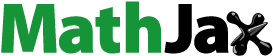
ABSTRACT
Ground subsidence is a common geological hazard in urban areas that endangers the safety of infrastructure, such as subways. In this study, the ground subsidence risk assessment method considering both ground subsidence intensity and susceptibility is proposed and applied to assess ground subsidence risk of the Shanghai Metro network. Initially, PS-InSAR is used for the ground subsidence survey in the Shanghai Metro area. Subsequently, ten subsidence causal factors are collected, and the LightGBM machine learning algorithm is employed to conduct the ground subsidence susceptibility analysis. Then, a risk matrix is introduced to define ground subsidence risk by combining subsidence intensity and susceptibility. Finally, the risk map is generated in ArcGIS and classified into five levels. The assessment results were used to identify ground subsidence risk at different scales. The results indicate that the risk is higher in the southwest part of the study area, and the ground subsidence risk of the metro network exhibits a regional-related characteristic. On-site investigations were conducted to verify the results. The method enables fast ground subsidence assessment over a large area at a low cost and the assessment results can provide data for the prevention and management of ground subsidence hazards in the city.
1. Introduction
Ground subsidence is a common geohazard caused by multiple factors in urban areas, and it is characterized by long-lasting effects and a wide range of occurrences (Bagheri-Gavkosh et al. Citation2021). It not only damages surface structures but also affects underground infrastructure, such as metro lines (Wu, Shen, and Yang Citation2017). Ground subsidence has been observed in many cities worldwide, including Mexico City (Cabral-Cano et al. Citation2008), Tokyo (Sato, Haga, and Nishino Citation2006), and Shanghai (Xue et al. Citation2005). Shanghai has undergone rapid economic and urban development over the past few decades and is now China's international center for economy, finance, and trade. However, factors such as urban construction (Xu et al. Citation2012), groundwater extraction (Xu et al. Citation2016), unfavorable geological conditions (Gong, Li, and Yang Citation2009), among others, have led to significant ground subsidence in Shanghai. Meanwhile, the Shanghai Metro network has rapidly expanded with the population growth. Ground subsidence can induce tunnel settlement (Wu, Shen, and Yang Citation2017). For example, Shanghai Metro Line 1 experienced pronounced uneven settlement, and it was observed that the corresponding ground subsidence was also serious where tunnel settlement happened, such as People's Square Station (Shen et al. Citation2014). Uneven settlement in tunnels can further lead to joint opening, water leakage and segment cracking, and other tunnel diseases. These diseases can deteriorate the operating environment of the tunnel and endanger the operational safety of the tunnel. Therefore, it is necessary to conduct the ground subsidence assessment of the metro network to ensure its safe operation.
Specification for Land Subsidence Survey and Monitor in china specifies that ground subsidence assessment needs to consider both its intensity and susceptibility. On the one hand, the intensity reflects the severity of ground subsidence. And higher intensity levels typically result in more significant ground damage. Ground subsidence surveys play a crucial role in assessing the intensity and spatial distribution of subsidence. Traditional methods like levelling and GPS offer highly accurate measurements (Motagh et al. Citation2007). However, their reliance on instruments or manual operation poses challenges for large-scale and high-density surveys due to manpower and financial constraints in practical engineering scenarios. Satellite-based Synthetic Aperture Radar (SAR) is a remote sensing technology that emits microwaves toward the ground and receives the echo signal (Chan and Koo Citation2008). Subsequently, the reflective properties and phase information of the ground object are recorded in SAR images. Interferometric Synthetic Aperture Radar (InSAR) technique was initially exploited for the inversion of ground surface elevation through the processing of two SAR images (Graham Citation1974). Subsequently, Differential interferometric Synthetic Aperture Radar technique (D-InSAR) was proposed to measure ground derformation (Gabriel, Goldstein, and Zebker Citation1989). And it has been successfully applied to measure deformations related to earthquake (Yang et al. Citation2017), volcano (Tomiyama, Koike, and Omura Citation2004), and other crustal activities. Nevertheless, the measurement accuracy of D-InSAR suffers from the spatial and temporal decorrelation, as well as atmospheric delay. To address these limitations, time-series InSAR techniques were developed. Persistent Scatterer Interferometric Synthetic Aperture Radar (PS-InSAR) and Small-Baseline Subset (SBAS-InSAR) are two popular time-series InSAR techniques (Berardino et al. Citation2002; Ferretti, Prati, and Rocca Citation2001). PS-InSAR excels in monitoring stable and coherent scatterers over time, making it particularly suitable for urban and infrastructure deformation studies. In contrast, SBAS-InSAR optimizes coherence by selecting interferograms with small temporal and spatial baselines, making it suitable for regional-scale deformation assessments. Some pixels of SAR images can maintain high coherence and strong reflective properties over a long period of time, which are referred to as Persistent Scatterers (PSs) (Ferretti, Prati, and Rocca Citation2001). In the real world, PSs can be buildings, lighthouses, rocks, concrete dikes, and artificial corner reflectors, among others. PS-InSAR processes multiple SAR images to search PSs and analyzes their phase information, then the deformation of PSs can be calculated through mathematical modeling. PS-InSAR offers several advantages, including a broad monitoring range, high accuracy, dense monitoring results, cost-effectiveness, and access to historical data. Consequently, it has been widely applied to measure surface deformation of different phenomena: urban land subsidence (Malik et al. Citation2022; Wang et al. Citation2022), groundwater extraction (Hussain et al. Citation2022), coastal reclamation area (Li et al. Citation2022), and infrastructure subsidence (An et al. Citation2023; Chang, Dollevoet, and Hanssen Citation2018; Reinders et al. Citation2021; Wang et al. Citation2017).
On the other hand, ground subsidence is a complex phenomenon influenced by multiple causal factors (Bianchini et al. Citation2019). Ground subsidence susceptibility (GSS) analysis is used to assess and predict ground vulnerability to subsidence under the multiple causal factors (Fadhillah, Achmad, and Lee Citation2020). Many statistical methods, including the Frequency Ratio model (Cao et al. Citation2016), Weigh of Evidence (WOE) (Xie et al. Citation2017) and mathematical probabilistic models like Fuzzy Logic (Bianchini et al. Citation2019), are applied in GSS analysis. With advancements in computer science, machine learning algorithms, such as Artificial Neural Network (ANN) (Lee, Park, and Choi Citation2012), Logistic Regression (Tien Bui et al. Citation2018), Support Vector Machine (Abdollahi et al. Citation2019), Decision Tree (Lee and Park Citation2013), Random Forest (Chen et al. Citation2017), have been gradually used in GSS analysis. Machine learning algorithms can explore the correlation between features and labels better when facing large amounts of data than statistical methods. Some studies on the comparison of machine learning algorithms show that Gradient Boosting Decision Tree (GBDT) algorithms have better accuracy compared with other algorithms(Rong et al. Citation2020; Sachdeva and Kumar Citation2021). However, many GBDT algorithms usually face the problem of inefficiency when dealing big data (Friedman Citation2001). LightGBM was proposed to solve the above problem. It has the advantages of faster computational speed and less memory consumption compared to other GBDT algorithms (Ke et al. Citation2017). In GSS analysis, the Receiver Operating Characteristic (ROC) curve and the Area Under the ROC Curve (AUC) value are commonly employed to assess the performance of models (Fadhillah, Achmad, and Lee Citation2020; Fawcett Citation2006).
Currently, within the field of ground subsidence assessment, most of the studies mainly focus on ground subsidence survey by satellite InSAR or ground subsidence susceptibility analysis. Only a limited number of studies consider both the subsidence intensity and susceptibility (Jin et al. Citation2022). In this study, a comprehensive ground subsidence assessment method, incorporating both subsidence intensity and susceptibility, was proposed and applied to assess ground subsidence within the Shanghai Metro network. Initially, PS-InSAR technology was utilized to investigate ground subsidence within the Shanghai Metro area from May 2016 to December 2017. Ten causal factors for ground subsidence were selected to conduct GSS analysis by LightGBM. A risk matrix was introduced to define ground subsidence risk levels by combining ground subsidence intensity and susceptibility. Finally, the ground subsidence risk map was generated in ArcGIS. Subsequently, it was utilized to identify areas with higher risk levels at different scales and to analyze risk characteristics of the Shanghai Metro network. Verification of the assessment results was carried out through on-site investigations.
The paper is structured as follows. The study area is introduced in section 2. And section 3 presents materials and methods. The results are introduced in Section 4 and discussed in Section 5. Conclusions are summarized in Section 6.
2. Study area
Shanghai is situated in the Yangtze River Delta region of China, with coordinates ranging from 120°52′ to 122°12′ longitude and 30°40′ to 31°53′ latitude, covering an area of 6340.5 km2. The Yangtze River Delta region is characterized by the presence of soft and loose sediments, including clay, silt, and sand. This geological composition makes it susceptible to compaction and consolidation when subjected to heavy loads or excessive groundwater extraction. Shanghai experiences a humid subtropical climate with distinct wet and dry seasons. High levels of precipitation during the wet season can lead to significant groundwater recharge. Over the past few decades, rapid development in Shanghai has been accompanied by notable ground subsidence. The main factors triggering ground subsidence include the exploitation of groundwater (Shen and Xu Citation2011), urbanization (Xu et al. Citation2012) and unfavorable geology, etc.
As the end of 2021, the resident population of Shanghai was about 24.87 million. To reduce the pressure of urban traffic, 19 metro lines with a total operational mileage of 802 km had been built and put into operation ().
3. Materials and methods
3.1. Research data
3.1.1. SAR datasets
In this study, 40 Sentinel 1-A ascending SAR images were used to investigate ground subsidence in the Shanghai Metro area. The Sentinel 1-A satellite is equipped with a C-band SAR and offers various types of Single Look Complex (SLC) images. The Interferometric Wide (IW) swath mode images cover a large area with medium resolution. Sentinel 1-A has a revisit period of 12 days, enabling continuous monitoring of ground subsidence ().
Table 1. The parameters of Sentinel 1-A SAR images.
3.1.2. Ground subsidence causal factors
Many factors contribute to ground subsidence. Previous GSS studies have revealed correlations between ground subsidence and various factors such as topography, geology, hydrology, and human activities (Bianchini et al. Citation2019; Fadhillah, Achmad, and Lee Citation2020). Accordingly, ten ground subsidence causal factors were collected in this study.
The Digital Elevation Model (DEM) provides the elevation information about the ground (). Shanghai's topography is relatively flat with an average elevation of approximately 4 meters. The strata in Shanghai are mainly composed of soft soils. Typically, higher elevation values correspond to thicker strata. The long-term deformation of these strata due to the compression is an important reason for ground subsidence in Shanghai (Gong, Li, and Yang Citation2009).
Slope represents the steepness of the terrain and serves as an indicator of ground stability, as terrain naturally gravitates towards lower slopes (Kim et al. Citation2018). Consequently, slope can be considered as one of the factors influencing ground subsidence. The DEM data was used to generate slope data ().
Geological factors include landform, quaternary strata and distance to fault. Landforms refer to surface features that define the topography. Landforms denote surface features defining topography, each possessing distinct attributes such as elevation, orientation, slope, soil type, and characteristics. These attributes directly or indirectly contribute to ground subsidence. I4 is Huangpu River Floodplain, II1 is Ancient Coastal Plain, II2 is Old Coastal Plain, II3 is Early Coastal Plain, II4 is Mid-period Coastal Plain, II5 is New Coastal Plain, II6 is Coastal Plain, III1 is Lacustrine Plain, III2 is Lake-swamp Plain, III3 is Lake-swamp Depression, III4 is Lagoon Plain and Ⅳ is Shell Sand Bank (c).
Figure 2. Ten causal factors of ground subsidence: (a) DEM; (b) Slope; (c) Landform; (d) Quaternary strata; (e) Distance to fault; (f) Distance to river; (g) TWI; (h) Landuse; (i) Distance to road; (j) Distance to metro lines.
The thickness of the Quaternary strata in shanghai is approximately 300 m. The compression of these strata due to water extraction significantly contributes to ground subsidence (Xu, Shen, and Du Citation2009). 1 is Littoral Zone Tidal Flat Deposition, 2 is Gangshen Zone Tidal Flat Deposition, 3 is Littoral Zone Tidal Flat Deposition of North shore of Hangzhou Bay, 4 is Estuarine Shore Deposition, 5 is Sandspit Deposition, 6 is Tributary Channel Deposition, 7 is Lake Deposition, 8 is Lake Highland Deposition, 9 is Lake Lowland Deposition, 10 is Lacustrine Deposition, 11 is Shell Sand Deposition (d).
Faults play a significant role in controlling the structural features of ground subsidence (Cigna et al. Citation2012). The tectonic activity of faults may change in the structure of nearby strata, resulting in differential ground subsidence on both sides of fault line (). Typically, this effect diminishes as the distance from the fault increases (Chen et al. Citation2017).
Groundwater extraction and replenishment are the main factors affecting subsidence in Shanghai (Shi et al. Citation2008). Rivers have a replenishing effect on groundwater. There are many rivers and lakes in Shanghai, such as the well-known Huangpu River and its tributaries, the Suzhou River, and the Dianshan Lake, etc. Previous studies have demonstrated a correlation between groundwater levels and proximity to rivers (Fadhillah, Achmad, and Lee Citation2020) ().
The topographic witness index (TWI) is a factor that describes the influence of topography on hydrological processes ( 2g). It represents the degree of accumulated water flow at a certain location, which can indirectly contribute to ground subsidence (Abdollahi et al. Citation2019).
Landuse data includes various land types, each associated with different ground building density, loads, and vegetation coverage (). These attributes mirror both natural landscapes and human-induced alterations (Minderhoud et al. Citation2018). Human activities, such as construction activities, can transform land type from undeveloped area to urban region. Such construction activities and the subsequent introduction of new loads are important causal factors of ground subsidence.
Roads experience soil compression beneath them due to traffic loads, leading to ground subsidence during operation (Lu et al. Citation2018). Generally, the subsidence magnitude typically correlates with load intensity and years of usage ().
Metro construction activities, such as excavation, tunneling, and dewatering, can induce ground subsidence (Tan et al. Citation2017). Moreover, during metro operation, factors like train vibrations, leaks, and compression settlement of the underlying soil layers can also contribute to ground subsidence. In general, ground subsidence tends to decrease with an increase in the distance from the metro lines (Peck Citation1969). Therefore, distance to metro lines is also chosen as a causal factor ().
3.2. Methods
The workflow of the entire methodology employed in this work is presented ().
3.2.1. Ground subsidence survey by PS-InSAR
PS-InSAR was used to process 40 Sentinel 1-A SAR images, identifying Persistent Scatterers (PSs) and analyzing their deformation. Then the deformation data of PSs was exported to generate ground subsidence survey map ().
The first step of PS-InSAR is to select a primary image. The selection of the primary image generally considers parameters such as temporal coherence, spatial coherence, doppler coherence, etc.
Secondly, the remaining images are sequentially registered with the primary image, then the interferograms can be generated. The interferometric phase consists of multiple components, such as deformation, topography, noise, orbit and atmosphere phase, etc. as shown in equation (1).
(1)
(1) where
is the interferometric phase;
is the deformation phase;
is the topography error phase;
is the atmospheric delayed phase;
is the orbit phase;
is the noise phase. Furthermore, an external DEM is used to remove the topographic phase. The deformation phase of pixels can be obtained after removing the other phases.
Thirdly, the phase standard deviation is to identify the PSs, as shown in equation (2). In the condition of high signal-to-noise ratio (SNR), the amplitude dispersion index (ADI) is closer to the phase standard deviation (Ferretti, Prati, and Rocca Citation2001).
(2)
(2) where,
is the phase standard deviation;
is the amplitude standard deviation;
is the amplitude mean difference;
is the amplitude dispersion index.
The atmospheric phase delay is estimated by using the temporal–spatial filtering operation. Low-pass and high-pass filtering operation is used in the spatial domain and temporal domain, respectively.
Finally, delaunay triangular network is constructed after the PSs were selected. Then the mathematical model about phase difference, deformation velocity and elevation correction between two adjacent PS points is established. Then the deformation component can be determined. The line of sight (LOS) deformation SLOS obtained by PS-InSAR can be transformed to vertical direction by equation (3). The deformation data of PSs is exported in ArcGIS to generate the ground subsidence survey map. Sv θ is the local incidence angle.
(3)
(3)
3.2.2. Frequency ratio method
The frequency ratio is a statistical method for analyzing the spatial correlation between two kinds of data (Manap et al. Citation2014). In this study, Equation (4) was used to analyze the correlation between subsidence and its causal factors.
(4)
(4)
represents the number of subsidence pixels in the
classification of the
causal factor;
represents the total number of subsidence pixels of the
factor;
represents the total pixels of the
classification in the
causal factor;
represents the total pixels of the
causal factor. If the frequency ratio FR value is greater than 1, it is considered that there is a strong correlation between the factor and the subsidence. Instead, if the frequency ratio FR value is less than 1, it is considered that there is a weak correlation between the factor and the subsidence (Manap et al. Citation2014).
3.2.3. GSS by LightGBM and model validation
LightGBM is an improved gradient boosting decision tree (GBDT) algorithm, which employs multiple decision trees to make predictions by aggregating the conclusions of all decision trees. Given a dataset with a total of
samples, each with
features. LightGBM algorithm trains a strong model
by continuously learning and adding new decision trees while ensuring that the loss function
decreases gradually with addition of trees.
(5)
(5)
LightGBM adopts the histogram algorithm to improve the efficiency of finding split points of features. In addition, it applies the Gradient-based One-Side Sampling (GOSS) and exclusive feature bundling techniques to handle large amounts of data and features, greatly improving computational efficiency.
In binary classification tasks, the receiver operating characteristic curve (ROC) and area under the ROC curve (AUC) are used to assess the predictive performance of a model. ROC is created using the true positive rate (TPR) as the Y-axis against the false positive rate (FPR) as the X-axis at various threshold settings (Fawcett Citation2006). TPR measures the proportion of actual positive cases correctly identified by a classification model out of the total positive cases. FPR represents the proportion of negative cases that are incorrectly classified as positive by the model, out of the total negative cases.
(6)
(6)
(7)
(7)
Furthermore, the predictive accuracy has also been used to assess the predictive performance of classification model. It represents the proportion of correctly classified samples out of the total number of samples.
(8)
(8)
3.2.4. Ground subsidence risk matrix
In this study, ground subsidence risk levels were defined by the risk matrix combining ground subsidence susceptibility and intensity (Cigna and Tapete Citation2021). The susceptibility represents the possibility of subsidence and was divided into 5 categories: very low (0∼0.3), low (0.3∼0.5), medium (0.5∼0.7), high (0.7∼0.9), very high (0.9∼1). Intensity reflects the severity of ground subsidence and was divided into 5 categories, velocity > 0 mm/y, 0 mm/y > velocity > −5 mm/y, −5 mm/y > velocity > −10 mm/y, −10 mm/y > velocity > −20 mm/y, velocity < −20 mm/y. Ground subsidence risk was defined in five levels: very safe (R1), safe (R2), medium (R3), dangerous (R4), very dangerous (R5) ().
Table 2. Ground subsidence risk matrix defined by susceptibility and intensity.
4. Results
4.1. PS-InSAR ground subsidence survey results and validation
4.1.1. PS-InSAR ground subsidence survey results
40 Sentinel 1-a SAR images were used to perform PS-InSAR. The primary image acquired in 2017/05/22 was selected to minimize the temporal and perpendicular baselines (). DEM data from the STRM dataset was used to remove the topographic phase. Pixels with an ADI value less than 0.25 were selected as PSs. The settings for the atmospheric corrections included a 365-day atmospheric low-pass filter and a 1200-meter atmospheric high-pass filter ().
Table 3. The parameters of PS-InSAR data processing.
The LOS deformation results were transformed to the vertical direction and then imported into ArcGIS to generate ground subsidence survey map (). The color legend shows the deformation velocities of the ground, which ranges from −91.13 mm/y to 29.36 mm/y. The map shows that southeast part of Shanghai exhibits more pronounced subsidence trend. These areas are located in the Pudong, Fengxian, Jinshan, Songjiang and Minhang districts. In the middle part of the subsidence map, which corresponds to Hongkou, Yangpu, Jing’an, Huangpu, Changning and Xuhui districts. The subsidence velocities are relatively slow. In the north and northwest parts of the subsidence map, corresponding to Qingpu, Jiading and Baoshan districts, fewer areas show subsidence trend.
4.1.2. Validation of InSAR results
Measurements from five second-order precise levelling points were used to validate InSAR vertical deformation results. The red-numbered dots pinpoint these locations (): Minhang (point 1), Jiangding (point 2), Yangpu (point 3), and Pudong (point 4 and point 5). The maximum difference between the two datasets is 6.18 mm, with a root mean square error (RMSE) of 2.91 mm ().
4.2. FR values
In this study, all causal factors of ground subsidence were reclassified into ten categories using the quantile classification method, which ensures that each category represents an approximately equal proportion of the respective factor. Landuse, landform, and quaternary strata data had been classified in advance. Equation (4) was used to calculate the frequency ratio values ().
Table 4. FR values of 10 casual factors.
4.3. Ground subsidence susceptibility analysis by LightGBM and validation
The dataset used to train the GSS model consists of over 1.7 million samples. Each sample comprises FR values of the 10 causal factors along with InSAR ground subsidence measurement results at the same pixel. The FR values serve as features, while InSAR ground subsidence measurement results are used as labels. The dataset was divided into two groups, with 70% for model training and 30% for validation. The GSS model was trained using the LightGBM algorithm. Subsequently, the model predicted ground subsidence susceptibility for each pixel. Finally, the GSS map was generated in ArcGIS ().
The ground subsidence susceptibility values are high in the south, east and southeast part of map. These areas are located in the Pudong, Fengxian, Jinshan, Songjiang and Minhang districts. In the middle part of the subsidence map, which corresponds to the Hongkou, Yangpu, Jing’an, Huangpu, Changning, Putuo and Xuhui districts. The susceptibility values are moderate. In the north and northwest parts of the subsidence map, which corresponds to Qingpu, Jiading and Baoshan districts, the susceptibility values are low.
ROC curve and AUC were generated to assess the performance of the GSS model (). The AUC value is 0.902 and the accuracy is 0.817.
4.4. Ground subsidence risk mapping, assessment and analysis
4.4.1. Ground subsidence risk mapping and assessment at different scales
The ground subsidence risk map was generated in ArcGIS using the ground subsidence and susceptibility maps according to the risk matrix (a). The southeast area of the map has higher risk levels compared to the other areas. The risk levels mainly are R4 and R5 in the south part of the Pudong, Fengxian and east part of Jinshan districts. The risk levels are mainly R3 and R4 in the south part of Minhang, the east part of Songjiang and the middle of Pudong districts.
Figure 10. Ground subsidence risk mapping at different scales: (a) the whole city, (b) the southeast part of the map, (c) Line 16 (from Lingang Avenue Station to Dishui Lake Station), (d) the central part of the map, (e) Line11 (from Longyao Road Station to Oriental Sports Center Station), (f) the northwest part of the map.

The southeast area of the map was zoomed in (b). And there are many areas in the east coastal areas are at R5 risk. Metro Line 16 crosses the Pudong District, and ground subsidence risk level increases with the extension of the line 16 in southwest direction. The interval from Lingang Avenue Station to Dishuihu Station along Line 16 was further zoomed in (c), and most areas along the interval are at R5 risk.
In the central downtown area, including Huangpu, Yangpu, Jing'an, Putuo, Changning, Xuhui, Hongkou and northwest part of Pudong districts (d). Many metro lines run through these areas. The risk levels of ground subsidence in these areas are mainly R1 and R2. Meanwhile, there are some areas along the Huangpu River are at relatively higher risk levels. The interval from Longyao Road Station to the Oriental Sports Center Station is located in these areas, and ground subsidence risk of the interval located in the Pudong district is relatively high (e).
The risk levels in the north, west and northwest part of the map are mainly R1 and R2 (a, f). These areas are located in Baoshan, Jiading, Qingpu and the west part of Jinshan and Songjiang districts.
On-site investigations were conducted to validate the assessment results at four locations (c, e). Road collapses and cracks, building cracks were found near Dishui Lake Station (a, b). Road collapses and cracks were also found around Lingang Avenue Station (c) and along the Longyao Road Station to the Oriental Sports Center Station interval (d).
Figure 11. Ground subsidence risk on-site investigations results: (a) road collapses and (b) building cracks near Dishui Lake Station, (c) road collapses and cracks near Lingang Avenue Station, (d) road collapses and cracks along the interval from Longyao Road Station to the Oriental Sports Center Station.

4.4.2. Ground subsidence risk assessment of the administrative districts
The proportions of the five risk levels in different administrative districts were calculated, excluding Chongming (). Fengxian exhibits the highest proportions of R4 and R5 risks, while Songjiang demonstrates the highest proportion of R3 risk. Similarly, Changning shows the highest proportion of R2 risk, and Putuo district presents the highest proportion of R1 risk. With the exception of Songjiang, Minhang, Pudong, Jinshan and Fengxian, the proportions of the five ground subsidence risk levels gradually decrease from R1 to R5 in the other districts ().
Figure 12. The figure of ground subsidence risk levels and proportions in Shanghai administrative districts.

Table 5. The proportions of five ground subsidence risk levels in Shanghai administrative districts.
4.4.3. Ground subsidence risk assessment and analysis of metro network
The metro lines in Shanghai encompass stations and intervals, with a vital protection zone extending 50 m on both sides of these lines. Considering the 30 m resolution of the risk map, a 60 m buffer zone was chosen along the metro lines for assessment and analysis.
The proportions of the five risk levels were calculated for each metro line (). Among the metro lines, except for Line 5, Line 5 Branch, Line 16, and Pujiang line, R1 risk has the highest proportion. For these lines, there was a decreasing trend in the proportion of risk levels from R1 to R5, except for Line 18. Conversely, R3 and R4 are the primary risks for Line 5, Line 5 Branch, Line 16, and Pujiang line. These lines geographically cross areas with higher ground subsidence risk levels, such as Pudong, Minhang and Fengxian. Specifically, Line 11 Branch has the highest proportion of R1 risk among all risk levels, at 97.30%. Line 10 Branch has the highest proportion of R2 risk, at 40.18%. Pujiang line has the highest proportion of R3 risk, at 52.56%. Line 16 has the highest proportions of R4 and R5 risk levels, at 37.73% and 21.47%, respectively ().
Table 6. Ground subsidence risk levels of Shanghai Metro lines.
The proportions of the five risk levels for both station and interval areas were calculated (a). For interval areas, the proportions are as follows: 66.16% for R1, 16.21% for R2, 8.57% for R3, 6.65% for R4, and 2.41% for R5. Similarly, for station areas, the proportions are 74.37% for R1, 13.66% for R2, 6.29% for R3, 4.27% for R4, and 1.40% for R5. Additionally, we calculated the proportions of the five risk levels for areas within a radius of less than 10 meters, from 10 meters to 20 meters, and from 20 meters to 30 meters on both sides of the metro line. Within the radius of less than 10 meters from the metro line, 63.12% falls into the R1 risk category, 16.34% into R2, 10.05% into R3, 7.66% into R4, and 2.83% into R5. Within the range from 10 meters to 20 meters from the metro line, 67.19% falls into the R1 risk category, 14.45% into R2, 8.86% into R3, 7.15% into R4 and 2.35% into R5. Within the range from 20 meters to 30 meters from the metro line, 63.59% falls into the R1 risk category, 16.38% into R2, 9.90% into R3, 7.89% into R4, and 2.24% into R5.
5. Discussion
5.1. Ground subsidence survey results of Shanghai metro area by PS-InSAR
An accurate ground subsidence survey is crucial for generating a subsidence intensity map and conducting subsequent ground subsidence risk analysis. In this study, 40 Sentinel 1-A ascending-orbit SAR images were employed to generate the ground subsidence map in the Shanghai Metro area from May 2016 to December 2017. Vertical InSAR ground subsidence results were converted into a raster layer with a resolution of 30 meters. Measurements from five second-order precise levelling points were used to validate the InSAR results. The maximum difference observed was 6.18 mm, with a RMSE of 2.91 mm, demonstrating the high reliability of the PS-InSAR ground subsidence results.
A 200-meter-wide buffer zone along the metro network was established to analyze ground deformation velocities within it (). Within this zone, 93.94% of the metro area exhibited ground deformation velocities ranging from −10 mm/a to 10 mm/a (). Notably, Line 2 (from Yuandong Avenue to Pudong International Airport), Line 5 (from Xidu to Fengxian Xincheng), Line 15 (from Shujian Road to Zizhu Hi-tech Park), and Line 16 (from Xinchang to Dishui Lake) displayed higher subsidence velocities, with over 95% of the areas exhibiting a subsidence trend. The primary subsidence velocities ranged from −5 mm/a to −20 mm/a.
Furthermore, it is worth noting that Line 2 (from Yuandong Avenue to Pudong International Airport) and Line 16 (from Xinchang to Dishui Lake) are situated in the Pudong district, while Line 5 (from Xidu to Fengxian Xincheng) is located in the Fengxian district, and Line 15 (from Shujian Road to Zizhu Hi-tech Park) is situated in the southern part of the Minhang district. The subsidence intensity map reveals that the severity and extent of subsidence are most pronounced in the southeast portion of the map, particularly in the Pudong, Fengxian and south part of Minhang districts. In Pudong, more than 75% of the area is experiencing ground subsidence velocities ranging from −20 mm/a to 0 mm/a. Meanwhile, in Fengxian, over 97% of the area is within the same subsidence velocity range. Specifically, the average subsidence velocity in Pudong is 3.89 mm/a (a), while in Fengxian, it reaches 8.68 mm/a (b).
On one hand, a study analyzing nighttime light data observed a significant increase in nighttime illumination in Pudong and Fengxian districts, indicating heightened urbanization activities (Li et al. Citation2022). And urbanization often coincides with ground subsidence. On the other hand, these regions are geographically closer to the sea and lack the presence of the first and second (or second hard soil) layers (Wei Citation2010; Xu, Chen, and Zhao Citation2008). Therefore, these areas are more likely to produce subsidence, a typical consequence of unfavorable geological conditions. In contrast, inland regions such as the Qingpu and Jiading districts exhibited smaller subsidence intensity and area, owing to their more favorable geological conditions.
Sentinel-1A SAR images offer broad coverage with medium resolution, enabling comprehensive ground subsidence surveys of entire cities, such as Shanghai. They enable retrospective assessments, unlike traditional methods like levelling or GPS. While PS-InSAR provides dense and cost-effective data, it faces limitations. Atmospheric Phase Screen affects accuracy by inducing radar signal delays during transmission. Additionally, PS-InSAR struggles in vegetated or rapidly changing areas, limiting coverage along metro lines in this study. Furthermore, it is essential to emphasize that all deformation maps should be interpreted in a relative context. PS-InSAR measurements do not provide absolute deformation values. Since PS measurements are relative to a reference point, they offer high precision in estimating relative changes. Finally, the absolute localization accuracy of the PSs is limited due to factors such as orbit uncertainty, propagation delays, and scattering center uncertainty (Sousa et al. Citation2010). Nowadays, there are other high-resolution SAR sensors available. It is worth noting that high-resolution data can provide more details on ground subsidence and offer a more refined analysis (Macchiarulo et al. Citation2021).
5.2. Ground subsidence susceptibility analysis by LightGBM
In this study, ten causal factors related to ground subsidence in Shanghai were selected for GSS analysis, including factors related to topography, geology, hydrology, and human activities. They were subsequently transformed into raster layers with a resolution of 30 m × 30 m using ArcGIS. The FR model was then applied to calculate FR values for each class of the factors.
To assess model superiority, LightGBM, GBDT, and RF models were compared for efficiency and accuracy, aligning with prior research (Mohammady, Pourghasemi, and Amiri Citation2019; Rong et al. Citation2020). Results revealed LightGBM's superior performance, surpassing RF in both efficiency and accuracy. While LightGBM's accuracy and AUC were slightly higher than GBDT, its efficiency improved by over sixfold compared to GBDT. AUC corresponds to the prediction performance of a model: 0.9–1 (excellent), 0.8–0.9 (very good), 0.7–0.8 (good), 0.6–0.7 (average), and 0.5–0.6 (poor) (Huang et al. Citation2022). The AUC and accuracy of LightGBM were 0.902 and 0.817 respectively, indicating excellent model performance .
Table 7. Comparison of training efficiency and performance among RF, GBDT and LightGBM.
The GSS results revealed that three factors, distance to the river, distance to the road, and the topographic wetness index, played the most significant roles in GSS model (). Distance to river and TWI are hydrology factors related to groundwater content, while distance to road is a human activity factor related to ground load. These findings align with prior research, which has identified groundwater variations and urbanization as the primary drivers of ground subsidence in Shanghai (Xu et al. Citation2012; Citation2016). Proximity to rivers can increase the risk of ground subsidence due to factors like sediment deposition, groundwater extraction, natural processes, and soil characteristics. TWI reflects the extent of surface water accumulation, with higher TWI values indicating lower topography that is more susceptible to water accumulation, thereby increasing ground subsidence risk. Greater proximity to roads is associated with an increased likelihood of ground subsidence, primarily due to factors such as traffic-induced vibrations, road construction activities, and vehicular loads, among others.
Furthermore, Landuse, Landform, and Quaternary strata demonstrated lower contributions to the model, potentially attributed to their pre-classified nature and relatively less sophisticated data compared to other factors.
The ground subsidence susceptibility map indicated that the southeast part of Shanghai is more susceptible to subsidence, aligning with the observed subsidence patterns of PS-InSAR ground subsidence survey results.
5.3. Ground subsidence risk mapping, assessment and analysis
The subsidence intensity and susceptibility maps were integrated in ArcGIS to create a comprehensive subsidence risk map. ArcGIS provides varied useful tools for data management, analysis, and mapping.
In contrast to prior studies using area proportion and natural breakage, our study adopted susceptibility values for classification due to their direct reflection of subsidence likelihood. Susceptibility values were categorized into five groups based on direct likelihood indications. Additionally, subsidence intensity was classified into five classes based on 5 mm/y intervals, as detailed in section 3.2.4. Furthermore, it's worth noting that the classification standards for intensity and susceptibility can be modified according to specific assessment requirements.
The final subsidence risk levels were defined using a risk matrix, which categorized risks into five levels: very safe (R1), safe (R2), medium (R3), dangerous (R4), and very dangerous (R5).
The ground subsidence risk map serves as a valuable tool for analyzing ground subsidence risk patterns and identifying high-risk areas at various scales. In this study, the map reveals a distinct spatial distribution pattern of ground subsidence risk across the entire city, with R4 and R5 risk levels primarily concentrated in the southeastern region. These areas are predominantly situated within Pudong District and Fengxian District. Additionally, the map can be zoomed in to assess ground subsidence risk along specific metro lines. We validated the assessment results through on-site investigations, revealing varying degrees of ground damage in these high-risk areas ().
At the administrative district scale, most areas display R1 and R2 risk levels, suggesting relative safety. However, Songjiang, Minhang, Pudong, Jinshan, and Fengxian exhibit higher proportions of R3, R4, and R5, signaling increased subsidence risks in these regions. The risk map's versatility allows assessing subsidence risks for various objects, such as buildings, roads, and underground facilities.
5.4. Ground subsidence risk assessment and analysis of Shanghai metro network
In soft soil areas, ground subsidence risk levels R1, R2, and R3 are typically considered safe for metro operation, while R4 and R5 indicate levels of danger and very high danger. Therefore, the distribution of R4 and R5 risks along the metro network was analyzed (). The metro network has been categorized into three groups based on the proportion of R4 and R5 risks associated with each line. Group 1 (proportion of R4 and R5 less than 5%): Line 1, Line 3, Line 4, Line 6, Line 7, Line 10, Line 10 Branch, Line 11, Line 11 Branch, Line 12, Line 13, Line 14, and Line 17. Group 2 (proportion of R4 and R5 between 5% and 10%): Line 8, Line 9, and Line 15. Group 3 (proportion of R4 and R5 more than 10%): Line 2, Line 5, Line 5 Branch, Line 16, Line 18, and Pujiang Line.
Table 8. The overview and risk distributions of Shanghai Metro Lines.
In Group 1, the occurrence of R4 and R5 risks was primarily localized around specific stations and sections of these lines. Conversely, in Group 2 and Group 3, R4 and R5 risks exhibited a more widespread distribution (a). Specifically, Line 9 (from Jiuting to Songjiang South Railway Station) is situated within Songjiang District. Line 5 Branch, Line 8 (from Pujiang Town to Shendu Highway), Line 15 (from Shuangbai Road to Zizhu Hi-tech Park), and the Pujiang Line are located in Minhang District (b). Additionaly, Line 2 (from Chuansha to Pudong International Airport), Line 16 (from Longyang Road to Dishui Lake), and Line 18 (from Yuqiao to Hangtou) are located in Pudong District. Line 5 (from Dongchuan Road to Fengxian Xincheng) is located in Fengxian District (c). It is noteworthy that these four administrative districts exhibited higher proportions of R4 and R5 risk (), signifying the impact of regional ground subsidence risk on metro lines.
Figure 19. Ground subsidence risk along Shanghai Metro Lines: (a) the metro network, (b) Line 5, Line 5 Branch, Line 8, Line 9, Line 15 and Pujiang Line, (c)Line 2, Line 11, Line 13 and Line 16.

The analysis of ground subsidence risk across the metro network demonstrated that, on average, stations exhibit lower risk levels compared to intervals (a). Additionally, areas within a radius of less than 10 meters exhibited higher proportions of R4 and R5 risks (b). Furthermore, notably higher ground subsidence risk levels were observed around interchange stations such as Yuqiao, Middle Huaxia Road, Luoshan Road, Oriental Sports Center, and Shendu Highway compared with the surrounding stations.
Metro construction can disturb the surrounding soil in soft areas, leading to super pore water pressure and subsequent ground subsidence (Peck Citation1969). Lines under construction during 2016–2017, like Line 5 (Dongchuan Road to Fengxian Xincheng), Line 15, Line 18, and Pujiang line, exhibited higher subsidence risks compared to surrounding areas (b, c). This construction-related ground disturbance tends to cause continuous subsidence during the initial years of metro operation but stabilizes over time. To investigate this trend, statistical analysis of ground subsidence risk was conducted for metro lines operating before and after 31 December 2009. Group 1 represents areas of metro lines that were initially operated before 31 December 2009, while Group 2 represents areas of metro lines that began operating after this date. The proportions of the five risk levels of these two groups were calculated (). The proportions of group 1 are 73.45%, 15.24%, 5.83%, 4.40%, and 1.09%, respectively, while for group 2, they are 55.82%, 16.95%, 12.63%, 10.61%, and 3.99%, respectively. These results suggest that ground subsidence risks tend to be higher in metro lines with fewer years in operation.
The above analysis indicates that more attention should be paid to metro lines under construction, newly operating lines, intervals, interchange stations, and areas in close proximity to the metro line.
6. Conclusions
In this study, a ground subsidence assessment method combining subsidence intensity and susceptibility was proposed, and it was used to assess the ground subsidence risk of the Shanghai Metro network. The following conclusions can be drawn:
The proposed method offers remarkable efficiency and cost-effectiveness for large-scale ground subsidence assessment. The assessment results are valuable for identifying high-risk areas at different scales and providing guidance for subsequent field surveys, reducing workload, and improving overall assessment efficiency.
PS-InSAR can achieve rapid ground subsidence surveys with dense data. The results revealed more severe subsidence in districts such as Pudong, Fengxian, Jinshan, Songjiang, and Minhang.
The LightGBM model in GSS analysis showcased superior efficiency and accuracy, reflected by an AUC of 0.902 and accuracy of 0.817, highlighting its excellent performance. It identified distance to rivers, roads, and topographic wetness index as crucial factors, emphasizing susceptibility to hydrological and human activity influences.
The risk matrix application allowed a comprehensive evaluation considering both subsidence intensity and susceptibility. Ground subsidence, susceptibility, and risk exhibited distinct regional patterns, particularly in the southeastern region, consistently displaying higher levels of ground subsidence and associated risk.
The Shanghai Metro network is susceptible to regional ground subsidence risks. Prioritizing protection for areas including under-construction lines, new operational lines, intervals, interchange stations, and those near metro lines, due to heightened ground subsidence risk in these locations.
Acknowledgements
The authors sincerely thank the European Space Agency (ESA) for providing the Sentinel – 1A SAR images. The authors thank the National Aeronautics and Space Administration (NASA) for providing the DEM data. The authors thank the Shanghai Institute of Geological Survey for providing the Landform data, Quaternary strata data and faults data. The authors thank the Resource and Environmental Science and Data Center of the Chinese Academy of Sciences (CAS) for providing the Landuse data. The authors thank the anonymous reviewers for their valuable comments.
Disclosure statement
No potential conflict of interest was reported by the author(s).
Data availability statement
The data that support the findings of this study are available from the corresponding author upon reasonable request.
Additional information
Funding
References
- Abdollahi, S., H. R. Pourghasemi, G. A. Ghanbarian, and R. Safaeian. 2019. “Prioritization of Effective Factors in the Occurrence of Land Subsidence and its Susceptibility Mapping Using an SVM Model and Their Different Kernel Functions.” Bulletin of Engineering Geology and the Environment 78 (6): 4017–4034. https://doi.org/10.1007/s10064-018-1403-6.
- An, B., Y. Jiang, C. Wang, P. Shen, T. Song, C. Hu, and K. Liu. 2023. “Ground Infrastructure Monitoring in Coastal Areas Using Time-Series inSAR Technology: The Case Study of Pudong International Airport, Shanghai.” International Journal of Digital Earth 16 (1): 355–374. https://doi.org/10.1080/17538947.2023.2171144.
- Bagheri-Gavkosh, M., S. M. Hosseini, B. Ataie-Ashtiani, Y. Sohani, H. Ebrahimian, F. Morovat, and S. Ashrafi. 2021. “Land Subsidence: A Global Challenge.” Science of The Total Environment 778: 146193. https://doi.org/10.1016/j.scitotenv.2021.146193.
- Berardino, P., G. Fornaro, R. Lanari, and E. Sansosti. 2002. “A new Algorithm for Surface Deformation Monitoring Based on Small Baseline Differential SAR Interferograms.” IEEE Transactions on Geoscience and Remote Sensing 40 (11): 2375–2383. https://doi.org/10.1109/TGRS.2002.803792.
- Bianchini, S., L. Solari, M. Del Soldato, F. Raspini, R. Montalti, A. Ciampalini, and N. Casagli. 2019. “Ground Subsidence Susceptibility (GSS) Mapping in Grosseto Plain (Tuscany, Italy) Based on Satellite InSAR Data Using Frequency Ratio and Fuzzy Logic.” Remote Sensing 11 (17): 2015. https://doi.org/10.3390/rs11172015.
- Cabral-Cano, E., T. H. Dixon, F. Miralles-Wilhelm, O. Díaz-Molina, O. Sánchez-Zamora, and R. E. Carande. 2008. “Space Geodetic Imaging of Rapid Ground Subsidence in Mexico City.” Geological Society of America Bulletin 120 (11-12): 1556–1566. https://doi.org/10.1130/B26001.1.
- Cao, C., P. Xu, Y. Wang, J. Chen, L. Zheng, and C. Niu. 2016. “Flash Flood Hazard Susceptibility Mapping Using Frequency Ratio and Statistical Index Methods in Coalmine Subsidence Areas.” Sustainability 8 (9): 948. https://doi.org/10.3390/su8090948.
- Chan, Y. K., and V. Koo. 2008. “An Introduction to Synthetic Aperture Radar (SAR).” Progress In Electromagnetics Research B 2: 27–60. https://doi.org/10.2528/PIERB07110101.
- Chang, L., R. P. Dollevoet, and R. F. Hanssen. 2018. “Monitoring Line-Infrastructure with Multisensor SAR Interferometry: Products and Performance Assessment Metrics.” IEEE Journal of Selected Topics in Applied Earth Observations and Remote Sensing 11 (5): 1593–1605. https://doi.org/10.1109/TGRS.2002.803792.
- Chen, B., H. Gong, X. Li, K. Lei, L. Zhu, M. Gao, and C. Zhou. 2017. “Characterization and Causes of Land Subsidence in Beijing, China.” International Journal of Remote Sensing 38 (3): 808–826. https://doi.org/10.1080/01431161.2016.1259674.
- Chen, W., X. Xie, J. Wang, B. Pradhan, H. Hong, D. T. Bui, Z. Duan, and J. Ma. 2017. “A Comparative Study of Logistic Model Tree, Random Forest, and Classification and Regression Tree Models for Spatial Prediction of Landslide Susceptibility.” Catena 151: 147–160. https://doi.org/10.1016/j.catena.2016.11.032.
- Cigna, F., B. Osmanoğlu, E. Cabral-Cano, T. H. Dixon, J. A. Ávila-Olivera, V. H. Garduño-Monroy, C. DeMets, and S. Wdowinski. 2012. “Monitoring Land Subsidence and its Induced Geological Hazard with Synthetic Aperture Radar Interferometry: A Case Study in Morelia, Mexico.” Remote Sensing of Environment 117: 146–161. https://doi.org/10.1016/j.rse.2011.09.005.
- Cigna, F., and D. Tapete. 2021. “Present-day Land Subsidence Rates, Surface Faulting Hazard and Risk in Mexico City with 2014–2020 Sentinel-1 IW InSAR.” Remote Sensing of Environment 253: 112161. https://doi.org/10.1016/j.rse.2020.112161.
- Fadhillah, M. F., A. R. Achmad, and C.-W. Lee. 2020. “Integration of InSAR Time-Series Data and GIS to Assess Land Subsidence Along Subway Lines in the Seoul Metropolitan Area, South Korea.” Remote Sensing 12 (21): 3505. https://doi.org/10.3390/rs12213505.
- Fawcett, T. 2006. “An Introduction to ROC Analysis.” Pattern Recognition Letters 27 (8): 861–874. https://doi.org/10.1016/j.patrec.2005.10.010.
- Ferretti, A., C. Prati, and F. Rocca. 2001. “Permanent Scatterers in SAR Interferometry.” IEEE Transactions on Geoscience and Remote Sensing 39 (1): 8–20. https://doi.org/10.1109/36.898661.
- Friedman, J. H. 2001. “Greedy Function Approximation: A Gradient Boosting Machine.” Annals of Statistics 29 (5): 1189–1232.
- Gabriel, A. K., R. M. Goldstein, and H. A. Zebker. 1989. “Mapping Small Elevation Changes Over Large Areas: Differential Radar Interferometry.” Journal of Geophysical Research: Solid Earth 94 (B7): 9183–9191. https://doi.org/10.1029/JB094iB07p09183.
- Gong, S., C. Li, and S. Yang. 2009. “The Microscopic Characteristics of Shanghai Soft Clay and its Effect on Soil Body Deformation and Land Subsidence.” Environmental Geology 56 (6): 1051–1056. https://doi.org/10.1007/s00254-008-1205-4.
- Graham, L. C. 1974. “Synthetic Interferometer Radar for Topographic Mapping.” Proceedings of the IEEE 62 (6): 763–768. https://doi.org/10.1109/PROC.1974.9516.
- Huang, J., S. Ling, X. Wu, and R. Deng. 2022. “GIS-based Comparative Study of the Bayesian Network, Decision Table, Radial Basis Function Network and Stochastic Gradient Descent for the Spatial Prediction of Landslide Susceptibility.” Land 11 (3): 436. https://doi.org/10.3390/land11030436.
- Hussain, M. A., Z. Chen, Y. Zheng, M. Shoaib, J. Ma, I. Ahmad, A. Asghar, and J. Khan. 2022. “PS-InSAR Based Monitoring of Land Subsidence by Groundwater Extraction for Lahore Metropolitan City, Pakistan.” Remote Sensing 14 (16): 3950. https://doi.org/10.3390/rs14163950.
- Jin, B., K. Yin, Q. Li, L. Gui, T. Yang, B. Zhao, B. Guo, T. Zeng, and Z. Ma. 2022. “Susceptibility Analysis of Land Subsidence Along the Transmission Line in the Salt Lake Area Based on Remote Sensing Interpretation.” Remote Sensing 14 (13): 3229. https://doi.org/10.3390/rs14133229.
- Ke, G., Q. Meng, T. Finley, T. Wang, W. Chen, W. Ma, Q. Ye, and T.-Y. Liu. 2017. “Lightgbm: A Highly Efficient Gradient Boosting Decision Tree.” Advances in Neural Information Processing Systems 30), https://doi.org/10.5555/3294996.3295074.
- Kim, K., J. Kim, T.-Y. Kwak, and C.-K. Chung. 2018. “Logistic Regression Model for Sinkhole Susceptibility due to Damaged Sewer Pipes.” Natural Hazards 93 (2): 765–785. https://doi.org/10.1007/s11069-018-3323-y.
- Lee, S., and I. Park. 2013. “Application of Decision Tree Model for the Ground Subsidence Hazard Mapping Near Abandoned Underground Coal Mines.” Journal of Environmental Management 127: 166–176. https://doi.org/10.1016/j.jenvman.2013.04.010.
- Lee, S., I. Park, and J.-K. Choi. 2012. “Spatial Prediction of Ground Subsidence Susceptibility Using an Artificial Neural Network.” Environmental Management 49 (2): 347–358. https://doi.org/10.1007/s00267-011-9766-5.
- Li, D., B. Li, Y. Zhang, C. Fan, H. Xu, and X. Hou. 2022. “Spatial and Temporal Characteristics Analysis for Land Subsidence in Shanghai Coastal Reclamation Area Using PS-InSAR Method.” Frontiers in Marine Science 9: 1000523. https://doi.org/10.3389/fmars.2022.1000523.
- Li, J., L. Zhou, Z. Zhu, J. Qin, L. Xian, D. Zhang, and L. Huang. 2022. “Surface Deformation Mechanism Analysis in Shanghai Areas Based on TS-InSAR Technology.” Remote Sensing 14 (17): 4368. https://doi.org/10.3390/rs14174368.
- Lu, Z., R. Fang, H. Yao, Z. Hu, and J. Liu. 2018. “Evaluation and Analysis of the Traffic Load–Induced Settlement of Roads on Soft Subsoils with low Embankments.” International Journal of Geomechanics 18 (6): 04018043. https://doi.org/10.1061/(ASCE)GM.1943-5622.0001123.
- Macchiarulo, V., P. Milillo, C. Blenkinsopp, C. Reale, and G. Giardina. 2021. “Multi-temporal InSAR for Transport Infrastructure Monitoring: Recent Trends and Challenges.” Proceedings of the Institution of Civil Engineers-Bridge Engineering 176 (2): 92–117. https://doi.org/10.1680/jbren.21.00039.
- Malik, K., D. Kumar, D. Perissin, and B. Pradhan. 2022. “Estimation of Ground Subsidence of New Delhi, India Using PS-InSAR Technique and Multi-Sensor Radar Data.” Advances in Space Research 69 (4): 1863–1882. https://doi.org/10.1016/j.asr.2021.08.032.
- Manap, M. A., H. Nampak, B. Pradhan, S. Lee, W. N. A. Sulaiman, and M. F. Ramli. 2014. “Application of Probabilistic-Based Frequency Ratio Model in Groundwater Potential Mapping Using Remote Sensing Data and GIS.” Arabian Journal of Geosciences 7 (2): 711–724. https://doi.org/10.1007/s12517-012-0795-z.
- Minderhoud, P., L. Coumou, L. Erban, H. Middelkoop, E. Stouthamer, and E. Addink. 2018. “The Relation Between Land use and Subsidence in the Vietnamese Mekong Delta.” Science of The Total Environment 634: 715–726. https://doi.org/10.1016/j.scitotenv.2018.03.372.
- Mohammady, M., H. R. Pourghasemi, and M. Amiri. 2019. “Land Subsidence Susceptibility Assessment Using Random Forest Machine Learning Algorithm.” Environmental Earth Sciences 78 (1): 1–12. https://doi.org/10.1007/s12665-019-8518-3.
- Motagh, M., Y. Djamour, T. R. Walter, H.-U. Wetzel, J. Zschau, and S. Arabi. 2007. “Land Subsidence in Mashhad Valley, Northeast Iran: Results from InSAR, Levelling and GPS.” Geophysical Journal International 168 (2): 518–526. https://doi.org/10.1111/j.1365-246X.2006.03246.x.
- Peck, B.. 1969. “Deep Excavation and Tunnelling in Soft Ground.” 7th International Conference on Soil Mechanics and Foundation Engineering (Mexico) 4: 225–290.
- Reinders, K. J., R. F. Hanssen, F. J. van Leijen, and M. Korff. 2021. “Augmented Satellite InSAR for Assessing Short-Term and Long-Term Surface Deformation due to Shield Tunnelling.” Tunnelling and Underground Space Technology 110: 103745. https://doi.org/10.1016/j.tust.2020.103745.
- Rong, G., S. Alu, K. Li, Y. Su, J. Zhang, Y. Zhang, and T. Li. 2020. “Rainfall Induced Landslide Susceptibility Mapping Based on Bayesian Optimized Random Forest and Gradient Boosting Decision Tree Models—A Case Study of Shuicheng County, China.” Water 12 (11): 3066. https://doi.org/10.3390/w12113066.
- Sachdeva, S., and B. Kumar. 2021. “Comparison of Gradient Boosted Decision Trees and Random Forest for Groundwater Potential Mapping in Dholpur (Rajasthan), India.” Stochastic Environmental Research and Risk Assessment 35 (2): 287–306. https://doi.org/10.1007/s00477-020-01891-0.
- Sato, C., M. Haga, and J. Nishino. 2006. “Land Subsidence and Groundwater Management in Tokyo.” International Review for Environmental Strategies 6 (2): 403–424.
- Shen, S., H. Wu, Y. Cui, and Z. Yin. 2014. “Long-term Settlement Behaviour of Metro Tunnels in the Soft Deposits of Shanghai.” Tunnelling and Underground Space Technology 40: 309–323. https://doi.org/10.1016/j.tust.2013.10.013.
- Shen, S., and Y. Xu. 2011. “Numerical Evaluation of Land Subsidence Induced by Groundwater Pumping in Shanghai.” Canadian Geotechnical Journal 48 (9): 1378–1392. https://doi.org/10.1139/t11-049.
- Shi, X., Y. Xue, J. Wu, S. Ye, Y. Zhang, Z. Wei, and J. Yu. 2008. “Characterization of Regional Land Subsidence in Yangtze Delta, China: The Example of Su-Xi-Chang Area and the City of Shanghai.” Hydrogeology Journal 16 (3): 593–607. https://doi.org/10.1007/s10040-007-0237-2.
- Sousa, J. J., A. M. Ruiz, R. F. Hanssen, L. Bastos, A. J. Gil, J. Galindo-Zaldívar, and C. S. de Galdeano. 2010. “PS-InSAR Processing Methodologies in the Detection of Field Surface Deformation—Study of the Granada Basin (Central Betic Cordilleras, Southern Spain).” Journal of Geodynamics 49 (3-4): 181–189. https://doi.org/10.1016/j.jog.2009.12.002.
- Tan, Y., H. Zhu, F. Peng, K. Karlsrud, and B. Wei. 2017. “Characterization of Semi-top-Down Excavation for Subway Station in Shanghai Soft Ground.” Tunnelling and Underground Space Technology 68: 244–261. https://doi.org/10.1016/j.tust.2017.05.028.
- Tien Bui, D., H. Shahabi, A. Shirzadi, K. Chapi, B. Pradhan, W. Chen, K. Khosravi, M. Panahi, B. Bin Ahmad, and L. Saro. 2018. “Land Subsidence Susceptibility Mapping in South Korea Using Machine Learning Algorithms.” Sensors 18 (8): 2464. https://doi.org/10.3390/s18082464.
- Tomiyama, N., K. Koike, and M. Omura. 2004. “Detection of Topographic Changes Associated with Volcanic Activities of Mt. Hossho Using D-InSAR.” Advances in Space Research 33 (3): 279–283. https://doi.org/10.1016/S0273-1177(03)00483-6.
- Wang, H., G. Feng, B. Xu, Y. Yu, Z. Li, Y. Du, and J. Zhu. 2017. “Deriving Spatio-Temporal Development of Ground Subsidence due to Subway Construction and Operation in Delta Regions with PS-InSAR Data: A Case Study in Guangzhou, China.” Remote Sensing 9 (10): 1004. https://doi.org/10.3390/rs9101004.
- Wang, R., M. Yang, T. Yang, J. Lin, and M. Liao. 2022. “Decomposing and Mapping Different Scales of Land Subsidence Over Shanghai with X-and C-Band SAR Data Stacks.” International Journal of Digital Earth 15 (1): 478–502. https://doi.org/10.1080/17538947.2022.2036835.
- Wei, Z. 2010. Urban Geological Atlas of Shanghai. Beijing: Geological Publishing House.(in chinese)
- Wu, H., S. Shen, and J. Yang. 2017. “Identification of Tunnel Settlement Caused by Land Subsidence in Soft Deposit of Shanghai.” Journal of Performance of Constructed Facilities 31 (6): 04017092. https://doi.org/10.1061/(ASCE)CF.1943-5509.0001082.
- Xie, Z., G. Chen, X. Meng, Y. Zhang, L. Qiao, and L. Tan. 2017. “A Comparative Study of Landslide Susceptibility Mapping Using Weight of Evidence, Logistic Regression and Support Vector Machine and Evaluated by SBAS-InSAR Monitoring: Zhouqu to Wudu Segment in Bailong River Basin, China.” Environmental Earth Sciences 76 (1): 1–19. https://doi.org/10.1007/s12665-017-6640-7.
- Xu, S., Y. Chen, and C. Zhao. 2008. “One-dimensional Consolidation Tests of Creep Deformation and Secondary Consolidation Characteristics of Soft Soils in Shanghai Area.” Journal of Engineering Geology 16 (4): 495–501.
- Xu, Y., L. Ma, Y. Du, and S. Shen. 2012. “Analysis of Urbanisation-Induced Land Subsidence in Shanghai.” Natural Hazards 63 (2): 1255–1267. https://doi.org/10.1007/s11069-012-0220-7.
- Xu, Y., S. Shen, and Y. Du. 2009. “Geological and Hydrogeological Environment in Shanghai with Geohazards to Construction and Maintenance of Infrastructures.” Engineering Geology 109 (3-4): 241–254. https://doi.org/10.1016/j.enggeo.2009.08.009.
- Xu, Y., S. Shen, D. Ren, and H. Wu. 2016. “Analysis of Factors in Land Subsidence in Shanghai: A View Based on a Strategic Environmental Assessment.” Sustainability 8 (6): 573. https://doi.org/10.3390/su8060573.
- Xue, Y., Y. Zhang, S. Ye, J. Wu, and Q. Li. 2005. “Land Subsidence in China.” Environmental Geology 48 (6): 713–720. https://doi.org/10.1007/s00254-005-0010-6.
- Yang, Y., Q. Chen, Q. Xu, Y. Zhang, Q. Yong, and G. Liu. 2017. “Coseismic Surface Deformation of the 2014 Napa Earthquake Mapped by Sentinel-1A SAR and Accuracy Assessment with COSMO-SkyMed and GPS Data as Cross Validation.” International Journal of Digital Earth 10 (12): 1197–1213. https://doi.org/10.1080/17538947.2017.1299806.