interval 2, and Interval 3) is shorter than that between log-off and the next log-in (Interval 4). In addition, considering that people’s time spent on every page is relatively short, each web domain is recognized as a valid node. These sequential records were then used to build directed graphs.
The head/tail break method is a well-recognized method for effectively capturing imbalanced data distributions containing significantly more small values than large ones. The process involves sorting the data based on their values and then dividing them into two parts, head and tail, based on the mean value. The head part contains a small number of data points, while the tail contains a larger number, thus highlighting the imbalance of datasets (Jiang Citation2013). In this study, we consider that the time intervals of consecutive records within a segment are much shorter than the time interval between the last records in the former segment and the first records in the latter segment. Additionally, the cyber records are mostly clustered within the segments, resulting in a larger number of short time intervals and a smaller number of large time intervals. Therefore, utilizing head/tail breaks of the time intervals will effectively distinguish different segments for the subsequent construction of motifs from these segments.
4.1.2. Construction of activity-based motifs
M denotes the number of segments utilized for further analysis in this study. Each segment contains an activity chain that is denoted as S(se) = {Activity1, Activity2, … , ActivityZ}, where Z represents the number of times that cyber activities are accessed. Subsequently, the corresponding activity network structure G(se) = {V(se), E(se)} is constructed based on S(se). The vertex set V(se) = {V1, V2, … , VR} contains all distinct performed activities, where R refers to the number of activity types. The edge set is defined as E(se) = { dea,b | a,b∈V(se)∧a ≠ b}, and it encompasses all distinct directed movements, where dea,b represents the directed movement from vertex a to vertex b. Ultimately, each segment is abstracted into a graph, and the activity-based motifs are formed by aggregating the same graph abstractions extracted from all users. In these motifs, each node represents a distinct web domain, and each edge represents a directed movement between different web domains. The process of extracting the activity-based motifs from the aggregation of all users is illustrated on the right-hand side of .
4.2. Construction of cyber human activity networks
There are three kinds of cyber human activity networks constructed in this work: the global cyber human activity network (NGlobal), the space-dependent cyber human activity network (NSpace), and the motif-dependent cyber human activity network (NMotif). Additionally, three reference networks are also included for further analysis: scale-free cyber human activity network (NScale), random cyber human activity network (NRandom), and small-world cyber human activity network (NSmall). A brief introduction is provided in .
Table 3. The introduction of the networks used in the study.
4.2.1. Global cyber human activity network (NGlobal)
To reflect the complete topology of the cyber human activity network, NGlobal is constructed by aggregating all cyber activities. NGlobal is a directed network and is defined as follows:
(1)
(1) where
contains all unique cyber nodes, and
contains all unique movements between pairs of nodes. represents part of NGlobal, from which the imbalanced visits among different websites are obvious.
4.2.2. Space-dependent cyber human activity networks (Nspace)
To capture the spatial variations in cyber human activity, we construct space-dependent cyber human activity networks using URL records from distinct regions. These regions are delineated based on administrative divisions, specifically, nine cities within Jilin Province, as illustrated in . By considering the locations of the cellular towers connected to by users during their online activities, the entire dataset is segmented into nine parts, with each part corresponding to each city. Subsequently, all the data within each city are aggregated to construct multiple directed networks, denoted as space-dependent cyber human activity networks (NSpace). The mathematical formulation for an NSpace is defined as follows:
(2)
(2) where
contain all unique nodes belonging to region
, and
contain all unique movements between these nodes.
4.2.3. Motif-dependent cyber human activity networks (Nmotif)
To capture the differences among human activity patterns, we construct cyber human activity networks using URL records grouped by every specific motif. Similar to the process of NGlobal, the data belonging to the same motif were aggregated to form multiple directed networks, which are called motif-dependent cyber human activity networks (NMotif). The mathematical definition for an NMotif is presented as follows:
(3)
(3) where
contains all of the unique cyber nodes belonging to motif
, and
contains all of the unique movements between these nodes.
4.2.4. Reference networks
In the study, NRandom and NScale represent two extreme phenomena of network structures. They serve as particularly important baselines when evaluating the development of networks. NRandom, represents a purely random connection between different web domains devoid of any preference, which reflects a completely uniform distribution of resources and facilities. It is a typical representative of the entirely homogeneous network in cyber space. According to previous explorations, random networks have the characteristic of small clustering coefficients, and their degree distributions follow the Poisson distribution (Frieze and Karoński Citation2016). The NScale is the exact opposite of NRandom. The most notable characteristic of the NScale is heterogeneity, which can be reflected in the node degree. In other words, a small number of nodes can have a significantly higher than average number of connections, and such nodes are often named ‘hubs,’ These hub nodes are believed to serve specific functions in the network. Another key feature is the negative correlation between the node degree and the clustering coefficient (Saramäki et al. Citation2007), indicating that low-degree nodes belong to highly interconnected subgraphs connected through hub nodes. NSmall exhibits a distinctive feature in which most nodes are not direct neighbors, yet the neighbors of any node are highly likely to be connected to each other. Consequently, most neighboring nodes can be reached from any node within a few steps, leading to one of the significant characteristics of NSmall, i.e. its small average shortest path length (Watts and Strogatz Citation1998). Additionally, NSmall is also characterized by relatively large global clustering coefficients and a heavy-tailed degree distribution, which serve as two other prominent traits of this network.
4.3. Characteristics of cyber human activity networks
Cyber human activity networks are evaluated through two aspects, namely, scaling relations and statistical measures. The statistical measures include node degree, betweenness centrality, average shortest path length, and clustering coefficient, which are then compared with those of reference networks. The scaling relations between these measures are investigated to explore the structures of cyber human activity networks. A detailed description and mathematical equations are provided in .
Table 4. Description of the network indicators.
5. Results
5.1. Properties of activity-based motifs
Based on the results of applying the head/tail break method, an interval of 98.9 min was selected for dividing the entire individual trajectories into segments representing every cyber activity. After processing the dataset, 151,413 segments were extracted and characterized by 610 eligible unique motifs. The top 14 motifs were selected as the most frequent and marked for further analysis. displays the percentage of the 14 selected motifs and their structures. It is obvious that the percentage increases when the number of nodes decreases: the highest percentage is 28.5% and corresponds to a single node, followed by the motifs with two nodes (20.2%), three nodes (18.1%), four nodes (5.4%), and five nodes (1.3%). The results suggest two main findings. First, cyber activity patterns exhibit clustering in a few specific motifs, indicating a high degree of regularity among individuals engaging in cyber activities. Second, motifs with fewer nodes are preferred in cyber space. In addition, individuals with simpler motifs tend to have specific goals or purposes when engaging in cyber activities, which implies that individuals often use cyber platforms as tools to accomplish their specific goals to avoid aimless exploration or wandering in cyber space. These findings highlight the regularities and goal-oriented nature of cyber activities for many individuals.
Figure 6. The top 14 most frequent motifs extracted from URL data and their corresponding structures.

To reveal the spatial heterogeneity of the top 14 motifs, the proportions of the top 14 motifs in the capital and eight other secondary administrative regions are calculated and displayed in . There is one distinct region, Changchun, which is different from the other eight regions: Changchun holds a higher percentage of simple motifs. For example, as shown in , the probability of motifs with one and two nodes in Changchun is 0.678, while that in other cities is between 0.619 and 0.67. One possible explanation for these findings is that individuals residing in prosperous areas exhibit lower engagement in cyber activities and tend to have more highly focused goals when participating in such activities compared to those of individuals who live in less prosperous areas. This could be attributed to the fact that prosperous areas generally offer a wider range of physical facilities and resources that sufficiently cater to the needs of individuals. Consequently, people in these areas may rely less on cyber platforms to fulfill their requirements, leading to a reduced frequency and a narrow purpose underlying their cyber activities.
Figure 7. The proportions of the top 14 motifs in the nine administrative regions of Jilin Province.

Table 5. The percentage of different motif groups in the nine regions of Jilin.
5.2. Properties of the global cyber human activity network
There are 13,505 nodes and 582,789 edges in NGlobal, and it covers the entire study area. Regarding the statistical measures, the average degree < K > of the NGlobal is 86.307, comprising out-degree and in-degree. This suggests that, on average, every web domain is connected with the other 43 web domains for every activity segment and that the connection of NGlobal is relatively high. demonstrates a high relevance between in-degree and out-degree; thus, the slight difference between the two degrees can be ignored, and the sum of the two degrees is regarded as the degree marked for further analysis. shows the complementary cumulative distribution functions (CDF) of degree and betweenness centrality through a logarithmic plot. The red lines represents the power-law fits of the empirical data (blue points) based on the method proposed by (Clauset, Shalizi, and Newman Citation2009). The distributions from NRandom, NScale, and NSmall are predicted with the same < K > and are displayed in (with yellow, green, and pink lines, respectively). Although the degree and betweenness centrality distributions in the three reference networks all display heavy-tailed characteristics, the specific trends of these distributions exhibit significant differences. It is evident that for larger values, P(K) and P(BC) obey power law distributions (), which are roughly consistent with the distribution of NScale. The most obvious characteristic of the power-law distribution is the heavy tail, which represents heterogeneous spatial patterns. The distribution of nodes with moderate betweenness centrality is similar to that of NSmall. However, the disparity becomes more pronounced as the level of betweenness centrality increases. For small degree and betweenness centrality, the difference between the empirical data, the power-law, and the Poisson distribution indicates that the small values in NGlobal cannot be characterized by either a random network, a small world network, or a scale-free network. The results suggest the presence of cyber division, where certain web domains exhibit higher connectivity and influence than the average, while others have limited connections and influence in the overall network. Furthermore, the average clustering coefficient of NGlobal (
) is significantly higher than that of NRandom (
) and NSmall (
), indicating a substantial clustering effect in NGlobal, which serves as a manifestation of the cyber division phenomenon. Additionally, NGlobal exhibits a slightly higher average shortest path length (
) than NSmall (
). These results imply that the small-world network might not be the most appropriate descriptor for the cyber human activity network. This finding diverges from those of studies on physical human activity networks where the small-world characteristic is more prominent.
Figure 9. (a) Complementary cumulative distribution functions (CDF) of the node degree, and (b) complementary CDF of the betweenness centrality.

Scaling relations are applied to investigate the structure of NGlobal, and there are two types of scaling relations that are used for this, as shown in . Because the scaling relations in small-world networks are highly influenced by the rewiring probability (Newman Citation2005; Xia, Fan, and Hill Citation2010), NSmall is not included in the analysis of scaling relations. According to (a), on a log–log plot, the betweenness centrality plotted against degree follows a linear relationship, which is depicted by the red line corresponding to with
. The observation of a
that is larger than
in NRandom and NScale, respectively, suggests that the betweenness centrality in NGlobal increases at a faster rate than that in NRandom and NScale, especially for the larger degree nodes. In other words, the influence of highly connected nodes on the network increases disproportionately as the connection of nodes increases, which implies that it is easier for webs that are well connected in the human activity network to further increase their influence in information propagation. However, for newly established webs, establishing a foothold in the current market is more challenging. This nonbeneficial competition might lead to a monopolistic situation where several webs come to dominate cyber space.
Figure 10. (a) The scaling relation between degree and betweenness centrality and (b) the scaling relation between degree and clustering coefficient.

(b) describes another scaling relation between the degree and clustering coefficients. Generally, the scaling relation can be divided into two parts based on degree. For small degrees, the clustering coefficient is independent of degree (), which is similar to that in NRandom and NScale. Regarding the large degrees, the appearance of a decreasing relation suggests that denser areas do not correspond to higher connectivity. Then, the decreasing relation is compared with the form
, which has been shown to represent complete hierarchical polycentric networks (Ravasz and Barabási Citation2003). A hierarchical polycentric structure indicates that a highly clustered area consists of several sparsely distributed areas connected by only a few hubs. The high similarity between the empirical data and the complete hierarchical polycentric networks suggests that there exist hierarchically polycentric groups for large degree nodes in cyber space. These findings imply that human activities in cyber spaces are mostly clustered within these polycentric groups, which makes them important to information dissemination and network security.
5.3. Properties of the space-dependent cyber human activity networks
Based on previous research on NGlobal, this subsection delves deeper into the spatial characteristics of the cyber human activity network by using space-dependent cyber human activity networks (NSpace). displays the results of the statistical measures and coefficients used to describe the scaling relations for NSpace. First, considering the number of nodes and edges and their averages, Changchun and Jilin City exhibit significantly higher values than the other seven cities, suggesting that Changchun and Jilin City are involved in more diverse and frequent cyber activities. This polarized distribution of nodes and edges across cities is similar to the socioeconomic disparities observed in Jilin Province, where Changchun and Jilin City prominently stand out. Furthermore, the clustering level of the cyber human activity network also falls into two groups according to clustering coefficients, with Jilin City, Siping, Songyuan, and Changchun belonging to the high clustering group. This clustering categorization, which is different from the node and edge categorization, implies that cyber human activity, under the same logic as physical human activity, is also influenced by diverse factors beyond simply the socioeconomic levels of cities. (a,b) shows the complementary cumulative distribution functions (CDF) of degree and betweenness centrality through a logarithmic plot, where different colors correspond to different cities. It is evident that the trends of these two complementary CDFs are similar to those of NGlobal, following heavy-tailed distributions and implying that the cyber divisions are not restricted by space. For the coefficients of complementary CDF (), the coefficients of degree distributions for all nine cities are smaller than those for NGlobal, whereas the betweenness centrality coefficients are larger than those for NGlobal (). Concerning the scaling relation, as depicted in (c, d), the overall distributions are also similar to those in NGlobal, indicating that regardless of the city, cyber human activity networks always exhibit hierarchical polycentric structures. In terms of magnitude, the scaling coefficients between degree and betweenness centrality for all cities are smaller than those for NGlobal (
), as displayed in . In summary, the basic statistical measures of cyber human activity networks exhibit spatial polarization across cities in terms of nodes, edges, and clustering coefficients. However, the network structures display high similarity across space, which is similar to NGlobal. This finding suggests that spatial information has a limited impact on cyber human activities, further confirming that unlike physical space, cyber space is not constrained by spatial distance.
Figure 11. (a) Complementary cumulative distribution function (CDF) distributions of betweenness centrality, (b) complementary CDF distributions of degree, (c) scaling relation between degree and betweenness centrality, and (d) scaling relation between degree and clustering coefficient in the NSpace.

Table 6. The measures of nine space-dependent cyber human activity networks.
The spatial results for the nine cities are also included and presented in . This figure displays the spatial distributions of average statistical measures (degree, betweenness centrality, and clustering coefficients) in the nine cities. It is evident that spatial autocorrelation is present in all three maps. Regarding the spatial patterns, average degree and clustering coefficients exhibit similar spatial distributions, delineated by a boundary line from the northeast to the southwest. Regions north of the boundary line demonstrate high average degree and clustering coefficients, while those south of the boundary line show low average degree and clustering coefficients. However, the spatial distribution of betweenness centrality is opposite, with cities in the southeast displaying high betweenness centrality. This divergent spatial distribution may be linked to the definitions and meanings represented by these statistical measures or may be associated with other socio-economic factors.
5.4. Properties of the motif-dependent cyber human activity networks
Based on the top 14 motifs, 14 motif-dependent cyber human activity networks (NMotif) are constructed and then compared from two aspects, namely, statistical measures and scaling relations, which can reflect the heterogeneities in the activity patterns. summarizes the results of the statistical measures for these 14 networks. First, the findings reveal differences among the NMotif in terms of their node numbers, indicating varying demands for web domains within these motifs. The complementary CDF plot in illustrates the node degree and betweenness centrality distributions for each NMotif, showing that all 14 NMotif follow power-law distributions. However, the rate parameters of these distributions differ, as summarized in . For example, M-1-1 exhibits a power-law behavior in its degree distribution but with a relatively large rate parameter that does not indicate a scale-free network. On the other hand, the other 13 NMotifs display scale-free networks with different decay rates. In terms of betweenness centrality, all NMotifs display power-law distributions for larger betweenness centrality, similar to that in NGlobal but with different decay rates. Overall, these characteristics closely resemble the global cyber human activity network, suggesting a scale-free structure for cyber space regardless of the different activity patterns. The scale-free structure of cyber space highlights the importance of certain nodes in shaping the overall flow of information and interactions in cyber space.
Figure 13. (a) The distribution of the complementary cumulative distribution function (CDF) distributions of betweenness centrality and (b) the complementary CDF distributions of degree in the 14 NMotifs.

Table 7. The statistical measures of 14 motif-dependent cyber human activity networks.
(a) describes the scaling relation between the degree and betweenness centrality of the NMotifs in a logarithmic plot, where different colors correspond to different NMotifs. displays the scaling exponents, which range from 1.571–2.160. The observation of all exponents that are larger than 1 indicates a superlinear increase in betweenness centrality with node degree, which is the same as that in NGlobal. The findings imply that unbalanced competition among relatively well-developed web domains and newly created domains exists within every activity pattern. As a result, a monopolistic situation is possible for all activity patterns, which hinders the further structural improvement of cyber space.
Figure 14. (a) Scaling relation between degree and betweenness centrality for different NMotifs, and (b) scaling relation between degree and clustering coefficient for different NMotifs.

Table 8. The scaling exponents between betweenness centrality and degree for 14 motif-dependent cyber human activity networks.
The scaling relation between the degree and clustering coefficient for the 14 NMotifs are shown in (b). The 14 distributions share similar patterns but deviate in terms of degree. For smaller degrees, the clustering coefficient is approximately consistent, while decreasing trends between the clustering coefficient and degree are observed for larger degrees. Additionally, the extent to which they resemble the complete hierarchical structure differs for large degrees among the 14 NMotifs, which indicates that different NMotifs have different evolutionary structural states and those activity patterns that are further away from the complete hierarchical structure are more unstable, and the webs in these activity patterns are more prone to replacement over time.
6. Discussion and conclusion
Quantitative measures used to characterize human activity can provide a deeper understanding of the structure of such activities. Motivated by complex network theory and the availability of massive human internet records, a new framework is proposed in this study to explore the structure of cyber space from the perspective of a cyber human activity network. In contrast to previous studies that have primarily examined physical space structures, this study not only focuses on a rarely studied space, namely, cyber space, but also further explores the spatial patterns of cyber human activity using multiple cyber human activity networks in different cities and the impacts of the different cyber activity patterns on the structure of cyber space. The different cyber activity patterns are represented by different activity-based motifs and the corresponding multiple cyber human activity networks. These cyber human activity networks are evaluated through two aspects: statistical measures and scaling relations. By applying this framework to Jilin Province, its effectiveness in understanding the structure of cyber space is demonstrated in this study.
Through an examination of activity-based motifs, the findings indicate a strong regularity and clustering of cyber activity patterns, which is similar to those of activities observed in physical space (Cao et al. Citation2021; Gao et al. Citation2018). Additionally, both physical and cyber spaces are dominated by simple motifs. The consistency of these patterns between the two spaces suggest the stability of human activity independent of the activity space in which it occurs, contributing further evidence to the literature in regard to the consistent nature of human behavior across of spatial contexts. However, there are notable differences in the spatial distribution of motifs between physical and cyber spaces compared to that of previous studies. Previous research has indicated that simple physical activity patterns tend to cluster within suburban areas, whereas this study suggests that simple cyber activity patterns are more concentrated within metropolitan areas. This disparity may arise from the uneven distribution of resources and relatively poorer infrastructure in suburban regions. Therefore, it is essential to focus on resource reallocation and accelerate the pace of urbanization, which aligns with the nation's major goals formed in recent decades. Moreover, the spatial heterogeneity of simple activity patterns between physical and cyber spaces might serve as an indicator for policy-makers of the degree of urbanization.
Second, the heavy-tailed distributions observed for network degree and betweenness centrality provide evidence to support the claim of cyber division, regardless of the different activity patterns or cities. Cyber division refers to a scenario where a few websites receive a disproportionately large number of visits, while the majority of websites only receive a few visits. This imbalance significantly impacts the efficiency of information propagation, meaning that information on these highly visited websites can spread faster and reach a larger audience than information on less visited websites. Furthermore, the hierarchically polycentric structures of cyber human activity networks, as revealed by the scaling relation between the clustering coefficient and degree, offer valuable insights to inform information diffusion strategies for different purposes. For instance, in cases where information needs to rapidly reach a large audience, such as in advertising, disaster warnings, or business recommendation algorithms, targeting the central websites in every polycentric structure would be more effective. This approach can minimize advertising costs or reduce the human and financial losses resulting from disasters. Moreover, the cyber division and hierarchically polycentric structures also have implications for enhancing the safety of cyber networks. While the hierarchically polycentric structure can improve the robustness of cyber networks, it is crucial to be cautious about the potential spread of viruses through these trajectories. Therefore, careful attention and focus on central websites and hub websites that connect different polycentric structures are necessary to avoid potential risks. Furthermore, the consistent structure of the cyber human activity network across different regions also highlights the limited influence of factors such as distance on cyber space as compared to that on physical space. Additionally, the small-world characteristics widely prevalent in physical space are not as prominent in cyber space compared to other network characteristics, such as the heavy tail. These findings imply that while many similarities exist between human activities in cyber and physical spaces, as confirmed by this study, it is crucial to pay attention to these differences and explore the underlying reasons in subsequent research.
Third, the findings reveal a nonlinear relationship between degree and betweenness centrality, indicating that the influence of highly connected websites increases at a faster rate than that of less connected websites regardless of regions and cyber activity patterns. This disproportionate increase in influence can lead to monopolization by certain website companies, which is detrimental to maintaining a healthy market economy. To avoid this issue, the relevant government authorities must implement policies to protect newly established companies, thereby promoting fair market competition and preventing the dominance of a few websites.
Nevertheless, a few limitations need to be acknowledged. First, it is understandable that the weighted networks can better reflect the flows between nodes. However, flows are greatly influenced by fluctuations that occur in shorter-term datasets, and short-term datasets are not reliable enough to depict the dynamic characteristics of flows in networks. Therefore, a greater focus is placed in this study on exploring the topological characteristics of networks. However, the increasing availability of longer-term datasets may offer solutions for addressing this limitation in future research. Moreover, the use of longer-term datasets can also provide insights into the temporal variations of the time intervals used in our study, aiming to reflect the temporal fragmentation of cyber access. For instance, the time intervals may differ for different days, weeks, or months, which could represent certain activity patterns. Furthermore, these time-series data also enable the dynamic monitoring of cyber human activity networks across various time scales. Over a longer timescale, differences between workdays and weekends, as well as weekly or monthly variations, can be investigated. Additionally, examining the differences before and after the pandemic is also valuable. Over a shorter timescale, the use of longer datasets can compensate for the uneven distribution of data within different periods observed in single-day data. They can also address the occasionalism and data biases present in single-day data. In addition to delving deeper into the temporal aspects, the use of longer-term datasets can also provide instant guidance on adjusting the information propagation strategy, which could further optimize the efficiency of information propagation for specific purposes.
Acknowledgments
All authors contributed critically to the draft and gave final approval for publication. The authors would like to thank the editors and reviewers for their valuable comments, and suggestions.
Disclosure statement
No potential conflict of interest was reported by the author(s).
Data availability statement
The mobile phone data used in the study cannot be shared publicly due to restrictions.
Additional information
Funding
References
- Adamic, Lada A., Rajan M. Lukose, Amit R. Puniyani, and Bernardo A. Huberman. 2001. “Search in Power-Law Networks.” Physical Review E 64: 4. APS: 046135.
- Albert, Réka, and Albert-László Barabási. 2002. “Statistical Mechanics of Complex Networks.” Reviews of Modern Physics 74: 1. American Physical Society: 47–97. doi:10.1103/RevModPhys.74.47.
- Alexander, Bayarma, Dick Ettema, and Martin Dijst. 2010. “Fragmentation of Work Activity as a Multi-Dimensional Construct and Its Association with ICT, Employment and Sociodemographic Characteristics.” Journal of Transport Geography 18 (1): 55–64. doi:10.1016/j.jtrangeo.2009.05.010.
- Alexander, Bayarma, Christa Hubers, Tim Schwanen, Martin Dijst, and Dick Ettema. 2011. “Anything, Anywhere, Anytime? Developing Indicators to Assess the Spatial and Temporal Fragmentation of Activities.” Environment and Planning B: Planning and Design 38 (4), SAGE Publications Sage UK: London, England: 678–705.
- Barthélemy, Marc. 2004. “Betweenness Centrality in Large Complex Networks.” The European Physical Journal B - Condensed Matter 38 (2): 163–168. doi:10.1140/epjb/e2004-00111-4.
- Batty, Michael. 1993. “The Geography of Cyberspace.” Environment and Planning B: Planning and Design 20 (6): 615–616. doi:10.1068/b200615.
- Batty, Michael. 1997. “Virtual Geography.” Futures 29 (4–5), Elsevier: 337–352.
- Batty, Michael. 2013. The New Science of Cities. Cambridge: MIT Press.
- Ben-Elia, Eran, Bayarma Alexander, Christa Hubers, and Dick Ettema. 2014. “Activity Fragmentation, ICT and Travel: An Exploratory Path Analysis of Spatiotemporal Interrelationships.” Transportation Research Part A: Policy and Practice 68 (October): 56–74. doi:10.1016/j.tra.2014.03.016.
- Benedikt, Michael. 1991. Cyberspace: First Steps. Cambridge: MIT Press.
- Boos, Tobias. 2017. Inhabiting Cyberspace and Emerging Cyberplaces: The Case of Siena, Italy. New York: Springer.
- Cao, Jinzhou, Qingquan Li, Wei Tu, Qili Gao, Rui Cao, and Chen Zhong. 2021. “Resolving Urban Mobility Networks from Individual Travel Graphs Using Massive-Scale Mobile Phone Tracking Data.” Cities 110 (March): 103077. doi:10.1016/j.cities.2020.103077.
- Cao, Jinzhou, Qingquan Li, Wei Tu, and Feilong Wang. 2019. “Characterizing Preferred Motif Choices and Distance Impacts.” PLOS ONE 14: 4. Public Library of Science: e0215242. doi:10.1371/journal.pone.0215242.
- Clauset, Aaron, Cosma Rohilla Shalizi, and M. E. J. Newman. 2009. “Power-Law Distributions in Empirical Data.” SIAM Review 51 (4): 661–703. doi:10.1137/070710111.
- Couclelis, Helen. 1998. “The new field workers.” Environment and Planning B: Planning and Design 25 (3): 321–323.
- Couclelis, Helen. 2003. “Housing and the New Geography of Accessibility in the Information Age.” Open House International 28 (4): 7–13.
- Couclelis, Helen. 2004. “Pizza over the Internet: E-Commerce, the Fragmentation of Activity and the Tyranny of the Region.” Entrepreneurship & Regional Development 16 (1): Taylor & Francis: 41–43.
- Dodge, Martin. 2001. “Cybergeography.” Environment and Planning B: Planning and Design 28 (1), SAGE Publications Sage UK: London, England: 1–2.
- Dorogovtsev, Sergei N., and José FF Mendes. 2003. Evolution of Networks: From Biological Nets to the Internet and WWW. Oxford, New York: Oxford University Press.
- Fahrig, Lenore. 2019. “Habitat Fragmentation: A Long and Tangled Tale.” Global Ecology and Biogeography 28 (1): 33–41. doi:10.1111/geb.12839.
- Floyd, Sally, and Vern Paxson. 2001. “Difficulties in Simulating the Internet.” IEEE/ACm Transactions on Networking 9 (4), IEEE: 392–403.
- Franklin, Seb. 2015. Control: Digitality as Cultural Logic. Cambridge: MIT Press.
- Frieze, Alan, and Michał Karoński. 2016. Introduction to Random Graphs. Cambridge: Cambridge University Press.
- Gao, Qi-Li, Qing-Quan Li, Yang Yue, Yan Zhuang, Zhi-Peng Chen, and Hui Kong. 2018. “Exploring Changes in the Spatial Distribution of the low-to-Moderate Income Group Using Transit Smart Card Data.” Computers, Environment and Urban Systems 72 (November): 68–77. doi:10.1016/j.compenvurbsys.2018.02.006.
- Gibson, William. 1984. Neuromancer. London: Ace Books.
- Hägerstrand, Torsten. 1989. “Reflections on ‘What about People in Regional Science?’.” Papers of the Regional Science Association 66 (1): 1–6. doi:10.1007/BF01954291.
- Hazarie, Surendra, Hugo Barbosa, Adam Frank, Ronaldo Menezes, and Gourab Ghoshal. 2020. “Uncovering the Differences and Similarities Between Physical and Virtual Mobility.” Journal of The Royal Society Interface 17: 168. Royal Society: 20200250. doi:10.1098/rsif.2020.0250.
- Hillier, Bill. 2007. Space Is the Machine: A Configurational Theory of Architecture. Space Syntax. https://discovery.ucl.ac.uk/id/eprint/3881/.
- Hillier, Bill, and Julienne Hanson. 1989. The Social Logic of Space. Cambridge: Cambridge University Press.
- Hillier, B., A. Leaman, P. Stansall, and M. Bedford. 1976. “Space Syntax.” Environment and Planning B: Planning and Design 3 (2): 147–185. doi:10.1068/b030147.
- Huang, Chin-Hui. 2001. “A Preliminary Study of Spatializing Cyberspace.” CAADRIA2001, Key Centre of Design Computing and Cognition, University of Sydney, Australia, 27–37.
- Jiang, Bin. 2013. “Head/Tail Breaks: A New Classification Scheme for Data with a Heavy-Tailed Distribution.” The Professional Geographer 65 (3): 482–494. doi:10.1080/00330124.2012.700499.
- Kademi, Anas Mu’azu. 2020. “Formal Perspectives on Conceptualization of Cyberspace.” International Journal of Advanced Trends in Computer Science and Engineering 9 (4): 6111–6120. doi:10.30534/ijatcse/2020/282942020.
- Kademi, Anas Mu’azu, and Ahmet Hasan Koltuksuz. 2020. “Dynamic Cyberspace Modeling from Network Automata.” International Journal of Computer Science and Network Security 20 (12): 30–41. doi:10.22937/IJCSNS.2020.20.12.4.
- Kademi, Anas Mu’azu, and Ahmet Hasan Koltuksuz. 2021. “Cyber-Physical-Social-Information-Thinking Hyperspace: A Manifold of Cyberspatial Entities.” Kybernetes 52: 3. Emerald Publishing Limited: 921–936. doi:10.1108/K-03-2021-0198.
- Kwan, Mei-Po. 2001. “Cyberspatial Cognition and Individual Access to Information: The Behavioral Foundation of Cybergeography.” Environment and Planning B: Planning and Design 28 (1), SAGE Publications Sage UK: London, England: 21–2.
- Liu, Zhicheng, Jinbin Yu, Weiting Xiong, Jian Lu, Junyan Yang, and Qiao Wang. 2016. “Using Mobile Phone Data to Explore Spatial-Temporal Evolution of Home-Based Daily Mobility Patterns in Shanghai.” In 2016 International Conference on Behavioral, Economic and Socio-Cultural Computing (BESC), 1–6. IEEE. https://ieeexplore.ieee.org/abstract/document/7804481/.
- Lodish, Harvey, Arnold Berk, Chris A. Kaiser, C. Kaiser, M. Krieger, M. P. Scott, A. Bretscher, and H. Ploegh. 2008. “325 PMatsudaira, et Al.” Molecular Cell Biology. Macmillan 326.
- Newman, M. E. J. 2003. “The Structure and Function of Complex Networks.” SIAM Review 45 (2): 167–256. doi:10.1137/S003614450342480.
- Newman, M. E. J. 2005. “A Measure of Betweenness Centrality Based on Random Walks.” Social Networks 27 (1): 39–54. doi:10.1016/j.socnet.2004.11.009.
- Newman, Mark. 2018. Networks. Oxford: Oxford University Press.
- Parthasarathi, Pavithra. 2014. “Network Structure and Metropolitan Mobility.” Journal of Transport and Land Use 7 (2), JSTOR: 153–170.
- Pastor-Satorras, Romualdo, Alexei Vázquez, and Alessandro Vespignani. 2001. “Dynamical and Correlation Properties of the Internet.” Physical Review Letters 87: 25. APS: 258701.
- Pastor-Satorras, Romualdo, and Alessandro Vespignani. 2001. “Epidemic Spreading in Scale-Free Networks.” Physical Review Letters 86 (14): 3200–3203. doi:10.1103/PhysRevLett.86.3200.
- Ravasz, Erzsébet, and Albert-László Barabási. 2003. “Hierarchical Organization in Complex Networks.” Physical Review E 67 (2): 026112. doi:10.1103/PhysRevE.67.026112.
- Rutledge, Daniel Thomas. 2003. “Landscape Indices as Measures of the Effects of Fragmentation: Can Pattern Reflect Process?” Department of Conservation Wellington.
- Saramäki, Jari, Mikko Kivelä, Jukka-Pekka Onnela, Kimmo Kaski, and János Kertész. 2007. “Generalizations of the Clustering Coefficient to Weighted Complex Networks.” Physical Review E 75 (2): 027105. doi:10.1103/PhysRevE.75.027105.
- Shi, Wenzhong, Michael Goodchild, Michael Batty, Qingquan Li, Xintao Liu, and Anshu Zhang. 2022. “Prospective for Urban Informatics.” Urban Informatics 1 (1): 2. doi:10.1007/s44212-022-00006-0.
- Sui, Daniel Z. 2004. “GIS, Cartography, and the ‘Third Culture’: Geographic Imaginations in the Computer Age.” The Professional Geographer 56 (1): 62–72. doi:10.1111/j.0033-0124.2004.05601008.x.
- Tran, Martino, and Christina Draeger. 2021. “A Data-Driven Complex Network Approach for Planning Sustainable and Inclusive Urban Mobility Hubs and Services.” Environment and Planning B: Urban Analytics and City Science 48 (9): 2726–2742. doi:10.1177/2399808320987093.
- Tu, Wei, Rui Cao, Yang Yue, Baoding Zhou, Qiuping Li, and Qingquan Li. 2018. “Spatial Variations in Urban Public Ridership Derived from GPS Trajectories and Smart Card Data.” Journal of Transport Geography 69 (May): 45–57. doi:10.1016/j.jtrangeo.2018.04.013.
- Wang, Minjie, Su Yang, Yi Sun, and Jun Gao. 2017. “Discovering Urban Mobility Patterns with PageRank Based Traffic Modeling and Prediction.” Physica A: Statistical Mechanics and Its Applications 485 (November): 23–34. doi:10.1016/j.physa.2017.04.155.
- Watts, Duncan J., and Steven H. Strogatz. 1998. “Collective Dynamics of ‘Small-World’ Networks.” Nature 393 (6684), Nature Publishing Group: 440–442. doi:10.1038/30918.
- Xia, Yongxiang, Jin Fan, and David Hill. 2010. “Cascading Failure in Watts–Strogatz Small-World Networks.” Physica A: Statistical Mechanics and Its Applications 389 (6): 1281–1285. doi:10.1016/j.physa.2009.11.037.
- Xu, Jianguo. 2022. “Developments and Implications of Central Bank Digital Currency: The Case of China E-CNY.” Asian Economic Policy Review 17 (2): 235–250. doi:10.1111/aepr.12396.
- Zhang, Yuyang, Yan Li, Enjia Zhang, and Ying Long. 2022. “Revealing Virtual Visiting Preference: Differentiating Virtual and Physical Space with Massive TikTok Records in Beijing.” Cities 130, Elsevier: 103983.
- Zhao, Zhi-Dan, Zi-Gang Huang, Liang Huang, Huan Liu, and Ying-Cheng Lai. 2014. “Scaling and Correlation of Human Movements in Cyberspace and Physical Space.” Physical Review E 90 (5): 050802. doi:10.1103/PhysRevE.90.050802.