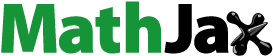
ABSTRACT
The constant need for decarbonization has led to the replacement of artificial light at night (ALAN) with light-emitting diodes (LEDs), inducing blue light pollution and its consequent adverse effects. As a result, there is an urgent need for the development of a technique for the rapid, accurate, and large-scale discrimination of the various illumination sources. The newly launched Sustainable Development Science Satellite-1 (SDGSAT-1) can play this role by supplementing the existing nighttime light data with multispectral and high-resolution features. Along these lines, in this work, a novel approach to identify various types of illumination sources using machine learning in SDGSAT-1 images was proposed, taking Beijing as a worked example. The results indicate that: (1) The method can effectively distinguish the various types of light sources with an overall accuracy of 0.92 for ALAN and 0.95 for streetlights. (2) The illumination patterns can be clearly depicted, indicating distinct spatial heterogeneity in ALAN along Beijing’s 5th Ring Road. (3) Statistically significant disparities between road classes and streetlight types were detected, with a notable increase in LED streetlight usage as the road class diminishes. This work emphasizes the crucial role of SDGSAT-1 in analysing ALAN, providing valuable insights in urban lighting management.
1. Introduction
Illumination facilities are closely related to various facets of modern life (Han et al. Citation2014). As human habitation, transportation, infrastructure, and economic activities are currently experiencing rapid expansion, artificial light at night (ALAN) coverage from streetlights and multiple other sources is becoming more extensive and denser, rendering nights progressively brighter (Falchi et al. Citation2019; Kyba et al. Citation2017). Statistics from the International Energy Agency (IEA) reveal that lighting accounts for 19% of the global electricity consumption, producing a carbon dioxide (CO2) load equivalent to 70% of the global automotive exhaust emissions (IEA Citation2006). In line with cities’ transitions toward green and low-carbon paradigms, illumination systems are gradually shifting from high-energy-consuming sources, such as high-pressure sodium lamps and metal halides, towards more energy-efficient options such as light emitting diodes (LEDs); a phenomenon known as the lighting revolution. However, this shift has led to an increase in blue light emissions (Elvidge et al. Citation2010; Gaston and de Miguel Citation2022; Schulte-Römer et al. Citation2019). Numerous works in the literature have emphasized that excessive illumination, particularly that rich in blue wavelengths, has an adverse impact on both human health (Gaston et al. Citation2014; Hatori et al. Citation2017; Kobav and Bizjak Citation2012) and ecosystems (Davies et al. Citation2013; Grubisic et al. Citation2018; Longcore et al. Citation2018). Moreover, the phenomenon of light pollution attributed to ALAN must be also considered (Falchi and Bara Citation2020; Gaston et al. Citation2012; Kyba Citation2018). The latter effect is associated with the spectral composition of lighting sources in the environment (Gaston et al. Citation2014; Schroer and Hölker Citation2016). From the perspective of the lighting revolution, the differentiation of the various types of illumination sources not only aids in further understanding the adverse effects and patterns of change induced by ALAN, but also can provide a scientific foundation for formulating more precise urban lighting management policies to mitigate light pollution.
Acquiring the spectral profile of ALAN at a large scale and distinguishing between different types of illumination sources is a challenging task. This is because the primary sources of night-time light (NTL) image data – the Defence Meteorological Program Operational Line-Scan System (DMSP-OLS), Suomi National Polar-orbiting Partnership Visible Infrared Imaging Radiometer Suite (NPP-VIIRS), and Luojia 1-01 satellite (LJ1-01) of Wuhan University in China – only work with a single panchromatic spectral band (Levin et al. Citation2020; Zhao et al. Citation2019). However, the collection of multispectral data is crucial for discriminating between the various types of illumination sources (Elvidge et al. Citation2010). The majority of the previously reported works in the literature has typically relied upon fixed, vehicular, or airborne equipment to gather data. For instance, Dobler et al. (Citation2016) and Puschnig, Posch, and Uttenthaler (Citation2014) employed stationary spectrometers for high-spectral observations of the Manhattan area and Vienna, respectively, achieving an effective distinguishing between the various light sources. In the vehicular context, Yin, Oliveira, and Murthy (Citation2017) utilized an onboard spectrometer to identify the types of streetlights along four roads in Rosendale, New York. Furthermore, in the works of Kuechly et al. (Citation2012), Hale et al. (Citation2013), Tardà et al. (Citation2011), and Kruse and Elvidge (Citation2011), airborne hyperspectral sensors were employed to conduct classification studies on the light emissions in city centres of Berlin, Birmingham, Tarragona, and Las Vegas, respectively. However, these approaches were limited to specific locations and were significantly costly.
In recent years, the use of urban night-time images captured by astronauts aboard the International Space Station (ISS) using digital single-lens reflex (DSLR) cameras has garnered significant research attention. These images have been employed in ALAN studies in various locations such as Milan, Italy (Sánchez de Miguel et al. Citation2019) and Haifa, Israel (Rybnikova et al. Citation2021), demonstrating that sensors operating in three visible spectral bands can facilitate the identification of the type of illumination source. In addition, emerging commercial satellites, such as JL1-3B, are promising for investigating ALAN variations owing to their ability to acquire multi-spectral nighttime images (Cheng et al. Citation2020). However, there are concerns regarding the retirement of the ISS, affecting the future availability of data. Furthermore, the spatial coverage of ISS images is constrained, and the lack of radiometric calibration hampers both the stability and replicability of its imaging outputs (Guo, Hu, and Zheng Citation2023). In addition, the JL1-3B images, which are not publicly shared, are better suited for commercial applications rather than large-scale scientific inquiries.
The Glimmer Imager (GI), a payload of the Sustainable Development Science Satellite 1 (SDGSAT-1), possesses the capability to capture NTL images with a multispectral resolution of 40-m, panchromatic resolution of 10-m, and a width of 300 km (Guo et al. Citation2023). In September 2022, the data from SDGSAT-1 were made globally accessible, establishing GI images as the most exceptional publicly available source of NTL data These images are characterized by the highest resolution and quality, which renders them a pivotal complement to the existing NTL data. Their multispectral and high spatial resolution traits can also provide robust data support for in-depth investigations into urban community and street-scale issues. Consequently, the primary aim of this study work was to harness the multi-spectral bands of GI images, which can help develop a cost-effective, efficient, and large-scale approach for identifying various types of illumination sources. This will not only further our understanding of urban illumination patterns and dynamics, providing a scientific foundation for urban planning and development, but also has significant implications for environmental conservation and energy management. Ultimately, valuable insights for the sustainable advancement of cities are delivered.
2. Study area and datasets
2.1. Study area
Beijing, the capital of China, is situated between the latitudes of 39°28′N to 41°05′N and longitudes of 115°25′E to 117°30′E, spanning an area of approximately 16,410 square kilometres, with a permanent population of 21.89 million people. Over the past half-century, the urban area of Beijing has rapidly expanded in the shape of an outwardly extending single central layer structure [(a)]. Alongside the rapid development of the city, its urban illumination system has experienced multiple transformations. In 1997, a comprehensive lighting overhaul was conducted, effectively increasing the nocturnal luminance of the city. In recent years, in response to calls for energy conservation and emissions reductions, several illumination facilities have had energy-efficient renovations, addressing the escalating energy requirements caused by urbanization.
Figure 1. Map of the study area: (a) GI images from SDGSAT-1 satellite, (b) NPP-VIIRS images, (c) Landsat8 OLI images, and (d) Beijing administrative district.

The study area of this work encompasses the territory within the 6th Ring Road of Beijing (referred to as the 6th Ring Zone), spanning a total area of 2267 square kilometres, which accounts for approximately 13.8% of the total area of Beijing [(d)]. The 6th Ring Zone accommodates nearly 80% of Beijing’s permanent residents and is the metropolitan region of the city. Significant human activity occurs at night, and different types of lamps are widely used, leading to colourful lighting effects.
2.2. Datasets
2.2.1. GI images of SDGSAT-1
The GI images from SDGSAT-1 have unrivalled advantages over night-time light images such as DMSP-OLS, NPP-VIIRS, and LJ1-01: (1) Multi-spectral bands covering the visible range (); (2) higher radiometric resolution (≥12-bit), which helps to significantly reduce saturation effects; (3) earlier overpass time (Guo, Hu, and Zheng Citation2023; Zhang et al. Citation2022). In this work, a cloud-free, stripe-free, high-quality image captured on 26 November 2021 was acquired through the International Research Centre of Big Data for Sustainable Development Goals (CBAS, https://www.sdgsat.ac.cn/). CBAS processed the image and image fused the 40-m multispectral and 10-m panchromatic images to generate a 10-m high-resolution multi spectral GI image.
2.2.2. Openstreetmap data
OpenStreetMap (OSM) is a global geographic dataset collaboratively created by the online community, characterized by its open-source and editable features (Boeing Citation2019). This dataset encompasses sub-datasets such as points of interest (POI), building contours, and road networks, with this work specifically utilizing the road dataset. Considering the GI image resolution, five classes of roads – Motorway, Trunk, Primary, Secondary, and Tertiary – were selected for subsequent analysis. Given OSM’s reliance on contributions from amateur geographers, inherent inaccuracies exist (Wang et al. Citation2013; Xiaona and Nianxue Citation2019). To address this issue, Google Earth was combined for validation and refinement, ensuring increased data accuracy and dependability.
2.2.3. Lighting source samples
High-pressure sodium (HPS), light-emitting diode (LED) and fluorescent (FL) are the main sources of illumination within the study area. Concerning the work of Elvidge et al. (Citation2010), the spectral curves of each light source () were analysed to classify the light source types. As can be observed, for the HPS light source, the strongest emission line comes from the sodium emission line at 819 nm, and there are dense clusters of strong emission lines at 569–616 nm. For the LED light source, the strongest emission occurs between 450 and 460 nm and there is a strong secondary emission in the range of 500–700 nm, but almost no emission larger than 800 nm. For the FL light sources, three primary emission lines exist, at 437, 544, and 611 nm, and dense clusters of weak emission lines exist between 578 and 636 nm.
A list of lighting facilities close to the time of the image from the Beijing Municipal Commission of Urban Management was collected. Due to issues such as data accessibility and confidentiality, 1743 samples [(a)] were acquired and pinpointed using Google earth for subsequent training and validation. The number of samples of different types is presented in .
Table 1. Sample statistics for each type of light source.
3. Method
ALAN encompasses a multitude of urban foundational illumination facilities, including streetlights, architectural façade illumination, public area lighting, etc. It acts as a principal illumination source within GI images. Given the significance of streetlights as a vital component within urban lighting systems and their substantial share in power expenditure, the buffer threshold proposed by Wang et al. (Citation2020) was adopted here to extract GI images within road buffers and achieve a more refined investigation into streetlight classification.
3.1. Data preprocessing
In this work, the raw digital numbers (DN) values were converted to radiance for each band using metadata files. For subsequent analysis, the grey-scale radiance of the GI images was computed using the method of Grundland and Dodgson (Citation2007) (Equation 1).
(1)
(1) where
denotes the greyscale brightness and
, and
are the corresponding radiant brightness values of the R, G, and B bands, respectively. Due to the narrower spectral range of the panchromatic band (450–900 nm) compared to the blue band (430–520 nm), the panchromatic band of the GI was not utilized in this work to mitigate blue light information loss(Guo, Hu, and Zheng Citation2023).
In the GI images, there are background pixels with low brightness values. To simplify the analysis and improve the stability of the algorithm, the thresholding method was used to construct the extraction mask (Equation 2). The following method was designed to determine the threshold (T): using the reclassification tool of ArcGIS software, the greyscale brightness of the image was classified into K different classes using the Jenks natural breakpoint method. This clustering algorithm can maximize the difference between the classes and minimize the difference within the class. The value of K was adjusted from small to large until the threshold for the division of the lowest class was stabilized, which is the threshold value T.
(2)
(2)
3.2. Feature extraction
The following three types of feature variables were extracted based on GI images and OSM data.
Spectral features are widely employed for the distinction of ground target types in remote-sensing images (Decker et al. Citation1992; Javed et al. Citation2021). Inspired by the colour–colour technique (Dixon Citation1965; Öhman Citation1949), which is widely used in astrophysics for identifying target light sources, proposing three spectral indices were proposed (): Simple index B/G (SIBG, Equation 3), Simple index G/R (SIGR, Equation 4), and Simple index B/R (SIBR, Equation 5).
The texture features serve as complementary attributes for remote-sensing image recognition and classification, capable of differentiating unique information that spectral features may struggle to separate (Hall-Beyer Citation2017; Iqbal et al. Citation2021). The grey-level co-occurrence matrix (GLCM), a common method for extracting texture features, describes texture through the computation of the spatial relationships among pixels (Haralick, Shanmugam, and Dinstein Citation1973). Python was employed to compute six texture features (), namely Homogeneity (Equation 6), Contrast (Equation 7), Dissimilarity (Equation 8), Entropy (Equation 9), Angular Second Moment (Equation 10), and Correlation (Equation 11), using a 5 × 5 sliding window on the R, G, and B bands of the GI image.
Table 2. Formula for calculating feature variables.
The road class variable is a discrete feature variable that can be obtained from the fclass field in the OSM data, and this feature variable is only available for GI images within the road buffer.
3.3. Feature reduction and normalization
Principal component analysis (PCA) for the dimensionality reduction of the feature variables was employed, mitigating feature redundancy and enhancing the operational efficiency of the model. PCA through matrix transformation (Equation 12), transmutes a set of original variables into new variables referred to as the principal components (PCs), maintaining the same dimensionality (Moghtaderi, Moore, and Mohammadzadeh Citation2007). Via PCA, several initially intercorrelated variables are compressed into a handful of mutually uncorrelated novel variables.
(12)
(12) where E is the transformation matrix and B stands for the multiband image combined with different texture features. PC1 holds the highest amount of principal component information, followed by PC2, and so on.
PCA requires the selection of interdependent and highly correlated variables for optimal dimensionality reduction. This work was evaluated using KMO (Kaiser Meyer Olkin) test (Kaiser Citation1970; Kaiser and John Citation1974). The KMO test (Equation 13) tests the suitability of data for principal component analysis by comparing the relative magnitude of the simple and partial correlation coefficients between the original variables.
(13)
(13) where
is the simple correlation coefficient,
states the partial correlation coefficient, and KMO ranges from 0 to 1. When KMO > 0.7, it indicates that the data are more suitable for PCA.
Since different types of feature variables have different magnitudes, after PCA, feature variables were normalized using Min–Max Scaling for subsequent model training.
3.4. Model selection
To precisely identify the various types of illumination sources, machine learning models were systematically compared to select the most optimal one.
Logistic Regression: This constitutes a generalized linear regression model to address multi-classification problems. It transforms the linear regression outputs into probabilities for each category by utilizing the SoftMax function. The class associated with the highest probability is then chosen as the prediction outcome (Wright Citation1995).
Neural Network: Drawing inspiration from the biological neural system, this model comprises an arrangement of input layers, multiple hidden layers, and an output layer (Shanmuganathan Citation2016). Neural networks are of various types, and the one employed in this work was the multi-layer perceptron (MLP).
Random Forest: Falling under the bagging category of classifier models, the random forest algorithm aggregates several independent and uncorrelated decision trees. The output results are collectively determined by the voting outcomes of the multitude of trees (Breiman Citation2001).
XGBoost: Belonging to the boosting category, this classifier model is a variant of the gradient boosting tree algorithm. It constructs a powerful ensemble classifier by combining weak classifiers based on classification and regression trees (CART), effectively implementing classification (Chen, Guestrin, and Assoc Comp Citation2016).
All machine learning models were implemented within the Python 3.10 environment. In terms of parameter configuration, the number of decision trees for both random forest and XGBoost was set to 100, while the remaining model parameters adopted default values. Preliminary testing indicated that increasing the number of decision trees leads to saturation in the predictive accuracy, whereas adjustments to other parameters have a relatively minor impact on the model’s precision.
Since only GI images within the road buffer have road class features, spectral and texture variables were used to train models when identifying ALAN types, and spectral, texture, and road class variables when identifying streetlight types.
3.5. Evaluation of model’s performance
A stratified ten-fold cross-validation approach was adopted in this work. In contrast to alternative validation methods, this technique offers a more robust consideration of imbalanced sample classes, while also maximizing the utilization of sample data, thereby yielding more dependable evaluative outcomes. This method involves the random partitioning of the original dataset into ten subsets, ensuring that the proportions of different class samples within each subset mirror those of the original dataset. During each iteration, nine subsets were employed for model training, with one subset reserved for validation, until all ten subsets have been utilized as validation sets. In addition, since a small number of the light source samples were from buildings and landscapes (), these samples did not contain the feature variable of road class. For this reason, for the prediction of the streetlight types and performing the accuracy test, only samples from streetlights were used.
The four metrics for evaluating the machine learning model were calculated based on the confusion matrix (). Precision (Equation 14) is the ratio of the number of correctly classified positive samples to the number of the positive samples predicted, and is used to evaluate the commission error of the model. Recall (Equation 15) is the ratio of the number of correctly classified positive samples to the number of actual positive samples, and is used to evaluate the omission error of the model. F1-score (Equation 16) combines the precision and recall of the model. Overall accuracy (OA, Equation 17) is the percentage of correct results in the total sample.
(14)
(14)
(15)
(15)
(16)
(16)
(17)
(17)
4. Results
4.1. Model training and validation
In this work, twenty-two feature variables were extracted from the GI imagery, including three spectral features, eighteen texture features, and one road class. The road class variable, which was only present in the GI images of the road buffer, was a discrete-type variable consisting of five road classes: trunk, motorway, primary, secondary, and tertiary. Pearson correlation analyses were conducted on 21 continuous variables to initially explore the relationships between the variables (). The correlation analyses showed that there was significant dependence and redundancy between 18 texture variables. The KMO test was performed on 18 texture variables, and KMO = 0.77 > 0.7, indicating that the texture variables meet the dimensionality reduction requirements of PCA. After PCA, the variables were normalized so that their magnitudes were restricted to the range 0–1.
Figure 5. Correlation matrix of the 21 characteristic variables. Blue (red) pies indicate a positive (negative) relationship between the analysed variables.

The texture variables were dimensionality reduced and the optimal number of principal components was determined using the method of Kaiser (Citation1970). As shown in , three principal components account for 87.23% of the total explained variance. More specifically, PC1, PC2, and PC3 account for 59.13%, 19.66%, and 8.18% of the total variance, respectively. Additionally, the dissimilarity in the R, G, and B bands (R3, G3, B3) and angular second moment in the R band (R5) had the highest contributions to the principal components.
Figure 6. Principal component analysis: (a) Variance explained and eigenvalues; (b) Contribution of each component, where R, G, and B refer to the three bands of GI images, and 1–6 refer to GLCM texture variables, in the order shown presented in .

The performance of four machine learning models was evaluated using different combinations of feature variables. As can be seen in , in terms of overall accuracy, the addition of texture variables and road class variables improved the overall accuracy of all three models except Logistic Regression. Comparing the overall precision performance of the four models, the XGBoost and Random Forest models exhibited the highest overall precision, with an OA of 0.92 using the spectral and texture variables, and an OA of 0.95 with the addition of the road class variable. Since the overall precision of the XGBoost and Random Forest models was similar, this work delved deeper into the performance of these two models for different light sources. In terms of the F1-score that take into account precision and recall, the F1-score values of all three light sources were significantly improved by adding texture variables and road grade variables. Among them, HPS and LED light sources showed similar improvement, and FL light sources showed the greatest improvement. Among the three light sources, under different combinations of feature variables, the F1-score values of HPS and LED light sources were similar and larger than those of FL light source, indicating that FL has more commission and omissions than the other two type of light sources. Comprehensively analysing the performance of different models, it can be argued that the random forest model can obtain the best performance.
To deeply explore the performance of the Random Forest model in different regions, statistical analyses of the circled regions divided according to the ring roads in Beijing were conducted (). Due to the different area sizes of the different regions, there are some differences in the number of samples in the regions, which may bias the performance assessment. From the analyses of ‘In 2nd’ and ‘From 5th to 6th’ regions, which have the largest number of samples, it is clear that the inclusion of texture variables and road class variables improves the overall accuracy of the different loop regions in terms of overall accuracy. For the analysis of the different light sources, similar to OA, the F1-score in the ‘In 2nd’ region was significantly lower than that in the ‘From 5th to 6th’ region, which is related to the complex lighting situation and the intertwined coexistence of multiple light sources in the city centre. This leads to more misclassification and misestimation.
4.2. Illumination source identification results
Given the excellent performance of the random forest model over other models, the said model for pixel-by-pixel identification and classification was employed. To mitigate the ‘salt and pepper’ effect, the outcomes experienced post-processing through a majority filter (Lillesand, Kiefer, and Chipman Citation2015; Munyati Citation2004).
(a) depicts the results of training the model using spectral and texture variables and identifying the ALAN type. As can be seen, it is evident that HPS light sources have high coverage, accounting for approximately 58.15% of the total area, followed by LED light sources, at around 39.70%; FL light sources have limited application, constituting a mere 2.15%. (b) shows the results of streetlight identification using spectral, texture and road class variables. The figure shows that HPS streetlights dominate in Beijing, spanning a length of 5911.67 km, roughly 59.82% of the total. LED streetlights are the second most prevalent, covering 2309.43 km, approximately 23.37% of the total, while FL streetlights occupy a mere 0.27%. Furthermore, it has been observed that there are still 1635.11 km of roads lacking streetlights in Beijing, constituting about 16.54% of the total. This observation can be explained from two perspectives: first, by correlating it with the analysis in (b), it can be observed that unlit roads primarily exist on the city’s outskirts, encompassing streets with lower traffic volumes and motorways, where the absence of streetlights is plausible. Second, this could also arise due to some roads having luminance that fall below the predetermined threshold, resulting in error.
The identification and classification outcomes of illumination sources aptly depict the urban lighting pattern. Considering the circle distribution structure of Beijing, statistical analyses were conducted according to the circle areas divided by the ring roads. As can be seen in , the 5th Ring Road serves as a significant boundary for Beijing’s lighting pattern. In terms of ALAN types, within the 5th Ring Road area, HPS light sources account for around 70%, while beyond it, the use of LED light sources notably increased, with a nearly balanced proportion of HPS and LED. A similar situation is also reflected in streetlights: inside the 5th Ring Road, HPS streetlights predominate, constituting over 80% of the total; outside the 5th Ring Road, the usage of LED streetlights substantially rises to 32.52%, while HPS sources decrease to 41.83%. Additionally, outside the Fifth Ring, 25.53% of roads lack streetlights, a notably higher percentage compared to those within it. The differences in the use of HPS and LED sources inside and outside the Fifth Ring Road reflect Beijing’s urban development. The inner area primarily comprises the older districts of Beijing, where lighting infrastructure was established earlier, which is an explanation for the prevalent use of HPS. In contrast, the outer area, beyond the Fifth Ring Road, constitutes the emerging districts of Beijing, where energy-efficient and environmentally friendly LED sources are favoured and widely implemented within the context of green, low-carbon urban development.
5. Discussion
5.1. Importance analysis of the feature variables
The training of the model involved several feature variables. To understand the impact of each feature variable on the outcomes, a permutation importance analysis was carried out (Gregorutti, Michel, and Saint-Pierre Citation2017). As can be seen in , spectral variables emerged as the most crucial features. Among these, the significance of the SIGR variable took precedence, aligning with the findings of Guo, Hu, and Zheng (Citation2023). Additionally, texture variables played a pivotal role, with the PC1 variable ranking second and third in identifying ALAN and streetlight types, respectively. It is important to note that the road class variable, which is not derived from the GI image but from the OSM dataset’s feature variables, is the second most important for streetlight categorization. This implies an association between streetlight types and road classes.
To further understand the relationship between the streetlight types and road classes, Geodetector was employed to analyse their spatial differentiation (Wang and Xu Citation2017; Wang, Zhang, and Fu Citation2016). The results emphasize a statistically significant distinction (P < 0.01) between streetlight types and road classes, with road classes accounting for approximately 25.19% of the variation in streetlight types within the sample. The streetlight types across different road classes were further analysed (), revealing that as road classes descend (from trunk to tertiary), the proportion of LED streetlights gradually rises, increasing from 8.81% to 34.18%. This phenomenon elucidates the elevated importance of the road class variable. Multiple factors could explain this phenomenon; on one hand, LED lighting tends to create concentrated illumination, leading to alternating brightness on road surfaces (Brons, Bullough, and Frering Citation2021). On the other hand, constraints such as heat dissipation and semiconductor materials limit the power of LED light sources (Hu et al. Citation2023; Ma and Li Citation2015), making them less suitable for higher-class roads, such as trunks with greater widths and higher speeds.
5.2. Evaluation of classification results
and demonstrate the categorical performance of the different light sources in various combinations of variables and in different regions. It can be seen that in almost all cases, the F1 scores of the FL light source are significantly lower than those of HPS and LED light source. In the ‘In 2nd’ region, both FL recall and LED precision are significantly lower than that of the HPS light source, which means that the risk of omission error for FL and the risk of commission error for LED are increased. The reason for this may be that FL and LED have similar spectral profiles (). In addition, the introduction of texture features brings some improvement to the classification results. In particular, the F1-score improvement is about 0.1 for HPS and LED, and about 0.3 for FL. This is because the texture variables are calculated based on windows, and therefore more global feature information can be obtained than the spectral variables calculated based on pixels. Furthermore, since the texture variables were calculated through the R, G, and B bands, the texture variables also contain the original spectral information.
5.3. Increased application potential of the GI images
GI images possess the distinct characteristics of being multispectral and high-resolution, with comparative advantages that are unmatched by other night-time light images. Consequently, GI images hold significant potential for a multitude of applications. Primarily, leveraging the temporal dimension of GI images facilitates an understanding of the patterns in the installation and replacement of illumination sources. For instance, as observed in the biennial GI images (), the South 5th Ring Road, previously devoid of streetlights, shows the presence of LED streetlights. Furthermore, the previously existing HPS streetlights along Liyuan Road and Qingyuan Road have been substituted with their LED counterparts. Second, studies indicate that, at similar luminances, LEDs exhibit lower power consumption than HPS lamps (Brons, Bullough, and Frering Citation2021; Djuretic and Kostic Citation2018). Hence, employing GI images for monitoring the progress of lighting facility transitions can help compute the potential benefits in terms of energy consumption reduction and carbon emission mitigation within urban settings. Finally, this work emphasized the correlation between streetlight types and road classes, while prior research also suggests an association between illumination source types and land use patterns (Guo, Hu, and Zheng Citation2023; Zheng et al. Citation2018). In the future, by integrating light source classification outcomes with human activities and socio-economic data, an in-depth understanding of urban spatial structures and socio-economic processes can be conducted, facilitating the analysis of interrelationships among urbanization, economic growth, poverty, population density, population migration, electricity penetration, and other factors, providing valuable insights and guidance for urban governance and policy formulation.
5.4. Limitations
In this work, satisfactory results in distinguishing between ALAN types and streetlight types in Beijing, China were achieved. However, constrained by data sources and the methodology, this work has the following limitations:
The GI sensor solely captures the visible spectrum, which to a certain extent restricts its ability to differentiate between the various types of illumination sources and the precision of its outcomes. Therefore, in areas where LED or HPS light sources are not predominant, such as Tokyo, Japan or Berlin, Germany (Elvidge et al. Citation2010; Levin et al. Citation2020), this approach may not be applicable. The concepts of NASA’s Nightsat mission (Elvidge et al. Citation2007) and research by Elvidge et al. (Citation2010) indicate that sensors that use both visible and near-infrared spectra can distinguish various illumination source types, such as metal halide, mercury vapour, light-emitting diode, high-pressure sodium, and more.
This work did not fully consider scenarios involving mixed lighting. However, instances where different types of lighting methods are combined at the same location do exist. For instance, for the streetlights on Chang'an Street, the upper layer is FL lamps, and the lower layer is HPS lamps [(a)]. Similarly, for the streetlights on Diaosuyuan South Street, the lane is illuminated with HPS lamps, while the pedestrian walkway employs LED lamps [(b)]. Fortunately, such occurrences are infrequent. However, certain buildings adjacent to roads might feature decorative LED light sources on their facades, which could be mistaken for the road’s HPS streetlights, resulting in mixed spectra within image pixels and influencing the outcomes to a certain extent.
The GI images themselves exhibit some quality issues, such as stripes, misalignment, and stray light (Yu et al. Citation2023; Zhang et al. Citation2022). Although the stripe issue can be somewhat alleviated through anomaly detection and spectral similarity algorithms proposed by Zhang et al. (Citation2022), other issues still moderately impact the application of GI images in multi-temporal analysis.
6. Conclusion
In this work, a novel methodology for identifying and classifying illumination sources was proposed using freely accessible GI images captured by the SDGSAT-1 satellite, exemplified effectively using the case of Beijing. This research first derived three distinct sets of feature variables – spectral, textural, and road class – based on GI images and OSM road data. Subsequently, these feature variables were subjected to redundancy analysis followed by dimensionality reduction to enhance computational efficiency. Ultimately, a range of models was established and compared, with the optimal performer model chosen for light source prediction. The following conclusions can be drawn:
The proposed method can effectively distinguish the three primary illumination source types in Beijing: LED, HPS, and FL. When using spectral and textural variables for ALAN type differentiation, the overall accuracy reached 0.92. Upon incorporating road type variables, the overall accuracy for streetlight type differentiation increased to 0.95.
The lighting classification results appropriately reveal urban illumination patterns. HPS light sources dominate both ALAN types and streetlight types in Beijing, followed by LED sources, while the application of FL sources remains notably limited. The illumination pattern in Beijing is demarcated by the 5th Ring Road, wherein HPS sources constitute over 70% within its bounds. Beyond the 5th Ring Road, LED usage experiences a significant increase, with LED and HPS sources nearly evenly distributed.
The geodetector results demonstrate that road class accounts for approximately 25.19% of the variance in streetlight types, and there is a statistically significant difference between the road class and streetlight type. As road class decreases, the utilization of LED streetlights grows from 8.81% to 34.18%. This phenomenon is attributed to LED light sources exhibiting uneven illumination and lower power outputs, rendering them unsuitable for high-speed roads, broad thoroughfares, and other higher road classes.
This work validated the pivotal role of GI images in ALAN research, while also offering valuable insights into understanding urban development and its influencing factors.
Acknowledgments
We thank the anonymous reviewers and the editors for constructive comments that helped improve the manuscript.
Disclosure statement
No potential conflict of interest was reported by the author(s).
Data availability statement
The data that support the findings of this study are available from the corresponding author upon reasonable request.
Additional information
Funding
References
- Boeing, Geoff. 2019. “Urban Spatial Order: Street Network Orientation, Configuration, and Entropy.” Applied Network Science 4 (1): 67. https://doi.org/10.1007/s41109-019-0189-1.
- Breiman, L. 2001. “Random Forests.” Machine Learning 45 (1): 5–32. https://doi.org/10.1023/A:1010933404324.
- Brons, J. A., J. D. Bullough, and D. C. Frering. 2021. “Rational Basis for Light Emitting Diode Street Lighting Retrofit Luminaire Selection.” Transportation Research Record 2675 (9): 634–638. https://doi.org/10.1177/03611981211003890.
- Chen, T. Q., C. Guestrin, and Machinery Assoc Comp. 2016. “XGBoost: A Scalable Tree Boosting System.” Paper Presented at the 22nd ACM SIGKDD International Conference on Knowledge Discovery and Data Mining (KDD), San Francisco, CA, August 13–17.
- Cheng, B., Z. Q. Chen, B. L. Yu, Q. X. Li, C. X. Wang, B. B. Li, B. Wu, Y. Li, and J. P. Wu. 2020. “Automated Extraction of Street Lights from JL1-3B Nighttime Light Data and Assessment of their Solar Energy Potential.” Ieee Journal of Selected Topics in Applied Earth Observations and Remote Sensing 13:675–684. https://doi.org/10.1109/JSTARS.2020.2971266.
- Davies, Thomas W., Jonathan Bennie, Richard Inger, Natalie Hempel Ibarra, and Kevin J. Gaston. 2013. “Artificial Light Pollution: Are Shifting Spectral Signatures Changing the Balance of Species Interactions?” Global Change Biology 19 (5): 1417–1423. https://doi.org/10.1111/gcb.12166.
- Decker, A. G., T. J. Malthus, M. M. Wijnen, and E. Seyhan. 1992. “The Effect of Spectral Bandwidth and Positioning on the Spectral Signature Analysis of Inland Waters.” Remote Sensing of Environment 41 (2–3): 211–225. https://doi.org/10.1016/0034-4257(92)90079-Y.
- Dixon, M. E. 1965. “The Two-colour Diagram as a Key to Past Rates of Star Formation and Past Rates of Maetal Enrichment of the Interstellar Medium.” Monthly Notices of the Royal Astronomical Society 129 (1): 51–61. https://doi.org/10.1093/mnras/129.1.51.
- Djuretic, A., and M. Kostic. 2018. “Actual Energy Savings When Replacing High-pressure Sodium with LED Luminaires in Street Lighting.” Energy 157:367–378. https://doi.org/10.1016/j.energy.2018.05.179.
- Dobler, G., M. Ghandehari, S. E. Koonin, and M. S. Sharma. 2016. “A Hyperspectral Survey of New York City Lighting Technology.” Sensors 16 (12): 2047. https://doi.org/10.3390/s16122047.
- Elvidge, C. D., P. Cinzano, D. R. Pettit, J. Arvesen, P. Sutton, C. Small, R. Nemani, et al. 2007. “The Nightsat Mission Concept.” International Journal of Remote Sensing 28 (12): 2645–2670. https://doi.org/10.1080/01431160600981525.
- Elvidge, C. D., D. M. Keith, B. T. Tuttle, and K. E. Baugh. 2010. “Spectral Identification of Lighting Type and Character.” Sensors 10 (4): 3961–3988. https://doi.org/10.3390/s100403961.
- Falchi, F., and S. Bara. 2020. “A Linear Systems Approach to Protect the Night Sky: Implications for Current and Future Regulations.” Royal Society Open Science 7 (12): 201501. https://doi.org/10.1098/rsos.201501.
- Falchi, F., R. Furgoni, T. A. Gallaway, N. A. Rybnikova, B. A. Portnov, K. Baugh, P. Cinzano, and C. D. Elvidge. 2019. “Light Pollution in USA and Europe: The Good, the Bad and the Ugly.” Journal of Environmental Management 248:109227. https://doi.org/10.1016/j.jenvman.2019.06.128.
- Gaston, K. J., T. W. Davies, J. Bennie, and J. Hopkins. 2012. “REVIEW: Reducing the Ecological Consequences of Night-time Light Pollution: Options and Developments.” Journal of Applied Ecology 49 (6): 1256–1266. https://doi.org/10.1111/j.1365-2664.2012.02212.x.
- Gaston, K. J., and A. S. de Miguel. 2022. “Environmental Impacts of Artificial Light at Night.” Annual Review of Environment and Resources 47 (1): 373–398. https://doi.org/10.1146/annurev-environ-112420-014438.
- Gaston, K. J., J. P. Duffy, S. Gaston, J. Bennie, and T. W. Davies. 2014. “Human Alteration of Natural Light Cycles: Causes and Ecological Consequences.” Oecologia 176 (4): 917–931. https://doi.org/10.1007/s00442-014-3088-2.
- Gregorutti, B., B. Michel, and P. Saint-Pierre. 2017. “Correlation and Variable Importance in Random Forests.” Statistics and Computing 27 (3): 659–678. https://doi.org/10.1007/s11222-016-9646-1.
- Grubisic, M., R. H. A. van Grunsven, A. Manfrin, M. T. Monaghan, and F. Holker. 2018. “A Transition to White LED Increases Ecological Impacts of Nocturnal Illumination on Aquatic Primary Producers in a Lowland Agricultural Drainage Ditch.” Environmental Pollution 240:630–638. https://doi.org/10.1016/j.envpol.2018.04.146.
- Grundland, M., and N. A. Dodgson. 2007. “Decolorize: Fast, Contrast Enhancing, Color to Grayscale Conversion.” Pattern Recognition 40 (11): 2891–2896. https://doi.org/10.1016/j.patcog.2006.11.003.
- Guo, H. D., C. Y. Dou, H. Y. Chen, J. B. Liu, B. H. Fu, X. M. Li, Z. M. Zou, and D. Liang. 2023. “SDGSAT-1: The World's First Scientific Satellite for Sustainable Development Goals.” Science Bulletin 68 (1): 34–38. https://doi.org/10.1016/j.scib.2022.12.014.
- Guo, Biyun, Deyong Hu, and Qiming Zheng. 2023. “Potentiality of SDGSAT-1 Glimmer Imagery to Investigate the Spatial Variability in Nighttime Lights.” International Journal of Applied Earth Observation and Geoinformation 119:103313. https://doi.org/10.1016/j.jag.2023.103313.
- Hale, J. D., G. Davies, A. J. Fairbrass, T. J. Matthews, C. D. F. Rogers, and J. P. Sadler. 2013. “Mapping Lightscapes: Spatial Patterning of Artificial Lighting in an Urban Landscape.” PLoS One 8 (5): e61460. https://doi.org/10.1371/journal.pone.0061460.
- Hall-Beyer, M. 2017. “Practical Guidelines for Choosing GLCM Textures to Use in Landscape Classification Tasks Over a Range of Moderate Spatial Scales.” International Journal of Remote Sensing 38 (5): 1312–1338. https://doi.org/10.1080/01431161.2016.1278314.
- Han, P. P., J. L. Huang, R. D. Li, L. H. Wang, Y. X. Hu, J. L. Wang, and W. Huang. 2014. “Monitoring Trends in Light Pollution in China Based on Nighttime Satellite Imagery.” Remote Sensing 6 (6): 5541–5558. https://doi.org/10.3390/rs6065541.
- Haralick, Robert M., K. Shanmugam, and Its'Hak Dinstein. 1973. “Textural Features for Image Classification.” IEEE Transactions on Systems, Man, and Cybernetics SMC-3 (6): 610–621. https://doi.org/10.1109/TSMC.1973.4309314.
- Hatori, Megumi, Claude Gronfier, Russell N. Van Gelder, Paul S. Bernstein, Josep Carreras, Satchidananda Panda, Frederick Marks, et al. 2017. “Global Rise of Potential Health Hazards Caused by Blue Light-induced Circadian Disruption in Modern Aging Societies.” NPJ Aging and Mechanisms of Disease 3 (1): 9–9. https://doi.org/10.1038/s41514-017-0010-2.
- Hu, X. F., C. Z. Hu, H. C. Xu, Y. C. He, and D. W. Tang. 2023. “Polyethersulfone Wick and Metal Wick Based Loop Heat Pipe for LED Street Light Thermal Management.” Case Studies in Thermal Engineering 49:103175. https://doi.org/10.1016/j.csite.2023.103175.
- IEA. 2006. Light's Labour's Lost. Paris: IEA.
- Iqbal, N., R. Mumtaz, U. Shafi, and S. M. H. Zaidi. 2021. “Gray Level Co-occurrence Matrix (GLCM) Texture Based Crop Classification Using Low Altitude Remote Sensing Platforms.” Peerj Computer Science, e536. https://doi.org/10.7717/peerj-cs.536.
- Javed, A., Q. M. Cheng, H. Peng, O. Altan, Y. Li, I. Ara, E. Huq, Y. Ali, and N. Saleem. 2021. “Review of Spectral Indices for Urban Remote Sensing.” Photogrammetric Engineering and Remote Sensing 87 (7): 513–524. https://doi.org/10.14358/PERS.87.7.513.
- Kaiser, Henry F. 1970. “A Second Generation Little Jiffy.” Psychometrika 35 (4): 401–415. https://doi.org/10.1007/BF02291817.
- Kaiser, Henry F., and Rice John. 1974. “Little Jiffy, Mark Iv.” Educational and Psychological Measurement 34 (1): 111–117. https://doi.org/10.1177/001316447403400115.
- Kobav, M. B., and G. Bizjak. 2012. “Led Spectra and Melatonin Suppression Action Function.” Light & Engineering 20 (3): 15–22.
- Kruse, F. A., and C. D. Elvidge. 2011. “Identification and Mapping of Night Lights Signatures Using Hyperspectral Data.” Paper Presented at the Conference on Algorithms and Technologies for Multispectral, Hyperspectral, and Ultraspectral Imagery XVII, Orlando, FL, April 25–28.
- Kuechly, H. U., C. C. M. Kyba, T. Ruhtz, C. Lindemann, C. Wolter, J. Fischer, and F. Holker. 2012. “Aerial Survey and Spatial Analysis of Sources of Light Pollution in Berlin, Germany.” Remote Sensing of Environment 126:39–50. https://doi.org/10.1016/j.rse.2012.08.008.
- Kyba, C. C. M. 2018. “Is Light Pollution Getting Better or Worse?” Nature Astronomy 2 (4): 267–269. https://doi.org/10.1038/s41550-018-0402-7.
- Kyba, C. C. M., T. Kuester, A. S. de Miguel, K. Baugh, A. Jechow, F. Holker, J. Bennie, C. D. Elvidge, K. J. Gaston, and L. Guanter. 2017. “Artificially Lit Surface of Earth at Night Increasing in Radiance and Extent.” Science Advances 3 (11). https://doi.org/10.1126/sciadv.1701528.
- Levin, N., C. C. M. Kyba, Q. L. Zhang, A. S. de Miguel, M. O. Roman, X. Li, B. A. Portnov, et al. 2020. “Remote Sensing of Night Lights: A Review and an Outlook for the Future.” Remote Sensing of Environment 237:111443. https://doi.org/10.1016/j.rse.2019.111443.
- Lillesand, Thomas, Ralph W Kiefer, and Jonathan Chipman. 2015. Remote Sensing and Image Interpretation. New York: John Wiley & Sons.
- Longcore, T., A. Rodriguez, B. Witherington, J. F. Penniman, L. Herf, and M. Herf. 2018. “Rapid Assessment of Lamp Spectrum to Quantify Ecological Effects of Light at Night.” Journal of Experimental Zoology Part a-Ecological and Integrative Physiology 329 (8–9): 511–521. https://doi.org/10.1002/jez.2184.
- Ma, H. K., and Y. T. Li. 2015. “Thermal Performance of a Dual-sided Multiple Fans System with a Piezoelectric Actuator on LEDs.” International Communications in Heat and Mass Transfer 66:40–46. https://doi.org/10.1016/j.icheatmasstransfer.2015.05.008.
- Moghtaderi, A., F. Moore, and A. Mohammadzadeh. 2007. “The Application of Advanced Space-borne Thermal Emission and Reflection (ASTER) Radiometer Data in the Detection of Alteration in the Chadormalu Paleocrater, Bafq Region, Central Iran.” Journal of Asian Earth Sciences 30 (2): 238–252. https://doi.org/10.1016/j.jseaes.2006.09.004.
- Munyati, Christopher. 2004. “Use of Principal Component Analysis (PCA) of Remote Sensing Images in Wetland Change Detection on the Kafue Flats, Zambia.” Geocarto International 19 (3): 11–22. https://doi.org/10.1080/10106040408542313.
- Öhman, Yngve. 1949. “Photoelectric Work by the Flicker Method.” Stockholms Observatoriums Annaler 15:8.1–8.46.
- Puschnig, J., T. Posch, and S. Uttenthaler. 2014. “Night Sky Photometry and Spectroscopy Performed at the Vienna University Observatory.” Journal of Quantitative Spectroscopy & Radiative Transfer 139:64–75. https://doi.org/10.1016/j.jqsrt.2013.08.019.
- Rybnikova, N., A. S. de Miguel, S. Rybnikov, and A. Brook. 2021. “A New Approach to Identify On-ground Lamp Types from Night-time ISS Images.” Remote Sensing 13 (21): 4413. https://doi.org/10.3390/rs13214413.
- Sánchez de Miguel, Alejandro, Christopher C. M. Kyba, Martin Aubé, Jaime Zamorano, Nicolas Cardiel, Carlos Tapia, Jon Bennie, and Kevin J. Gaston. 2019. “Colour Remote Sensing of the Impact of Artificial Light at Night (I): The Potential of the International Space Station and Other DSLR-Based Platforms.” Remote Sensing of Environment 224:92–103. https://doi.org/10.1016/j.rse.2019.01.035.
- Schroer, Sibylle, and Franz Hölker. 2016. “Impact of Lighting on Flora and Fauna.” In Handbook of Advanced Lighting Technology, edited by Robert Karlicek, Ching-Cherng Sun, Georges Zissis, and Ruiqing Ma, 1–33. Cham: Springer International Publishing.
- Schulte-Römer, Nona, Josiane Meier, Max Söding, and Etta Dannemann. 2019. “The LED Paradox: How Light Pollution Challenges Experts to Reconsider Sustainable Lighting.” Sustainability 11 (21): 6160. https://doi.org/10.3390/su11216160.
- Shanmuganathan, Subana. 2016. “Artificial Neural Network Modelling: An Introduction.” In Artificial Neural Network Modelling, edited by Subana Shanmuganathan and Sandhya Samarasinghe, 1–14. Cham: Springer International Publishing.
- Tardà, Anna, Vicenç Palà, Roman Arbiol, Fernando Pérez, Oriol Viñas, Luca Pipia, and Lucas Martínez. 2011. Detección de la iluminación exterior urbana nocturna con el sensor aerotransportado CASI-550. Barcelona, Spain: Internation Geomatic Week.
- Wang, M., Q. Q. Li, Q. W. Hu, and M. Zhou. 2013. “Quality Analysis of Open Street Map Data.” 8th International Symposium on Spatial Data Quality XL-2/W1:155–158. https://doi.org/10.5194/isprsarchives-XL-2-W1-155-2013.
- Wang, Jinfeng, and Chengdong Xu. 2017. “Geodetector: Principle and Prospective.” Acta Geographica Sinica 72 (1): 116–134.
- Wang, J. F., T. L. Zhang, and B. J. Fu. 2016. “A Measure of Spatial Stratified Heterogeneity.” Ecological Indicators 67:250–256. https://doi.org/10.1016/j.ecolind.2016.02.052.
- Wang, F. Y., K. Zhou, M. C. Wang, and Q. Wang. 2020. “The Impact Analysis of Land Features to JL1-3B Nighttime Light Data at Parcel Level: Illustrated by the Case of Changchun, China.” Sensors 20 (18): 5447. https://doi.org/10.3390/s20185447.
- Wright, Raymond E.. 1995. “Logistic Regression.” In Reading and understanding multivariate statistics, edited by Grimm Laurence G and Yarnold Paul R, 217–244. Washington, DC: American Psychological Association.
- Xiaona, Li, and Luo Nianxue. 2019. “Positional Accuracy Analysis Method and Experiment of OSM Planar Elements Based on Geometric Integrity.” Journal of Geomatics 44 (2): 101–104. https://doi.org/10.14188/j.2095-6045.2017081.
- Yin, S. R., T. Oliveira, A. Murthy, and ACM. 2017. “Automated Lamp-Type Identification for City-Wide Outdoor Lighting Infrastructures.” Paper Presented at the 18th International Workshop on Mobile Computing Systems and Applications (HotMobile), Sonoma, CA, February 21–22.
- Yu, B., F. Chen, C. Ye, Z. W. Li, Y. Dong, N. Wang, and L. Wang. 2023. “Temporal Expansion of the Nighttime Light Images of SDGSAT-1 Satellite in Illuminating Ground Object Extraction by Joint Observation of NPP-VIIRS and Sentinel-2A Images.” Remote Sensing of Environment 295:113691. https://doi.org/10.1016/j.rse.2023.113691.
- Zhang, D. G., B. Cheng, L. Shi, J. Gao, T. F. Long, B. Chen, and G. Z. Wang. 2022. “A Destriping Algorithm for SDGSAT-1 Nighttime Light Images Based on Anomaly Detection and Spectral Similarity Restoration.” Remote Sensing 14 (21): 5544. https://doi.org/10.3390/rs14215544.
- Zhao, M., Y. Y. Zhou, X. C. Li, W. T. Cao, C. Y. He, B. L. Yu, X. Li, C. D. Elvidge, W. M. Cheng, and C. H. Zhou. 2019. “Applications of Satellite Remote Sensing of Nighttime Light Observations: Advances, Challenges, and Perspectives.” Remote Sensing 11 (17): 1971. https://doi.org/10.3390/rs11171971.
- Zheng, Qiming, Qihao Weng, Lingyan Huang, Ke Wang, Jinsong Deng, Ruowei Jiang, Ziran Ye, and Muye Gan. 2018. “A New Source of Multi-spectral High Spatial Resolution Night-Time Light Imagery—JL1-3B.” Remote Sensing of Environment 215:300–312. https://doi.org/10.1016/j.rse.2018.06.016