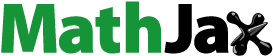
ABSTRACT
Land surface emissivity (LSE) represents an essential parameter for understanding land change and surface energy balance. While numerous algorithms have been proposed to retrieve LSE, they are seldom applied to remote sensing data with high spatial resolution. The SDGSAT-1 satellite provides an opportunity to retrieve LSE with high spatial resolution that can be regarded as references for validating LSE products generated from coarse-spatial-resolution satellite data. However, no study has yet explored LSE-retrieval based on SDGSAT-1. This research seeks to fill this gap through evaluating the radiometric calibration accuracy of SDGSAT-1, using five methods to retrieve pixel-level emissivity of Guangzhou, and comparing such results with emissivity generated from other satellites (e.g. Landsat 8, ECOSTRESS, and MODIS) that transited Guangzhou during the similar period. Our results indicated that SDGSAT-1 performed well in radiometric calibration. Heterogeneous vegetation and man-made surfaces registered significant emissivity differences in varying spatial resolutions, whereas relatively homogeneous water bodies registered smaller differences. Decreasing spatial resolution amplifies the emissivity differences of the same land cover among distinctive sensors. The five emissivity-retrieval methods performed exceptionally well based on SDGSAT-1, registering average absolute differences less than 0.026 as compared with Landsat 8; with decreasing spatial resolutions, the maximum difference between SDGSAT-1 and MODIS LSE product reached 0.124.
Nomenclature
LST | = | land surface temperature |
RS | = | remote sensing |
RTE | = | radiative transfer equation |
LSE | = | land surface emissivity |
SEM | = | semi-empirical method |
TES | = | temperature-emissivity-separated |
PBM | = | physically based method |
NDVI | = | normalized difference vegetation index |
VNIR | = | visual and near-infrared |
TIR | = | thermal infrared |
MIR | = | mid-infrared |
NBEM | = | NDVI-based emissivity method |
SDGSAT-1 | = | Sustainable Development Science Satellite 1 |
VIHM | = | vegetation index mixing model |
= | NDVI threshold method | |
= | vegetation coverage | |
= | retrieved LSE | |
= | emissivity of pure vegetation, bare soil, and buildings | |
= | topographic correction parameter | |
= | NDVI corresponding to pure bare soil pixel and pure vegetation pixel | |
= | temperature ratio of pure vegetation, buildings, and bare soil | |
= | at-sensor long-wave radiance | |
= | at-sensor brightness temperature | |
= | central wavelength | |
= | reflectance values in the red and near-infrared bands | |
= | emissivity retrieved based on SDGSAT-1 (band 2) | |
= | emissivity retrieved based on Landsat 8 (band 10) | |
= | emissivity generated from ECOSTRESS product (band 4) | |
= | emissivity generated from MODIS product (band 31) | |
UAV | = | Unmanned aerial vehicle |
1. Introduction
Land surface temperatures (LSTs), as a critical parameter for understanding regional-scale surface energy balance and water cycle, provide valuable information on the variations of a given thermal environment over time and space (Li et al. Citation2023; Liang et al. Citation2019). With advances in information technology, LST applications have increased significantly in different fields such as assessments of urban thermal environments (Siddiqui et al. Citation2021; Chang et al. Citation2022; Xia et al. Citation2022), estimations of evapotranspiration (Jiang, Tang, and Li Citation2022; Zhou et al. Citation2023; Olivera-Guerra et al. Citation2017), and monitoring of thermal disasters (Mohseni et al. Citation2021; Yu et al. Citation2022; Li et al. Citation2021). To accurately retrieve LSTs from multimodal remote sensing (RS) data, numerous algorithms have been proposed based on sensor characteristics (Ru et al. Citation2023; Wang et al. Citation2019; Wigneron et al. Citation2017). These algorithms are classified into single-channel (Wang et al. Citation2015; Cristóbal et al. Citation2018; Wang et al. Citation2018), split-window (Jimenez-Munoz et al. Citation2014; Zarei et al. Citation2021; Li et al. Citation2023), and multi-channel (Zheng et al. Citation2022; Chen et al. Citation2021; Pu and Bonafoni Citation2023) approaches. Accurate LST retrieval requires obtaining complex parameters within radiative transfer equations (RTEs), with emissivity playing a crucial role.
Land surface emissivity (LSE) represents an inherent characteristic of both natural and man-made materials, determined by properties such as water content (Hulley, Hook, and Baldridge Citation2010; Zhong et al. Citation2022), roughness (Brodu et al. Citation2014) and temperature (Coll et al. Citation2019). LSE is widely used for differentiating material compositions (Baldridge et al. Citation2008; Heiden et al. Citation2007) and for observing land changes (Hulley et al. Citation2015). Besides, it is a crucial parameter used in estimating LSTs (Chakraborty et al. Citation2021), with evidence indicating that even minute errors in LSE (as low as 1%) can result in substantial errors (ranging from 0.4–1.0 K) when retrieving LSTs under different atmospheric conditions (Chen et al. Citation2016; Li et al. Citation2012). Thus, it is essential to obtain precise LSE values to ensure accurate LST retrieval. Although LSE of continuous land covers is effectively retrieved from RS data (Dash et al. Citation2002; Jiang, Li, and Nerry Citation2006), different observational conditions, including band setting and resolution of sensors (Salisbury and D'Aria Citation1992), angles (Rüdisser et al. Citation2022), and even weather conditions (Chakraborty et al. Citation2021), can affect the retrieved LSE. Moreover, differences in retrieval algorithms can significantly impact the accuracy of the retrieved LSE under the same observational conditions.
At present, commonly-used LSE-retrieval algorithms can be divided into three categories: multi-channel temperature-emissivity-separated (TES) methods; physically based methods (PBMs) (Li et al. Citation2013b); and semi-empirical methods (SEMs). Multi-channel TES methods and PBMs retrieve LSE based on physically reasonable assumptions or constraints on RTEs, mainly applied to data with two or more mid-infrared (MIR) and TIR channels (Li et al. Citation2012). SEMs rely on either classification-based lookup tables (Snyder et al. Citation2010; Peres and DaCamara Citation2004; Sun and Pinker Citation2003) or statistical correlations between spectral indices and LSE, such as the normalized difference vegetation index (NDVI) from visual and near-infrared (VNIR) bands and the LSE in the thermal infrared (TIR) band (Neinavaz, Skidmore, and Darvishzadeh Citation2020; Van De Griend and Owe Citation2012; Valor and Caselles Citation1996; Sobrino et al. Citation2008). Of these three modalities, SEMs demonstrate advantages in terms of easy operation and broad applicability, as they are insensitive to complex atmospheric parameters and require little prior knowledge or experimental data. To compute pixelwise LSE, researchers have adopted existing NDVI-based emissivity methods (NBEMs) for specific needs in their individual studies (Sobrino et al. Citation2008; Yu, Guo, and Wu Citation2014; Li and Jiang Citation2018; Jimenez-Munoz et al. Citation2014). However, the accuracy of NBEMs is affected by observational conditions and land-cover heterogeneity. Therefore, high-quality RS data is critical to the effectiveness of NBEMs. Generally, VNIR data with less cloud contamination and higher spatial resolution (up to 30 meters, such as Landsat OLI) are optimal choices for NBEMs. Nonetheless, to retrieve precise LSE over highly heterogeneous urban surfaces, VNIR data with even higher spatial resolutions are warranted.
The launch of the Sustainable Development Science Satellite 1 (SDGSAT-1) signifies an efficient means to achieve sustainable development goals related to the environment, socioeconomic development, and human activities. SDGSAT-1 (www.sdgsat.ac.cn) uses three sensors to synergistically observe regions, including a multispectral imager with a spatial resolution of 10 m, a TIR spectrometer with a spatial resolution of 30 m, and a glimmer imager with spatial resolutions of 10 m for the Panchromatic band and 40 m for the RGB bands. Nonetheless, its potential in monitoring and assessing thermal environmental indicators remains unexplored.
Against the background, this study initially examined the radiometric accuracy of multispectral and TIR data from SDGSAT-1 via comparison with calibrated Landsat 8 data. Based on SDGSAT-1 and Landsat 8 data, representative NBEMs were utilized to derive LSE estimations of urban surfaces, which were then demonstrated and compared. Moreover, emissivity values retrieved from SDGSAT-1 were compared with existing LSE products generated from RS data with varying spatial resolutions (i.e. ECOSTRESS and MODIS). Based on the outcomes of these comparisons, this study further analyzed not only emissivity derived from different retrieval methods for the same land cover, but also emissivity derived from the same retrieval method for the same land cover under different spatial resolutions.
2. Existing NDVI-based emissivity methods (NBEMs)
NBEMs are commonly classified into three types, the Van de Griend and Owe method (Van De Griend and Owe Citation2012); vegetation index mixing model (VIHM) (Valor and Caselles Citation1996); and NDVI threshold method () (Sobrino et al. Citation2008). The Van de Griend and Owe method is suitable for vegetation surfaces with NDVI values ranging from 0.157–0.727. However, it cannot be applied to urban surfaces with negative NDVI values, such as bare land, rocks, buildings, roads, and water bodies, and thus has not been considered in this study. While VIHM and
, numerous studies have improved the original versions based on different research objects and data sources (Qin, Karnieli, and Berliner Citation2010; Yu, Guo, and Wu Citation2014; Cristóbal et al. Citation2018). Both the VIHM and
have been utilized for LSE retrieval in this paper, as discussed below.
2.1. Vegetation index hybrid model (VIHM)
Inspired by the work of Van de Griend and Owe, Valor and Caselle proposed the VIHM to estimate effective emissivity while considering the cavity effect (Valor and Caselles Citation1996). Depending on the NDVI range, the VIHM defines pixels as pure bare soil, vegetation, or mixed land covers. The calculation of LSE involves an area weighting of each component within the pixel (EquationEq. 1(1)
(1) ). Vegetation coverage (
), determined by the NDVI (EquationEq. 2
(2)
(2) ), is used as a commonly-recognized area-weighting factor. A
closer to 1.0 signifies a purer vegetation pixel whereas a
closer to 0 signifies a purer bare-soil pixel.
(1)
(1) where
is the retrieved LSE;
and
respectively represent the emissivity of pure vegetation and that of bare soil (
= 0.985,
= 0.960 (Rubio, Caselles, and Badenas Citation1997)); and
is the topographic correction parameter and can be calculated from
:
.
(2)
(2) where
and
separately signify NDVI corresponding to pure bare soil pixel and to pure vegetation pixel, which are usually taken as 0.2 and 0.5, respectively (Wang et al. Citation2022).
Valor and Caselle's VIHM approach is effective in retrieving emissivity values from natural surfaces. However, the challenge arises from addressing more complex urban surfaces due to their combination of both natural and man-made components. To better characterize emissivity values for such surfaces consisting primarily of buildings and vegetation, efforts have been underway to improve the original VIHM by proposing a hybrid model (Qin, Karnieli, and Berliner Citation2010). This model defines surface properties based on different ranges. Pixels with
values less than or equal to 0.5 are categorized as artificial surfaces, and their emissivity values are calculated through EquationEq. 3
(3)
(3) . Conversely, pixels with
values greater than 0.5 are categorized as natural surfaces, and their emissivity values are calculated through EquationEq. 4
(4)
(4) . Water is considered homogeneous and assigned a fixed emissivity value of 0.995.
(3)
(3)
(4)
(4) where
,
and
respectively refer to the emissivity of pure vegetation, buildings, and bare soil (
= 0.986,
= 0.970,
= 0.972);
,
and
respectively indicate the temperature ratio of pure vegetation, buildings and bare soil, which are related to the
and are calculated as:
,
,
; and
is also the topographic correction parameter and can be determined by
:
.
2.2. NDVI threshold methods (
)
Evidence have suggested that, while vegetation emissivity exhibits a strong correlation with , non-vegetation emissivity exhibits a stronger correlation with reflectance in short-wave bands such as the red band. Accordingly, many researchers have made enhancements to the VIHM, thereby creating a series of
for the respective emissivity-retrieval for vegetated and non-vegetated surfaces. This study employed several representative
that have demonstrated successful application alongside Landsat 8 data. The classification of pixels was threefold: pixels with NDVI less than 0.20 were regarded as pure non-vegetated surfaces (i.e. man-made surfaces), including buildings, roads, and bare soil; those with NDVI greater than 0.50 were regarded as pure vegetated surfaces; and those with NDVI between 0.20 and 0.50 were regarded as mixed surfaces. Based on visual interpretation, the above values of NDVI thresholds were reasonable and could more accurately delineate vegetated and non-vegetated surfaces in this study. Then, the emissivity values of urban surfaces were retrieved according to the empirical formulas () of reflectance and emissivity corresponding to different NDVI ranges.
Table 1. Expressions of common .
3. Methodology
3.1. Site
As the hub of the Pearl River Delta region, Guangzhou () is located in southern China (22.26°∼23.56°N,112.92°∼114.32°E). The city registers a subtropical monsoon climate characterized by warm, humid, and rainy conditions throughout the year. Given its level terrain, well-developed river network, and expansive water areas, Guangzhou has emerged as one of the largest cities in China by population size, urban area, and gross domestic product. However, rapid urbanization in Guangzhou has compromised its thermal environment, leading to elevated temperatures, and pronounced urban heat island effects in recent years (Chen et al. Citation2023; Hu et al. Citation2019).
3.2. Data preprocessing
To minimize the influences of observational conditions on emissivity retrieval, we selected winter data from periods with clear and stable weather. This decision was made considering that optical images in southern China tend to be contaminated by clouds during summer months with high rainfall. Thus, the multispectral and TIR data () obtained from SDGSAT-1, which passed over Guangzhou at 10:14 am on December 2022 were downloaded.
Table 2. Information of SDGSAT-1, Landsat 8, ECOSTRESS and MODIS used in this study.
Given that SDGSAT-1 Level 4 data have already undergone geometric correction, radiometric calibration of the multispectral and TIR data was done based on the calibration coefficients, thus enabling us to obtain orthophotos of at-sensor short-wave radiance and long-wave radiance. Next, the ENVI FLAASH module was used to perform atmospheric correction of at-sensor short-wave radiance, thus enabling us to obtain reflectance orthophotos. Additionally, the at-sensor long-wave radiance () was converted into brightness temperature (
) using the Planck's formula (EquationEq. 5
(5)
(5) ).
(5)
(5) where
is the central wavelength of the TIR band,
,
Of note, the process of calibration differs between Landsat 8 and SDGSAT-1 sensors. Calibration coefficients of sensors can shift at different times due to aging components or a changing space environment, thus warranting continuous tracking of load performances to update such coefficients. To achieve consistency and comparability of data collected at different times and locations, Landsat 8 operates by regularly recording readings from internal stable radiation sources (e.g. black bodies and white plates), which are then used to calibrate its sensor in real time. Conversely, SDGSAT-1 does not have an onboard calibration system and instead conducts absolute radiometric calibration through annual site calibrations. Given its ideal calibration accuracy, Landsat 8 offers data that serve as a reference to evaluate the calibration performance of SDGSAT-1 in advance, ensuring reliability and accuracy of subsequent retrieval results.
In this study, the Landsat 8 multispectral and TIR data () with a similar transit period were used as the reference, Radiometric and atmospheric calibrations were conducted on the Landsat 8 to obtain orthophotos of reflectance and at-sensor brightness temperature. To maintain the original spectral characteristics of the raw data and accelerate data processing, the nearest-neighbor method was used to resample (Zhou, Jing, and Jiang Citation2003) the SDGSAT-1 data (10 m) to match the Landsat 8 data (30 m). Then, 10000 samples of representative and pure land covers were randomly extracted from orthophotos of both reflectance and at-sensor brightness temperature based on SDGSAT-1 and Landsat 8. Considering differences in the band settings of different sensors, samples of both reflectance and at-sensor brightness temperature were normalized (EquationEq. 6(6)
(6) ). To evaluate the calibration performance of SDGSAT-1 data, correlation analyses were further conducted between the normalized reflectance and brightness temperature.
(6)
(6) where
denotes the normalized values of the retrieved variables,
denotes the original values of the retrieved variables,
and
respectively denote the minimum and the maximum values of the original retrieved variables.
To conduct a more detailed analysis of the retrieved emissivity data from SDGSAT-1, except for emissivity retrieved from Landsat 8, LSE products from ECOSTRESS and MODIS () that transited Guangzhou during a similar period and under similar meteorological conditions were also downloaded (Hu et al. Citation2023; Wan, Hook, and Hulley Citation2015). Moreover, to enable cross-comparisons, the following retrieved variables (e.g. NDVI, LSE) were also normalized (EquationEq. 6(6)
(6) ) due to differences in band configurations.
Since the central wavelength of SDGSAT-1 TIR B2 approximated that of Landsat 8 TIR B10, the five NBEMs (i.e. methods proposed by Valor, Li, Jordi, Yu, and Qin) previously used to retrieve LSE from Landsat 8 TIR B10 were employed in this paper. Firstly, NDVI (EquationEq. 7(7)
(7) ) values were calculated based on reflectance orthophotos of both SDGSAT-1 and Landsat 8 as an input for the five NBEMs; differences in the retrieved emissivity between SDGSAT-1 and Landsat 8 were then counted. Besides, SDGSAT-1 data (10 m) were also resampled to match the spatial resolutions of ECOSTRESS (70 m) and MODIS (1000 m) through the nearest-neighbor method. Emissivity values from SDGSAT-1 were also compared with the corresponding LSE products generated from ECOSTRESS and MODIS (Hook and Hulley Citation2019). For each land cover, differences in the retrieved emissivity were then computed.
(7)
(7) where
and
respectively denote the reflectance values in the red and near-infrared bands.
4. Results
4.1. Comparison of calibration between SDGSAT-1 and Landsat 8
Based on typical land covers (i.e. roads, tree covers, grasslands, croplands, buildings, barren and sparse vegetations, and water bodies) of Guangzhou (Li, He, and Zhang Citation2023) (), the correlation between SDGSAT-1 and Landsat 8 in terms of normalized reflectance in four bands (i.e. VNIR bands) and brightness temperature in the TIR band was assessed ().
Figure 3. Scatterplot on the correlation analysis between SDGSAT-1 and Landsat 8: (a) normalized reflectance in the blue band, (b) normalized reflectance in the green band, (c) normalized reflectance in the red band, (d) normalized reflectance in the near-infrared band, (e) normalized brightness temperature in the TIR band.

The resultant correlation coefficients between SDGSAT-1 and Landsat 8 for both normalized reflectance and brightness temperature were higher than 0.90, indicating a strong positive correlation and consistency between these two datasets. This statistical finding suggested that the observational performance and calibration of the two satellites in the corresponding bands were close to each other. Besides, it suggested that the radiometric calibrations of SDGSAT-1 multispectral and TIR sensors had been performed accurately.
In terms of the reflectance, Landsat 8 exhibited a lower trend than SDGSAT-1, as illustrated by the scatterplots. This suggested that Landsat 8 was limited by the spatial resolution, with most of its pixels being mixed with various types of land covers. Contrarily, SDGSAT-1 was equipped with a higher spatial resolution and thus could better distinguish complex types of land covers and their corresponding properties. This phenomenon was especially significant for urban surfaces with intermixing of buildings and vegetation. Besides, different bands were affected by the mixing effect to varying extents. Bands more sensitive to the information of vegetation with high discrete distribution in the city (e.g. green, red, and near-infrared bands) would be more substantially affected by the mixing effect. In this context, SDGSAT-1 with its higher spatial resolution could better differentiate the vegetation types and details of their distributions, whereas Landsat 8 with its lower resolution and corresponding mixed pixels would average out these subtle differences, contributing to the latter’s lower reflectance in these bands.
In terms of brightness temperature, SDGSAT-1 exhibited a lower trend than Landsat 8 due to different observational conditions caused by varying transit times, as illustrated by the scatterplots. It should be recognized that brightness temperature was governed by surface temperatures and atmospheric radiance, which were determined by solar radiation. In this context, such radiation was greater during the transit of Landsat 8 than that of SDGSAT-1. Accordingly, those urban surfaces covered mainly by metallic roofs composed of materials that could heat up rapidly contributed to escalating surface temperatures, leading to slightly higher brightness temperature as measured by Landsat 8. Additionally, there is a small amount of noise was noted in the image of brightness temperature, and corresponding points deviated from numerous highly-clustered points.
4.2. LSE retrieved from five NBEMs based on SDGSAT-1
Orthophotos of LSE retrieved based on SDGSAT-1 through the five NBEMs are shown in , alongside the corresponding emissivity values of each land cover.
Figure 4. Orthophotos of Guangzhou LSE and average emissivity values of each land cover retrieved based on: (a) original VIHM proposed by (Valor and Caselles Citation1996), (b) improved hybrid model (Qin, Karnieli, and Berliner Citation2010), (c) of (Cristóbal et al. Citation2018), (d)
of Yu (|Yu, Guo, and Wu Citation2014);, (e)
of Li (Li and Jiang Citation2018).

For distinctive land covers, the emissivity values retrieved by five algorithms differed significantly, with the smallest emissivity difference (0.015) being generated from the VIHM by Valor and the largest (0.057) from the method by Li. Regardless of the method used, vegetation was characterized by a higher emissivity value. Except for Qin’s method, the four NBEMs yielded higher average emissivity values for vegetation, especially for tree covers (Valor: 0.9867, Jordi: 0.9898, Yu: 0.9885, Li: 0.9853). These four methods yielded lower average emissivity values for built-up areas, especially for buildings (Valor: 0.9760, Jordi: 0.9779, Yu: 0.9730, Li: 0.9734). Qin's method considered spectral differences between urban and natural surfaces in its modeling, resulting in lower values of retrieved emissivity for vegetation (0.977) and built-up areas (0.964) than the other four NBEMs. Retrieval results for water bodies exhibited significant discrepancies, with the highest value (0.979) being generated from the method by Qin and the lowest (0.975) from the VIHM by Valor.
4.3. Differences in LSE between SDGSAT-1 and Landsat 8
Differences in the normalized emissivity between resampled SDGSAT-1 and Landsat 8 are shown as .
Figure 5. Distribution of emissivity differences () based on: (a) original VIHM proposed by (Valor and Caselles Citation1996), (b) improved hybrid model (Qin, Karnieli, and Berliner Citation2010), (c)
of (Cristóbal et al. Citation2018), (d)
of (Yu, Guo, and Wu Citation2014);, (e)
of (Li and Jiang Citation2018).

Emissivity values of more than half of the pixels of Landsat 8 were lower than those of SDGSAT-1, whereas 99.95% of the pixel-level differences fluctuated between −0.07 and 0.05. Notably, emissivity differences varying between −0.02 and 0.02 represented a considerable proportion (Valor: 100.00%, Qin: 95.48%, Jordi: 99.89%, Yu: 98.92%, Li: 96.87%, respectively); such a emissivity range could lead to temperature errors of at least about 2.0 K (Hulley et al. Citation2015; Ren et al. Citation2017; Chen et al. Citation2016). On the whole, the VIHM by Valor yielded relatively consistent emissivity values between SDGSAT-1 and Landsat 8.
Furthermore, larger differences in emissivity were observed mostly for highly heterogeneous urban surfaces, including vegetation and man-made surfaces, whereas small differences were observed for water bodies which exhibited more uniform distributions. Combining this observation with the land classifications, the average absolute differences in emissivity for each land cover based on the five NDEMs were computed ().
Figure 6. Average absolute differences in emissivity of each land cover based on the five NDEMs (SDGSAT-1 vs Landsat 8).

Regardless of the type of land covers, the largest average absolute differences in emissivity were registered by the method by Qin (road: 0.014, tree cover: 0.026, grassland: 0.018, cropland: 0.015, building: 0.013, barren and sparse veg.: 0.015, water: 0.006). In contrast, the smallest differences were registered by by Valor (road: 0.007, tree cover: 0.013, grassland: 0.009, cropland: 0.007, building: 0.006, barren and sparse veg.: 0.007, water: 0.003).
4.4. Inter-comparison with ECOSTRESS/MODIS LSE product
According to the geographical overlaps between SDGSAT-1 and ECOSTRESS when they transited Guangzhou, the pixelwise-emissivity was accordingly normalized and compared ().
Figure 7. Distributions of emissivity differences () based on: (a) original VIHM proposed by (Valor and Caselles Citation1996), (b) improved hybrid model (Qin, Karnieli, and Berliner Citation2010), (c)
of (Cristóbal et al. Citation2018), (d)
of (Yu, Guo, and Wu Citation2014);, (e)
of (Li and Jiang Citation2018).

Analogously, pixel-level emissivity differences were found to fluctuate from −0.070–0.049 and fell primarily between −0.02 and 0.02 (Valor: 87.71%, Qin: 92.12%, Jordi: 95.50%, Yu: 93.61%, Li: 94.85%, respectively). Additionally, the largest difference was still observed for the more discontinuous and heterogeneous spatial distributions of tree covers, whereas the smallest was observed for the relatively homogeneous water bodies. For each class (), the largest average absolute differences in emissivity were registered by the method of Valor (road: 0.036, tree cover: 0.060, grassland: 0.042, cropland: 0.041, building: 0.034, barren and sparse veg.: 0.033, water: 0.020). Conversely, the smallest differences were registered by the method of Li (road: 0.017, tree cover: 0.029, grassland: 0.022, cropland: 0.021, building: 0.018, barren and sparse veg.: 0.018, water: 0.009). Notably, the emissivity differences between SDGSAT-1 and ECOSTRESS exceeded those between SDGSAT-1 and Landsat 8.
Figure 8. Average absolute differences in emissivity of each land cover based on the five NDEMs (SDGSAT-1 vs ECOSTRESS LSE product).

Furthermore, the pixel-level differences of the normalized LSE between resampled SDGSAT-1 and MODIS product were calculated (). It was noteworthy that the MODIS-derived emissivity of water was consistently zero, resulting in pronounced emissivity differences for its pixels. The proportion of pixels with retrieved emissivity differences between −0.02 and 0.02 were smaller (Valor: 93.88%, Qin: 69.55%, Jordi: 84.76%, Yu: 76.20%, Li: 84.07%, respectively) than those of previous comparisons. In particular, the method by Valor yielded the smallest overall emissivity difference across the entire image, whereas that by Qin yielded the largest.
Figure 9. Distributions of emissivity differences () based on (a) original VIHM proposed by (Valor and Caselles Citation1996), (b) improved hybrid model (Qin, Karnieli, and Berliner Citation2010), (c)
of (Cristóbal et al. Citation2018), (d)
of (Yu, Guo, and Wu Citation2014);, (e)
of Li (Li and Jiang Citation2018).

During the upscaling process of classification, land covers with small and discrete distributions, such as grasslands, were indistinguishable in classified images with a coarse spatial resolution (1000 m). For other land covers, the largest differences were registered by the method of Qin (road: 0.062, tree cover: 0.124, cropland: 0.093, building: 0.066, barren and sparse veg.: 0.107, water: 0.049). Conversely, the smallest were registered by the method of Valor (road: 0.035, tree cover: 0.070, cropland: 0.057, building: 0.043, barren and sparse veg.: 0.069, water: 0.031). It was noteworthy that the average absolute emissivity differences of water were lower, but emissivity differences of water shown in were higher: this might be attributed to statistical anomalies caused by geographic alignment errors between the classification image and images of emissivity difference. Overall, given the substantial discrepancies in the spatial resolution between SDGSAT-1 and MODIS, the differences in emissivity between them () was significantly larger than those between SDGSAT-1 and Landsat 8.
5. Discussion
5.1. Comparison between different LSE-estimation algorithms
For non-water surfaces, four out of the five methods (i.e. those by Valor, Li, Jordi, and Yu) exhibited only insignificant differences in retrieved emissivity (about 0.01) for the same type of land cover (). However, the method by Qin exhibited a notable difference. The four said methods would divide the surfaces into pure vegetation, pure bare soil, and mixed vegetation and bare soil based on the NDVI differences. They would then employ different formulas to respectively calculate the emissivity for each surface type. In contrast, the method by Qin would consider differences in spectral and temperature ratios between the urban and natural surfaces before calculating the corresponding emissivity through different formulas. Thus, the method by Qin better able to distinguish spectral differences in complex urban surfaces and thus retrieve more accurate emissivity of non-water surfaces.
For water bodies, the different algorithms provided varying emissivity values. The method by Qin appeared to be reasonably accurate, yielding an emissivity value of water that was higher than those of vegetation and urban surfaces. This was partly because water would significantly absorb solar radiation and simultaneously emit heat from the sediment-water interface back to the water surface, resulting in its property capable of emitting substantial thermal radiation. Contrarily, the other four methods yielded emissivity values of water that were generally lower than those of other land covers. This might be due to the fact that these methods had been formulated without considering the specificities of thermal radiation from water bodies. Additionally, the lower emissivity values might be attributed to the misclassification of water bodies with small NDVI values as bare soil. Therefore, these findings underline the need to improve the precision of emissivity values for water as retrieved by the NDEMs.
5.2. Differences in retrieved emissivity on different spatial resolutions
The LSE retrieved from SDGSAT-1 were resampled to lower spatial resolutions (30, 70 m and even 1000 m) for comparisons with not only the corresponding emissivity retrieval results from Landsat 8, but also products from ECOSTRESS and MODIS. Despite subtle differences in the central wavelengths of the TIR bands based on different RS data, measurements of emissivity spectra for common land covers have demonstrated that the they would exert only a negligible impact on emissivity differences (Zhong et al. Citation2022; Kotthaus et al. Citation2014). This phenomenon was due to the smoothing effect of the spectrum over the TIR range from 10 to 11 . Therefore, it is reasonable to compare the emissivity derived from different thermal infrared data sources without considering the impact of slight differences in center wavelengths.
Retrieved emissivity values based on RS data with different spatial resolutions (i.e. 30 and 70 m) registered smaller differences for spatially homogeneous water bodies, but larger ones for heterogeneous vegetation and built-up areas. Absolute differences in retrieved emissivity values grew progressively significant with decreasing spatial resolutions of the data. Several reasons could explain this phenomenon. Firstly, SDGSAT-1 and Landsat 8 exhibited similar transit periods with comparable meteorological conditions; hence, the relatively small differences in the retrieved emissivity through the same method. Conversely, the transit times of ECOSTRESS and MODIS differed considerably from those of SDGSAT-1; hence, the more pronounced differences in the retrieved emissivity. Additionally, even for the same land cover, the use of different retrieval algorithms could result in varying emissivity values. Notably, ECOSTRESS and MODIS employed the multi-channel TES algorithm, whereas this study used NBEMs that retrieved emissivity from a single TIR channel. Lastly, declining spatial resolution could bring about numerous mixed pixels, leading to not only inaccurate classification results and retrieved emissivity, but also more critical geographic alignment errors. This could therefore translate into significant emissivity differences for land covers with high heterogeneity and scattered distribution. Accordingly, the emissivity differences of the tree covers between SDGSAT-1 and MODIS were the most substantial, highlighting the need to retrieve the emissivity of highly heterogeneous urban surfaces through high-spatial-resolution images that are less affected by mixed pixels.
Given the differences in the scale, it would be challenging to use the ground truth data to validate the emissivity retrieved from lower-spatial-resolution images. Emissivity extracted from SDGSAT-1 at a spatial resolution of 10 m could mitigate the impacts of such differences and serve as the validation data for emissivity products generated from lower-spatial-resolution data. To further verify the accuracy of emissivity retrieved from SDGSAT-1 with the higher spatial resolution, the average emissivity values of each land cover were compared with those obtained from previous studies that employed either UAVs or field measurements (Zhong et al. Citation2021). Our results demonstrated that all NBEMs produced emissivity values for lush vegetation (ranging from almost 0.970–0.990) that were highly consistent with those obtained from ground truth and UAV-derived images. Moreover, for man-made surfaces (e.g. roads or buildings), the average emissivity values retrieved across the five NBEMs ranged from 0.964–0.980, while values retrieved from UAVs and validated via ground measurements fluctuated from 0.933–0.976. Such slight differences could be explained by variations in the spatial resolution and in the properties of the man-made materials. Overall, emissivity retrieval based on SDGSAT-1 could ensure accurate emissivity retrieval of land covers through the use of high-spatial-resolution RS images, demonstrating the potential of such RS images in validating products generated from coarser spatial resolutions and in improving the accuracy of quantitative retrieval.
5.3. Future work on TIR quantitative retrieval of SDGSAT-1
The VNIR data obtained from SDGSAT-1, with a higher spatial resolution, served as a fundamental tool for retrieving the emissivity of surfaces with distinct features, especially those in heterogeneous built-up areas. However, existing algorithms for emissivity retrieval largely focus on natural surfaces, since complex urban surfaces are by contrast characterized by thermodynamic properties that are challenging to quantify with a unified model. To enhance the accuracy of emissivity retrieval in built-up areas, it is necessary to optimize the retrieval algorithms. In particular, empirical emissivity-retrieval models for special urban land covers, such as trees, water, metal, roofs (metallic or non-metallic), and roads composed of different materials, can be established based on spectral measurements on the ground (Zhong et al. Citation2022). Such empirical models can then be utilized in high-spatial-resolution data, such as SDGSAT-1, to separately retrieve the emissivity of the corresponding types.
Moreover, it is vital to compare the accuracy of different LST-retrieval algorithms with SDGSAT-1 data. Apart from single-channel algorithms, split-window, and multi-channel algorithms may be used based on the multiple TIR bands of SDGSAT-1. Based on the temperature readings obtained via UAVs in real time (Zhong et al. Citation2022), it is feasible to select the optimal algorithm for retrieving LSTs with SDGSAT-1. From the perspective of applications to the thermal environment, LST products that obtain a high spatiotemporal resolution created from SDGSAT-1 can provide a dependable and accurate foundation for analyzing spatiotemporal distributions, causes, and corresponding cooling measures of surface urban heat island, (primarily at the local scale). Besides, LST products with a higher spatial resolution can also be regarded as the reference for comprehensively validating the accuracy of LST products generated from satellite data with coarser spatial resolutions.
6. Conclusion
Accurate acquisition of LSE plays a crucial role in the retrieval accuracy of LSTs. The accuracy of retrieved emissivity has been found to depend not only on differences in surface properties, but also on the radiometric calibration performance of the observational sensors, the spatial resolution of the data, and the algorithms used. In this study, the SDGSAT-1 data and five typical NBEMs were used to retrieve the LSE of Guangzhou. Highly heterogeneous and discretely distributed vegetation and man-made surfaces exhibited significant emissivity differences at varying spatial resolutions, whereas relatively homogeneous water bodies registered smaller differences. Other insightful findings are following:
The high correlation (almost exceeding 0.9) between reflectance and brightness temperature obtained from both SDGSAT-1 and Landsat 8 indicated good radiometric calibration performance of SDGSAT-1.
Based on SDGSAT-1, the average emissivity values of lush vegetation retrieved from five different NBEMs were almost higher than 0.980, whereas those of built-up areas drastically fluctuated from 0.964–0.980.
Compared to Landsat 8, five NDEMs registered the ideal performance based on SDGSAT-1, yielding average absolute emissivity differences less than 0.026 among all land covers.
Decreasing spatial resolution led to progressively pronounced differences in retrieved emissivity values among different land covers, with the maximum difference between SDGSAT-1 and MODIS LSE product of up to 0.124.
Disclosure statement
No potential conflict of interest was reported by the author(s).
Data availability statement
Data supporting the findings of this study are available on request from the first author. They are not publicly available as they contain information that can compromise the research participants’ privacy.
Correction Statement
This article has been corrected with minor changes. These changes do not impact the academic content of the article.
Additional information
Funding
References
- Baldridge, A. M., S. J. Hook, C. I. Grove, and G. Rivera. 2008. “The ASTER Spectral Library Version 2.0.” Remote Sensing of Environment 113: 711–715. https://doi.org/10.1016/j.rse.2008.11.007.
- Brodu, E., M. Balat-Pichelin, J. L. Sans, and J. C. Kasper. 2014. “Influence of Roughness and Composition on the Total Emissivity of Tungsten, Rhenium and Tungsten–25% Rhenium Alloy at High Temperature.” Journal of Alloys and Compounds 585: 510–517. https://doi.org/10.1016/j.jallcom.2013.09.184.
- Chakraborty, T. C., Xuhui Lee, Sofia Ermida, and Wenfeng Zhan. 2021. “On the Land Emissivity Assumption and Landsat-Derived Surface Urban Heat Islands: A Global Analysis.” Remote Sensing of Environment 265: 112682. https://doi.org/10.1016/j.rse.2021.112682.
- Chang, Yue, Jingfeng Xiao, Xuxiang Li, Decheng Zhou, and Yiping Wu. 2022. “Combining GOES-R and ECOSTRESS Land Surface Temperature Data to Investigate Diurnal Variations of Surface Urban Heat Island.” Science of The Total Environment 823: 153652. https://doi.org/10.1016/j.scitotenv.2022.153652.
- Chen, G., H. He, Y. Q. Chen, L. H. Zhao, Y. N. Cai, and Y. Ma. 2023. “Applying a Diagnostic Equation for Maximum Urban Heat Island Intensity Based on Local Climate Zones for Guangzhou, China.” Building and Environment 228, https://doi.org/10.1016/j.buildenv.2022.109817.
- Chen, Shanshan, Huazhong Ren, Xin Ye, Jiaji Dong, and Yitong Zheng. 2021. “Geometry and Adjacency Effects in Urban Land Surface Temperature Retrieval from High-Spatial-Resolution Thermal Infrared Images.” Remote Sensing of Environment 262: 112518. https://doi.org/10.1016/j.rse.2021.112518.
- Chen, Feng, Song Yang, Z. Su, and Kai Wang. 2016. “Effect of Emissivity Uncertainty on Surface Temperature Retrieval Over Urban Areas: Investigations Based on Spectral Libraries.” ISPRS Journal of Photogrammetry and Remote Sensing 114: 53–65. https://doi.org/10.1016/j.isprsjprs.2016.01.007.
- Coll, César, Raquel Niclòs, Jesús Puchades, Vicente García-Santos, Joan Miquel Galve, Lluís Pérez-Planells, Enric Valor, and Evangelos Theocharous. 2019. “Laboratory Calibration and Field Measurement of Land Surface Temperature and Emissivity using Thermal Infrared Multiband Radiometers.” International Journal of Applied Earth Observation and Geoinformation 78: 227–239. https://doi.org/10.1016/j.jag.2019.02.002.
- Cristóbal, Jordi, Juan Jiménez-Muñoz, Anupma Prakash, Cristian Mattar, Dražen Skoković, and José Sobrino. 2018. “An Improved Single-Channel Method to Retrieve Land Surface Temperature from the Landsat-8 Thermal Band.” Remote Sensing 10 (3), https://doi.org/10.3390/rs10030431.
- Dash, P., F. M. Göttsche, F. S. Olesen, and H. Fischer. 2002. “Land Surface Temperature and Emissivity Estimation from Passive Sensor Data: Theory and Practice-Current Trends.” International Journal of Remote Sensing 23 (13): 2563–2594. https://doi.org/10.1080/01431160110115041.
- Heiden, Uta, Karl Segl, Sigrid Roessner, and Hermann Kaufmann. 2007. “Determination of Robust Spectral Features for Identification of Urban Surface Materials in Hyperspectral Remote Sensing Data.” Remote Sensing of Environment 111 (4): 537–552. https://doi.org/10.1016/j.rse.2007.04.008.
- Hook, S., and G. Hulley. 2019. “ECOSTRESS Land Surface Temperature and Emissivity Daily L2 Global 70 m V001.” In NASA EOSDIS Land Processes DAAC. USA.
- Hu, Y. H., M. T. Hou, G. S. Jia, C. L. Zhao, X. J. Zhen, and Y. H. Xu. 2019. “Comparison of Surface and Canopy Urban Heat Islands Within Megacities of Eastern China.” ISPRS Journal of Photogrammetry and Remote Sensing 156: 160–168. https://doi.org/10.1016/j.isprsjprs.2019.08.012.
- Hu, Tian, Glynn C. Hulley, Kaniska Mallick, Zoltan Szantoi, and Simon Hook. 2023. “Comparison Between the ASTER and ECOSTRESS Global Emissivity Datasets.” International Journal of Applied Earth Observation and Geoinformation 118, https://doi.org/10.1016/j.jag.2023.103227.
- Hulley, Glynn C., Simon J. Hook, Elsa Abbott, Nabin Malakar, Tanvir Islam, and Michael Abrams. 2015. “The ASTER Global Emissivity Dataset (ASTER GED): Mapping Earth's emissivity at 100 meter spatial scale.” Geophysical Research Letters 42 (19): 7966–7976. https://doi.org/10.1002/2015GL065564.
- Hulley, Glynn C., Simon J. Hook, and Alice M. Baldridge. 2010. “Investigating the Effects of Soil Moisture on Thermal Infrared Land Surface Temperature and Emissivity Using Satellite Retrievals and Laboratory Measurements.” Remote Sensing of Environment 114 (7): 1480–1493. https://doi.org/10.1016/j.rse.2010.02.002.
- Jiang, Geng-Ming, Zhao-Liang Li, and Françoise Nerry. 2006. “Land Surface Emissivity Retrieval from Combined mid-Infrared and Thermal Infrared Data of MSG-SEVIRI.” Remote Sensing of Environment 105 (4): 326–340. https://doi.org/10.1016/j.rse.2006.07.015.
- Jiang, Yazhen, Ronglin Tang, and Zhao-Liang Li. 2022. “A Framework of Correcting the Angular Effect of Land Surface Temperature on Evapotranspiration Estimation in Single-Source Energy Balance Models.” Remote Sensing of Environment 283: 113306. https://doi.org/10.1016/j.rse.2022.113306.
- Jimenez-Munoz, J. C., J. A. Sobrino, D. Skokovic, C. Mattar, and J. Cristobal. 2014. “Land Surface Temperature Retrieval Methods from Landsat-8 Thermal Infrared Sensor Data.” IEEE Geoscience and Remote Sensing Letters 11 (10): 1840–1843. https://doi.org/10.1109/LGRS.2014.2312032.
- Kotthaus, Simone, Thomas E. L. Smith, Martin J. Wooster, and C. S. B. Grimmond. 2014. “Derivation of an Urban Materials Spectral Library Through Emittance and Reflectance Spectroscopy.” ISPRS Journal of Photogrammetry and Remote Sensing 94: 194–212. https://doi.org/10.1016/j.isprsjprs.2014.05.005.
- Li, Qiang, Jing Cui, Wenliang Jiang, Qisong Jiao, Lixia Gong, Jingfa Zhang, and Xuhui Shen. 2021. “Monitoring of the Fire in Muli County on March 28, 2020, Based on High Temporal-Spatial Resolution Remote Sensing Techniques.” Natural Hazards Research 1 (1): 20–31. https://doi.org/10.1016/j.nhres.2021.02.001.
- Li, Zhuohong, Wei He, and Hongyan Zhang. 2023. National-Scale 1-m Resolution Land-Cover Mapping for the Entire China Based on a low-Cost Solution and Open-Access Data. People’s Republic of China. arXiv. https://doi.org/10.48550/arXiv.2303.05305.
- Li, Shanshan, and Geng-Ming Jiang. 2018. “Land Surface Temperature Retrieval from Landsat-8 Data With the Generalized Split-Window Algorithm.” IEEE Access 6: 18149–18162. https://doi.org/10.1109/ACCESS.2018.2818741.
- Li, Z. L., W. Ning, Q. Shi, Jose A. Sobrino, Z. Wan, B. H. Tang, and G. Yan. 2012. “Land Surface Emissivity Retrieval from Satellite Data.” International Journal of Remote Sensing 34 (9-10): 3084–3127. https://doi.org/10.1080/01431161.2012.716540.
- Li, Zhao-Liang, Hua Wu, Si-Bo Duan, Wei Zhao, Huazhong Ren, Xiangyang Liu, Pei Leng, et al. 2023b. “Satellite Remote Sensing of Global Land Surface Temperature: Definition, Methods, Products, and Applications.” Reviews of Geophysics 61 (1): e2022RG000777. https://doi.org/10.1029/2022RG000777.
- Li, Z. L., H. Wu, N. Wang, S. Qiu, J. A. Sobrino, Z. M. Wan, B. H. Tang, and G. J. Yan. 2013b. “Land Surface Emissivity Retrieval from Satellite Data.” International Journal of Remote Sensing 34 (9-10): 3084–3127. https://doi.org/10.1080/01431161.2012.716540.
- Liang, Shunlin, Dongdong Wang, Tao He, and Yunyue Yu. 2019. “Remote Sensing of Earth’s Energy Budget: Synthesis and Review.” International Journal of Digital Earth 12 (7): 737–780. https://doi.org/10.1080/17538947.2019.1597189.
- Mohseni, Farzane, Maryam Kiani Sadr, Saeid Eslamian, Atta Areffian, and Ali Khoshfetrat. 2021. “Spatial and Temporal Monitoring of Drought Conditions Using the Satellite Rainfall Estimates and Remote Sensing Optical and Thermal Measurements.” Advances in Space Research 67 (12): 3942–3959. https://doi.org/10.1016/j.asr.2021.02.017.
- Neinavaz, Elnaz, Andrew K. Skidmore, and Roshanak Darvishzadeh. 2020. “Effects of Prediction Accuracy of the Proportion of Vegetation Cover on Land Surface Emissivity and Temperature Using the NDVI Threshold Method.” International Journal of Applied Earth Observation and Geoinformation 85: 101984. https://doi.org/10.1016/j.jag.2019.101984.
- Olivera-Guerra, L., C. Mattar, O. Merlin, C. Durán-Alarcón, A. Santamaría-Artigas, and R. Fuster. 2017. “An Operational Method for the Disaggregation of Land Surface Temperature to Estimate Actual Evapotranspiration in the Arid Region of Chile.” ISPRS Journal of Photogrammetry and Remote Sensing 128: 170–181. https://doi.org/10.1016/j.isprsjprs.2017.03.014.
- Peres, Leonardo F., and Carlos C. DaCamara. 2004. “Land Surface Temperature and Emissivity Estimation Based on the two-Temperature Method: Sensitivity Analysis Using Simulated MSG/SEVIRI Data.” Remote Sensing of Environment 91 (3): 377–389. https://doi.org/10.1016/j.rse.2004.03.011.
- Pu, Ruiliang, and Stefania Bonafoni. 2023. “Thermal Infrared Remote Sensing Data Downscaling Investigations: An Overview on Current Status and Perspectives.” Remote Sensing Applications: Society and Environment 29: 100921. https://doi.org/10.1016/j.rsase.2023.100921.
- Qin, Z., A. Karnieli, and P. Berliner. 2010. “A Mono-Window Algorithm for Retrieving Land Surface Temperature from Landsat TM Data and its Application to the Israel-Egypt Border Region.” International Journal of Remote Sensing 22 (18): 3719–3746. https://doi.org/10.1080/01431160010006971.
- Ren, Huazhong, Rongyuan Liu, Qiming Qin, Wenjie Fan, Le Yu, and Chen Du. 2017. “Mapping Finer-Resolution Land Surface Emissivity Using Landsat Images in China.” Journal of Geophysical Research: Atmospheres 122 (13): 6764–6781. https://doi.org/10.1002/2017JD026910.
- Ru, Chen, Si-Bo Duan, Xiao-Guang Jiang, Zhao-Liang Li, Cheng Huang, and Meng Liu. 2023. “An Extended SW-TES Algorithm for Land Surface Temperature and Emissivity Retrieval from ECOSTRESS Thermal Infrared Data Over Urban Areas.” Remote Sensing of Environment 290: 113544. https://doi.org/10.1016/j.rse.2023.113544.
- Rubio, E., V. Caselles, and C. Badenas. 1997. “Emissivity Measurements of Several Soils and Vegetation Types in the 8–14, μm Wave Band: Analysis of two Field Methods.” Remote Sensing of Environment 59 (3): 490–521. https://doi.org/10.1016/S0034-4257(96)00123-X.
- Rüdisser, D., R. S. McLeod, W. Wagner, and C. J. Hopfe. 2022. “Numerical Derivation and Validation of the Angular, Hemispherical and Normal Emissivity and Reflectivity of Common Window Glass.” Building and Environment 207: 108536. https://doi.org/10.1016/j.buildenv.2021.108536.
- Salisbury, John W., and Dana M. D'Aria. 1992. “Emissivity of Terrestrial Materials in the 8–14 μm Atmospheric Window.” Remote Sensing of Environment 42 (2): 83–106. https://doi.org/10.1016/0034-4257(92)90092-X.
- Siddiqui, Asfa, Gautami Kushwaha, Bhaskar Nikam, S. K. Srivastav, Ankita Shelar, and Pramod Kumar. 2021. “Analysing the day/Night Seasonal and Annual Changes and Trends in Land Surface Temperature and Surface Urban Heat Island Intensity (SUHII) for Indian Cities.” Sustainable Cities and Society 75: 103374. https://doi.org/10.1016/j.scs.2021.103374.
- Snyder, W. C., Z. Wan, Y. Zhang, and Y. Z. Feng. 2010. “Classification-based Emissivity for Land Surface Temperature Measurement from Space.” International Journal of Remote Sensing 19 (14): 2753–2774. https://doi.org/10.1080/014311698214497.
- Sobrino, Jose A., Juan C. Jimenez-Munoz, Guillem Soria, Mireia Romaguera, Luis Guanter, Jose Moreno, Antonio Plaza, and Pablo Martinez. 2008. “Land Surface Emissivity Retrieval from Different VNIR and TIR Sensors.” IEEE Transactions on Geoscience and Remote Sensing 46 (2): 316–327. https://doi.org/10.1109/TGRS.2007.904834.
- Sun, Donglian, and Rachel T. Pinker. 2003. “Estimation of Land Surface Temperature from a Geostationary Operational Environmental Satellite (GOES-8).” Journal of Geophysical Research: Atmospheres 108 (D11), https://doi.org/10.1029/2002JD002422.
- Valor, Enric, and Vicente Caselles. 1996. “Mapping Land Surface Emissivity from NDVI: Application to European, African, and South American Areas.” Remote Sensing of Environment 57: 167–184. https://doi.org/10.1016/0034-4257(96)00039-9.
- Van De Griend, A., and M. Owe. 2012. “On the Relationship Between Thermal Emissivity and the Normalized Difference Vegetation Index for Natural Surfaces.” International Journal of Remote Sensing 14 (6): 1119–1131. https://doi.org/10.1080/01431169308904400.
- Wan, Z., S. Hook, and G. Hulley. 2015. “MYD11_L2 MODIS/Aqua Land Surface Temperature/Emissivity 5-Min L2 Swath 1 km V006.” In, Edited by NASA EOSDIS Land Processes DAAC. USA.
- Wang, Mengmeng, Guojin He, Zhaoming Zhang, Guizhou Wang, Zhihua Wang, Ranyu Yin, Shiai Cui, Zhijie Wu, and Xiaojie Cao. 2019. “A Radiance-Based Split-Window Algorithm for Land Surface Temperature Retrieval: Theory and Application to MODIS Data.” International Journal of Applied Earth Observation and Geoinformation 76: 204–217. https://doi.org/10.1016/j.jag.2018.11.015.
- Wang, Fei, Zhihao Qin, Caiying Song, Lili Tu, Arnon Karnieli, and Shuhe Zhao. 2015. “An Improved Mono-Window Algorithm for Land Surface Temperature Retrieval from Landsat 8 Thermal Infrared Sensor Data.” Remote Sensing 7 (4): 4268–4289. https://doi.org/10.3390/rs70404268.
- Wang, M., Z. Zhang, T. Hu, and X. Liu. 2018. “A Practical Single-Channel Algorithm for Land Surface Temperature Retrieval: Application to Landsat Series Data.” Journal of Geophysical Research: Atmospheres, https://doi.org/10.1029/2018jd029330.
- Wang, Ziwei, Ji Zhou, Shaomin Liu, Mingsong Li, Xiaodong Zhang, Zhiming Huang, Weichen Dong, Jin Ma, and Lijiao Ai. 2022. “A Land Surface Temperature Retrieval Method for UAV Broadband Thermal Imager Data.” IEEE Geoscience and Remote Sensing Letters 19: 1–5. https://doi.org/10.1109/lgrs.2021.3100586.
- Wigneron, J. P., T. J. Jackson, P. O'Neill, G. De Lannoy, P. de Rosnay, J. P. Walker, P. Ferrazzoli, et al. 2017. “Modelling the Passive Microwave Signature from Land Surfaces: A Review of Recent Results and Application to the L-Band SMOS & SMAP Soil Moisture Retrieval Algorithms.” Remote Sensing of Environment 192: 238–262. https://doi.org/10.1016/j.rse.2017.01.024.
- Xia, Haiping, Yunhao Chen, Conghe Song, Junxiang Li, Jinling Quan, and Guomo Zhou. 2022. “Analysis of Surface Urban Heat Islands Based on Local Climate Zones via Spatiotemporally Enhanced Land Surface Temperature.” Remote Sensing of Environment 273: 112972. https://doi.org/10.1016/j.rse.2022.112972.
- Yu, Xiaolei, Xulin Guo, and Zhaocong Wu. 2014. “Land Surface Temperature Retrieval from Landsat 8 TIRS-Comparison Between Radiative Transfer Equation-Based Method, Split Window Algorithm and Single Channel Method.” Remote Sensing 6 (10): 9829–9852. https://doi.org/10.3390/rs6109829.
- Yu, Bing, Jie She, Guoxiang Liu, Deying Ma, Rui Zhang, Zhiwei Zhou, and Bo Zhang. 2022. “Coal Fire Identification and State Assessment by Integrating Multitemporal Thermal Infrared and InSAR Remote Sensing Data: A Case Study of Midong District, Urumqi, China.” ISPRS Journal of Photogrammetry and Remote Sensing 190: 144–164. https://doi.org/10.1016/j.isprsjprs.2022.06.007.
- Zarei, Arastou, Reza Shah-Hosseini, Sadegh Ranjbar, and Mahdi Hasanlou. 2021. “Validation of non-Linear Split Window Algorithm for Land Surface Temperature Estimation Using Sentinel-3 Satellite Imagery: Case Study; Tehran Province, Iran.” Advances in Space Research 67 (12): 3979–3993. https://doi.org/10.1016/j.asr.2021.02.019.
- Zheng, Xiaopo, Zhao-Liang Li, Tianxing Wang, Huabing Huang, and Françoise Nerry. 2022. “Determination of Global Land Surface Temperature Using Data from Only Five Selected Thermal Infrared Channels: Method Extension and Accuracy Assessment.” Remote Sensing of Environment 268: 112774. https://doi.org/10.1016/j.rse.2021.112774.
- Zhong, Xue, Lihua Zhao, Jie Wang, Xiang Zhang, Zichuan Nie, Yingtan Li, and Peng Ren. 2022. “A Retrieval Method for Land Surface Temperatures Based on UAV Broadband Thermal Infrared Images via the Three-Dimensional Look-up Table.” Building and Environment 226: 109793. https://doi.org/10.1016/j.buildenv.2022.109793.
- Zhong, Xue, Lihua Zhao, Jie Wang, Haichao Zheng, Junru Yan, Rong Jin, and Peng Ren. 2021. “Empirical Models on Urban Surface Emissivity Retrieval Based on Different Spectral Response Functions: A Field Study.” Building and Environment 197, https://doi.org/10.1016/j.buildenv.2021.107882.
- Zhong, Xue, Lihua Zhao, Xiang Zhang, Junru Yan, and Peng Ren. 2022. “Investigating the Effects of Surface Moisture Content on Thermal Infrared Emissivity of Urban Underlying Surfaces.” Construction and Building Materials 327: 127023. https://doi.org/10.1016/j.conbuildmat.2022.127023.
- Zhong, Xue, Lihua Zhao, Haichao Zheng, Yingtan Li, Yang Zhang, and Peng Ren. 2022. “An Improved Model for Emissivity Retrieval of Complex Urban Surfaces Based on Spectral Indices from UAV.” Sustainable Cities and Society 84: 104032. https://doi.org/10.1016/j.scs.2022.104032.
- Zhou, Q. X., Z. L. Jing, and S. Z. Jiang. 2003. “Remote Sensing Image Fusion for Different Spectral and Spatial Resolutions with Bilinear Resampling Wavelet Transform.” Fusion 2003: Proceedings of the Sixth International Conference of Information Fusion, Vols 1 AND 2.
- Zhou, Jianhong, Kun Yang, Wade T. Crow, Jianzhi Dong, Long Zhao, Huihui Feng, Mijun Zou, Hui Lu, Ronglin Tang, and Yaozhi Jiang. 2023. “Potential of Remote Sensing Surface Temperature- and Evapotranspiration-Based Land-Atmosphere Coupling Metrics for Land Surface Model Calibration.” Remote Sensing of Environment 291: 113557. https://doi.org/10.1016/j.rse.2023.113557.