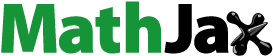
ABSTRACT
Geographic processes are dynamic, wherein geographical entities and phenomena change over time and are characterized by vagueness. However, most studies on geographic processes employ precise expression methods to describe and express the changing trajectories of geographical entities and phenomena, ignoring vagueness. This strategy fails to facilitate the objective description and expression of the entire process and may affect analysis results. Herein, a time feature expression model of vague geographic processes is established based on the interval type-2 fuzzy set theory to describe and express the vague time characteristics comprising three model stages that reflect the geographic process evolution sequence: production (beginning), development, and extinction (end). Finally, the practicability of the proposed model is verified using two case studies, i.e. the landing process of typhoon ‘Lekima’ and the desert greening process in Xinjiang, China.
1. Introduction
The increased adoption of advanced sensors in the collection of geospatial data enables researchers to engage with both spatial and temporal patterns in complex geographic processes (Goodchild, Yuan, and Cova Citation2007; McIntosh and Yuan Citation2005). The dynamic changes in the spatial characteristics of geographical entities and phenomena, such as the position, geometric shape, semantics, attributes, and interrelationships, which occur over time under the influence of external forces, are collectively referred to as geographic processes (He et al. Citation2023) and comprise one or more evolution sequences. Each sequence can be divided into three stages: production (beginning), development, and extinction (end) (Kjenstad Citation2006; Xue et al. Citation2019; Yu, Yang, and Jin Citation2018). Expressing geographic processes with appropriate methods is a prerequisite for exploring their underlying mechanisms and advancing prediction and forecasting research (Li et al. Citation2014). The changing directions of different geographical features in these processes may be inconsistent, with the time characteristics having non-plausibility and non-reversibility. This common element permeates the entire state change in the geographic process. Therefore, its accurate description and expression are the basis of reliably describing geographic processes.
Most researchers use precise expression methods to examine geographic processes by describing and expressing the changes in geographical entities and features of the phenomena (Tang and Zhang Citation2018). However, in some geographic processes, changes occur in the nature of geographical entities and phenomena. For example, during grassland desertification, the grassland area gradually decreases while the desert area increases, resulting in a corresponding change in the semantic and attribute characteristics. Depicting the geographic processes according to the changing features of geographical entities and phenomena involves altering the research subject, which can cause omissions and errors during complex geographic processes. Complexity is an objective property of geographical processes, including internal changes in geographical features that cannot be observed directly from the surface or simultaneous changes in two seemingly unrelated geographical features, forming a combined geographical process. Furthermore, several features of geographical entities and phenomena (e.g. the location, geometric form, semantics, attribute, and interrelationships) in the real world are often fuzzy; for example, the location, area, and vegetation type of a forest cannot be precisely expressed (Yin et al. Citation2022).
Furthermore, different users apply different methods to describe the geographic process, inevitably introducing uncertainty due to the influence of subjective factors. The uncertain expression of a fuzzy geographic process is referred to as vagueness and designated a vague geographic process (Yin et al. Citation2022). The vague expression of geographic processes can partially quantify the complexity of vagueness in the processes and associated applications, such as monitoring changes in land use (Jixing et al. Citation2021; Fisher Citation2010), segregation (Huck et al. Citation2023), coastal development (Urbanski Citation1999) and land suitability evaluation (Burrough Citation1989). New methods of describing and expressing vague geographic processes from the perspective of time features are required to objectively restore the geographical process in the model based on its real-world characteristics and provide a truer basis for predicting subsequent geographic processes.
The fuzzy set (FS) theory proposed by Zadeh (Citation1965) provides a powerful tool for describing and expressing fuzzy geographic objects and phenomena. Related studies have been conducted in numerous fields, including land use classification, segregation, image segmentation, and neural network training (Huck et al. Citation2023; Hwang and Rhee Citation2007; Yin et al. Citation2022). However, current studies describing and expressing vague geographic processes based on the FS theory have the following limitations. First, the FS theory uses a precise value to express the degree of an element belonging to a specific set; it cannot measure the membership error and fails to objectively describe and express vague geographical entities, phenomena, or geographic processes (Guo et al. Citation2018; Yin et al. Citation2022). Second, most studies have described the geographic process according to the changing geographical entities and phenomena, which can lead to omission and confusion during complex geographic processes (Abbasi et al. Citation2020). Furthermore, studies describing and expressing the production (beginning), development, and extinction (end) stages of geographic processes from the perspective of time features are lacking.
As a newly proposed theory, the interval type-2 fuzzy set theory (IT2 FS) provides a valuable tool for describing and expressing vague geographic processes. As an extension of the FS theory, IT2 FS involves upper membership function (UMF) and lower membership function (LMF) (Hwang and Rhee Citation2007), which can measure membership errors and are thus more suitable for describing and expressing the processes of vague geographical entities and phenomena (Guo, Shao, and Mo Citation2018). Numerous researchers have used IT2FS to express vague geographical spatiotemporal objects; however, less attention has been paid to the expression methods for ambiguous geographical processes. In the present study, a model based on the IT2 FS is proposed to express vague geographic processes from the perspective of time features and verify the practicality and performance of the proposed method in case studies describing the landing process of typhoon ‘Lekima’ and the desert greening process in Xinjiang, China. The proposed method improves the GIS expression ability for the geographical process, more realistically restoring the process while establishing a foundation for subsequent GIS analysis, considering vagueness and exploring its driving mechanism.
The remainder of this article is structured as follows. Section 2 provides background on the vagueness and uncertainty of geographic processes and describes the FS theory. Section 3 describes the methodological framework and model construction concerning the expression of fuzziness and temporal characteristics of vague geographic processes. Section 4 uses the landing process of typhoon ‘Lekima’ and the desert greening process in Xinjiang, China, as examples to demonstrate the implementation process of the proposed method and verify the accuracy of the expression results. Finally, Section 5 summarizes our findings and provides possible future research directions.
2. Related work
The early dynamic expression of geographic processes was realized by describing the change in a single geographical element at a specific time and place (Grenon and Smith Citation2004; Raper and Livingstone Citation1995). Although current research on geographic processes has advanced, a theoretical system is not yet established as most approaches can only determine time, location, and attributes while failing to fully express the spatiotemporal relationship between the characteristics of geographical entities and phenomena (Kavouras Citation2001; Pang and Shi Citation2002; Worboys Citation2001). Many scholars have contributed to improving the theoretical framework of geographic processes (Li, Klippel, and Yang Citation2011; Worboys and Hornsby Citation2004; Xie and Xue Citation2009). However, the description or expression of the time feature of geographic processes has been neglected.
Over the past decade, studies on the description and expression of geographic processes from the perspective of time features have increased (Devaraju, Kuhn, and Renschler Citation2015; Petnga and Austin Citation2013; Romero Citation2013; Tang and Zhang Citation2018). Nonetheless, these studies have focused exclusively on the time feature of geographic processes, neglecting their dynamic nature, including the production (beginning), development, and extinction (end) stages. Additionally, studies based on precise time features have failed to describe and express the vagueness of geographic processes.
FS is a set composed of objects with a continuous membership degree, in which membership functions assign a value between 0 and 1 to each element (Zadeh Citation1965). Many researchers, such as Burrough (Citation1989), and Cross and Firat (Citation2000), have successfully used the FS to model and represent fuzzy geographical entities and phenomena. However, inevitable subjective uncertainties can be introduced owing to differences in the collection environment, participants, methods, and other factors while collecting and processing geographic elements so that the final results contain membership errors (Guo, Shao, and Mo Citation2018). Consequently, Zadeh (Citation1975) proposed the type-2 fuzzy set (T2 FS), which adds a secondary membership function with a value of (0,1) based on FS to describe the uncertainty of the main membership function. High-order fuzziness is common in GIS (Fisher Citation2010); therefore, using the theory of the higher-order FS is more objective to study vague spatiotemporal objects (Guo et al. Citation2018). However, such approaches have overlooked the complexity of high-order geographic operations and, thus, remain in the theoretical stage without practical application.
To resolve the issue of membership error and achieve practical application, Liang and Mendel (Citation2000) proposed IT2 FS, a unique version of T2 FS, in which the secondary membership function is 1, dramatically reducing the computational complexity of T2 FS and expressing the range of membership function values with UMF and LMF instead of a single value (Sola et al. Citation2015). Geographical objects inevitably introduce subjective uncertainty during collection and processing and are influenced by subjective uncertainty. The suitable range of spatiotemporal objects is not an exact value but an interval. Therefore, IT2 FS has gradually displaced FS theory as a mainstream tool for studying vague problems (Guo, Shao, and Mo Citation2018; Sola et al. Citation2015; Yin et al. Citation2022). The ability to measure membership error and ease of interpretation makes the method more suitable for practical applications. Therefore, the present study describes and expresses geographic processes characterized by vagueness from the perspective of time features based on IT2 FS.
3. Methodology
3.1. Geographic process
Based on the classification of processes proposed by Sowa (Citation1996), geographic processes can be divided into continuous and discrete (). Continuous geographic processes include three stages: production (beginning), development, and extinction (end). Discrete geographic processes describe a sudden change in the characteristics of a geographic entity or phenomenon comprising a series of geographic events or states. Discrete geographic processes have no phase-wise development; the production (beginning) point coincides with the extinction (end) point.
Geographic processes can also be divided into quantitative and qualitative:
Quantitative: In a quantitative geographic process, the characteristic value of a geographic entity or phenomenon gradually changes over time, whereas its actual characteristics remain unchanged. For example, during a typhoon landfall, the magnitude of the pressure value at the landfall site changes.
Qualitative: In a qualitative geographic process, the characteristics of a geographic entity or phenomenon gradually change over time until they are transformed into a different entity or phenomenon. For instance, grassland is gradually transformed into a desert under high wind, water erosion, and soil salinization ().
Figure 2. Composition of a geographic process: (a) An event (or state) with a sudden change in the characteristics of a geographical entity or phenomenon; (b) quantitative geographical processes that only involve changes in one characteristic; (c) qualitative geographical process, in which the characteristic of Sub 1 changes over time until it transforms into the characteristic of Sub 2; (d) composite geographical process that can be expressed using the feature changes of Sub 3 and Sub 4.

3.2. Expression of fuzzy geographic process
In the real world, geographic processes are crisp and vague and can transform with scale changes. Therefore, we retained the definition of a crisp geographic process and extended it fuzzily. The membership degrees of each production (beginning), development, and extinction (end) stage are equal to 1 in the crisp geographic processes. However, these three stages can coexist with different membership degrees in fuzzy geographic processes, and the sum of the membership degrees of each part is equal to 1.
Fuzzy geographic processes have no clear boundaries between the production (beginning), development, and extinction (end) phases. Instead, they exist simultaneously with different membership degrees. Based on the fuzzy classification of the change in the characteristics of geographical entities and phenomena in a fuzzy geographic process by FS, the membership degree of each change value of the process can be obtained. A series of membership degrees in the time sequence constitutes the time series of the fuzzy geographic process expression model, as shown in .
Figure 3. Expression model of the fuzzy geographical process. The y-axis, μ(p), is the membership degree of the characteristics of the geographical entities and phenomena that constitute the time series belonging to geographic process A. The y-axis indicates time. (a) The fuzzy geographic process; , and
are the production (beginning), development, and extinction (end) phases of fuzzy geographic process A, respectively. Each time point belongs to each phase of the fuzzy geographic process with a certain membership degree. (b) The fuzzy geographic event, which is the particular case when
in the fuzzy geographic process.

The calculation formula for membership function is presented in Equation (1):
(1)
(1)
The membership degree of each stage can be expressed by Equations (2)−(4):
Production phase (beginning):
(2)
(2)
Development phase:
(3)
(3)
Extinction phase (end):
(4)
(4)
3.3. Expression of vague geographic processes
The characteristics of geographical entities and phenomena in many geographic processes are inherently fuzzy (Guo et al. Citation2018; Yin et al. Citation2022). When collected, processed, stored, and analyzed, uncertainty is inevitably introduced owing to different users, equipment, and methods. Hence, the final geographic process is an uncertain expression of fuzzy geographic process, referred to as the vague geographic process. Combining IT2 FS to extend the fuzzy geographic process expression model, UMF and LMF are used to jointly determine the membership range of each time point belonging to each phase of the vague geographic process.
By classifying the feature values of geographical entities and phenomena in the geographic process based on IT2 FS, we can obtain their membership UMF and LMF belonging to the vague geographic process. The time series composed of a series of UMF and LMF is the time feature expression model of the vague geographic process, as shown in .
Figure 4. Time expression model of the geographic process based on IT2 FS. The y-axis, , is the membership of the characteristics of the geographical entities and phenomena that constitute the time series belonging to geographic process A; the x-axis indicates time;
and
are the UMF (yellow line) and LMF (green line) of
, and
, respectively, and
. Here,
and
denote the production (beginning), development, and extinction (end) phases of vague geographic process A, respectively. The vague geographic event is a special case of a vague geographic process when
.

The duration of the vague geographic process is set as , where
is the phase before production (beginning),
is the production (beginning) phase,
is the development phase,
is the extinction (end) phase, and
is the phase after extinction (end). The membership of each stage can be expressed by Equations (5) – (9):
Minimum degree of production (beginning):
(5)
(5)
Maximum degree of production (beginning):
(6)
(6)
Development phase:
(7)
(7)
Maximum degree of extinction (end):
(8)
(8)
Minimum degree of extinction (end):
(9)
(9) where
,
,
,
, and
denote the membership of the phase before the production (beginning), production (beginning), development, extinction (end), and after extinction (end), respectively.
A qualitative change in the geographical process can comprise two or more quantitative changes. The sum of the membership degrees of two or more quantitative changes in the geographic processes should be ≤ 1. Ideally, it should be equal to 1, however, there may be unmeasurable geographic processes.
4. Case studies
Vague geographic processes have broad application prospects in disaster demonstration, disaster prediction, and analysis of the protection effectiveness of ecological protection areas. Typhoon landfall can cause a considerable number of disasters, such as floods, power outages, and damage to houses. The boundary of a typhoon is vague, and the specific location and time of its landfall also have vagueness. Using the proposed method to express the typhoon landfall process can describe it more objectively, which can facilitate a government to formulate prevention plans and disaster relief measures. Desert governance consists of two sub processes: reducing desert area and increasing green area.
4.1. Landing process of typhoon ‘Lekima’
4.1.1. Case introduction
The coastal areas of southeast China are prone to typhoons. Typhoon landfalls are often accompanied by violent winds, heavy rains, and storm surges, which cause secondary disasters, such as urban flooding and waterlogging of low-lying farmlands, resulting in significant casualties and property losses. For example, typhoon ‘Moranti’ in 1996 nearly paralyzed the electric grid and caused severe damage to infrastructure in Xiamen and Fujian. A total of 3.755 million people were affected, with 44 people dead or missing, and direct economic losses amounting to 31.65 billion yuan. Meanwhile, Typhoon ‘Lekima’ in 2019 affected at least 5.358 million people, with 185,000 hectares of crops affected, 36,000 houses damaged, and direct economic losses exceeding 15.75 billion yuan in Zhejiang Province. As a typical vague geographic process, accurately describing and expressing the production (beginning), development, and extinction (end) phases of a typhoon landfall can help authorities formulate policies to cope with typhoon disasters, control risks, and reduce losses. Typhoon ‘Lekima’ is used here as an example to demonstrate the feasibility and effectiveness of the proposed method for expressing the phases of vague geographic processes.
Typhoon ‘Lekima’ originated at 18:00 on August 4, 2019, over the open ocean to the east of the Philippines before moving in a north-westerly direction and was categorized by the Central Weather Bureau as a super typhoon at approximately 21:00 on August 6. ‘Lekima’ landed at approximately 17:45 on August 9 in Chengnan Town, Wenling City, Zhejiang Province, China. The maximum wind force near the center at the time of landfall reached grade 16 (52 m/s), and the minimum pressure in the center was 930 hPa, making it the strongest typhoon to land in China in 2019. After moving through Zhejiang and Jiangsu provinces and reaching the Yellow Sea, the typhoon made landfall again on August 11 at 12:50 in Huangdao District, Qingdao City, Shandong Province, with a maximum wind force of 9 (23 m/s) near the center and minimum pressure in the center of 980 hPa. Next, ‘Lekima’ continued to move northward until it weakened to a tropical depression in Laizhou Bay, and the Central Weather Bureau stopped grading it at 06:00 on August 13 ().
4.1.2. Data sets
Sea level pressure
The second Modern-Era Retrospective Analysis for Research and Applications (MERRA-2) is the latest atmospheric reanalysis data released by the National Aeronautics and Space Administration (NASA), which replaces the original MERRA reanalysis data. MERRA-2 updates the Goddard Earth Observing System (GEOS) using the newer GEOS-5 system and a grid point statistical interpolation scheme (Rienecker et al. Citation2011).
MERRA-2 images (http://goldsmr4.gesdisc.eosdis.nasa.gov/data/MERR-A2), with a horizontal resolution of 0.5° × 0.625° and a temporal resolution of 1 h, with monthly updates, were used in this study. The time range of the data was August 7–13, 2019. Preprocessing was first performed in MATLAB.
(2) | Precipitation |
The Global Precipitation Measurement (GPM) program developed by NASA and the Japan Aerospace Exploration Agency, with a dual-frequency precipitation radar and advanced microwave imager, has coverage from 65° to 65°S and is the latest global satellite precipitation product. GPM offers two near real-time products, IMGRE_G and IMGRE_L, corresponding to 4-h and 14-h data release delays, respectively, available at https://disc.gsfc.nasa.gov/.
The precipitation data used in this section had a temporal resolution of 30 min and a spatial resolution of 0.1° × 0.1°, spanning August 7–13, 2019. Preprocessing was first performed in MATLAB.
4.1.3. Data processing
Fuzzy C-means (FCM) is an unsupervised fuzzy classification algorithm based on FS (Bezdek Citation1981) that divides objects with the highest similarity into the same cluster. FCM is typically used to construct a fuzzy representation of fuzzy geographical phenomena. The algorithm includes the following parameters: number of clustering categories, c, fuzzy parameter, m, and iteration stop threshold, ϵ. The fuzzy parameter, m, is the weight index, an empirical value obtained through countless experiments. When the number of clustering categories, c, is constant, the value of m, the membership degree of objects belonging to a certain class, also changes accordingly; hence, the classification results have uncertainty. IT2 FCM (Hwang and Rhee Citation2007) is proposed based on IT2 FS, which extends the fuzzy parameter to and
comprehensively considering uncertainty in data processing.
The results of the classification of precipitation and sea level pressure data using the IT2FCM (combined interval type-2 and FCM) algorithm (Hwang and Rhee Citation2007) were used to determine the vague stage of the rainfall and typhoon process. The clustering categories, c, for the rainfall degree was set to six, corresponding to the following levels of the precipitation degree: no rain, light rain, medium rain, heavy rain, rainstorm, and heavy rainstorm. The number of typhoon range classifications, c, was set to three, and the corresponding sea level pressures were set to the non-typhoon range, typhoon boundary, and typhoon center. Many researchers have studied the value of m in FCM (Bezdek Citation1981; Bezdek, Ehrlich, and Full Citation1984; Fisher Citation2010; Okeke and Karnieli Citation2006; Pal and Bezdek Citation1995). some of whom suggest that the minimum value of m is 1.1 (Bezdek Citation1981; Okeke and Karnieli Citation2006), while others report it as 1.5 (Bezdek, Ehrlich, and Full Citation1984; Pal and Bezdek Citation1995). However, most agree that the maximum value of m is 2.5 (Fisher Citation2010; Okeke and Karnieli Citation2006; Pal and Bezdek Citation1995). Therefore, this study applies the mean of 1.3 as the minimum value of m and 2.5 as the maximum value of m in IT2FCM. The iteration-stopping threshold was set at ϵ < 0.001.
4.1.4. Landing process of typhoon ‘Lekima’
Several characteristics of geographical entities and phenomena change over time during a geographic process. The typhoon landing process involves changes in the atmospheric pressure, precipitation level, and typhoon location in the landing area. Therefore, changes in the rainfall degree and typhoon location in the landing area were used to synthetically verify the accuracy of the vague geographic process expression method proposed in this study.
Changes in sea level pressure
This section analyzes the minimum sea level pressure changes around the landfall of typhoon ‘Lekima’ in the Zhejiang Province. It determines the landfall process of typhoon ‘Lekima’ from the vague classification results, as shown in . The UMF and LMF of typhoon ‘Lekima’ during its landfall at 09:00 on August 9 was > 0.5, indicating that before this time, typhoon ‘Lekima’ was in the pre-landfall phase. The typhoon entered the beginning landfall phase from 14:00 on August 9 to 10:00 on August 10; the UMF and LMF were > 0.9, indicating that the typhoon was in the definite landfall phase. From 11:00 to 16:00 on August 10, the UMF and LMF decreased to 0.5–0.8, and the typhoon was in the vague departure phase. The UMF at 17:00 on August 10 was 0.8422, the LMF value was 0.426 (< 0.5), while the typhoon was at the outermost boundary of the vague process.
Note that LMF considers all possible influencing factors (even if the degree of influence is only 0.1), while UMF only considers factors with significant influence (for example, only those with a degree of influence > 70); therefore, UMF is relatively simple whereas LMF is considerably more complex.
The typhoon landfall process is often accompanied by heavy rainfall. The duration of heavy rainfall in Zhejiang Province during the landfall of typhoon ‘Lekima’ could be obtained by vague classification of the maximum precipitation data every 2 h to verify the evaluation results of the typhoon process. As shown in , the duration of heavy to torrential rainfall in Zhejiang Province during the ‘Lekima’ landfall was primarily concentrated from 09:00 on August 9 to 17:00 on August 10, during which the maximum and minimum membership degree of the rainfall level was > 0.8. This is consistent with the duration of the typhoon landfall process from 09:00 August 9 to 17:00 August 10. Most studies apply crisp methods to express geographical processes with boundaries that cannot be accurately defined (e.g. geographical processes with inherent vagueness). The successful use of the proposed method to express the typhoon landfall process shows that it can more objectively express geographical processes lacking accurately defined boundaries.
Figure 7. Membership degree of heavy rain to a rainstorm in Zhejiang Province during the landing of typhoon ‘Lekima.’.

(2) | Change in position relationship |
Changes in the topological relationship between the typhoon and landfall area also accompany the typhoon landfall process. Therefore, we selected a total of five time points based on : August 7 at 01:00 (before landfall), August 9 at 09:00 (vague landfall time point), August 10 at 03:00 (middle phase of landfall), August 10 at 17:00 (vague end time point), and August 13 at 01:00 (after departure). The accuracy of the proposed geographic process time expression method was verified by comparing the typhoon’s position relationship at these time points ().
Figure 8. Positional changes of Typhoon ‘Lekima’ at each stage (a) 01:00 on August 7, (b) 09:00 on August 9, (c) 03:00 on August 10, (d) 11:00 on August 10, and (e) 01:00 on August 13.

The ‘egg yolk’ model (Cohn and Gotts Citation1996) is used to express the positions of typhoon landfall at various time points. The core and boundaries of typhoons were determined using the Fuzzy C-means algorithm (Bezdek Citation1981), as shown in , and the results were compared with those obtained using the proposed method. Based on the positional changes depicted in , the typhoon did not make landfall at 01:00 on August 7 (a); rather, the typhoon border made landfall in Zhejiang Province at 09:00 on August 9 (b), indicating that this was the beginning of the landfall process. Moreover, the typhoon center made partial landfall at 14:00 on August 9 (c), implying that this point was the beginning of the development phase of the landfall process. The typhoon center made complete landfall at 03:00 on August 10 (d), implying that this moment was the beginning of the development of the landfall process. Subsequently, the typhoon boundary left Zhejiang Province at 11:00 on August 10 (e), representing the initiation of the end of the landfall process. The core of the typhoon then left Zhejiang Province at 16:00 on August 10 (f), representing the endpoint of the landfall process. Finally, the typhoon entirely left Zhejiang Province at 01:00 on August 13 (g). The locations of the typhoons at each time point in (a)–(g) are consistent with the locations of the time points of the phases of the typhoon process obtained from the classification. Hence, the proposed expression method could more accurately express the time feature of the vague geographic process phases.
4.2. Desert greening process in Xinjiang
4.2.1. Case introduction
The Xinjiang Uygur Autonomous Region of China (Xinjiang) is located at 34°15′ to 49°10′N and 73°20′ to 96°25′E (), covering an area of 1.66 million km2 and presenting the conformation physiognomy of ‘three mountains in two basins.’ The total area of desertification land is 1.0712 million km2, and the desert area is 746,700 km2. The Tarim Basin and Taklimakan Desert—the largest inland basin and mobile desert in China—and the Gurbantungut and Kumtag deserts are located within Xinjiang. Owing to its inland location, the arid climate conditions caused by the closed inland basin have led to the development of a highly fragile ecosystem vulnerable to the impact of disastrous weather, including sandstorms and blowing sand, causing soil degradation and desertification, and limiting the social progress and economic development within the region.
To protect the local ecological environment, China has launched many greening projects. (a) and (b) show the normalized difference vegetation indices (NDVIs) in 2005 and 2015, respectively, indicating that the greening project has achieved certain results. However, remote sensing images cannot obtain the real vegetation coverage area. For example, some parts of the vegetation land may be covered by yellow sand due to sandstorms. Thus, the objective description and expression of a vague desert greening process are helpful to understand the greening status in real time and formulate appropriate policies. In this section, the desert greening process in Xinjiang is used as an example to determine the practicability of the proposed vague geographical process model.
4.2.2. Datasets
The Moderate Resolution Imaging Spectroradiometer China synthetic product MOODND1M is provided by the Geospatial Data Cloud Platform (http://www.gscloud.cn/), calculated from MODND1D using the maximum synthesis method and subjected to geometric and atmospheric corrections before use. The time resolution of the dataset was one month, and the spatial resolution was 500 m. The time range was from January 2001 to December 2015.
4.2.3. Data processing
The vegetation coverage and desert areas in Xinjiang from 2001 to 2015 were classified to represent the greening process. The clustering categories, c, were set to 2, indicating that the greening degree was divided into ‘early greening’ and ‘current greenery.’ The desert was divided into before and during control; fuzzy factors and
were set to 1.3 and 2.5, respectively (Fisher Citation2010), and the iteration stop threshold, ϵ, was < 0.001.
4.2.4. Desert greening process in Xinjiang
The process of desert greening involves changes in the desert area and vegetation coverage. Therefore, the changes in Xinjiang desert and vegetation coverage areas from 2001 to 2015 were analyzed (). As shown in (a) and (b), the desert area in Xinjiang fluctuated significantly from 2001 to 2015, showing an overall downward trend. In contrast, the vegetation area in Xinjiang fluctuated significantly from 2001 to 2015, showing an overall upward trend.
The trajectories of area change were vaguely classified (). (a) shows the membership degree track of the annual desert and vegetation areas of the desert greening process, composed of UMF and LMF. The trend lines of the desert and vegetation area to the track of membership degree in greening and governance are shown in (b). From 2001 to 2015, Xinjiang was at the beginning of the process of increasing vegetation coverage and the end of the desertification process. These two processes jointly illustrate the greening process in Xinjiang from 2001 to 2015.
Figure 11. Change in the annual desert and vegetation areas belonging to the desert greening process in Xinjiang from 2001 to 2015: (a) membership track; (b) trend lines of the membership track.

These results are consistent with the detection results of the change in desertification in China from 2001 to 2015 (Jixing et al. Citation2021). Most studies apply crisp methods to express geographical processes with boundaries that cannot be accurately defined (e.g. geographical processes with inherent vagueness). Meanwhile, the successful use of the proposed method to express the typhoon landfall process demonstrates that it can more objectively express these geographical processes.
5. Conclusions
This study proposes a new method based on IT2FS for describing and expressing the vagueness of geographic processes from the perspective of time features. It addresses the limitations of current methods with descriptions and representations of geographic processes based on precise expression algorithms that fail to characterize vague geographic processes or consider the time feature that portrays their production (beginning), development, and extinction (end) stages. Furthermore, using the landfall process of typhoon ‘Lekima’ as a case study, three vague quantitative geographic processes were considered, i.e. sea level pressure, precipitation, and positional changes during typhoon landfall, to comprehensively verify the practicability of the proposed theory that the vague geographical process can be composed of multiple vague subprocesses. Moreover, the desert greening process in Xinjiang was used as an example to verify the practicality of the proposed theory to qualitatively describe a vague geographical process.
The expression model of vague geographical process proposed in this paper enhances the capacity to measure membership error from the perspective of time characteristics and is more suitable for expressing gradual geographic spatiotemporal information (i.e. vague spatiotemporal information). It is a powerful tool for describing, expressing, querying, analyzing, and reasoning complex geographic processes with vagueness in GIS. It also provides novel insights for addressing vagueness in various fields of study.
The two case studies validated the applicability of our model on global geographic processes in the natural world, which are complex and affected by human and social factors. However, as a complex research object, geographic processes involve changes in the geographical entity location, geometry, semantics, attributes, relationships, and other characteristics. Future studies should provide a comprehensive perspective to jointly express the changes in various features in the fuzzy geographical process to improve the ability of GIS to describe, express, and analyze this type of process. The research results have implications for areas such as segregation, land use change, ecological protection zone assessment, and other areas with vagueness.
Author contributions
Yue Yin contributed to the concept of the current research, formulated the overarching research goals, conducted the experiment, and wrote the manuscript. Yufeng He translated the manuscript and completed the revisions. Yizhe Feng collected data, formatted the manuscript, and corrected any errors observed. Xueying Zhang provided funding and provided professional comments. Yehua Sheng provided funding, directed the entire process, and was responsible for the quality audit of the manuscript.
Disclosure statement
No potential conflict of interest was reported by the author(s).
Data availability statement
All data used in this study are accessible online from https://disc.gsfc.nasa.gov/. The code of this study is openly available on ‘figshare’ at https://figshare.com/s/4c64213b60d747d08d10.
Additional information
Funding
References
- Abbasi, Mehdi, Seyed Mohammad, and Aliakbar Jalali. 2020. “Fuzzy Tracking Control of Fuzzy Linear Dynamical Systems.” ISA Transactions 97: 102–115. https://doi.org/10.1016/j.isatra.2019.07.028.
- Bezdek, James C. 1981. Pattern Recognition with Fuzzy Objective Function Algorithms. New York: Plenum Press.
- Bezdek, James C., Robert Ehrlich, and Willian Full. 1984. “FCM: The Fuzzy C-Means Clustering Algorithm.” Computers & Geosciences 10 (2–3): 191–203. https://doi.org/10.1016/0098-3004(84)90020-7.
- Burrough, Peter A. 1989. “Fuzzy Mathematical Methods for Soil Survey and Land Evaluation.” Journal of Soil Science 40 (3): 477–492. https://doi.org/10.1111/j.1365-2389.1989.tb01290.x.
- Cohn, Anthony G., and N. M. Gotts. 1996. “The ‘Egg-Yolk’ Representation of Regions with Indeterminate Boundaries.” In Proceedings, GISDATA Specialist Meeting on Geographical Objects with Undetermined Boundaries, edited by Peter A. Burrough, and A. M. Frank, 171–187. London, UK: CRC Press. https://doi.org/10.1201/9781003062660.
- Cross, Valerie, and Aykut Firat. 2000. “Fuzzy Objects for Geographical Information Systems.” Fuzzy Sets and Systems 113 (1): 19–36. https://doi.org/10.1016/S0165-0114(99)00010-X.
- Devaraju, Anusuriya, Werner Kuhn, and Chris S. Renschler. 2015. “A Formal Model to Infer Geographic Events from Sensor Observations.” International Journal of Geographical Information Science 29 (1): 1–27. https://doi.org/10.1080/13658816.2014.933480.
- Fisher, Peter F. 2010. “Remote Sensing of Land Cover Classes as Type 2 Fuzzy Sets.” Remote Sensing of Environment 114 (2): 309–321. https://doi.org/10.1016/j.rse.2009.09.004.
- Goodchild, Michael F., May Yuan, and Thomas J. Cova. 2007. “Towards a General Theory of Geographic Representation in GIS.” International Journal of Geographical Information Science 21 (3): 239–260. https://doi.org/10.1080/13658810600965271.
- Grenon, Pierre, and Barry Smith. 2004. “SNAP and SPAN: Towards Dynamic Spatial Ontology.” Spatial Cognition & Computation 4 (1): 69–104. https://doi.org/10.1207/s15427633scc0401_5.
- Guo, Jifa, Xiaodong Shao, and Xunqiang Mo. 2018. “Qualitative Topological Relations between Interval Type-2 Fuzzy Spatial Objects.” Transactions in GIS 22 (6): 1596–1631. https://doi.org/10.1111/tgis.12489.
- He, Yufeng, Barbara Hofer, Yehua Sheng, Yue Yin, and Hui Lin. 2023. “Processes and Events in the Center: A Taxi Trajectory-Based Approach to Detecting Traffic Congestion and Analyzing Its Causes.” International Journal of Digital Earth 16 (1): 509–531. https://doi.org/10.1080/17538947.2023.2182374.
- Huck, Jonathan J., Duncan Whyatt, Gemma Davies, J. Dixon, Brendan Sturgeon, Bree Hocking, Colin Tredoux, Neil Jarman, and Dominic Bryan. 2023. “Fuzzy Bayesian Inference for Mapping Vague and Place-Based Regions: A Case Study of Sectarian Territory.” International Journal of Geographical Information Science 37 (8): 1765–1786. https://doi.org/10.1080/13658816.2023.2229894.
- Hwang, Cheul, and Frank Chung-Hoon Rhee. 2007. “Uncertain Fuzzy Clustering: Interval Type-2 Fuzzy Approach to $C$-Means.” IEEE Transactions on Fuzzy Systems 15 (1): 107–120. https://doi.org/10.1109/TFUZZ.2006.889763.
- Jixing, Sun, Zhong Cheng, He Hongwei, Nugeman Guli-Tohuti, and Li Hui. 2021. “Continuous Remote Sensing Monitoring and Changes of Land Desertification in China from 2000 to 2015.” Journal of Northeast Forestry University 48 (3): 87–92.
- Kavouras, Marinos. 2001. “Understanding and Modelling Spatial Change.” In Life and Motion of Socio-Economic Units, edited by Andrew Frank, Jonathan Raper, and Jean-Paul Cheylan, 49–62. London, UK: Taylor & Francis.
- Kjenstad, Kjell. 2006. “On the Integration of Object-Based Models and Field-Based Models in GIS.” International Journal of Geographical Information Science 20 (5): 491–509. https://doi.org/10.1080/13658810600607329.
- Li, Rui, Alexander Klippel, and Jinlong Yang. 2011. “Geographic Event Conceptualization: Where Spatial and Cognitive Sciences Meet.” In Proceedings of the Annual Meeting of the Cognitive Science Society 33: 3168–3173.
- Li, Xiaolong, Jiansi Yang, Xuefeng Guan, and Huayi Wu. 2014. “An Event-Driven Spatiotemporal Data Model (E-ST) Supporting Dynamic Expression and Simulation of Geographic Processes.” Transactions in GIS 18 (S1): 76–96. https://doi.org/10.1111/tgis.12127.
- Liang, Qilian, and Jerry M. Mendel. 2000. “Interval Type-2 Fuzzy Logic Systems: Theory and Design.” IEEE Transactions on Fuzzy Systems 8 (5): 535–550. https://doi.org/10.1109/91.873577.
- McIntosh, John, and May Yuan. 2005. “Assessing Similarity of Geographic Processes and Events.” Transactions in GIS 9 (2): 223–245. https://doi.org/10.1111/j.1467-9671.2005.00214.x.
- Okeke, Francis, and Arnon Karnieli. 2006. “Linear Mixture Model Approach for Selecting Fuzzy Exponent Value in Fuzzy c-Means Algorithm.” Ecological Informatics 1 (1): 117–124. https://doi.org/10.1016/j.ecoinf.2005.10.006.
- Pal, Nikhil Ranjan, and James C. Bezdek. 1995. “On Cluster Validity for the Fuzzy c-Means Model.” IEEE Transactions on Fuzzy Systems 17 (5): 873–877. https://doi.org/10.1109/91.413225.
- Pang, Matthew Yick Cheung, and Wenzhong Shi. 2002. “Development of a Process-Based Model for Dynamic Interaction in Spatiotemporal GIS.” Geoinformatica 6 (4): 323–344. https://doi.org/10.1023/A:1020876609236.
- Petnga, Leonard, and Mark Austin. 2013. “Ontologies of Time and Time-Based Reasoning for MBSE of Cyber-Physical Systems.” Procedia Computer Science 16: 403–412. https://doi.org/10.1016/j.procs.2013.01.042.
- Raper, Jonathan, and David Livingstone. 1995. “Development of a Geomorphological Spatial Model Using Object-Oriented Design.” International Journal of Geographical Information Systems 9 (4): 359–383. https://doi.org/10.1080/02693799508902044.
- Rienecker, Michele M., Max J. Suarez, Ronald Gelaro, Ricardo Todling, Julio Bacmeister, Emily Liu, Michael G. Bosilovich, et al. 2011. “MERRA: NASA’s Modern-Era Retrospective Analysis for Research and Applications.” Journal of Climate 24 (14): 3624–3648. https://doi.org/10.1175/JCLI-D-11-00015.1.
- Romero, Gustavo E. 2013. “Adversus Singularitates: The Ontology of Space-Time Singularities.” Foundations of Science 18 (2): 297–306. https://doi.org/10.1007/s10699-012-9309-4.
- Sola, Humberto Bustince, Javier Fernandez, Hani Hagras, Francisco Herrera, Miguel Pagola, and Edurne Barrenechea. 2015. “Interval Type-2 Fuzzy Sets Are Generalization of Interval-Valued Fuzzy Sets: Toward a Wider View on Their Relationship.” IEEE Transactions on Fuzzy Systems 23 (5): 1876–1882. https://doi.org/10.1109/TFUZZ.2014.2362149.
- Sowa, John F. 1996. “Processes and Participants.” In Conceptual Structures: Knowledge Representations as Interlingua: 4th International Conference on Conceptual Structures, Sydney, Australia, August 19–22, 1996. Lecture Notes in Computer Science, 1115: 1–22. https://doi.org/10.1007/3-540-61534-2.
- Tang, Tao, and Jiazhen Zhang. 2018. “Time-scale Sensitive Sensor Applications in Collecting and Analyzing Geographic Event Data.” Annals of GIS 24 (4): 241–253. https://doi.org/10.1080/19475683.2018.1520299.
- Urbanski, Jacek Andrzej. 1999. “The Use of Fuzzy Sets in the Evaluation of the Environment of Coastal Waters.” International Journal of Geographical Information Science 13 (7): 723–730. https://doi.org/10.1080/136588199241085.
- Worboys, Michael F. 2001. “Modelling Changes and Events in Dynamic Spatial Systems with Reference to Socio-Economic Units.” In Life and Motion of Socio-Economic Units, ed. Frank, Raper and Cheylan. London: CRC Press.
- Worboys, Michael, and Kathleen Hornsby. 2004. “From Objects to Events: Gem, the Geospatial Event Model.” In Proceedings of the 3rd International Conference on Geographic Information Science, 327–343. Phelps Hall: NCGIA. https://doi.org/10.1007/978-3-540-30231-5_22.
- Xie, Jong, and Cunjin Xue. 2009. “A Top-Down Hierarchical Spatio-temporal Process Description Method and Its Data Organization.” In SPIE Proceedings International Symposium on Spatial Analysis, Spatial-Temporal Data Modeling, and Data Mining 74922. https://doi.org/10.1117/12.838353.
- Xue, Cunjin, Chengbin Wu, Jingyi Liu, and Fenzhen Su. 2019. “A Novel Process-Oriented Graph Storage for Dynamic Geographic Phenomena.” ISPRS International Journal of Geo-Information 8 (2): 100. https://doi.org/10.3390/ijgi8020100.
- Yin, Yue, Yehua Sheng, Yufeng He, and Jiarui Qin. 2022. “Modeling Vague Spatiotemporal Objects Based on Interval Type-2 Fuzzy Sets.” International Journal of Geographical Information Science 36 (6): 1258–1273. https://doi.org/10.1080/13658816.2022.2053538.
- Yu, Manzhu, Chaowei Yang, and Baoxuan Jin. 2018. “A Framework for Natural Phenomena Movement Tracking – Using 4D Dust Simulation as an Example.” Computers & Geosciences 121: 53–66. https://doi.org/10.1016/j.cageo.2018.10.003.
- Zadeh, Lotfi A. 1965. “Fuzzy Sets.” Information & Control 8 (3): 338–353. https://doi.org/10.1016/S0019-9958(65)90241-X.
- Zadeh, Lotfi A. 1975. “The Concept of a Linguistic Variable and Its Application to Approximate Reasoning—I.” Information Sciences 8 (3): 199–249. https://doi.org/10.1016/0020-0255(75)90036-5.