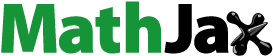
ABSTRACT
Accurately mapping large-scale areas of aquaculture ponds along China’s coastal areas is challenging. This study proposes a multi-featured decision tree method that incorporates multi-spectral indices, water inundation frequency (WIF), and object-oriented rules to extract aquaculture ponds in coastal zones using 65332 Sentinel-2 images on the GEE platform. The proposed method was validated, with the average overall accuracy of the extraction results from 2017 to 2021 reaching 90.80%, and an average F1 Score of 0.91. The results of this study indicate that the overall area of aquaculture ponds in China's coast in 2021 was 7633.83 km2, mainly located in coastal bay estuaries in Shandong Province, Jiangsu Province and Guangdong Province. In recent years, the area of coastal ponds for aquaculture in China has shown a decreasing trend, with an average annual decrease of 235.65 km2. The analysis combined with land use data shows that the ponds that have been reduced in the past five years have mainly been converted to abandoned aquaculture ponds, wetlands, and other land use types.
1. Introduction
Aquaculture ponds play an crucial role in the economy of coastal zones, where they are used to breed aquatic organisms and can represent important ecosystem functions (Chen et al. Citation2023a and Citation2023b; Pauly and Zeller Citation2017). According to the Food and Agriculture Organization (FAO) of the United Nations, China supplies more than 60% of aquaculture products for the world.(FAO Citation2016; Joffre, Poortvliet, and Klerkx Citation2019). The uncontrolled development of coastal aquaculture ponds in recent years is closely related to economic growth, increased demand for aquatic products and improved infrastructure. Previous studies have found that the rapid development of coastal ponds for aquaculture in recent years has led to a range of negative impacts, such as the rapid decline of natural coastal wetlands, seawater pollution and the loss of wildlife habitat (Duan et al. Citation2021; Liu et al. Citation2020; Loisel et al. Citation2017; Rubio-Portillo et al. Citation2019; Yang et al. Citation2022). According to the UN Sustainable Development Goal 14.1, by 2025, there is a need to prevent and significantly reduce marine pollution (Liang et al. Citation2023; Sun et al. Citation2023a). As a big country in fishery and aquaculture, China has always attached importance to pollution prevention and standardization of fishery and aquaculture. On March 4, 2016 and November 20, 2017, China promulgated the new version of the ‘Standards for the Establishment of Healthy Aquaculture Demonstration Farms’ and the ‘National Marine Pasture Demonstration Zone Construction Plan (2017–2025)’. Therefore, for the coordinated development of coastal zones, it is crucial to fully understand the most recent spatial distribution and temporal and spatial changes of coastal aquaculture ponds in China.
Traditional methods of monitoring aquaculture ponds for on-site sampling and survey are time-consuming and laborious. In recent years, the rapid development of remote sensing technology has provided more effective support for monitoring and mapping aquaculture ponds (Safikhani et al. Citation2022; Sun et al. Citation2023b). Automatic extraction methods such as machine learning, object-oriented methods, and deep learning are increasingly been used to extract and map aquaculture ponds. The Random Forest (RF) algorithm is one of the most used machine learning classifiers in aquaculture pond mapping (Xia, Guo, and Chen Citation2020; Xu et al. Citation2021) along with decision-tree models. By combining the multi-source features of ponds, the researchers realized the extraction and mapping of aquaculture ponds. (Duan et al. Citation2020; Peng et al. Citation2022; Wang et al. Citation2023). The use of deep learning methods to identify and extract aquaculture ponds can achieve satisfactory results but requires a large number of sample points, has poor generalizability, and is not scalable at large scales (Ferriby et al. Citation2021; Liang et al. Citation2021; Yue et al. Citation2023; Zou et al. Citation2022). Object-oriented methods based on high-resolution image data have been widely used in ponds extraction because of the unique geometric shape characteristics of aquaculture ponds. Researchers have used various segmentation algorithms combined with spectral and shape features to establish classification rules (Ren et al. Citation2019; Virdis Citation2014).
Early studies have mostly focused on the mono-temporal mapping of aquaculture ponds, ignoring phenological characteristics and mixing aquaculture ponds with other waterbodies. In contrast, time series remote sensing data can more accurately reveal the spatial distribution and temporal changes of aquaculture ponds, but there are still some challenges. First, using a single water index combined with a threshold extraction approach is not accurate in large-scale, complex water areas, as shown in Wang et al. (Citation2023), which completed the extraction and spatial change analysis of aquaculture ponds in China. Although the study provides a valuable reference, it used a single water index and a fixed segmentation scale, ignoring the spatial heterogeneity of aquaculture ponds, resulting in inaccurate extraction. Furthermore, previous aquaculture pond maps are incomplete or outdated, failing to reflect the latest spatial distribution of aquaculture ponds and changes in China. The use of the latest remote sensing images from Sentinel-2 and more advanced remote sensing techniques to accurately map the coverage and spatial distribution of aquaculture ponds in China. The Google Earth Engine (GEE) platform provides free access to massive remote sensing images and high-performance computing power, showing great potential for mapping the spatial distribution of coastal aquaculture ponds.
Therefore, a multi-featured decision tree method incorporating multi-spectral indices, water inundation frequency (WIF), and object-oriented methods based on 65332 Sentinel-2 images is proposed to accurately obtain the spatial distribution and temporal and spatial changes of aquaculture ponds in China's coastal zone. This method can solve the mixing of aquaculture ponds, salt ponds, abandoned aquaculture ponds and other seasonal waterbodies by taking into account the spectrum, shape, phenology characteristics, and spatial heterogeneity of the ponds.
2. Study area and datasets
2.1. Study area
China's coastline starts from the mouth of the Yalu River in the north to the mouth of the Beilun estuary in the south, with a total length of about 1.80 × 104 km, covering 12 provinces, cities and autonomous regions (Hou et al. Citation2022). The coastal zone is rich in fishery and biological resources, including marine creatures, mangrove and wetland ecosystems. As the greatest producer of fish in the world, the sustainable development of its aquaculture industry is critical to global economic growth and food security.
The rivers in the study area are distributed throughout the low-lying areas, forming rich deltas, bays, and other topographies that provide ideal conditions for the development of aquaculture. The study area was defined as the extent of a 30-km buffer zone inland based on the global multiple scale shorelines dataset (GMSSD). The study area includes 9 provinces of Liaoning, Hebei, Shandong, Jiangsu, Zhejiang, Fujian, Guangdong, Guangxi and Hainan, and 2 municipalities directly under the Central Government of Tianjin and Shanghai ().
2.2. Data
2.2.1. Sentinel-2 imagery and pre-processing
GEE (https://earthengine.google.com) provides high-performance computing power and rich geospatial datasets, greatly promoting large-scale remote sensing studies (Deng et al. Citation2023; Gorelick et al. Citation2017). Sentinel 2A/B data are satellites of the European Copernicus program that undertake multispectral high-resolution observations (Farmonov et al. Citation2023; Zhang et al. Citation2023). Sentinel-2A was launched in June 2015 and Sentinel-2B was put in orbit in July 2017, the combination of which confers the Sentinel-2 mission a short revisit period (2-5 days), and the details of Sentinel-2A/2B satellites are shown in (Sun et al. Citation2022; Wang et al. Citation2022). The Sentinel-2 time-series images (69,420) used in this study were derived from the Level-2A product of the GEE platform.
Table 1. Details of Sentinel-2A/2B satellites.
To construct high-quality time-series images, we performed the following processing. First, a time filtering window was set from April to October each year, when vegetation growth and pond aquaculture are at their peak, to facilitate the distinction between aquaculture ponds and background features. Secondly, the images with less than 20% clouds were screened and then cloud-masked using the quality assessment (QA) band. Most of the study area still had more than 36 good observations after the de-clouding process. Finally, the GEE platform was used to evaluate the image quality after filtering to ensure that the image was cloudless and clear.
2.2.2. Auxiliary datasets
We used the following auxiliary data to generate our study area and to assist in the mapping of the aquaculture ponds.
GMSSD shoreline data
(2) | SRTM digital elevation data |
(3) | Sample data |
Table 2. The number of sample points in this study from 2017 to 2021.
3. Methods
A decision tree method combined with multi-features based on Sentinel-2 data is proposed for the extraction and mapping of coastal aquaculture ponds. The extraction and mapping of the ponds can be summarized in the following main steps: (1) Data preprocessing, (2) extraction of potential aquaculture pond area, (3) extraction of permanent water, (4) extraction of fine aquaculture ponds, and (5) accuracy assessment ().
3.1. Extraction of potential aquaculture ponds
Based on GMSSD shoreline data, a 30-km buffer was generated along the Chinese coast to mask off the seawater. Since coastal aquaculture ponds are usually built in low-lying areas, SRTM DEM data were used to exclude areas with a high elevation and slope. Analysis of the elevation and slope histograms of aquaculture ponds based on training sample data revealed that most ponds were located in areas with an elevation under 10 m and slope less than 10° (). Therefore, areas meeting the criteria of DEM < 10 m and slope < 10° were considered as potential locations for aquaculture ponds.
3.2. Extraction of permanent water
Since aquaculture ponds and surface water have similar spectral characteristics, the accurate extraction of water is crucial for the mapping of aquaculture ponds. Water indices such as normalized difference water index (NDWI) (Gao Citation1996), modified normalized difference water index (mNDWI) (Xu Citation2006) and automated water extraction index (AWEI) (Feyisa et al. Citation2014) are widely used for waterbody extraction. Waterbodies have a low reflectivity and can easily be confused with vegetation and shadows in remote sensing images. Previous research has also shown that a typical waterbody extraction algorithm, which is based on a few spectral bands, has certain accuracy limitations (Pekel et al. Citation2016). Additionally, it is challenging to obtain a precise global waterbody threshold that works consistently over a large-scale complex study area.
Aquaculture ponds are usually covered with water year-round and can easily become misseparated from other permanent water such as rivers and lakes. Therefore, the extraction of waterbodies in this study was divided into two main steps. Firstly, waterbody pixels were identified based on a decision tree method constructed from multiple spectral indices. Pixels of features such as wetlands and paddy fields that are easy to be mixed with aquaculture ponds were removed. Secondly, the method based on water inundation frequency (WIF) was applied to extract all permanent waterbodies in the study area (i.e. aquaculture ponds, rivers, lakes, reservoirs, etc.) to avoid the misclassification of salt fields, abandoned aquaculture ponds, and seasonal inundation.
3.2.1. Water pixel recognition by multi-index combination
In order to effectively avoid the errors produced by waterbody indexes like MNDWI and NDWI in recognizing features like wetlands in mixed water-body-vegetation environments, Zou et al. (Citation2018) proposed the idea of combining a waterbody index with a vegetation index. The study identified only the pixels with a stronger water signal than the vegetation signal as the actual water pixels (MNDWI > NDVI or MNDWI > EVI). To further remove vegetation-induced noise, wetland pixels with vegetation were removed using EVI (EVI < 0.1). Therefore, the pixels that satisfy the criteria ((MNDWI > NDVI or MNDWI > EVI) and (EVI < 0.1)) are categorized as waterbody pixels; the rest of the pixels are categorized as non-water pixels. As a result, waterbody identification accuracy can be increased, and the method has been shown to perform well in the United States, Poyang Lake, and other places (Pickens et al. Citation2020; Tulbure and Broich Citation2019). Therefore, based on this idea, this paper designs a decision tree algorithm to identify waterbody pixels by combining multiple spectral indices.
In the actual extraction process, other non-water surface noises such as shadows are generated during the waterbody extraction using the above method. AWEI improves the extraction accuracy of surface water by suppressing noise from shadows and other non-water black surfaces. This study integrated multiple indices of NDVI, EVI, MNDWI and AWEI to design a decision tree process for water pixel recognition, combining prior studies and feature types in the study area.
AWEI indices are divided into AWEInsh and AWEIsh according to the difference of noise sources in different types of areas. AWEInsh can effectively eliminate dark building surface non-water pixels in urban background areas. Thus, it can be used in the case where shadows are not the main noise. AWEIsh is mainly applicable to the area where shadows are the main noise. Finally, a new rule for determining water pixels based on Sentinel-2 images was obtained: ((MNDWI > EVI or MNDWI > NDVI) and EVI < 0.1) and (AWEInsh > 0 or AWEIsh > −0.1). Pixels that satisfy the above rules are identified as waterbody pixels, otherwise, they are non-waterbody pixels ( and ).
Table 3. Spectral indices used for the determination of water pixels.
3.2.2. Permanent waterbodies recognition based on WIF in time series images
Aquaculture ponds are typically emptied of water in the winter and spring in a process known as clearing. During this time, it is not possible to extract water-based data using remote sensing techniques. To overcome this limitation, most studies utilize remote sensing images taken between April and October when the ponds are filled with water and vegetation growth is more robust. This time period is preferred because it allows for greater contrast between the aquaculture ponds and surrounding features, making it easier to extract the ponds from the images. Coastal aquaculture ponds are sometimes abandoned due to both natural and human factors. When these ponds fill with water due to precipitation or other reasons, they can appear as seasonal waters that are highly similar in spectral characteristics to actual aquaculture ponds. Therefore, distinguishing between permanent aquaculture ponds and seasonal waterbodies based solely on single-day images can be challenging.
The water inundation frequency (WIF) is defined as the ratio of the number of times any pixel is identified as water over a period of time to the total number of times that pixel is well observed. The WIF of each pixel can be calculated as:
(6)
(6) where S denotes the binary variable of the pixel location type, with a value of 1 for waterbodies and 0 for non-waterbodies; T is the total number of valid observations of a pixel in a period of time.
Waterbody types were divided into seasonal and permanent waterbodies based on the WIF values of each pixel. In order to find the right WIF threshold, we utilized a stepwise search strategy with a step size of 0.05 and a range of 0.05–0.95. depicts the relationship between the WIF threshold (horizontal axis) and the overall accuracy of permanent waterbody extraction (vertical axis). The results show that classifying permanent waterbodies and other waterbodies are best done with the WIF criterion set at 0.7.
Permanent waterbodies with WIF > 70% were extracted based on the time-series remote sensing images from 2017 to 2021, respectively. The permanent water extracted in this step includes other types of waterbodies such as aquaculture ponds lakes, rivers, and reservoirs. Next, based on the permanent waterbodies that was extracted, we take into account the object-oriented method to extract the fine aquaculture ponds.
3.3. Extraction of fine aquaculture ponds
3.3.1. Multiscale segmentation
Artificial ponds tend to be a regular rectangle in shape. Aquaculture ponds along the coast are frequently grouped together and divided by dykes or roadways, which makes a dense grid pattern in space. Aquaculture ponds can be distinguished from other waterbodies, such as lakes, rivers, and reservoirs, due to these characteristics. In order to distinguish aquaculture ponds from other permanent waterbodies, an object-oriented method was adopted.
The idea of the extraction of object-oriented methods is from simple to complex. Other irrelevant information is eliminated first, and then the useful feature categories are identified through multiple screening to realize the hierarchical extraction of features. The object-oriented method is generally divided into three steps. First, the images are segmented to form homogeneous objects. Then, various characteristics of these objects are extracted, such as spectrum, form, size, area based on the classification task requirements. Finally, according to the feature differences among the objects, a classification system is established and the final classification results are obtained. eCognition is one of the most mature tools in object-oriented classification software, and the subsequent object-oriented experiments in this paper were conducted in this software. The binary image map of permanent waterbodies processed in the previous step was used as a source of classification data, and the spectra, shapes, rectangularity, compactness, and features were calculated separately, based on which the images were segmented at multiple scales.
According to literature reviews and visual interpretation of high-resolution photographs, aquaculture ponds in China's coastal regions show significant spatial heterogeneity, which causes differences in optimal segmentation scales among different regions. Using the traditional single threshold approach for segmentation will inevitably lead to inaccurate subsequent classification. The optimal segmentation parameters for multi-scale segmentation are calculated by the ESP (estimation of scale parameters) tool, which is a very mature plug-in for evaluating the optimal segmentation scale of images and is widely used in object-oriented classification (). (Drǎguţ, Tiede, and Levick Citation2010; Hossain and Chen Citation2019; Martins et al. Citation2020).
Each province presents unique aquaculture pond characteristics in terms of size and shape because of regional variations in climate and geography. To avoid the mistakes brought on by applying a single segmentation scale, we thus examined the best segmentation scales for each province individually. The accuracy of the segmentation scale was verified by using sample points combined with visual interpretation. We observed that the optimal segmentation scale varied from province to province. The final optimal segmentation scales for each province were shown in the while shows the segmentation results of aquaculture ponds in four typical areas.
Figure 8. Segmentation results in three typical area. (A) Liaodong Bay, Liaoning Province; (B) Bohai Bay, Shandong Province; (C) Sanmen Bay, Zhejiang Province; (D) Jiao Bay, Guangdong Province.

Table 4. Optimal segmentation scale for each province.
3.3.2. Coastal aquaculture pond object extraction by decision tree method
We applied the decision tree method for the secondary classification of the permanent water objects. The main feature types to be distinguished in the secondary classification were aquaculture ponds, rivers, lakes, reservoirs, etc. The study classified ponds based on the unique spatial clustering and shape characteristics of aquaculture ponds. The specific features selected for classification were area, landscape shape index (LSI), compactness, rectangular fit and aspect ratio of the permanent water objects. provides a detailed explanation and definition of each classification feature index.
Table 5. The specific description and meaning of each classification feature.
Aquaculture ponds are often smaller and have regular geometries for ease of cultivation compared to natural waterbodies. The majority is less than 5 hectares and tend to be clustered. In contrast, natural waterbodies tend to have irregular shapes. Therefore, As a result, aquaculture ponds have far higher levels of rectangularity and compactness than other types of waterbodies, whereas their LSI feature values are significantly lower. The classification thresholds of Area, Compactness, LSI, Rectangular Fit and Aspect Ratio were trained according to the polygon sample data of each province (). Finally, other waterbodies were removed by setting appropriate threshold values.
Figure 9. Classification thresholds trained from polygon sample data. (OW: other waterbody types; AP: aquaculture ponds.) (a) Statistical results of the area; (b) Statistical results of LSI; (c) Statistical results of the compactness; (d) Statistical results of the rectangular fit.

Next, it is necessary to distinguish aquaculture ponds from regularly shaped independent reservoirs that are similar in both shape and size to aquaculture ponds (). These independent reservoirs cannot be distinguished from aquaculture ponds using spectral and geometric features only. Therefore, considering the spatial characteristics of the aggregation distribution of aquaculture ponds, the distance between the nearest neighbors of each potential aquaculture pond object was calculated. Then a suitable threshold value was set to eliminate the independently distributed reservoirs. After the training results, the minimum distance threshold as set at 20 m, and if no other water object existed within 20 m near a water object, it was considered an independent reservoir.
4. Results
4.1. Accuracy assessment
The study used four evaluation metrics, namely Recall, Precision and overall accuracy and F1 Score, to quantitatively evaluate the extraction accuracy in each period. Producer accuracy and user accuracy were used for aquaculture ponds, Ove.acc was used to represent the overall accuracy in distinguishing between aquaculture and non-aquaculture ponds. The results of the confusion matrix based on the sample point data from 2017 to 2021 were shown in .
(11)
(11)
(12)
(12)
(13)
(13)
(14)
(14)
Table 6. Confusion matrix and F1 scores for the aquaculture pond map in China during 2017-2021.
The results show that the various accuracy evaluation indexes of the aquaculture pond maps reached 0.90 or above each year. The average Ove. acc reached 90.81% and the average F1 Score reached 0.91. The results of the confusion matrix indicate that the aquaculture pond maps mapped in thisstudy are in high consistency with the validation sample points.
We compared the extraction results of the proposed method with the actual water surface of the aquaculture pond based on the visual interpretation in order to further assess the effectiveness of the method. Four representative bays with aquaculture ponds were selected, namely Liaodong Bay in Liaoning Province, Bohai Bay in Shandong Province, Sanmen Bay in Zhejiang Province and Jiao Bay in Guangdong Province. As shown in , from the visual interpretation, the extracted boundaries of each aquaculture pond are clear and complete. The results of the aquaculture pond extracted by our method are highly consistent with the visual interpretation results. The overlap between the two is high and the extraction is good with an average overlap rate of 0.89 (). There were errors in the extraction results at the edges of the pond. In general, the area of aquaculture ponds extracted based on this paper is smaller than the actual area, mainly because of spatial resolution limitations resulting in the inaccurate extraction of the boundaries of the aquaculture ponds.
Figure 11. Comparison of aquaculture ponds extracted with sample polygons obtained by automated extraction based on Google Earth. (a) Liaodong Bay, Liaoning Province; (b) Bohai Bay, Shandong Province; (c) Sanmen Bay, Zhejiang Province; (d) Jiao Bay, Guangdong Province.

Table 7. Comparison of the aquaculture ponds area in typical areas.
4.2. Areas and spatial distribution of China’s coastal aquaculture ponds
The spatial distribution of China's coastal aquaculture ponds during 2017–2021 is shown in . In 2021, the overall area of aquaculture ponds in the study area was 7633.83 km2, with uneven distribution among provinces. In general, the overall area in northern China is larger than the total area in southern China. We calculated the distribution area of aquaculture ponds in 2021 in each province. The provinces of Shandong, Guangdong, and Liaoning had the top area, 1978.35, 1124.71 and 1028.24 km2, respectively, accounting for 54.11% of the country. The provinces with the smallest aquaculture pond area were Hainan Province (136.92 km2) and Guangxi Province (297.02 km2).
Figure 12. The spatial distribution of China's coastal aquaculture ponds during 2017-2021. (A) Liaodong Bay, Liaoning Province; (B) Laizhou Bay, Shandong Province; (C) Yancheng City, Jiangsu Province; (D) Sanmen Bay, Zhejiang Province; (E) Changsha Bay, Guangdong Province; (F) Beihai Port, Guangxi Province.

We applied kernel density analysis in ArcGIS to show the spatial aggregation of aquaculture ponds in China (). The kernel density map of aquaculture pond distribution in 2021 clearly shows that coastal aquaculture ponds in China are mainly concentrated in various estuaries and bays. In general, they are mainly distributed in three regions: the Bohai Sea Rim, the coastal plain of Jiangsu, and the coastal area of Guangdong, indicating that these coastal provinces pay more attention to the development of aquaculture fisheries.
4.3. Temporal and spatial change of coastal aquaculture ponds in China
Previous studies found an overall trend of coastal aquaculture pond expansion in China until 2016. This study analyzed the changing pattern of aquaculture ponds in China's coastal areas from 2017–2021, in terms of the total national area of 8576.41 km2 in 2017 and 7633.82 km2 in 2021, with an overall decreasing trend, in contrast to the trend before 2016.
We calculated the annual rate of change (ACR) for each province in the entire coastal region (). During the period 2017–2021, the overall area of coastal aquaculture ponds decreased by 942.59 km2, with an overall ACR of −235.65 km2/year. In terms of individual provinces’ area change rates, Shandong, Guangdong, and Liaoning had the highest ACRs of −45.87 km2/year, −41.92 km2/year, and −48.85 km2/year, respectively, and were among the hot spot areas. The lowest ACR was −3.58 km2/year in Guangxi, −5.65 km2/year in Hainan, and −0.63 km2/year in Shanghai. In addition, we checked and compared the fisheries statistical bulletin of the Ministry of Agriculture and Rural Affairs of the People’s Republic of China (http://www.moa.gov.cn/) since 2017. The statistical bulletin also pointed out that the aquaculture pond area in China has been decreasing annually in the past five years, which also verifies the reliability of this study. Based on the analysis of statistical data, we found that the decline in China's coastal aquaculture production is much smaller than the decline in aquaculture area, indicating that the structure of aquaculture ponds has been optimized.
Figure 14. Aquaculture pond area changes in China's coastal zone from 2017 to 2021. (a) Aquaculture pond ACR in coastal provinces during 2017–2021. (b) Changes in the area of aquaculture ponds by province during 2017–2021. (c) National aquaculture pond total area changes during 2017–2021.

In addition, we analyzed the temporal and spatial change of coastal aquaculture ponds in four important bays in China (). According to the results 2017–2021, the trend of area change is different for each bay. The area of aquaculture ponds in Sanmen Bay and Liaodong Bay shows an increasing trend, while the area of Bohai Bay and Horn Bay remains almost stable.
4.4. Analysis of conversion of aquaculture ponds to other land use types
The percentage of coastal aquaculture ponds converted to different land cover types in China and by province is shown in . The analysis combined with land use data shows that the main reasons for the loss of coastal aquaculture ponds are conversion to abandonment (24.63%), wetland (22.55%), sea (11.81%) and agricultural land (10.18%). Overall, most aquaculture ponds that have been reduced in recent years are in an abandoned state and have not yet been converted to specific land types. In several provinces, such as Guangdong, Guangxi, Liaoning, Hebei and Jiangsu, the highest percentage of conversion into abandoned aquaculture ponds has reached more than 25%. The policy of returning aquaculture ponds to wetlands converted more than 20% of the reduced pond area in Jiangsu, Zhejiang, Fujian and Guangxi. The policy of returning the ponds to the sea, converted more than 20% of the reduced pond area in Shanghai, Zhejiang, Fujian and other regions. Returning ponds to farming resulted in the conversion of more than 15% of reduced pond area in in Hebei, Tianjin, Shandong and other regions.
Figure 16. Percentage of aquaculture ponds converted into land cover types in China’s coastal provinces during 2017–2021.

In response to the continuous expansion of aquaculture ponds, the government has introduced relevant policies to curb their uncontrolled expansion. Currently, aquaculture ponds have shown a decreasing trend, and government departments should pay attention to the problem of reusing abandoned aquaculture ponds. According to the regional conditions, corresponding measures are taken to transform the abandoned ponds into wetlands, oceans or agricultural land. According to Sustainable Development Goals(SDG) 14.7, the economic advantages to Small Island Developing States from sustainable use of marine resources shall grow by 2030, especially through sustainable management of fisheries, aquaculture. Therefore, the government and related departments should pay more attention to land use planning after the ponds are retired. Combined with the related planning of SDG14, emphasis should be paid on the restoration of the marine ecological environment.
5. Discussion
5.1. Effectiveness and significance of the extraction framework
We developed a decision tree based on image time-series information integrated with multiple spectral indices on the GEE platform, WIF and object-oriented classification methods to extract aquaculture ponds in China’s coastal area. The method has the following improvements relative to previous research methods.
Firstly, the accuracy of waterbody extraction is improved. Traditional methods extract waterbodies by combining a single waterbody index with a threshold. However, this study integrated multiple waterbody indices and vegetation indices to design a decision tree, effectively solving the problem of mixed classification of waterbody pixels and feature pixels such as wetlands and paddy fields that are common with the traditional approach. (2) Additionally, considering the time series characteristics of aquaculture ponds, we introduced the WIF method, which effectively solves the problem of mixing and dividing between aquaculture ponds and salt fields and abandoned aquaculture ponds. (3) We also adopted a multi-scale segmentation approach that fully considers the spatial heterogeneity of aquaculture ponds in different regions. Cloud computing saved manual workload and analysis time, making the method scalable and transferable.
To further verify the validity of the methods in this paper in detail, we compared the data products with two publicly land cover products at 10-m spatial resolution, FROM-GLC10(Gong et al. Citation2019), and CWaC (Li and Niu Citation2022). In both datasets, aquaculture ponds were obtained as a class of waterbodies by a pixel-based supervised classification method.
Although the boundaries of aquaculture ponds extracted from each dataset are almost the same, our product has a clear advantage in showing aquaculture pond details and more accurately identifying dikes between adjacent ponds to extract and map their boundaries. In contrast, the FROM-GLC10 dataset does not distinguish dikes due to the difference in research method and tends to merge several aquaculture ponds within a large area into one large aquaculture pond, which also causes errors in the extraction. The CWaC dataset solves the problems found in the FROM-GLC10 dataset, but it does not completely consider the temporal and spatial features of aquaculture ponds, resulting in aquaculture ponds and salt pans being misclassified.
More importantly, by using the temporal characteristics of aquaculture ponds, our method can more accurately distinguish aquaculture ponds from abandoned aquaculture ponds and salt pans (). In addition, our extraction method, being based on object-oriented classification, avoids the salt-and-pepper effect generated by pixel-based classification.
5.2. Uncertainties in aquaculture pond mapping
The remaining issues in our study can be primarily summed up in three points, which are also the areas that require improvement and development in future studies. First, mixed pixels arise as a result of image resolution limitations. Because the distances between adjacent ponds are less than 10 m, dikes will fall into mixed pixels, which results in the connection of several ponds. The use of higher-resolution images will yield better extraction results. Secondly, the coastline in this study is based on manually drawn vector data, which is generally highly accurate but can include errors generated by manual interpretation. Due to changes in land use in coastal areas, fixed shoreline vector data may not be sufficient to meet the needs of tracking the dynamic changes of aquaculture ponds.
5.3. Limits and future works
With the increased temporal and spatial resolution of remote sensing satellites, it is possible to avoid mixed image elements and extract aquaculture ponds with greater accuracy. Our method is highly portable and can be extended to a global scale to map global aquaculture ponds and explore their spatial and temporal changes patterns. This research could also be extended to investigate the impact of spatial–temporal changes in aquaculture ponds on coastal ecosystems through an interdisciplinary approach. This will help us to understand the impact of aquaculture ponds on the ecological environment and carbon cycle in more detail and develop sustainable ecological development measures.
It is increasingly recognized that the development of aquaculture ponds worldwide has negative effects on coastal zone ecological and offshore environments. SDG14.1 aims to reduce marine pollution of all kinds, in particular from land-based activities, including marine debris and nutrient pollution, by 2025. Coastal aquaculture effluents are an important source of marine nutrient pollution. Therefore, comprehensive environmental impact assessments and government policies are required to promote more sustainable coastal aquaculture ponds. Earth observation images can be used to analyze the long-time spatial–temporal changes of aquaculture areas and their impacts on the economy and ecological systems and further, provide support for sustainable developments of the study area.
6. Conclusions
Monitoring coastal aquaculture ponds in China is essential for the scientific management and sustainable development of its coastal zones.
In this study, we proposed a method for extracting and mapping landside aquaculture ponds using time series remote sensing images based on a multi-feature decision tree and analyzed the temporal and spatial change of aquaculture ponds in China's coastal areas during 2017–2021. Compared to prior research, this study refined the depiction of aquaculture pond patches, detailing the spatial distribution and changes of aquaculture ponds in the last five years. The average overall accuracy of the extraction results from 2017 to 2021 was 90.80% and the average F1 Score reached 0.91. The results of this study indicate that the total area of coastal ponds for aquaculture in China in 2021 was 7633.83 km2, mainly distributed in coastal bay estuaries in Shandong Province, Jiangsu Province and Guangdong Province. The results of this study indicate that the total area of coastal ponds for aquaculture in China in 2021 was 7633.83 km2.
Our analysis further revealed that the expansion of aquaculture ponds in China's coastal areas has ceased, and the area has decreased annually by an average of 235.65 km2. Analyzing the decline in combination with land use data shows that the ponds that have been reduced in the past five years have mainly been converted to abandoned aquaculture ponds, wetlands, and other land use types. Government departments should pay particular attention to the problem of reusing abandoned aquaculture ponds.
The proposed method can serve as an effective reference for the extraction and mapping of global aquaculture ponds. Additionally, the produced dataset can provide essential data support for the effective management and sustainable development of coastal zones.
Disclosure statement
No potential conflict of interest was reported by the author(s).
Additional information
Funding
References
- Chen, Chao, Yankun Chen, Haohai Jin, Li Chen, Zhisong Liu, Haozhe Sun, Junchi Hong, Haonan Wang, Shiyu Fang, and Xin Zhang. 2023b. “3D Model Construction and Ecological Environment Investigation on a Regional Scale Using UAV Remote Sensing.” Intelligent Automation & Soft Computing 37: 1655–1672. https://doi.org/10.32604/iasc.2023.039057.
- Chen, Chao, Jintao Liang, Gang Yang, and Weiwei Sun. 2023a. “Spatio-temporal Distribution of Harmful Algal Blooms and Their Correlations with Marine Hydrological Elements in Offshore Areas, China.” Ocean & Coastal Management 238: 106554. https://doi.org/10.1016/j.ocecoaman.2023.106554.
- Deng, Yawen, Weiguo Jiang, Ziyan Ling, Xiaoya Wang, Kaifeng Peng, and Zhuo Li. 2023. “Automated and Refined Wetland Mapping of Dongting Lake Using Migrated Training Samples Based on Temporally Dense Sentinel 1/2 Imagery.” International Journal of Digital Earth 16 (1): 3199–3221. https://doi.org/10.1080/17538947.2023.2241428.
- Drǎguţ, Lucian, Dirk Tiede, and Shaun R Levick. 2010. “ESP: A Tool to Estimate Scale Parameter for Multiresolution Image Segmentation of Remotely Sensed Data.” International Journal of Geographical Information Science 24: 859–871. https://doi.org/10.1080/13658810903174803.
- Duan, Yuanqiang, Xing Li, Lianpeng Zhang, Wei Liu, Dan Chen, and Hanyu Ji. 2020. “Detecting Spatiotemporal Changes of Large-Scale Aquaculture Ponds Regions Over 1988–2018 in Jiangsu Province, China Using Google Earth Engine.” Ocean & Coastal Management 188: 105144. https://doi.org/10.1016/j.ocecoaman.2020.105144.
- Duan, Yuanqiang, Bo Tian, Xing Li, Dongyan Liu, Dhritiraj Sengupta, Yujue Wang, and Ya Peng. 2021. “Tracking Changes in Aquaculture Ponds on the China Coast Using 30 Years of Landsat Images.” International Journal of Applied Earth Observation and Geoinformation 102: 102383. https://doi.org/10.1016/j.jag.2021.102383.
- FAO, I. 2016. “The State of World Fisheries and Aquaculture 2016.” In 200. Publications of Food and Agriculture Organization of the United Nations Rome.
- Farmonov, Nizom, Khilola Amankulova, József Szatmári, Jamol Urinov, Zafar Narmanov, Jakhongir Nosirov, and László Mucsi. 2023. “Combining PlanetScope and Sentinel-2 Images with Environmental Data for Improved Wheat Yield Estimation.” International Journal of Digital Earth 16 (1): 847–867. https://doi.org/10.1080/17538947.2023.2186505.
- Farr, Tom G, Paul A Rosen, Edward Caro, Robert Crippen, Riley Duren, Scott Hensley, Michael Kobrick, Mimi Paller, Ernesto Rodriguez, and Ladislav Roth. 2007. “The Shuttle Radar Topography Mission.” Reviews of Geophysics 45 (2): RG2004. https://doi.org/10.1029/2005RG000183.
- Ferriby, Hannah, Amir Pouyan Nejadhashemi, Juan Sebastian Hernandez-Suarez, Nathan Moore, Josué Kpodo, Ian Kropp, Rasu Eeswaran, Ben Belton, and Mohammad Mahfujul Haque. 2021. “Harnessing Machine Learning Techniques for Mapping Aquaculture Waterbodies in Bangladesh.” Remote Sensing 13: 4890. https://doi.org/10.3390/rs13234890.
- Feyisa, Gudina L., Henrik Meilby, Rasmus Fensholt, and Simon R. Proud. 2014. “Automated Water Extraction Index: A new Technique for Surface Water Mapping Using Landsat Imagery.” Remote Sensing of Environment 140: 23–35. https://doi.org/10.1016/j.rse.2013.08.029.
- Gao, Bo-Cai. 1996. “NDWI – A Normalized Difference Water Index for Remote Sensing of Vegetation Liquid Water from Space.” Remote Sensing of Environment 58: 257–266. https://doi.org/10.1016/S0034-4257(96)00067-3.
- Gong, Peng, Han Liu, Meinan Zhang, Congcong Li, Jie Wang, Huabing Huang, Nicholas E. Clinton, et al. 2019. “Stable Classification with Limited Sample: Transferring a 30-m Resolution Sample set Collected in 2015 to Mapping 10-m Resolution Global Land Cover in 2017.” Science Bulletin 64 (370–373): 3. https://doi.org/10.1016/j.scib.2019.03.002.
- Gorelick, Noel, Matt Hancher, Mike Dixon, Simon Ilyushchenko, David Thau, and Rebecca Moore. 2017. “Google Earth Engine: Planetary-Scale Geospatial Analysis for Everyone.” Remote Sensing of Environment 202: 18–27. https://doi.org/10.1016/j.rse.2017.06.031.
- Hossain, Mohammad D, and Dongmei Chen. 2019. “Segmentation for Object-Based Image Analysis (OBIA): A Review of Algorithms and Challenges from Remote Sensing Perspective.” ISPRS Journal of Photogrammetry and Remote Sensing 150: 115–134. https://doi.org/10.1016/j.isprsjprs.2019.02.009.
- Hou, Tingting, Weiwei Sun, Chao Chen, Gang Yang, Xiangchao Meng, and Jiangtao Peng. 2022. “Marine Floating Raft Aquaculture Extraction of Hyperspectral Remote Sensing Images Based Decision Tree Algorithm.” International Journal of Applied Earth Observation and Geoinformation 111: 102846. https://doi.org/10.1016/j.jag.2022.102846.
- Joffre, Olivier M., P. Marijn Poortvliet, and Laurens Klerkx. 2019. “To Cluster or not to Cluster Farmers? Influences on Network Interactions, Risk Perceptions, and Adoption of Aquaculture Practices.” Agricultural Systems 173: 151–160. https://doi.org/10.1016/j.agsy.2019.02.011.
- Li, Yang, and Zhenguo Niu. 2022. “Systematic Method for Mapping Fine-Resolution Water Cover Types in China Based on Time Series Sentinel-1 and 2 Images.” International Journal of Applied Earth Observation and Geoinformation 106. https://doi.org/10.1016/j.jag.2021.102656.
- Liang, Jintao, Chao Chen, Yongze Song, Weiwei Sun, and Gang Yang. 2023. “Long-term Mapping of Land use and Cover Changes Using Landsat Images on the Google Earth Engine Cloud Platform in bay Area – A Case Study of Hangzhou Bay, China.” Sustainable Horizons 7: 100061. https://doi.org/10.1016/j.horiz.2023.100061.
- Liang, Chenbin, Bo Cheng, Baihua Xiao, Chenlinqiu He, Xunan Liu, Ning Jia, and Jinfen Chen. 2021. “Semi-/Weakly-Supervised Semantic Segmentation Method and Its Application for Coastal Aquaculture Areas Based on Multi-Source Remote Sensing Images – Taking the Fujian Coastal Area (Mainly Sanduo) as an Example.” Remote Sensing 13: 1083. https://doi.org/10.3390/rs13061083.
- Liu, Yueming, Zhihua Wang, Xiaomei Yang, Yuanzhi Zhang, Fengshuo Yang, Bin Liu, and Peiyuan Cai. 2020. “Satellite-based Monitoring and Statistics for Raft and Cage Aquaculture in China’s Offshore Waters.” International Journal of Applied Earth Observation and Geoinformation 91: 102118. https://doi.org/10.1016/j.jag.2020.102118.
- Loisel, Hubert, Vincent Vantrepotte, Sylvain Ouillon, Dat Dinh Ngoc, Marine Herrmann, Viet Tran, Xavier Mériaux, David Dessailly, Cedric Jamet, and Thomas Duhaut. 2017. “Assessment and Analysis of the Chlorophyll-a Concentration Variability Over the Vietnamese Coastal Waters from the MERIS Ocean Color Sensor (2002–2012).” Remote Sensing of Environment 190: 217–232. https://doi.org/10.1016/j.rse.2016.12.016.
- Martins, Vitor S, Amy L Kaleita, Brian K Gelder, Hilton LF da Silveira, and Camila A Abe. 2020. “Exploring Multiscale Object-Based Convolutional Neural Network (Multi-OCNN) for Remote Sensing Image Classification at High Spatial Resolution.” ISPRS Journal of Photogrammetry and Remote Sensing 168: 56–73. https://doi.org/10.1016/j.isprsjprs.2020.08.004.
- Pauly, Daniel, and Dirk Zeller. 2017. “Comments on FAOs State of World Fisheries and Aquaculture (SOFIA 2016).” Marine Policy 77: 176–181. https://doi.org/10.1016/j.marpol.2017.01.006.
- Pekel, Jean-François, Andrew Cottam, Noel Gorelick, and Alan S Belward. 2016. “High-resolution Mapping of Global Surface Water and its Long-Term Changes.” Nature 540: 418–422. https://doi.org/10.1038/nature20584.
- Peng, Ya, Dhritiraj Sengupta, Yuanqiang Duan, Chunpeng Chen, and Bo Tian. 2022. “Accurate Mapping of Chinese Coastal Aquaculture Ponds Using Biophysical Parameters Based on Sentinel-2 Time Series Images.” Marine Pollution Bulletin 181: 113901. https://doi.org/10.1016/j.marpolbul.2022.113901.
- Pickens, Amy H, Matthew C Hansen, Matthew Hancher, Stephen V Stehman, Alexandra Tyukavina, Peter Potapov, Byron Marroquin, and Zainab Sherani. 2020. “Mapping and Sampling to Characterize Global Inland Water Dynamics from 1999 to 2018 with Full Landsat Time-Series.” Remote Sensing of Environment 243: 111792. https://doi.org/10.1016/j.rse.2020.111792.
- Ren, Chunying, Zongming Wang, Yuanzhi Zhang, Bai Zhang, Lin Chen, Yanbiao Xi, Xiangming Xiao, Russell B Doughty, Mingyue Liu, and Mingming Jia. 2019. “Rapid Expansion of Coastal Aquaculture Ponds in China from Landsat Observations During 1984–2016.” International Journal of Applied Earth Observation and Geoinformation 82: 101902. https://doi.org/10.1016/j.jag.2019.101902.
- Rubio-Portillo, Esther, Adriana Villamor, Victoria Fernandez-Gonzalez, Josefa Anton, and Pablo Sanchez-Jerez. 2019. “Exploring Changes in Bacterial Communities to Assess the Influence of Fish Farming on Marine Sediments.” Aquaculture 506: 459–464. https://doi.org/10.1016/j.aquaculture.2019.03.051.
- Safikhani, Saeed, Stephan Keller, Gerald Schweiger, and Johanna Pirker. 2022. “Immersive Virtual Reality for Extending the Potential of Building Information Modeling in Architecture, Engineering, and Construction Sector: Systematic Review.” International Journal of Digital Earth 15 (1): 503–526. https://doi.org/10.1080/17538947.2022.2038291.
- Sun, Weiwei, Chao Chen, Weiwei Liu, Gang Yang, Xiangchao Meng, Lihua Wang, and Kai Ren. 2023. “Coastline Extraction Using Remote Sensing: A Review.” GIScience & Remote Sensing 60 (1): 2243671. https://doi.org/10.1080/15481603.2023.2243671.
- Sun, Genyun, Zhaojie Pan, Aizhu Zhang, Xiuping Jia, Jinchang Ren, Hang Fu, and Kai Yan. 2023. “Large Kernel Spectral and Spatial Attention Networks for Hyperspectral Image Classification.” IEEE Transactions on Geoscience and Remote Sensing 61: 1. https://doi.org/10.1109/TGRS.2023.3292065.
- Sun, Weiwei, Kai Ren, Xiangchao Meng, Gang Yang, Chenchao Xiao, Jiangtao Peng, and Jingfeng Huang. 2022. “MLR-DBPFN: A Multi-Scale low Rank Deep Back Projection Fusion Network for Anti-Noise Hyperspectral and Multispectral Image Fusion.” IEEE Transactions on Geoscience and Remote Sensing 60: 1–14. https://doi.org/10.1109/TGRS.2022.3146296.
- Tulbure, Mirela G, and Mark Broich. 2019. “Spatiotemporal Patterns and Effects of Climate and Land use on Surface Water Extent Dynamics in a Dryland Region with Three Decades of Landsat Satellite Data.” Science of the Total Environment 658: 1574–1585. https://doi.org/10.1016/j.scitotenv.2018.11.390.
- Virdis, Salvatore Gonario Pasquale. 2014. “An Object-Based Image Analysis Approach for Aquaculture Ponds Precise Mapping and Monitoring: A Case Study of Tam Giang-Cau Hai Lagoon, Vietnam.” Environmental Monitoring and Assessment 186: 117–133. https://doi.org/10.1007/s10661-013-3360-7.
- Wang, Yumiao, Luwei Feng, Weiwei Sun, Zhou Zhang, Hanyu Zhang, Gang Yang, and Xiangchao Meng. 2022. “Exploring the Potential of Multi-Source Unsupervised Domain Adaptation in Crop Mapping Using Sentinel-2 Images.” GIScience & Remote Sensing 59 (1): 2247–2265. https://doi.org/10.1080/15481603.2022.2156123.
- Wang, Ming, Dehua Mao, Xiangming Xiao, Kaishan Song, Mingming Jia, Chunying Ren, and Zongming Wang. 2023. “Interannual Changes of Coastal Aquaculture Ponds in China at 10-m Spatial Resolution During 2016–2021.” Remote Sensing of Environment 284: 113347. https://doi.org/10.1016/j.rse.2022.113347.
- Xia, Zilong, Xiaona Guo, and Ruishan Chen. 2020. “Automatic Extraction of Aquaculture Ponds Based on Google Earth Engine.” Ocean & Coastal Management 198: 105348. https://doi.org/10.1016/j.ocecoaman.2020.105348.
- Xu, Hanqiu. 2006. “Modification of Normalised Difference Water Index (NDWI) to Enhance Open Water Features in Remotely Sensed Imagery.” International Journal of Remote Sensing 27 (14): 3025–3033. https://doi.org/10.1080/01431160600589179.
- Xu, Yue, Zhongwen Hu, Yinghui Zhang, Jingzhe Wang, Yumeng Yin, and Guofeng Wu. 2021. “Mapping Aquaculture Areas with Multi-Source Spectral and Texture Features: A Case Study in the Pearl River Basin (Guangdong), China.” Remote Sensing 13: 4320. https://doi.org/10.3390/rs13214320.
- Yang, Gang, Ke Huang, Weiwei Sun, Xiangchao Meng, Dehua Mao, and Yong Ge. 2022. “Enhanced Mangrove Vegetation Index Based on Hyperspectral Images for Mapping Mangrove.” ISPRS Journal of Photogrammetry and Remote Sensing 189: 236–254. https://doi.org/10.1016/j.isprsjprs.2022.05.003.
- Yue, Linwei, Baoguang Li, Shuang Zhu, Qiangqiang Yuan, and Huanfeng Shen. 2023. “A Fully Automatic and High-Accuracy Surface Water Mapping Framework on Google Earth Engine Using Landsat Time-Series.” International Journal of Digital Earth 16 (1): 210–233. https://doi.org/10.1080/17538947.2023.2166606.
- Zhang, Xiao, Liangyun Liu, Xidong Chen, Yuan Gao, Shuai Xie, and Jun Mi. 2021. “GLC_FCS30: Global Land-Cover Product with Fine Classification System at 30 m Using Time-Series Landsat Imagery.” Earth System Science Data 13: 2753–2776. https://doi.org/10.5194/essd-13-2753-2021.
- Zhang, Linjing, Xiaoxue Zhang, Zhenfeng Shao, Wenhao Jiang, and Huimin Gao. 2023. “Integrating Sentinel-1 and 2 with LiDAR Data to Estimate Aboveground Biomass of Subtropical Forests in Northeast Guangdong, China.” International Journal of Digital Earth 16 (1): 158–182. https://doi.org/10.1080/17538947.2023.2165180.
- Zou, Zhaohui, Chao Chen, Zhisong Liu, Zili Zhang, Jintao Liang, Huixin Chen, and Liyan Wang. 2022. “Extraction of Aquaculture Ponds Along Coastal Region Using U2-Net Deep Learning Model from Remote Sensing Images.” Remote Sensing 14: 4001. https://doi.org/10.3390/rs14164001.
- Zou, Zhenhua, Xiangming Xiao, Jinwei Dong, Yuanwei Qin, Russell B Doughty, Michael A Menarguez, Geli Zhang, and Jie Wang. 2018. “Divergent Trends of Open-Surface Water Body Area in the Contiguous United States from 1984 to 2016.” Proceedings of the National Academy of Sciences 115: 3810–3815. https://doi.org/10.1073/pnas.1719275115.