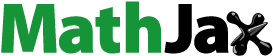
ABSTRACT
In the past decades, the environment has changed drastically in China with rapid economic development. Lack of data, especially data with spatiotemporal information, has however hindered evaluating progress of environmental indicators in the global Sustainable Development Goal (SDG) framework. Here, we evaluate and explore geospatial information of 20 environmental SDG indicators from six SDGs in China from 2010 to 2020 using big data. We show China has improved rapidly in most of the 20 indicators, except for indicator 13.2.2 (CO2 emission). 63.5% of China’s land mass showed improved states for the SDG indicators. By 2020, four of the indicators were found to have achieved their 2030 targets. It is predicted from the existing data that 10 of the 20 indicators can achieve their targets smoothly by 2030, but two indicators will lag behind. We quantitatively show China’s progress towards the environmental SDG indicators, and put forward timelines and suggestions for policy makers.
1. Introduction
The Sustainable Development Goals (SDGs) are at the core of ‘Transforming our world: the 2030 Agenda for Sustainable Development’ adopted by the 193 Member States of the United Nations (UN) in September 2015. These SDGs represent the commitment to address tri-dimensional social, economic, and environmental issues facing humankind to achieve sustainable development. The achievement of the SDGs requires monitoring and evaluation (Castro, Fernández, and Colsa Citation2021). Nearly half of the time has passed since the Agenda was adopted in 2015 yet gaps remain in measuring and monitoring the progress of SDG indicators at national and global levels (Schmidt-Traub et al. Citation2017; Xu Citation2020; Mastrángelo Citation2019; Huan et al. Citation2021) About 41% of the total 230 + indicators have no available data as at December 2022 (IAEG-SDG Citation2022) Furthermore, even countries with available data only have a small portion of data over time and space (UN Citation2021).
In the current SDG framework, China lacks an objective evaluation of progress and gaps. The rapid urbanization and industrialization of China over the past decades have generally promoted economic and social issues, but also have put great pressure on the environment. Meanwhile, China has taken positive measures in recent years to restore and improve the environment (Lu Citation2020; Wang et al. Citation2021b). Therefore, nature and the built environment may have undergone drastic changes and need frequent observation and evaluation. Most of the existing studies have examined environmental changes in China using local indicators and standards (Xu Citation2020; Wang et al. Citation2021b; Wang et al. Citation2020a; Jiang et al., Citation2022) but it is difficult to judge the distance to the targets of the 2030 Agenda. Therefore, there is an urgent need to objectively evaluate China’s environmental SDG indicators to aid scientific research and decision-makers.
Methodologies for data collection for the SDG indicators are developed and implemented by national statistical agencies, UN, and other organizations across the world (Huan et al. Citation2021; Mulholland, Dimitrova, and Hametner Citation2018) However, their implementation and use also face several important challenges. The main problem is that the process is time-consuming and updates are infrequent (Sachs Citation2020; Burke et al. Citation2021) The integration of geospatial and statistical information is also urgently needed for policymakers and the public to understand and respond to spatial imbalances and changes over different time horizons (UN Citation2020; UN Citation2021; Scown Citation2020).
Satellite observations have great potential to provide national and global scale environmental data for the SDG indicators (Burke et al. Citation2021; GEO et al. Citation2017; Anderson Citation2017; ESA Citation2018; Estoque Citation2020; Persello et al. Citation2022). It provides repeatable, long-term, and low-cost data. Satellite observations could contribute significantly to monitoring six SDGs (UN Citation2021), namely SDG 2 Zero hunger (Whitcraft et al. Citation2019), SDG 6 Clean water and sanitation (Mariathasan, Bezuidenhoudt, and Olympio Citation2019; Mulligan et al. Citation2020), SDG 11 Sustainable cities and communities (Aquilino et al. Citation2020), SDG 13 Climate action (Boesch et al. Citation2021), SDG 14 Life below water (Wang Citation2021a) and SDG 15 Life on land (Prince Citation2019; Mondal, McDermid, and Qadir Citation2020). In most cases, satellite data need other auxiliary statistical and basic geographic data, and often field observations for verification, to be translated into indicators (Whitcraft et al. Citation2019; Andries et al. Citation2019; Kavvada Citation2020; Chen et al. Citation2020).
This study is a novel attempt at evaluating the progress of the environmental SDG indicators using big data at the national scale. We combine data ( and Table S1) from satellite observations, field surveys, statistics, navigation, and basic geography (i.e. terrain, population density, and boundary), collectively known as Big Earth Data (Guo Citation2018; Guo Citation2019; Guo et al. Citation2021). Big Earth Data was employed to evaluate SDG indicators in the annual reports of Big Earth Data in support of Sustainable Development Goals since 2019, focusing on 6 SDGs including SDG 2, 6, 11, 13, 14, 15. However, in these reports indicators are usually calculated and analyzed one by one, and here it is a new attempt to integrate different indicators together with spatial–temporal information. The study makes three contributions: first, the values, states, and trends of environmental SDG indicators were newly evaluated or updated. Second, compared with traditional national or provincial level statistics, grid cell information was provided to better understand the nature and characteristics of the SDG indicators. Third, the state of the SDG indicators in 2030 was predicted using current data, which provides an opportunity to adjust work and policy according to real-time progress.
2. Data and method
The methods, data sources, standards, and results for all 20 environmental SDG indicators are displayed in and . These data sources include remote sensing, statistics, surveying, and field observation. In most cases, we use different data sources to make cross-validations. The employed methods are mainly developed from publications or UN’s definition of the indicators to ensure data production quality. Selection, normalization, and spatialization of the indicator data are presented below.
Table 1. Data and methods of the evaluated indicators.
Table 2. Results and standards of the evaluated indicators.
2.1. Indicator selection
From the global indicator framework, two factors were considered in the selection of indicators for the environment. Firstly, indicators should effectively reflect the changes in the environment, covering the natural environment such as water, air, soil, and biology, as well as the built environment such as agriculture, residence, and transportation. There is also climate change, which has important impacts on the whole environment. Secondly, the data available for the indicators should have abundant spatial and temporal information. Many environmental indicators also happen to be the areas where satellite observation-based Big Earth Data can play an important role in data acquisition. Therefore, we try to select indicators that can be observed by satellite.
Using Big Earth Data, 20 SDG indicators that are closely related to the natural and built environment were selected and evaluated within the 2010–2020 period (). We matched the evaluation standards of the SDG indicators with their 2030 targets (Methods), which gave a more objective evaluation of the state and distance to each SDG. The score for each SDG indicator was normalized based on thresholds ranging from 0 (worst performance) to 100 (fully achieved) (Xu Citation2020; Sachs et al. Citation2021). An SDG indicator was classified as being very close to or having achieved the 2030 target if the score was no less than 90 ().
Table 3. Progress of the evaluated 20 environmental SDG indicators in China during the 2010-2020 period.
2.2. Normalization of the selected indicators
To make the data comparable across indicators, each indicator was rescaled from 0 to 100 with 0 denoting the worst performance and 100 describing the optimal performance. The equation for normalization is given as:
where x’ is the normalized score, x is the value of the indicators, min(x) is the lower bound, and max(x) is the upper bound.
The bound settings refer to the following standards. (1) Global indicator framework has clear targets and definitions, such as zero growth in land degradation; the Sendai Framework for Disaster Reduction 2015-2030; (2) The targets were suggested by UN or international agencies, such as PM2.5 concentration, wetland change, etc. (3) The limits of 0 and 100 were defined for some of the indicators measured by percentages. (4) The target was defined using the average value of a few top countries (such as top 5%) in the indicator ranking (excluding the extreme value of 2.5%) that was taken from international agencies’ databases or the Sustainable Development Solutions Network (Sachs et al. Citation2021). More details for the bounds and sources are listed in Table S2.
2.3. Definition of the states and trends of the indicators
An indicator was classified as being very close to or having achieved the 2030 target (score ≥ 90), showing a small gap to the targets (90 > score ≥ 70), facing a big gap to the targets (70 > score ≥ 50), and facing a huge gap the targets (score < 50).
Two periods were considered to monitor the change in indicators: 2010-2015 and 2015-2020. The results in for the change in indicators were divided according to three situations, including rising values (improvement), stable values, and declining values (deterioration). Among them, indicator results were labeled as ‘improving’ if the score of the indicator increased greater than 2. Moreover, the indicator’s progress was defined as ‘deteriorating’ if the decrease was greater than 2, and ‘stable’ if the range was between ±2.
2.4. Generation of grid cell map of the indicators
The grid cell score maps were generated based on the following steps. (1) The indicators acquired from satellite imagery with spatial information for thematic maps were normalized and resampled to 1 km resolution. (2) The indicators acquired from statistics or survey data included indicators 6.4.1 (water use efficiency), 6.5.1 (water resource management), 11.5.1 (loss in disasters), and 11.5.2 (economic loss in disaster). These indicators were examined at the urban or provincial scale and projected to a 1 km resolution map of the province or city. (3) Indicators that did not have sufficient data in 2010, 2015, or 2020 were linearly interpolated from data in the neighboring period. (4) All of the available indicator score maps were overlaid together, and the value of each grid was the average score of the corresponding indicator. The weighted value for each indicator was based on the density of population, considering the relationship of different indicators with human activity. Indicators 14.1.1 and 15.5.1 were not included in the final map because the spatial information was only available for one year.
2.5 Prediction for 2030
Whether the 2030 Agenda can be achieved is a matter of common concern (UN Citation2022). We predicted 2030 values for the indicators. The prediction for 2030 was divided into three categories according to different indicators. First, the four indicators that had already achieved their targets of 2030 in 2020 and had a consistent trend were not subjected to predictions for 2030; Second, there was an attempt to use different curves or lines to find the best fit and prediction expression for the indicators with sufficient annual observation data for the 2010–2020 period. In the third category, the indicators with only two or three observations were predicted using a linear method.
3. Results
3.1. State and progress of the 20 environmental SDG indicators
Most of the measured 20 SDG indicators improved during the 2010-2020 period in China albeit at different rates (). Indicator 11.3.1 (land use efficiency) decreases during 2010–2015 due to fast spatial expansion coupled with slowdown in population in urban areas. There has been a notable decrease in the areal extent of new urban land since 2015, reversing the trend of the indicator. Indicator 13.2.2 (CO2 emission) first declined and then remained stable during this period. Especially from 2015 to 2020, in the first five years after the implementation of the 2030 Agenda, none of the 20 SDG indicators deteriorated.
By 2020, four of the SDG indicators, including indicator 11.2.1 (public transport access), 11.5.2 (economic loss from natural disasters), 15.3.1 (land degradation), and 15.4.2 (Mountain Green Cover Index (MGCI)), were found to be very close to or had achieved their 2030 targets value. Although the average MGCI in China was 82.05% in 2020, the indicator’s target was still considered close or achieved. This is because the values in the western provinces of China were much lower than other provinces since a large proportion of mountains were higher than 4000 m above sea level and many were covered by glaciers and snow. Therefore, based on our results and spatial information, we believe that the value of 82.05% approaches the limit of the indicator’s value for China. In addition, indicators 11.5.1 and 13.1.1 (human loss from natural disasters) also reached their 2030 targets based on their 2020 values. However, the realization standards for these two SDG indicators need to measure the average values during the period 2020–2030 according to the Sendai Framework for Disaster Reduction (UNDRR Citation2015) because the annual values for the indicators often fluctuate greatly. Therefore, they were only considered to have temporarily realized their 2030 targets in 2020 in this study.
As of 2020, three SDG indicators, i.e. indicators 6.4.1 (water-use efficiency), 11.4.1 (per capita expenditure on heritage), and 15.5.1 (Red List Index (RLI)) have constantly improved, but there were still huge gaps to reach their 2030 targets.
3.2. Spatial imbalance of SDG indicators
Spatial information helps to understand natural differences (Liu Citation2021). The SDG indicators measured by different data sources have different resolutions (), which introduces data interoperability challenges. In order to improve the presentation and evaluation, the 20 measured SDG indicators were integrated into a score and normalized (a) to a unified 1 km resolution map for the years 2010, 2015, and 2020.
Figure 1. Spatial differences and score maps of China. a, sketch map of the method of integrating different SDG indicator’s value into a score. b-d, score map in 2010 (b), 2015 (c) and 2020 (d).

It can be observed from b-d that in northwest China the scores were generally very low in 2010, 2015, and 2020. In particular, for the areas in the rectangle, there were mainly desert or Gobi Desert areas, which were the main sources of sand and dust with very high concentrations of PM10 and PM2.5. In addition, there were also arid high-altitude mountains with very low MGCI values.
In China’s middle and east (the three ellipses), the improvement of scores in the period 2015–2020 was more pronounced than in the period 2010–2015. The challenge of CO2 emissions increased in the highly populated regions within the three ellipses, but the per capita CO2 emissions may not increase due to the growing population in urban regions. Meanwhile, urban infrastructure in terms of public transport, disaster resistance, and land/water use efficiency improved, and PM2.5 concentration decreased, so the scores in these eastern regions improved significantly. The remaining scattered red points in the ellipse in (d) were mainly lakes, which means that the water clarity in these regions improved at a slow rate and challenges remain to reach the target corresponding to the indicator.
Changes in the scores during the 20102020 period are shown in . In 63.5% of China’s land area, the score improved, for 26% it was stable, and in the remaining 10.5%, the score deteriorated (a). To better interpret the results, the score map is combined with a land cover classification map (Liu and Zhang Citation2019) (b). Land cover is roughly classified into five area types: built up area (city and town), cropland, vegetation (forest and grassland), water (water and wetland), and extreme environment area (desert, Gobi desert, glacier, and multi-year snow). Based on the combined maps, scores improved for 77.3% of the built up area, 71.3% of the water area, 68.9% of the vegetation area, 67.9% of the cropland areas, and 48.1% of the extreme environment area. Scores deteriorated less than 10% for built up area, water area, vegetation area, and cropland area, respectively, but deteriorated for 20.75% of the extreme environment areas. The remaining areas were stable. Even if extreme environment areas were excluded, it can be seen that the improvement in north China still lags behind that in the south (b). There were more improvements of built up land, cropland, and vegetation areas, but less improvement in water areas in south China than in the north.
Figure 2. Score change maps during the 2010–2020 period on grid and provincial level. a, Score changes map on grid level. b, Score changes on different land cover types including built up land, cropland, vegetation, water, and extreme environment area. Score changes for extreme environment area are excluded in the map. c, Score ranks for the 31 provinces (Hong Kong, Macao, and Taiwan are not included because of incomplete data).

We ranked 31 provinces (including the four province-level municipalities of Beijing, Shanghai, Tianjin, and Chongqing. Hong Kong, Macao, and Taiwan are not included because of incomplete data) based on their averaged SDG indicator scores (c). The extreme environment area was excluded in the calculation of the score for each province. The three eastern provinces Beijing, Zhejiang, and Guangdong ranked in the top. Beijing and Zhejiang scored more than 80 in 2020, which means that the average scores of these provinces are not far from achieving their 2030 targets on the whole for the 20 environmental SDG indicators. The three northern provinces of Jilin, Inner Mongolia, and Xinjiang scored lower than 60 and were ranked as the furthest in achieving their 2030 targets. Inner Mongolia and Xinjiang lagged behind while scores for indicators 6.4.1 (water-use efficiency), 11.7.1 (public space), and 13.2.2 (per capita CO2 emission) were very low. Jilin scored low on indicators 6.4.1 and 11.7.1, but higher on indicator 13.2.2 than the other two provinces. Jilin was also the province that showed the slowest improvement during the 2010-2020 period, which caused the province to lag behind in 2020, although it was ahead of more than half of the other provinces in 2015. Most other provinces scored in the 60–80 range in 2020 and made significant improvements during the 2010–2020 period.
3.3. Predicted state in 2030
We have predicted 2030 values for the 20 environmental SDG indicators based on a trend analysis of existing monitoring data ().
Figure 3. Trend analysis of the observed environmental indicators during the 2010–2020 period and the predictions for 2030. (a) trend analysis and prediction of indicators which have annual measurements; (b) linear analysis of indicators which only have 2 or 3 observations. The arrows in (a) and (b) represent indicator trends, and the translucent areas in (a) are the prediction zones.

The predicted states of the eight indicators were compared with predictions from other literature or national plans (). The predicted data included the GDP, population, and CO2 emissions in 2030 obtained from scientific research. The national plans included long-term planning for agriculture, land use, and environmental protection for 2030, and they contained future goals and government action projects, so they were also good references for our results. The results show that the average absolute score deviation between our work and other predictions was 2.6, which means they agreed very closely. The most significant difference is PM2.5 concentration, and our prediction is more optimistic (the score is 7 points higher) than the government’s planning.
Table 4. Comparison of our results with other predictions and the national plans for 2030.
3.4. Suggestions for decision-making
Based on the calculations and the predictions discussed, the average score values of the 20 evaluated indicators for 2015, 2020, and 2030 are respectively: 63.5, 74.3, and 85.6 (), respectively. At the current rate, 10 of the 20 environmental SDG indicators are expected to achieve their 2030 targets. There are two indicators that have a huge gap to their 2030 targets, indicator 13.2.2 (per capita CO2 emissions), and indicator 15.5.1 RLI (score around 60). China has pledged to reach peak CO2 emissions before 2030, which means that indicator 13.2.2 will not improve much before that time. Global biodiversity is facing serious threats due to climate change and human activity (Cowie, Bouchet, and Fontaine Citation2022), and indicator 15.5.1 will be difficult to improve greatly in a short period. Above all, these two indicators will lag behind in 2030 and will need long-term attention from policy makers.
Figure 4. Observed and predicted states of the 20 indicators. a,b, observed states of indicators in 2015 (a) and 2020 (b). c, predicted state in 2030. The red line in (a-c) is the average score for the year.

Aside from the ten SDG indicators that are likely to reach their 2030 targets and the two indicators that are lagging behind (13.2.2 and 15.5.1), there are two SDG indicators close to achieving their 2030 targets based on our predictions: indicator 14.1.1 (floating debris) will score 86, and indicator 6.5.1 (water resource management) will score 87. Some non-governmental organizations (NGOs) in China have established a net plastic action network in 2019 to achieve zero marine plastic waste emissions by 2030. The progress of indicator 14.1.1 may be accelerated and achieved if this action can be effectively implemented. The other six indicators, including indicators 2.4.1 (sustainable agriculture), 6.3.2 (water clarity), 6.4.1 (water use efficiency), 11.6.2 (PM 2.5 concentration), 11.4.1 (per capita expenditure on heritage) and 11.7.1 (open public space), will feature scores ranging between 70 and 80 in 2030 based on our predictions. These indicators require special attention from decision-makers since they are challenging but not impossible to achieve if more ambitious policies are developed.
It should be noted that our predictions were only based on indicator trends from the 2010-2020 period, and other factors including the COVID-19 pandemic, climate change, and policy changes were not considered. The COVID-19 pandemic may have temporary effects on economic development and GHG emissions but will not change long-term trends (UNEP Citation2020). Climate change is a real long-term challenge to human sustainable development (Cheng Citation2020; WEF Citation2021). It will bring higher uncertainties to indicators 11.5.1 (loss from natural disasters) and 11.5.2 (economic loss from natural disasters), which are directly related to natural disasters, and will gradually influence many other indicators (Nerini Citation2019).
The 20 indicators were divided into four groups according to their progress to better interpret the observed and predicted results, and different suggestions and timelines for action were provided in . The first group (in the red box of ) consists of the indicators that will lag far behind and have little chance of achieving their 2030 targets but have a chance to achieve them during the 2040-2050 period based on our predictions. The second group (in the orange box) consists of indicators that face great challenges in achieving their 2030 targets. The third group (in the yellow box) represents indicators that only have a small distance left and are very likely to achieve their 2030 targets if a little more effort is made. The fourth group (in the green box) represents indicators that will smoothly achieve their 2030 targets if they maintain their current progress. The classification of different groups can help decision-makers know which indicators need urgent action and which need long-term planning.
4. Discussions
It is very important to obtain SDG indicator data that has temporal and spatial information. On the one hand, these data can help us recognize the spatial imbalance in sustainable development. On the other hand, it helps to recognize the inconsistent steps that hamper progress. This also helps us gain a better understanding of the factors that limit the reliability of some of the indicators, such as the mountain green cover index mentioned earlier that could be biased by high-mountain areas. Another example of an SDG indicator which needs deep understanding is indicator 14.7.1 (sustainable fisheries). The value of this indicator has decreased in recent years, but it is not easy to judge whether it is a good or bad trend in the case of China, which is the world’s largest fish producer. Using satellite observations, the area of aquaculture ponds in coastal waters was found to decrease from 1.07 × 104 km2 in 2015 to 0.94 × 104 km2 in 2018, and then further decrease to 0.9 × 104 km2 in 2020 (). The decrease in the area and density of aquaculture ponds was the result of offshore protection policies, which are beneficial for improving the marine environment. Therefore, we considered the decrease in proportion as a good and sustainable trend for indicator 14.7.1 from the perspective of environmental protection. However, the applicability of our standard elsewhere requires further testing, particularly in the context of small islands and developing countries that rely on fishing.
Another problem when evaluating the SDG indicators is how to set the 2030 targets. The setting of targets will directly influence judgements of the indicators’ status and may bring uncertainties for evaluation. Only a few of the selected 20 SDG indicators have quantifiable targets in the global indicator framework. In this work, many indicators refer to recommendations by international organizations or to the state of a few countries at the top of the indicator ranking in 2015 (Sachs et al. Citation2021; OECD Citation2022). Some of the indicators that use top country values do not have strict 2030 targets but only consist of relative evaluations between countries. It is difficult for most developing countries, not just China, to reach the level of developed or high-income countries for indicators involving money or expenditures, such as SDG indicators 6.4.1 (water use efficiency) and 11.4.1 (per capita expenditure on heritage). Therefore, using a change rate of the country itself for these indicators, such as setting a target of doubling efficiency or investment in 2030 compared to 2015, may be fairer for developing countries. However, it may then become too strict for developed countries where indicator values are already very high. Unfortunately, there is no such research or reference that can be used to make a widely accepted target at present. Another example of an indicator that needs a better 2030 target is indicator 11.3.1 (land use efficiency). This indicator promotes a well-planned compact urban environment, where lower values indicate a higher land use efficiency that is more friendly to the natural environment, and the 2030 target for the indicator is suggested to be lower than one (UN-Habitat Citation2018; Estoque et al. Citation2021). However, cities that are too compact can also produce problems for the living environment, so it is difficult to judge sustainable urbanization using only this indicator (UN-Habitat Citation2018).
The uncertainty for the employed method and data includes three factors. The first factor is the evaluation accuracy of each indicator. We try to use the methods recognized by UN, or the published papers with given accuracy to reduce the uncertainty. The second factor is the comparison of different provinces. The comparison among different provinces adopts the commonly used method of average weight of each indicator in this work, but we also found that the average weight is not fair for provinces of different types and characteristics. At the provincial level, take the highly urbanized coastal Shanghai and the highly natural inland Tibet as an example, how to compare their sustainable development level is a big challenge. This is also a problem for comparison among different countries worldwide. Using the spatial information, the land cover ratio can be considered as a weight in SDGs scoring and sorting in future work. The third factor comes from the interpolation and prediction on temporal scale. Some of the indicators are observed every five years, so there are not many available observation data during 2010-2020. The limited frequency of observation may lead to bias in interpolation and prediction, especially the indicators which may change severely.
Above all, as the year 2030 approaches, we need some adjustments for the SDG framework. Based on our work, we have two suggestions for future work. (1) Use more big data in SDG progress evaluation. Big data can not only be used in indicator state assessment but also provide much more detailed spatial–temporal information than statistics for SDG evaluation, which is very beneficial for decision making. (2) Set more reasonable and quantitative targets for each SDG indicator, taking into full consideration of the country-specific status (developed, developing) and conditions is urgently needed to judge whether the goals of the 2030 Agenda could be achieved.
5. Summary
At present, the data used for SDG indicator evaluation is still very scarce and in urgent need, and Big Earth Data is an effective means to solve this problem. This study evaluated the changing trend and mapped the spatial imbalance of 20 environmental SDG indicators in China using Big Earth Data, and provided suggestions on how to achieve the targets of the 2030 Agenda step by step for decision-makers.
Using the data of SDG indicators calculated from remote sensing, navigation, statistics, surveying et al. it is found that China’s environmental SDG indicators have made great progress in the first trimester of the 2030 Agenda (2015-2020), but still face great challenges. Four SDG indicators have already achieved their targets by 2020. From the current state and recent changing trend, it is also predicted the state of the indicators in 2030. The indicators are divided into four groups, including two indicators being lagged far behind, six indicators facing great challenge, two indicators being close to achieve the 2030 targets, and 10 indicators that will achieve the targets. Different suggestions and timelines for action were provided for the four types.
The spatiotemporal information of Big Earth Data has unique advantages in SDG index evaluation, imbalance, and change trend analysis, and will play a greater role in the future. Lessons learned from the mapping, evaluation, and prediction of SDG progress in China can be used and extended for the benefit of SDG implementation knowledge and practice in other countries in the world.
Author contributions
H.G. and J.L. designed and led the study. L.H., Y.C., Z.S., X.L., S.L., L.Z., and F.W worked on the data acquisition, processing and analysis. L.H and Y.C designed the figures. I.N., Z.X., Q.L., L.L., M.W., Y. C. contributed to interpretation of the results. All of the authors led the writing.
Competing interests
The authors declare no competing interests.
Acknowledgments
This work is supported by the Strategic Priority Research Program of the Chinese Academy of Sciences (grant number XDA19090000 and XDA19090122)
Disclosure statement
No potential conflict of interest was reported by the author(s).
Data availability statement
The data that support the findings of this study are available from the corresponding author upon request.
Correction Statement
This article has been corrected with minor changes. These changes do not impact the academic content of the article.
Additional information
Funding
Notes
1 1 USD≈6.2 RMB in 2017, 1 USD≈6.7 RMB in 2017, 1 USD≈6.6 RMB in 2018.
2 1 PPP USD≈4.2 RMB in 2017.
References
- Agamuthu, P., S. Mehran, A. Norkhairah, and A. Norkhairiyah. 2019. “Marine Debris: A Review of Impacts and Global Initiatives.” Waste Management & Research 37 (10): 987–1002. doi: 10.1177/0734242X19845041
- Anderson, K. 2017. “Earth Observation in Service of the 2030 Agenda for Sustainable Development.” Geo-spatial Information Science 20: 77–96. doi: 10.1080/10095020.2017.1333230
- Andries, A., S. Morse, R. Murphy, J. Lynch, E. Woolliams, and J. Fonweban. 2019. “Translation of Earth Observation Data Into Sustainable Development Indicators: An Analytical Framework.” Sustainable Development 27: 366–376. doi: 10.1002/sd.1908
- Aquilino, M., C. Tarantino, M. Adamo, and A. Barbanente. 2020. “Earth Observation for the Implementation of Sustainable Development Goal 11 Indicators at Local Scale Monitoring of the Migrant Population Distribution.” Remote Sensing 12: 950. https://doi.org/10.3390/rs12060950.
- Bian, J., A. Li, G. Lei, Z. Zhang, and X. Nan. 2020. “Global High-Resolution Mountain Green Cover Index Mapping Based on Landsat Images and Google Earth Engine.” ISPRS Journal of Photogrammetry and Remote Sensing 162: 63–76. doi: 10.1016/j.isprsjprs.2020.02.011
- Boesch, H., Y. Liu, J. Tamminen, D. Yang, P. I. Palmer and H. Lindqvist. 2021. “Monitoring Greenhouse Gases from Space.” Remote Sensing 13: 2700. https://doi.org/10.3390/rs13142700.
- Burke, M., A. Driscoll, D. Lobell, and L. Ermon. 2021. “Using Satellite Imagery to Understand and Promote Sustainable Development.” Science 371: 1219. https://doi.org/10.1126/science.abe8628.
- Cai, J. N., H. Huang, and P. S. Leung. 2019. Understanding and Measuring the Contribution of Aquaculture and Fisheries to Gross Domestic Product (GDP). FAO Fisheries and Aquaculture Technical Paper No. 606. Rome, FAO. 80 pp. Licence: CC BY-NC-SA 3.0 IGO.
- Castro, G., M. Fernández, and Á Colsa. 2021. “Unleashing the Convergence Amid Digitalization and Sustainability Towards Pursuing the Sustainable Development Goals (SDGs): A Holistic Review.” Journal of Cleaner Production 280: 122204. doi: 10.1016/j.jclepro.2020.122204
- Center for International Earth Science Information Network (CIESIN), Columbia University. 2020. Gridded Population of the World, Version 4 (GPWv4): Population Density, Revision 11. Palisades NY: NASA Socioeconomic Data and Applications Center (SEDAC). Accessed 26 January 2022.
- Chen, Jun, Shu Peng, Hao Chen, Xuesheng Zhao, Yuejing Ge, and Zhilin Li. 2020. “A Comprehensive Measurement of Progress Toward Local SDGs with Geospatial Information: Methodology and Lessons Learned.” ISPRS Geo-Spatial Information Science 9: 522. doi: 10.3390/ijgi9090522
- Chen, G., X. Wang, and K. Ma. 2020. “Red List of China's Forest Ecosystems: A Conservation Assessment and Protected Area Gap Analysis.” Biological Conservation 248: 108636. doi: 10.1016/j.biocon.2020.108636
- Cheng, H. 2020. “Future Earth and Sustainable Developments.” The Innovation 1: 100055. doi: 10.1016/j.xinn.2020.100055
- Cowie, R. H., P. Bouchet, and B. Fontaine. 2022. “The Sixth Mass Extinction: Fact, Fiction or Speculation?” Biological Reviews 97: 640–663. https://doi.org/10.1111/brv.12816.
- Crippa, M., D. Guizzardi, E. Solazzo, M. Muntean, E. Schaaf, F. Monforti-Ferrario and M. Banja. 2021. Emissions Database for Global Atmospheric Research, version v6.0_FT_2020 (GHG time-series). European Commission, Joint Research Centre (JRC) [Dataset] PID: http://data.europa.eu/89h/2f134209-21d9-4b42-871c-58c3bdcfb549.
- ESA. 2018. “Satellite Earth Observations in Support of the Sustainable Development Goals.” Edited by Paganini, M., Petiteville, I.
- Estoque, R. C. 2020. “A Review of the Sustainability Concept and the State of SDG Monitoring Using Remote Sensing.” Remote Sensing 12: 1770. doi: 10.3390/rs12111770
- Estoque, R. C., M. Ooba, T. Togawa, Y. Hijioka, and Y. Murayama. 2021. “Monitoring Global Land-Use Efficiency in the Context of the UN 2030 Agenda for Sustainable Development.” Habitat International 115: 102403. doi: 10.1016/j.habitatint.2021.102403
- FAO. 2020. “The State of World Fisheries and Aquaculture 2020: Sustainability in Action.” Rome 2–36. https://doi.org/10.4060/ca9229en.
- GEMI. 2019. Step-by-step monitoring methodology for SDG Indicator 6.4.1.
- Group on Earth Observations (GEO). 2017. “Earth Observations in Support of the 2030 Agenda for Sustainable Development.” Japan Aerospace Exploration Agency (JAXA) on Behalf of GEO under the EO4SDG Initiative, 1–19. http://ceos.org/ourwork/ad-hoc-teams/sustainable-development-goals/.
- Guo, H. 2018. “Steps to the Digital Silk Road.” Nature 554: 25–27. doi: 10.1038/d41586-018-01303-y
- Guo, H. 2019. “From Digital Earth to Big Earth Data: Accelerating Scientific Discovery and Supporting Global Sustainable Development.” International Journal of Digital Earth 12: 1–1. doi: 10.1080/17538947.2018.1559481
- Guo, H., F. Chen, Z. Sun, J. Liu, and D. Liang. 2021. “Big Earth Data: A Practice of Sustainability Science to Achieve the Sustainable Development Goals.” Science Bulletin 66: 1050–1053. doi: 10.1016/j.scib.2021.01.012
- Huan, Y., T. Liang, H. Li, and C. Zhang. 2021. “A System Method for Assessing Progress of Achieving Sustainable Development Goals: A Case Study of 15 Countries.” Science of Total Environment 752: 141875. doi: 10.1016/j.scitotenv.2020.141875
- Inter-agency and Expert Group on SDG Indicators (IAEG-SDG). 2022. “Tier Classification for Global SDG Indicators.” https://unstats.un.org/sdgs/iaeg-sdgs/tier-classification/.
- Jia, M. M., Z. M. Wang and Y. Z. Zhang. 2018. “Monitoring Loss and Recovery of Mangrove Forests During 42 Years: The Achievements of Mangrove Conservation in China.” International Journal of Applied Earth Observation and Geoinformation 73: 535–545. https://doi.org/10.1016/j.jag.2018.07.025.
- Jiang, Q., Z. Li, S. Qu, Y. Cui, H. Zhang, and Z. Xu. 2022. “High-resolution map of China’s Sustainability.” Resources, Conservation and Recycling 178: 106092. https://doi.org/10.1016/j.resconrec.2021.106092.
- Kavvada, A. 2020. “Towards Delivering on the Sustainable Development Goals Using Earth Observations.” Remote Sensing of Environment 247: 111930. doi: 10.1016/j.rse.2020.111930
- Liu, Y. 2021. “Evenness is Important in Assessing Progress Towards Sustainable Development Goals.” National Science Review 8. https://doi.org/10.1093/nsr/nwaa238.
- Liu, D., H. Duan and S. Loiselle. 2020. “Observations of Water Transparency in China’s Lakes from Space.” International Journal of Applied Earth Observation and Geoinformation 92: 102187. doi: 10.1016/j.jag.2020.102187
- Liu, L., and X. Zhang. 2019. “Global 30 m Land-Cover Products with Fine Classification System in 2015.” https://doi.org/10.5281/zenodo.3986872. http://hdl.pid21.cn/21.86109/casearth.5d904b7a0887 164a5c7f bfa0.
- Lu, X. 2020. “Progress of air Pollution Control in China and Its Challenges and Opportunities in the Ecological Civilization ear.” Engineering 6: 1423–1431. https://doi.org/10.1016/j.eng.2020.03.014.
- Mao, D. H., Z. M. Wang and B. J. Du. 2020. “National Wetland Mapping in China: A New Product Resulting from Object-Based and Hierarchical Classification of Landsat 8 OLI Images.” ISPRS Journal of Photogrammetry and Remote Sensing 164: 11–25. doi: 10.1016/j.isprsjprs.2020.03.020
- Mariathasan, V., E. Bezuidenhoudt, and K. R. Olympio. 2019. “Evaluation of Earth Observation Solutions for Namibia's SDG Monitoring System.” Remote Sensing 11: 1612. doi: 10.3390/rs11131612
- Mastrángelo, M.. 2019. “Key Knowledge Gaps to Achieve Global Sustainability Goals.” Nature Sustainability 2: 1115–1121. https://doi.org/10.1038/s41893-019-0412-1.
- Mondal, P., S. S. McDermid, and A. Qadir. 2020. “A Reporting Framework for Sustainable Development Goal 15 Multi-Scale Monitoring of Forest Degradation Using MODIS, Landsat and Sentinel Data.” Remote Sensing of Environment 237: 111592. doi: 10.1016/j.rse.2019.111592
- Mulholland, E., A. Dimitrova, and M. Hametner. 2018. “SDG Indicators and Monitoring: Systems and Processes at the Global, European, and National Level.” ESDN Quarterly Report 48, Vienna.
- Mulligan, M., A. van Soesbergen, D. G. Hole, T. M. Brooks, S. Burke, and J. Hutton. 2020. “Mapping Nature's Contribution to SDG 6 and Implications for Other SDGs at Policy Relevant Scales.” Remote Sensing of Environment 239: 111671. https://doi.org/10.1016/j.rse.2020.111671.
- Neil, C. S., R. E. Jacqueline and J. N. Glenn. 2019. “Developing Good Practice Guidance for Estimating Land Degradation in the Context of the United Nations Sustainable Development Goals.” Environmental Science & Policy 92: 349–355. https://doi.org/10.1016/j.envsci.2018.10.014.
- Nerini, F. 2019. “Connecting Climate Action with Other Sustainable Goals.” Nature Sustainability 2: 674–680. doi: 10.1038/s41893-019-0334-y
- Oda, T., and S. Maksyutov. 2015. ODIAC Fossil Fuel CO2 Emissions Dataset (Version name: ODIAC2020b), Center for Global Environmental Research, National Institute for Environmental Studies, (Reference date: 2021/10/28).
- OECD. 2022. “The Short and Winding Road to 2030:Measuring Distance to the SDG Targets.” https://www.oecd.org/wise/the-short-and-winding-road-to-2030-af4b630d-en.htm.
- Persello, C., J. D. Wegner, R. Hänsch, D. Tuia, P. Ghamisi, M. Koeva, and G. Camps-Valls. 2022. “Deep Leaning and Earth Observation to Support the Sustainable Development Goals.” IEEE Geoscience and Remote Sensing Magazine 10: 172–200. https://doi.org/10.1109/MGRS.2021.3136100.
- Prince, S. D. 2019. “Challenges for Remote Sensing of the Sustainable Development Goal SDG 15.3.1 Productivity Indicator.” Remote Sensing of Environment 234: 111428. https://doi.org/10.1016/j.rse.2019.111428.
- Sachs, J. 2020. Sustainable Development Report 2020. Cambridge: Cambridge University Press.
- Sachs, J., C. Kroll, G. Lafortune, G. Fuller, and F. Woelm. 2021. Sustainable Development Report 2021. Cambridge: Cambridge University Press.
- Schmidt-Traub, G., C. Kroll, K. Teksoz, D. Durand-Delacre, and J. Sachs. 2017. “National Baselines for the Sustainable Development Goals Assessed in the SDG Index and Dashboards.” Nature Geoscience 10: 547–555. doi: 10.1038/ngeo2985
- Scown, M. W. 2020. “The Sustainable Development Goals Need Geoscience.” Nature Geoscience 13: 714–715. doi: 10.1038/s41561-020-00652-6
- Sun, Z., Y. Liu, and Y. Yu. 2019. “China's Carbon Emission Peak Pre-2030: Exploring Multiscenario Optimal low-Carbon Behaviors for China's Regions.” Journal of Cleaner Production 231. https://doi.org/10.1016/j.jclepro.2019.05.159.
- UN. 2021. The Sustainable Development Goals Report 2021.
- UN. 2022. The Sustainable Development Goals Report 2022.
- UNEP. 2020a. Emissions Gap Report 2020.
- UNEP. 2020b. Progress on Integrated Water Resources Management. Global Baseline for SDG 6 Indicator 6.5.1: Degree of IWRM Implementation. https://www.unwater.org/ publications/progress-on-integrated-water-resources.
- UNESCO-UIS. 2021. Tracking Investment to Safeguard the World’s Cultural and Natural Heritage—Results of the 2020 UIS Survey on Expenditure on Cultural and Natural Heritage (SDG Indicator 11.4.1). http://uis.unesco.org/sites/default/files/documents/uis_culture_and_heritage_report_ 2021_web.pdf.
- UN-Habitat. 2018. “SDG Indicator 11.3.1 Training Module: Land Use Efficiency.” United Nations Human Settlement Programme (UN-Habitat), Nairobi.
- United Nations Office for Disaster Risk Reduction (UNDRR). 2015. The Sendai Framework for Disaster Risk Reduction 2015-2030. https://www.preventionweb.net/files/resolutions /N1516716.pdf (2015).
- United Nations (UN). 2020. The Sustainable Development Goals Report 2020.
- Waltham, N. J., M. Elliott, S. Y. Lee, C. Lovelock, C. M. Duarte, C. Buelow, C. Simenstad, I. Nagelkerken, L. Claassens and C. K.-C. Wen 2020. “Decade on Ecosystem Restoration 2021–2030—What Chance for Success in Restoring Coastal Ecosystems?” Frontiers in Marine Science 7: 71. https://doi.org/10.3389/fmars.2020.00071.
- Wang, Y., Y. Lu, G. He, C. Wang, J. Yuan, and X. Cao. 2020a. “Spatial Variability of Sustainable Development Goals in China: A Provincial Level Evaluation.” Environmental Development 35: 100483. https://doi.org/10.1016/j.envdev.2019.100483.
- Wang, P., Y. Ma, X. Zhao, B. Wang, J. Wang, and F. Gao. 2020b. “Regional Differences and Influential Factors of Open Public Space in Chinese Cities Based on Big Earth Data.” Sustainability 12: 2514. https://doi.org/10.3390/su12062514.
- Wang, X., H. Ren, P. Wang, R. Yang, L. Luo, and F. Cheng. 2018. “A Preliminary Study on Target 11.4 for UN Sustainable Development Goals.” International Journal of Geoheritage and Parks 6: 18–24. doi: 10.17149/ijgp.j.issn.2577.4441.2018.02.002
- Wang, X., X Xiao, X Xu, and Z Zou 2021a. “Rebound in China’s Coastal Wetlands Following Conservation and Restoration.” Nature Sustainability 4: 1076–1083. https://doi.org/10.1038/s41893-021-00793-5.
- Wang, K., B. Zhao, L. Ding, and Z. Miao. 2021b. “Government Intervention, Market Development, and Pollution Emission Efficiency: Evidence from China.” Science of Total Environment 757: 143738. doi: 10.1016/j.scitotenv.2020.143738
- Wetlands International. 2021. Wetlands International welcomes the 2030 Action Targets and calls for a focus on wetlands. https://www.wetlands.org/news/wetlands-international-welcomes-the-2030-action-targe ts-and-calls-for-a-focus-on-wetlands/.
- Whitcraft, A. K., I. Becker-Reshef, C. O. Justice, L. Gifford, A. Kavvada, and I. Jarvis. 2019. “No Pixel Left Behind: Toward Integrating Earth Observations for Agriculture Into the United Nations Sustainable Development Goals Framework.” Remote Sensing of Environment 235: 111470. doi: 10.1016/j.rse.2019.111470
- World Economic Forum (WEF). 2021. The Global Risks Report 2021, 16th Edition.
- World Health Organization (WHO). 2021. Ambient (outdoor) air pollution. https://www.who.int/news-room/fact-sheets/detail/ambient-(outdoor)-air-quality-and-health
- Xu, Z. 2020. “Assessing Progress Towards Sustainable Development Over Space and Time.” Nature 57: 74–78. doi: 10.1038/s41586-019-1846-3
- Zeng, Q. L., J. Tao and F. Chen 2020. “Estimating Ground-Level Particulate Matter in Five Regions of China Using Aerosol Optical Depth.” Remote Sensing 12 (5): 881. https://doi.org/10.3390/rs12050881.
- Zhou, C. C., X. Liu, W. Wang, et al. 2016. “Assessment of Marine Debris in Beaches or Seawaters Around the China Seas and Coastal Provinces.” Waste Management 48: 652–660. doi: 10.1016/j.wasman.2015.11.010
- Zuo, L. J., Z. X. Zhang, K. M. Carlson, et al. 2018. “Progress Towards Sustainable Intensification in China Challenged by Land-Use Change.” Nature Sustainability 1: 304–313. https://doi.org/10.1038/s41893-018-0076-2.