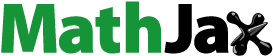
ABSTRACT
Lake distribution on the Tibetan Plateau (TP) is extensive, and lake area changes are key indicators of the TP's climate change response. Many multisource remote sensing big data for the TP, particularly optical images, are unusable due to cloud cover. Therefore, an improved isobath interpolation-based lake area extraction method is proposed and applied to obtain annual average lake areas (≥ 50 km²) on the TP from 1986 to 2020 using remote sensing big data. The lake area result accuracy was verified using existing lake area and level datasets, yielding correlation coefficients of ∼0.9. The change points and segmented trends of each lake's interannual area sequence were obtained. The relationships between lake area and climatic variables were investigated. The positive accumulation of the total precipitation minus total evaporation explains the overall lake area expansion trend after 1995. The exorheic lake interannual area is related to precipitation more than that of endorheic lakes, but endorheic lake area changes are stronger. The shrinking of lakes on the southern TP may not be climate-driven but probably attributed to lake bottom leakage. We explore detailed interannual variation characteristics of lake areas on the TP and provide reference data for studying lake responses to climate change.
1 . Introduction
The Tibetan Plateau (TP), with an average altitude of more than 4000 m, is colder than other regions except the polar regions and is known as the 'third pole of the Earth' (Qiu Citation2008). The TP has the largest ice and snow reserves outside the Antarctic and Arctic regions and is the headwaters of more than 10 major Asian rivers, known as the 'Asian Water Tower' (Yao et al. Citation2022). In addition, the TP is very sensitive to global climate change. Its warming rate (∼ 0.36 °C per decade) during 1970–2018 was approximately twice that of the global mean (∼ 0.19 °C per decade), and the TP also exhibited an obvious increase in precipitation from 1980 to 2018 (GISTEMP Citation2019; Zhang et al. Citation2021b; Zhu et al. Citation2019).
Lakes are an important natural synthesis that connects the atmosphere, biosphere, pedosphere and terrestrial hydrosphere by participating in the global/local water cycle and energy balance. Since 2018, there have been approximately 1400 lakes with an area larger than 1 km² on the TP, with a total area of approximately 5 × 104 km² (Zhang et al. Citation2019a). The main factors affecting the changes in lakes on the TP include precipitation, evaporation, glacier melting, and permafrost degradation, which are closely related to climate change. Thus, lake area (Lei et al. Citation2014; Zhang et al. Citation2019a), lake level (Crétaux et al. Citation2011), lake volume (Yang et al. Citation2017), lake surface temperature (Zhang et al. Citation2014a) and lake ice phenology (Cai et al. Citation2019) are often used as important climate change indicators on the TP. The lake area can be effectively obtained through satellite remote sensing images due to the obvious spectral difference between water bodies and land surfaces. Commonly used satellite remote sensing data, such as Landsat, MODIS (Moderate Resolution Imaging Spectroradiometer), Sentinel and GaoFen (Chinese high-resolution optical/radar satellites) series images, are applied for lake mapping on the TP (Lu et al. Citation2017; Wangchuk and Bolch Citation2020; Zhang et al. Citation2020a; Zhang et al. Citation2017b; Zhao et al. Citation2022). Among them, Landsat images have the longest time span, which can be traced back to 1984 (excluding Landsat-1∼4), with a moderate temporal resolution (16 days) and high spatial resolution (30 m). Generally, in the field of surface water dynamics remote sensing, there exists a duality between high spatial resolution and low temporal resolution studies versus low spatial resolution and high temporal resolution studies (Sogno, Klein, and Kuenzer Citation2022). The use of Landsat images in this study facilitates the study of the response of long-term interannual changes of lake area to climate changes on the TP.
Commonly used water extraction methods based on optical remote sensing images include the single-band threshold method (Jupp et al. Citation1985), spectral relationship method (Zhou, Du, and Luo Citation1996), water index method (McFeeters Citation1996; Xu Citation2006), decision tree method (Du et al. Citation2001), and object-oriented analysis method (Shen et al. Citation2012). Among these methods, the water index is a fast, simple and efficient water identification method. A lake is a relatively closed water body with a certain area. To obtain the vector boundary of the lake, the main methods include edge detection (Liu and Jezek Citation2004), regional segmentation (Setiawan, Rusydi, and Pradityo Citation2017), active contour modeling (Kass, Witkin, and Terzopoulos Citation1988), and manual digitization. Canny edge detection is a fast and accurate method to obtain lake boundaries (Liu and Jezek Citation2004). It is a good choice to combine the water index and the edge detection methods to obtain the water surface and lake boundary.
However, when using optical remote sensing images to monitor the lake area, it is not feasible to extract the complete water body and boundary of lake due to contamination by clouds and cloud shadows, especially on the TP. It is necessary to select images during the melting period from May to October on the TP to reduce the influence of snow and ice cover on water body extraction. Taking 2020 as an example, a total of 1834 Landsat-8 surface reflectance Tier1 images were collected over the TP from May to October, among which 580 images with 0–10% cloud cover were collected, accounting for only 31.6% of the total number of images. There were more clouds over the TP from May to July, and the images with less than 10% cloud cover accounted for less than 21% of the total images of that month. The high cloud cover over the TP region further results in a limited number of usable images, which may lead to long time intervals between lake area results. In some cases, the images corresponding to certain lakes are contaminated by clouds for an entire year.
For lake images that are only partially contaminated by clouds, some researchers proposed an automatic correction method based on the Global Surface Water Data Set (GSWD) (Pekel et al. Citation2016; Zhao and Gao Citation2018). This method uses the probability that each pixel is a water body to restore contaminated images, and it has been applied to the dynamic evaluation of reservoir surface area. Moreover, there is a high-frequency time series method for constructing global lake and reservoir areas using Landsat images (Yao et al. Citation2019), which uses isobaths to perform interpolation estimations of complete lake areas in contaminated images. However, neither of these two methods provided a solution to area extraction of natural lakes with complex boundaries, and visual inspection and post-processing are required to ensure the quality of the automatically generated lake area time series. In Yao et al.'s algorithm (Citation2019), the boundaries of natural lakes are automatically extracted from good images as isobaths. Nevertheless, there may be some topological errors due to the influence of small ponds around them, such as non-closure and hanging arcs. Complicated cases, such as a lake that may shrink from one whole to several parts or expand from many parts to one whole, have not been discussed.
The goal of this study is to improve the isobath-based interpolation method for extracting complete lake areas from cloudy and other contaminated optical satellite remote sensing images to make it suitable for complex lake situations with high extraction accuracy without visual inspection and post-processing requirements, so as to obtain the interannual changes in lake area on the TP during 1986–2020. This study (1) improved the interpolation method by extracting isobaths from the lake boundaries in the existing lake area datasets and conducting preprocessing, (2) extracted the area of lakes ≥ 50 km² on the TP from Landsat-5/7/8 images during 1986–2020 using the improved algorithm, (3) evaluated the extracted lake area results with the existing lake area and water level datasets, (4) analyzed the interannual lake area changes through the detection of multiple change points and segmented trend analysis, and (5) investigated the relationship between lake area changes and main climatic variables (such as air temperature, precipitation, and evaporation) based on ERA5-Land reanalysis data.
2. Study area and data
2.1. Study area
In this study, a 2500 m contour line was used to delineate the TP (approximately 25°−40°N, 67°−105°E) with an area of approximately 3 × 106 km² and an average elevation of approximately 4000 m (Zhang et al. Citation2013). The westerlies, Indian summer monsoon, and East Asian summer monsoon bring abundant water vapor to the TP, affecting the precipitation in this area (Yao et al. Citation2013). Precipitation on the TP gradually decreases from southeast to northwest, concentrated from June to September, accounting for 60–90% of the total annual precipitation (Kang et al. Citation2010). More precipitation may also lead to more cloud cover during the same period. According to the influences of different large-scale atmospheric circulation systems, the locations of lakes can be divided into four regions: the northwestern TP (mainly affected by westerly circulation), southern TP (mainly affected by Indian monsoon circulation), central TP (roughly between 30°N and 35°N, with both westerly and Indian monsoon circulation as the main influences, also known as the transition region), and northeastern TP (mainly affected by East Asian monsoon circulation) () (Maussion et al. Citation2014; Zhang, Zhang, and Zhu Citation2020b).
Figure 1. The boundary of the Tibetan Plateau and the distribution of 180 lakes. (i) Northwestern TP (Westerlies domain), (ii) Central TP (Transition domain), (iii) Southern TP (Indian monsoon domain), (iv) Northeastern TP (East Asian monsoon domain).

From 1986 to 2020, there were 187 lakes on the TP with an area larger than 50 km² for at least one year (Zhang et al. Citation2019a). Here, 180 of these lakes were selected as study objects, excluding 7 salt lakes with significant changes in area and boundary due to human development (). A total of 145 lakes are distributed in the Inner Plateau basin, accounting for 80.6% of all 180 lakes. Second, there are 8 lakes in the Qaidam River Basin, accounting for 4.4% of all 180 lakes, while other basins contain fewer lakes.
2.2. Data
The datasets used in this study mainly include Landsat-5/7/8 images, digital elevation model, existing datasets of lake area on the TP, a lake water level dataset, climate reanalysis data, and El Niño index data ().
Table 1. Multiple datasets used in this study.
2.2.1. Landsat image and digital elevation model (DEM)
In this study, the Landsat-5, 7 and 8 Surface Reflectance Tier 1 datasets on the Google Earth Engine (GEE) platform were used as remote sensing data sources to extract the lake area, and a total of approximately 92,000 images were used. All the datasets were subjected to atmospheric correction and orthography correction (USGS Citation2019a; Citation2019b), and the C Function of Mask (CFMASK) algorithm (Foga et al. Citation2017) was used to generate masks of clouds, shadows and snow. The 30 m DEM in 2000 from the Shuttle Radar Topography Mission (SRTM) of the USGS (Farr et al. Citation2007) was used to exclude the impacts due to mountains and mountain shadows.
2.2.2. Existing datasets of lake area
To provide high-precision isobaths for the lake area extraction method, this study used the lake datasets published by the National Tibetan Plateau Data Center. For the China lake dataset (1960s–2020), both Landsat images and topographic maps were used to obtain lake areas (>1 km²) in the 1960s, 1970s, 1990, 1995, 2000, 2005, 2010, 2015, and 2020 over China by combining semiautomatic water extraction with manual visual interpretation (Zhang et al. Citation2019b). The long-term sequence dataset of lake area on the Tibetan Plateau (1970–2013) includes the areas of 364 lakes (>10 km²) from the 1970s to 2013 (Zhang et al. Citation2017a). This dataset mainly uses Landsat images from October, which have less cloud cover and more stable lake area, and they obtain lake area every three years (1976–1980, 1986–1990, 1991–1993, 1994–1997, 1998–2000, 2001–2003, 2004–2007, 2008–2010, 2011–2013) to maximize the available data while reducing seasonal variation. The lakes larger than 1 km² in Tibetan Plateau (v2.0) (1970s–2018) dataset covers the periods of the 1970s, 1990, 1995, 2000, 2005, 2010, 2013, 2014, 2015, 2016, 2017 and 2018 (Zhang et al. Citation2019a; Citation2014b). Because the abovementioned three existing lake area datasets have been visually inspected, manually edited, and fully verified, they are highly accurate. The three datasets for the TP provide the same lake area in the same year, but they complement each other in different years. Their union will be used for the extraction of isobaths. Recently, the v2.0 dataset was updated to v3.1, with new data for 2019, 2020, 2021 and 2022. The new version of the data is not used to extract the isobaths but is used to verify the accuracy of the lake area extraction results.
2.2.3. Lake water level dataset
Since the lake water level is highly correlated with the lake area, the high-temporal-resolution water level and storage change dataset for lakes on the Tibetan Plateau during 2000–2017 was used to verify the extraction accuracy of lake area (Li et al. Citation2019a). The dataset is based on multisource altimetry satellite data and Landsat images, and the systematic deviation between multisource altimetry water levels was removed using optical water levels with an accuracy of 0.1–0.2 m. The dataset provides water levels of 52 large and medium-sized lakes on the TP from 2000 to 2017.
2.2.4. Other data
The enhanced global dataset ERA5-Land (Muñoz Sabater Citation2019) was generated by replaying the land part of the fifth generation of European reanalysis (ERA5). ERA5-Land's data product 'ERA5-Land Monthly Averaged' provides monthly averages of 50 climate reanalysis parameters, including air temperature, total precipitation and total evaporation. These data can be used to calculate the annual average air temperature, annual total precipitation, annual total evaporation, and annual total precipitation minus total evaporation of each lake ROI (Region of Interest). Refer to Table S1 for the main abbreviations used in this article.
The precipitation derived from the ERA5-Land reanalysis data may be overestimated relative to rain gauge data and satellite-based precipitation products (Xu et al. Citation2022; Liu et al. Citation2023a). However, the evaluation methods greatly depend on meteorological station data, but the distribution of meteorological observation stations on the TP is sparse (almost none in the central and western TP regions). The matching of stations and grids may result in loss of geographical detail, which may affect the evaluation results (Liu et al. Citation2023a). In addition, Wu et al. (Citation2023) found the ERA5-Land reanalysis data can better reproduce the intra-annual variation and spatial–temporal pattern of precipitation on the TP. The multiyear (2003–2016) average evaporation amounts corresponding to the ROI of lakes from the ERA5-Land reanalysis data were compared with the published multiyear (2003–2016) average evaporation amounts of 75 lakes on the TP ( and
are the evaporation amount during the ice-free season and the annual evaporation amount, respectively) (Wang et al. Citation2020). Both datasets reveal proportional relationships (
≈ 0.51,
≈ 0.47) (Figure S1). The low evaporation within the lake ROI attributes to the influence of evaporation from the surrounding land. In general, ERA5-Land reanalysis data can provide long-term and spatially complete estimates of meteorological variables on the TP. Although climate variables from the ERA5-Land reanalysis data may exhibit overestimation or underestimation errors, their interannual variation characteristics are relatively reliable. These climate variables can be used to study the relationship between them and interannual lake area changes.
El Niño events are characterized by abnormal warming in the eastern equatorial Pacific Ocean and have global climate teleconnections. It is one of the most significant features of interannual climate variability (Yeh et al. Citation2009). Niño 3.4 index data (Trenberth Citation1997) were mainly used to study the relationship between El Niño or La Niña events and changes in lake area. El Niño or La Niña events are defined when the Niño 3.4 index exceeds +/−0.4 °C for a period of six months or more.
3. Methods
The flow chart of this study is shown in , which is divided into two main parts: lake area extraction and accuracy verification, and interannual lake area trend analysis. The lake area extraction mainly includes (1) determining the three isobaths corresponding to the 180 lakes; (2) removing the contaminated parts and low-quality images; (3) calculating the modified normalized difference water index (MNDWI); (4) using the Otsu method to calculate the optimal water body segmentation thresholds; and (5) lake area interpolation. The verification of lake extraction accuracy mainly used the existing lake area datasets and the water level dataset to conduct correlation analysis with the results of this study. The Breaks for Additive Season and Trend (BFAST) change points detection, Mann-Kendall (M-K) trend test and Sen's slope methods were used to analyze the temporal variation trend in interannual lake area.
Figure 2. The overall technical flow chart of lake area extraction, accuracy verification, and interannual lake area trend analysis.

3.1. Improvement in the lake area extraction method
3.1.1. Determination of lake isobaths and ROI
The boundary of the lake can be considered the isobath of the lake, as the elevation of the whole lake surface is nearly the same. The lake boundaries corresponding to the maximum area, medium area and minimum area of the 180 lakes (≥ 50 km²) during 1986–2020 were selected from the abovementioned three existing lake area datasets, and the selected lake boundaries were recorded as the highest isobath, medium isobath and lowest isobath, respectively, as references for interpolation. Due to the high accuracy of the three existing lake area datasets mentioned above, the isobaths extracted in this study also have high precision.
Prior to using the derived isobaths to assist lake area extraction, there are several special situations that necessitate further processing and editing. (1) When three isobath lines partially intersect at the edge of some lakes with large slopes, to ensure the inclusion relationship between isobath lines of different heights, they need to be slightly edited. (2) For cases where multiple lakes expand and eventually connect into one lake or one lake shrinks and eventually separates into several smaller lakes, the isobaths of multiple smaller lakes need to be edited so that they are connected at the shortest distance and added very little area (Figure S2a and b). (3) For the presence of a large island in the center of the lake, the central island is excluded by establishing two lines at the shortest distance from the central island to the lake boundary (Figure S2c and d). These preprocessing methods for the derived isobaths can greatly improve the consistency of the research object and universality of the algorithm and ensure the suitability of the extracted isobaths for the subsequent fully automated process of lake area extraction, without visual correction and post-processing of the extraction results. Moreover, high-precision isobaths can result in the improvement in the final lake area extraction accuracy. The determination of lake ROIs is conducive to narrowing the research scope of lakes, reducing the amount of calculation, and facilitating the subsequent application of image segmentation algorithms (). For each lake, the highest isobath region is selected to expand the buffer zone so that the area of the ROI is approximately twice the lake area.
3.1.2. Remove the contaminated parts and low-quality images
Based on the pixel quality assessment (pixel_qa) attribute contained in the Landsat-5/7/8 images and SRTM DEM 30 m data, all Landsat-5/7/8 images from May to October including each lake during 1986–2020, were masked by removing clouds, cloud shadows, snow and mountain areas. In addition, to avoid the reduction in the extraction accuracy of lake areas caused by excessive cloud cover and poor image quality, only the images of unmasked areas within the ROI accounting for at least 50% of the total ROI were retained for subsequent processing.
3.1.3. Calculation of the MNDWI
The MNDWI has a better water recognition effect than the normalized difference water index (NDWI) (Xu Citation2006), especially when water features are mixed with buildings or vegetation (Singh et al. Citation2015). The MNDWI can also reduce the influence of mountain shadows on water body extraction. By using the MNDWI, the distinction between water bodies and non-water bodies can be largely improved.
(1)
(1) where
is the surface reflectance of the green band, corresponding to band 2 of Landsat-5/7 and band 3 of Landsat-8;
is the surface reflectance of the mid-infrared band, corresponding to band 5 of Landsat-5/7 and band 6 of Landsat-8.
3.1.4. Calculation of the optimal water segmentation threshold of the Otsu method
The Otsu algorithm, known as the maximum interclass variance method, is efficient in dividing gray images into binary images, as proposed by Otsu (Citation1979). The Otsu method was used to segment the water and non-water areas in the MNDWI images by the optimized threshold determined by Fisher's linear discriminant analysis (Fisher Citation1936). In the MNDWI, pixels less than or equal to this threshold are identified as water bodies, while other pixels are identified as non-water bodies.
Then, Canny edge detection was used to obtain the lake boundaries from the water body distinction results, which were recorded as the known parts of the lake boundary from the part of the image that was not covered by clouds or other contaminated parts ().
3.1.5. Lake area interpolation calculation
According to the known parts of the lake boundary extracted above, the two nearest isobaths are automatically selected from three reference isobaths, denoted as the lower isobath and the higher isobath
, respectively. Yao et al. (Citation2019) assumed that between the two nearest isobaths, the change of water areas in the effective observation portion (unmasked) of a contaminated image is proportional to that of their full areas (). Based on this assumption,
and
represent the full area corresponding to higher isobath h and lower isobath
, respectively, and
and
represent the partial area corresponding to higher isobath
and lower isobath
observed in the effective observation portion of the bad image, respectively. The variable
is the partial lake area in the effective observation portion within the higher isobath range in the image, that is, the known part of the lake area identified in Section 3.1.4.
is the complete lake area corresponding to
, which can be estimated by the following formula.
(2)
(2) By applying the abovementioned method to each Landsat image corresponding to each lake from 1986 to 2020, the lake area can be calculated from the available images. To analyze the interannual change in lake area results and reduce the seasonal difference within the year, it is necessary to calculate the annual average lake area.
3.2. Interpolation of missing values in interannual lake area series
In the obtained interannual lake area series from 1986 to 2020, there are a small number of missing area values for some lakes in some years. To obtain the complete lake area series, the interannual lake area series are interpolated according to adjacent values. When the missing value is in the middle of the interannual area series, linear interpolation is performed by averaging forward and backward. When the missing value is at the beginning or end of the interannual area series, it is assigned the nearest area value.
3.3. Change points detection method
The BFAST method (Verbesselt et al. Citation2010) is commonly used for the decomposition and change identification of time series data, such as the normalized difference vegetation index (NDVI) (Geng et al. Citation2019) or air temperature (Li et al. Citation2019b). The BFAST method integrates the iterative decomposition of time series into trend, seasonal and noise components with methods for detecting change within time series, without the need to select a reference period, set a threshold, or define the trajectory of change (Verbesselt et al. Citation2010).
Multiple change points can be detected by applying the BFAST method without considering the seasonal term for the area series data of each lake from 1986 to 2020. The existence of abrupt changes is assessed by the ordinary least squares (OLS) residuals-based moving sum (MOSUM) test (Zeileis Citation2005). The trend components are estimated using a robust regression based on the M-estimation. The gradual change and mutation of variables are characterized by deducing the change time, amplitude and direction in the trend components of the time series. The positions of the detected change points usually correspond to the time points when the trend term of the lake area changes in direction and magnitude. Two parameters need to be set here. The parameter h represents the minimum segment size between potentially detected breaks in the trend model, given as a fraction relative to the sample size, and breaks represents the integer of the maximum number of breaks to be calculated. Many experiments that involve setting the values of h and breaks parameters were conducted. The parameters h = 0.2 and breaks = 3 were appropriate for 35-year sequences, and the detected change points were representative of shifts in long-term series data.
3.4. Trend analysis methods
The M-K trend test (Mann Citation1945), a non-parametric method that can be applied to time-series data with various distributions and tolerates outliers and missing values, was used to determine the trends and their significance for lake area and meteorological data. Sen's slope method (Sen Citation1968) was used to analyze the change rates of lake area and meteorological data. When the Sen's slope statistic β < 0, it shows a decreasing trend. When β = 0, there is no trend. When β > 0, there is an increasing trend. When the absolute value of the M-K test statistic Z is greater than or equal to 1.96 and 2.58, it means that it has passed the significance test at the 95% and 99% confidence levels, respectively. The significance values of the change trends are provided in .
Table 2. Significance of the change trends.
4. Results and discussion
4.1. Accuracy verification for the lake area series
A direct comparison of the annual total area of lakes over 50 km² obtained in this study with the annual total lake area from the existing dataset provided by Zhang et al. (Citation2019a) is presented in . The overall temporal variation trend in the total lake area between them is consistent in most years, and the Pearson's correlation coefficient (r) is approximately 0.997. The improved lake area extraction algorithm takes advantage of the long-term series of Landsat images. There is a slight difference between the lake area results obtained in this study and the existing lake area dataset in some years, mainly attributed to the seasonal fluctuations and interpolation errors in missing values that were induced by too few original images containing lake regions ().
Figure 5. The variation trend of annual total lake area in existing datasets, total lake area in this study, the count of Landsat images containing lake and the count of lakes with void annual mean lake area.

Moreover, correlation analysis was conducted between the annual area sequence of each lake and the corresponding annual area sequence in the existing lake dataset, and the correlation coefficient of each lake is presented in . The average value of r for 180 lakes is approximately 0.88, and 144 lakes (accounting for approximately 80%) yield an r greater than 0.8. This finding indicates the relatively high accuracy of the extracted lake area. To further explore the reason for the small r value of some lakes, the ratio of the maximum to minimum area of each lake during this period was calculated based on the existing dataset (). The lakes with smaller r values mainly corresponded to those with smaller ratios of maximum to minimum area. This result suggests that when the lake area changes over a small range, some small extraction errors may lead to a reduction in the correlation coefficient.
Figure 6. The correlation coefficients between the annual lake area series extracted in this study and the existing lake dataset, as well as the ratio between the maximum and minimum lake areas in the existing lake dataset.

To further verify the accuracy of the lake area series extracted in this study, correlation analyses between the annual average area and annual average water level of each of 52 lakes from 2000 to 2017 (Li et al. Citation2019a) were performed. The average correlation coefficient of 52 lakes is approximately 0.9, among which 38 lakes (73.08%) have r ≥ 0.9, 45 lakes (86.54%) have r ≥ 0.8, and 50 lakes (96.15%) have r ≥ 0.6 (a). The results revealed that the lakes with small r values are mainly those with water level changes by less than 3 m, and the ratio of the maximum to minimum area of these lakes is also small, which are mainly distributed in the southern part of the TP. Taking Qinghai Lake, the largest lake on the TP, as an example, the annual average area and annual average water level of Qinghai Lake from 2000 to 2018 and their correlation are shown in b and c. The area results of Qinghai Lake in this study are highly consistent with the results of existing lake datasets, and the correlation coefficient with water level is as high as 0.987.
Figure 7. (a) The correlation coefficients between the annual lake area series extracted in this study and the lake water level dataset, and the range of variation in lake water level (maximum water level minus minimum water level). Taking Qinghai Lake as an example, the variation trends and correlation of annual average area and annual average water level are shown in (b) and (c), respectively.

Overall, the lake area on the TP extracted in this study is reliable, with an average correlation coefficient of approximately 0.9 with both the existing lake area and water level datasets. The existing lake datasets used in this study particularly refer to Zhang's lake area datasets, because they have been visually inspected, manually edited and fully verified, and their accuracy can be guaranteed. In Figure S3, Zhang's lake area datasets and lake water level dataset (Li et al. Citation2019a) were used as reference values to compare the lake area results obtained by the improved isobath interpolation-based lake area extraction method in this study with those obtained by the original isobath interpolation-based method (Yao et al. Citation2019). The lake area results of the two methods are highly correlated with Zhang's lake area datasets and water level dataset (all > 0.9), and the lake area results of this study are closer to Zhang's lake area datasets. This may occur because we use the existing lake area datasets (Zhang's lake area datasets) as prior data, which facilitates automatic lake area extraction, greatly improving the efficiency of the algorithm. These prior data also provide a reliable reference for the lake area, reduce abnormal lake area results extracted by automatic algorithms, and improve the lake area extraction accuracy. Moreover, this study overcomes the challenges due to cloud and atmospheric influences (Sogno, Klein, and Kuenzer Citation2022) in extracting lake areas with a high temporal resolution using Landsat images.
4.2. Multiple change points detection and segmented trend analysis
The variation trend of lake area in the TP during 1986–2020 was not monotonously increasing. To further explore the interannual variation pattern of lake areas, this study carried out multiple change points detection and segmented trend analysis for each lake area sequence.
4.2.1. Multiple change points detection
The BFAST method was applied to the area series data of each lake from 1986 to 2020. This study set a maximum of 3 change points that could be detected in each lake, and the obtained change points all passed the significance test of p ≤ 0.05. shows the distribution of the number of detected change points. The change points are mainly distributed between 1992 and 2013, and there are two peak years: 1999 and 2013. As shown in , from 1986 to 2020, the total area of 180 lakes increased from approximately 33,612.89 km² to 41,480.36 km², with a large magnitude of approximately 7,867.47 km² and a high rate of 231.40 km²/a. The lake area changes from 1986 to 1999 showed both increases and decreases, but with a small magnitude of approximately 204.62 km² and a low rate of 15.74 km²/a overall. From 1999 to 2013, the lake area consistently showed an increasing trend, with a large magnitude of approximately 5,544.15 km² and a high rate of 396.01 km²/a. The lake area from 2013 to 2020 initially showed a slight decreasing trend, and then exhibited a rapid increasing trend, with an overall magnitude of approximately 2,118.70 km² and a rate of 302.67 km²/a.
Previous studies found that there was a significant increase in lake area both after 1995 and 2015, and these two time points are often used as turning points for studying changes in lake area on the TP (Lei et al. Citation2019; Zhang et al. Citation2021b; Citation2019a). This difference might be caused by the use of the BFAST method, which determines change points based not only on the direction of the trend change but also on the magnitude of the trend change. In addition, the parameter settings of the method may cause slight differences in the results of the change points. Considering both the direction and magnitude of changes in lake area, the two peak years of the change points detected in this study are also reasonable.
4.2.2. Segmented trend analysis based on the multiple change points
Based on the detected change points in each lake area sequence, the segmented M-K test and Sen's slope methods were conducted on the annual lake area series from 1986 to 2020 for each lake. The segmented change trend of the annual lake area series of 180 lakes, classified according to the different sizes, locations, and types (endorheic/exorheic, glacier-fed/non-glacier-fed) of lakes, is presented in . According to the average lake area of each lake during 1986–2020 obtained in this study, the lakes were classified into four categories: (1) area ≥ 1000 km² (3 lakes, accounting for approximately 1.7%), (2) 500 ≤ area < 1000 km² (11 lakes, 6.1%), (3) 100 ≤ area < 500 km² (71 lakes, 39.4%), and (4) 50 ≤ area < 100 km² (95 lakes, 52.8%, including 28 lakes with an average area < 50 km², which had an area greater than 50 km² during the period of 1986–2020). The 180 lakes in this study are distributed in the northwest (westerlies domain), central (transition domain), south (Indian monsoon domain), and northeast (East Asian monsoon domain) TP, with 48, 114, 6, and 12 lakes, accounting for 26.7%, 63.3%, 3.3%, and 6.7%, respectively. In addition, based on the geomorphological features around the lakes and the sources of water supply, lakes can be divided into endorheic lakes or exorheic lakes, glacier-fed lakes or non-glacier-fed lakes (Zhang et al. Citation2021a; Zhang, Zhang, and Zhu Citation2020b). Among them, there are 132 endorheic lakes and 48 exorheic lakes, accounting for 73.3% and 26.7%, respectively. There are 114 glacier-fed lakes and 66 non-glacier-fed lakes, accounting for 63.3% and 36.7%, respectively.
Figure 9. The segmented trends of the annual lake area series of 180 lakes from 1986 to 2020. (a) the lakes classified by size, (b) the lakes classified by region, (c) the lakes classified by whether they are endorheic or exorheic, and (d) the lakes classified by whether they are glacier-fed or non-glacier-fed.

In , the horizontal axis is the lake ID, and the vertical axis is the year. The color of each column from bottom to top shows the change trend of each lake from 1986 to 2020. In a, most lakes showed an ‘SI’ trend after 2000, while lakes showed a ‘SI’, ‘SD', or ‘NS’ trend in a similar proportion before 2000. b shows the trends of lake area changes in four main regions (as shown in ). The most obvious finding is that the lakes in the southern TP generally showed a decreasing trend from 1986 to 2020. Most of the lakes with continuous ‘SD' or ‘NS’ trends from 1986 to 2020 are mainly exorheic lakes and glacier-fed lakes (c and d).
For the characteristics of different change points of each lake, to uniformly analyze the change in lakes, trend analyses of lake area in the whole period from 1986 to 2020 were carried out (a), and segmentation trend analyses were conducted according to the two peak years in 1999 and 2013 (b–d). The number of lakes with different classifications and change trends in each period are shown in .
Figure 10. The trends of lake area variation in (a) 1986–2020, (b) 1986–1999, (c) 1999–2013 and (d) 2013–2020, respectively.

Table 3. The number of lakes with different classifications and trends of changes in each period.
The lakes of different sizes showed similar variation trends during the same period (). From 1986 to 2020, most lakes (accounting for approximately 84.4%) exhibited the ‘SI’ trend regardless of the size of the lakes. From 1986 to 1999, approximately 42.2% of all lakes showed the ‘NS’ trend, and 33.9% showed the ‘SD' trend. Approximately 76.1% of all lakes showed the ‘SI’ trend from 1999 to 2013. For lakes ≥ 1000 km2 and lakes (500–1000 km2), higher proportions of the ‘SI’ trend (100% and 90.9%) were found. However, from 2013 to 2020, approximately 49.4% of all lakes showed the ‘NS’ trend, and 47.8% showed the ‘SI’ trend, with similar variation trends for different lake sizes. During 1986–2020, 1986–1999, 1999–2013 and 2013–2020, the main variation trends in lake area (≥ 50 km²) on the TP can be summarized as ‘SI’, ‘NS’ or ‘SD', ‘SI’, ‘NS’ or ‘SI’ ( and ).
For lakes in different regions, from 1986 to 2020, most lakes in the northwestern TP (westerlies domain) (approximately 91.7%), central TP (transition domain) (86.8%), and northeastern TP (East Asian monsoon domain) (66.7%) showed the ‘SI’ trend, but in the southern TP (Indian monsoon domain), they mostly (66.6%) showed the ‘SD' trend (). This finding is similar to the results of most previous studies, such as Zhang et al. (Citation2019a) and Song et al. (Citation2014). Lakes in any region mainly tended to be ‘NS’ from 1986 to 1999, with the second most common trend being ‘SD' and the least common being ‘SI’. From 1999 to 2013, most of the lakes in the northwestern TP (75.0%), central TP (78.1%), and northeastern TP (91.7%) exhibited the ‘SI’ trend, while most of the lakes in the southern TP (83.3%) showed the ‘SD' trend. From 2013 to 2020, the lakes in the northwestern TP and northeastern TP mainly showed the ‘SI’ trend (66.7% and 58.3%, respectively), followed by the ‘NS’ trend (33.3%, 41.7%). In the central TP, the lakes mainly showed the ‘NS’ trend (54.4%) and the ‘SI’ trend (41.2%). All the lakes in the southern TP presented the ‘NS’ trend. The mid-latitude westerlies, Indian monsoon, and East Asian monsoon transport water vapor to the TP (Bothe, Fraedrich, and Zhu Citation2009), and all these large-scale atmospheric circulation anomalies can influence water vapor convergence (Zhang, Zhang, and Zhu Citation2020b). Among the four regions classified in this study, the interannual variations in lake area in the northwestern and central TP exhibit the most similar characteristics. This similarity may be attributed to the strong influence of the westerlies in these two regions. The results revealed that large-scale atmospheric circulation systems, such as the westerlies, Indian monsoon, transition in between, and East Asian monsoon, might control the pattern of interannual lake area variations in different regions ( and ).
The endorheic lakes showed a larger proportion of significant changes than exorheic lakes during the same period (). During 1986–2020, approximately 91.7% of endorheic lakes exhibited the ‘SI’ trend, 64.6% of exorheic lakes showed the ‘SI’ trend and 20.8% showed the ‘NS’ trend. From 1986 to 1999, ‘SD' and ‘NS’ trends were mainly found in the endorheic lakes (38.6% and 35.6%, respectively). The exorheic lakes mainly exhibited the ‘NS’ trend (60.4%), with similar proportions of the ‘SD' (20.8%) and ‘SI’ (18.8%) trends. From 1999 to 2013, most of the endorheic lakes had the ‘SI’ trend (89.4%), while the exorheic lakes mainly presented ‘NS’ (43.8%) and ‘SI’ (39.6%) trends. From 2013 to 2020, most endorheic lakes showed ‘SI’ and ‘NS’ trends (57.6% and 38.6%, respectively); for the exorheic lakes, 79.2% exhibited the ‘NS’ trend, and 20.8% exhibited the ‘SI’ trend. These results showed that the interannual area variation patterns of the endorheic lakes are more pronounced than those of the exorheic lakes (). This finding is consistent with the results of other studies: Zhang et al. (Citation2021a) and Zhang, Zhang, and Zhu (Citation2020b) reported that the multiyear and seasonal changes in endorheic lakes are stronger than those in exorheic lakes and may be attributed to differences between the closed nature of endorheic lakes and the water inflows and outflows of exorheic lakes.
Most glacier-fed (80.7%) and non-glacier-fed (90.9%) lakes exhibited the ‘SI’ trend from 1986 to 2020 (). From 1986 to 1999, the glacier-fed lakes mainly showed ‘NS’ (41.2%) and ‘SD' (36.9%) trends. Most of the non-glacier-fed lakes had the ‘NS’ trend (43.9%), with a similar proportion of the ‘SD' (28.8%) or ‘SI’ (27.3%) trends. From 1999 to 2013, both the glacier-fed (73.7%) and non-glacier-fed lakes (80.3%) mainly presented the ‘SI’ trend. From 2013 to 2020, the glacier-fed and non-glacier-fed lakes mostly exhibited ‘SI’ (54.4% and 36.4%, respectively) or ‘NS’ (43.9%, 59.1%) trends. These results revealed that the climatic conditions in different periods may lead to differences in the melting rates of glaciers, which in turn leads to some differences in the variation trends of glacier-fed and non-glacier-fed lakes ().
4.3. Relationship between lake area variation and climatic variables
The climatic variables that affect changes in lake area mainly include air temperature, precipitation, evaporation, and the teleconnection effect of El Niño (Yeh et al. Citation2009; Zhang et al. Citation2020a). According to the classification of lakes based on size, location, and type (endorheic/exorheic, glacier-fed/non-glacier-fed), the annual average area of different classified lakes (mean lake area /km²) was calculated. The average air temperature (T/°C), average total precipitation (P /mm), average total evaporation (E /mm), and average values of total precipitation minus total evaporation (P-E /mm) within the ROIs of different classifications of lakes are presented in , respectively. lists the trends of the area variation in all lakes and different classified lakes and corresponding climatic variables during 1986–2020. The correlation coefficients between the annual lake area series and the annual series of T, P, E, and P-E of all lakes and different classifications of lakes from 1986 to 2020 are shown in .
Figure 11. The average values of lake area (mean lake area /km²), the average values of mean air temperature (T/°C), the average values of total precipitation (P /mm), the average values of total evaporation (E /mm), and the average values of total precipitation minus total evaporation (P-E /mm) of the total and different size classified lakes for each year, during 1986–2020. The yellow horizontal line represents the P-E value of 0. Additionally, the Niño 3.4 index curve from January 1985 to December 2021, and the red stripes represent El Niño years and the blue stripes represent La Niña years.

Figure 12. The average values of lake area and multiple climatic variables of the total and different region classified lakes for each year, during 1986–2020. (Refer to for others).

Figure 13. The average values of lake area and multiple climatic variables of the total and different types classified lakes for each year, during 1986–2020. (Refer to for others).

Figure 14. Correlation coefficients between mean area series of the total and each classified lakes, and climatic variables (T, P, E, and P-E), during 1986–2020. (a) All lakes and different size classified lakes. (b) All lakes and different region classified lakes. (c) All lakes and different types classified lakes.

Table 4. The variation trends of the area of all lakes and different classified lakes and corresponding climatic variables during 1986–2020.
From 1986 to 2020, the mean area of all lakes increased highly significantly ( and ). Moreover, the corresponding climatic variables (mainly T, P, E, and P-E) of all lakes also showed a significant increasing trend. The correlation between the lake area and E is the strongest (r ≈ 0.61), followed by P (r ≈ 0.39), T (r ≈ 0.38), and P-E (r ≈ 0.22), as shown in . Evaporation usually has a negative effect on the lake area, but the overall lake area is positively correlated with evaporation. The possible reason is that the change in lake area may be dominated by other factors and less influenced by evaporation, while the change in evaporation is more influenced by the lake area. The increase or decrease in lake area has an effect on the increase or decrease in E in the lake ROI, so the two are mostly positively correlated. Thus, E is not considered the main factor affecting the change in lake area in the subsequent study. For all lakes, the main climatic variables affecting lake area change are precipitation and air temperature. The effect of precipitation on the lake area is mainly through direct precipitation in the lake region and inflows of surface runoff. Additionally, air temperature usually affects the change in lake area by influencing summer precipitation, glacier/snow melting, permafrost degradation, and then changing runoff into lakes (Zhang et al. Citation2020a). In El Niño years, precipitation tends to decrease, and the lake area tends to decrease or slowly increase. In La Niña years, precipitation tends to increase, and the lake area tends to increase (). After approximately 1995, the values of P-E within the lake ROIs were nearly all positive, and the lake area showed an upward trend from 1995. This result indicates that the positive accumulation of P-E promotes the expansion of lakes.
The interannual variation in the lake area exhibited a similar increasing trend in lakes of different sizes from 1986 to 2020 ( and ). The larger the lakes are, the larger the increasing amplitude is. Among them, the area variation trend of the lakes (100–500 km2) is highly consistent with that of the overall lakes. The climatic variables corresponding to different size classes of lakes all showed significant or nonsignificant increasing trends from 1986 to 2020. As shown in a, among the correlation coefficients between the area of lakes (≥ 1000 km²) and climatic variables, the correlation coefficient with T is the highest (0.52), followed by E (0.51), P (0.13) and P-E (−0.01). For the lakes with areas of 500–1000 km2 and 100–500 km2, the correlation coefficients between lake area and E were the highest (0.48 and 0.61, respectively), followed by T (0.45, 0.39), P (0.42, 0.36) and P-E (0.28, 0.19). The area of lakes (50–100 km2) had the highest correlation coefficient with E (0.62), followed by P (0.44), T (0.35) and P-E (0.27). This finding indicates that the main climatic variable that has a great effect on the area change in the large lake (>100 km²) is air temperature, followed by precipitation. Precipitation is the main climatic variable affecting the area change in small lakes (50–100 km2), followed by air temperature. A more notable feature is that the larger the lake is, the greater the correlation between its area and T is, possibly because air temperature changes have a more significant influence on lakes with larger areas. The underlying reason may be that larger lakes have larger lake basins and reach peaks later than small lakes (Zhang, Zhang, and Zhu Citation2020b). Therefore, when the temperature rises by a certain degree, the area growth of large lakes is greater and more significant than that of small lakes.
The interannual variations in lake area of the southern TP (Indian monsoon domain) and northeastern TP (East Asian monsoon domain) lakes are quite different from those of all lakes. From 1986 to 2020, the lake areas of the northeastern TP had large amplitudes of changes, and those of the southern TP even showed a highly significant decreasing trend ( and ). The climatic variables corresponding to different location-classified lakes all showed significant or nonsignificant increasing trends from 1986 to 2020. For the lakes in the southern TP, T, P, E, and P-E had relatively high values, but negative correlations with the annual mean lake area were found at −0.28, −0.08, −0.18 and −0.03, respectively (b). Based on the lake water balance, the decrease in lake area in the southern TP may be attributed to the excessive outflows of surface and subsurface runoff. Chen et al. (Citation2021) and Liu et al. (Citation2023b) reported that the shrinkage of lakes in the southern TP is related to water leakage at the lake bottom and the existence of leakage channels in the rift zones. From the perspective of remote sensing big data analysis, this study provides indirect evidence that the decrease of lake area in the southern TP may not be driven by climate, but is probably related to the leakage of the lake bottom. In the northwestern TP (westerlies domain), the correlation coefficient between the area and E is the highest (0.54), followed by P (0.45), T (0.29) and P-E (0.28). Similarly, the correlation coefficient between the area and E is the highest (0.62) in the Central TP (Transition domain), followed by T (0.37), P (0.35) and P-E (0.18). In the northeastern TP (East Asian monsoon domain), among the correlation coefficients between the area and climatic variables, P is the highest (0.31), followed by E and P-E (both 0.24) and T (0.2). These results indicate that the lakes in the northwestern TP and northeastern TP, where the westerlies or East Asian summer monsoon bring abundant precipitation, may be influenced more by precipitation than by other climatic variables. Additionally, the lakes in the Central TP, where the westerlies and Indian monsoon co-occur, may be mainly affected by air temperature and precipitation at the same time.
During 1986–2020, the interannual variations in lake area of endorheic and exorheic lakes all exhibited increasing trends, while the amplitude of the area changes in endorheic lakes was larger than that in exorheic lakes. The corresponding climatic variables in the endorheic and exorheic lakes showed similar significant increasing trends ( and ). For the endorheic lakes, the correlation coefficient between the area and E is the highest (0.62), followed by T and P (both 0.37) and P-E (0.19). Similarly, the correlation coefficient between the area and E is the highest (0.61) of the exorheic lakes, followed by P (0.55), P-E (0.43) and T (0.31) (c). The results reveal that in the long-term interannual variation in lake area, endorheic lakes are mainly affected by precipitation and air temperature, while exorheic lakes are mainly affected by precipitation. The area of exorheic lakes is more related to precipitation than that of endorheic lakes, but the changes in endorheic lakes are stronger than those in exorheic lakes; that is, the amplitudes of changes are larger. This result is attributed to the difference between the natural characteristics (e.g. whether there is water flowing out) of the endorheic lakes and the exorheic lakes (Zhang et al. Citation2021a; Zhang, Zhang, and Zhu Citation2020b). There are no outflows of surface runoff in the endorheic lakes, and the inflows mainly caused by rivers, precipitation and glacier/snow meltwaters can be conserved. However, exorheic lakes have outflows of surface runoff; even if the inflows are extremely large, they will be discharged, so the changes in lake area are not strong.
The glacier-fed lakes and non-glacier-fed lakes presented similar increasing trends in interannual areas from 1986 to 2020. The amplitude of the area changes was larger for glacier-fed lakes than non-glacier-fed lakes. The climatic variables corresponding to glacier-fed lakes and non-glacier-fed lakes all showed significant or nonsignificant increasing trends ( and ). The annual mean area of the glacier-fed lakes is generally higher than that of the non-glacier-fed lakes, while all the corresponding climatic variables of the non-glacier-fed lakes are generally higher than those of the glacier-fed lakes. Among the correlation coefficients between lake area and climatic variables in both glacier-fed and non-glacier-fed lakes, the correlation coefficients between lake area and E are the highest (0.57 and 0.67, respectively), followed by P (0.39, 0.40), T (0.38, 0.34) and P-E (0.24, 0.21), as shown in c. This finding indicates that the areas of both glacier-fed and non-glacier-fed lakes are mainly affected by precipitation, followed by air temperature. The influence of air temperature on glacier-fed lakes is greater than that on non-glacier-fed lakes because of its effect on glacier melting.
5. Conclusions
This study proposed an improved lake area extraction method based on isobath interpolation, which can effectively extract the complete lake area from optical remote sensing images, particularly when the lake is partly contaminated by cloud cover. The lake area extraction method primarily improves the acquisition scheme for isobaths and their accuracy, especially for complex lake conditions. More complete and accurate isobaths were obtained as prior information for the subsequent automatic extraction procedure. The proposed method effectively solves the cloud contamination problem and improves data utilization, calculation efficiency and accuracy. The annual results of lake area obtained in this study are conducive to the highly refined analysis of their change trends.
The interannual area series from 1986 to 2020 for lakes ≥ 50 km² on the TP were obtained using the proposed extraction method based on Landsat big data mining, along with the change points and segmented trends for each lake. Overall, most of the lakes on the TP showed significant increasing trends (84.4%) from 1986 to 2020. During 1986–1999, most of the lakes exhibited no significant change (42.2%) or significant decreasing trends (33.9%), while during 1999–2013, most of the lakes showed significant increasing trends (76.1%). From 2013 to 2020, most of the lakes exhibited no significant change (49.4%) or significant increasing trends (47.8%).
Combined with meteorological data for preliminary qualitative analysis, it was found that the corresponding climatic variables (mainly T, P, E, and P-E) of all lakes and different classified lakes showed a significant or nonsignificant increasing trend. Precipitation and air temperature are the two main climatic variables affecting interannual variations in lake area. El Niño and La Niña events affect precipitation on the TP, thereby influencing lake area changes. The overall expansion trend of lake area after 1995 can be attributed to the positive accumulation of P-E. There is a slight difference in the influence of climatic variables on lake area changes in different sizes, regions and types (endorheic/exorheic, glacier-fed/non-glacier-fed) on the TP. The areas of larger lakes (>100 km²) are more significantly affected by air temperature than those of smaller lakes (50–100 km2). The large-scale atmospheric circulation systems acting on different regions, such as the westerlies, Indian monsoon, transition in between and East Asian monsoon, were found to affect the interannual variation pattern of lake area. Importantly, the decrease in lake area within the southern TP may not be climate-driven but may be related to leakage from the lake bottom. The interannual area of the exorheic lakes is more related to precipitation than that of the endorheic lakes, but the interannual variations in the area of the endorheic lakes are stronger than those of the exorheic lakes. Glacier-fed lakes are more affected by air temperature than non-glacier-fed lakes, along with stronger interannual area variations.
In summary, this study provides a new method, data, and characteristic results for research on the interannual variations in lake areas on the TP, which can be used by research communities and various hydrological and water resource management departments for practical applications. The limitations of the method proposed in this study are that the isobaths need to be preprocessed, and the isobaths need to be updated if the study time range is extended, especially the isobaths corresponding to the maximum and minimum lake areas. In the future, it is necessary to further improve the isobath extraction method and fully utilize the existing high-precision lake area datasets and multisource remote sensing big data. More high-precision isobaths need to be obtained to further study the seasonal variations in lake area on the TP. Additionally, the relative contributions of various climate variables to the lake area need to be more precisely assessed through in-depth water balance analysis.
Supplemental Material
Download MS Word (14 MB)Acknowledgements
The authors would like to acknowledge all the scientists and engineers who contributed data at the United States Geological Survey, the National Tibetan Plateau Data Center, the European Centre for Medium-Range Weather Forecasts, and the Climate Prediction Center of the National Oceanic and Atmospheric Administration. The authors also thank Google for providing free access to the Google Earth Engine platform and Esri for providing the service of World Hillshade basemap, and finally, thank anonymous reviewers and the editors for supporting this work.
Disclosure statement
No potential conflict of interest was reported by the author(s).
Data availability statement
The key codes for extracting lake area in this study are available to interested users: https://code.earthengine.google.com/17ce545ea551f0933587100715e9921d, https://code.earthengine.google.com/b9276a3fc78bfb8783ef38c8aaa049c9. The lake area dataset produced in this study was publicly published on the National Tibetan Plateau Data Center (https://doi.org/10.11888/Terre.tpdc.300883).
Additional information
Funding
References
- Bothe, Oliver, Klaus Fraedrich, and Xiuhua Zhu. 2009. “The Large-Scale Circulations and Summer Drought and Wetness on the Tibetan Plateau.” International Journal of Climatology 30 (6): 844–855. https://doi.org/10.1002/joc.1946.
- Cai, Yu, Chang-Qing Ke, Xingong Li, Guoqing Zhang, Zheng Duan, and Hoonyol Lee. 2019. “Variations of Lake Ice Phenology on the Tibetan Plateau from 2001 to 2017 Based on MODIS Data.” Journal of Geophysical Research: Atmospheres 124 (2): 825–843. https://doi.org/10.1029/2018JD028993.
- Chen, Xuegao, Zhongbo Yu, Qinghan Huang, Peng Yi, Xiaonan Shi, Ala Aldahan, Ling Xiong, Chengwei Wan, and Peng Chen. 2021. “Evaluating the Water Level Variation of a High-Altitude Lake in Response to Environmental Changes on the Southern Tibetan Plateau.” Journal of Hydrologic Engineering 26 (5), https://doi.org/10.1061/(ASCE)HE.1943-5584.0002050.
- Crétaux, J.-F., A. Arsen, Stéphane Calmant, A. Kouraev, V. Vuglinski, M. Bergé-Nguyen, M.-C. Gennero, Fernando Nino, R. Abarca Del Rio, and A. Cazenave. 2011. “SOLS: A Lake Database to Monitor in the Near Real Time Water Level and Storage Variations from Remote Sensing Data.” Advances in Space Research 47 (9): 1497–1507. https://doi.org/10.1016/j.asr.2011.01.004.
- Du, Jinkang, Yongsheng Huang, Xuezhi Feng, and Zhoulong Wang. 2001. “Study on Water Bodies Extraction and Classification from SPOT Image.” Journal of Remote Sensing 5 (3): 219–225. https://doi.org/10.3321/j.issn:1007-4619.2001.03.009.
- Farr, Tom G., Paul A. Rosen, Edward Caro, Robert Crippen, Riley Duren, Scott Hensley, Michael Kobrick, et al. 2007. “The Shuttle Radar Topography Mission.” Reviews of Geophysics 45 (2), https://doi.org/10.1029/2005RG000183.
- Fisher, Ronald A. 1936. “The Use of Multiple Measurements in Taxonomic Problems.” Annals of Eugenics 7 (2): 179–188. https://doi.org/10.1111/j.1469-1809.1936.tb02137.x.
- Foga, Steve, Pat L. Scaramuzza, Song Guo, Zhe Zhu, Ronald D. Dilley, Jr., Tim Beckmann, Gail L. Schmidt, John L. Dwyer, M. Joseph Hughes, and Brady Laue. 2017. “Cloud Detection Algorithm Comparison and Validation for Operational Landsat Data Products.” Remote Sensing of Environment 194: 379–390. https://doi.org/10.1016/j.rse.2017.03.026.
- Geng, Liying, Tao Che, Xufeng Wang, and Haibo Wang. 2019. “Detecting Spatiotemporal Changes in Vegetation with the BFAST Model in the Qilian Mountain Region During 2000–2017.” Remote Sensing 11 (2): 103. https://doi.org/10.3390/rs11020103.
- GISTEMP, Team. 2019. “GISS Surface Temperature Analysis (GISTEMP), Version 4.” NASA Goddard Institute for Space Studies, Dataset Available at: https://Data.Giss.Nasa.Gov/Gistemp/, Last Access 6.
- Jupp, David LB, K. K. Mayo, D. A. Kucher, S. J. Heggen, S. W. Kendall, B. M. Radke, and Tony Ayling. 1985. Landsat Based Interpretation of the Cairns Section of the Great Barrier Reef Marine Park. Canberra, ACT: CSIRO Division of Water & Land Resources.
- Kang, Shichang, Yanwei Xu, Qinglong You, Wolfgang-Albert Flügel, Nick Pepin, and Tandong Yao. 2010. “Review of Climate and Cryospheric Change in the Tibetan Plateau.” Environmental Research Letters 5 (1): 015101. https://doi.org/10.1088/1748-9326/5/1/015101.
- Kass, Michael, Andrew Witkin, and Demetri Terzopoulos. 1988. “Snakes: Active Contour Models.” International Journal of Computer Vision 1 (4): 321–331. https://doi.org/10.1007/BF00133570.
- Lei, Yanbin, Kun Yang, Bin Wang, Yongwei Sheng, Broxton W. Bird, Guoqing Zhang, and Lide Tian. 2014. “Response of Inland Lake Dynamics Over the Tibetan Plateau to Climate Change.” Climatic Change 125 (2): 281–290. https://doi.org/10.1007/s10584-014-1175-3.
- Lei, Yanbin, Yali Zhu, Bin Wang, Tandong Yao, Kun Yang, Xiaowen Zhang, Jianqing Zhai, and Ning Ma. 2019. “Extreme Lake Level Changes on the Tibetan Plateau Associated with the 2015/2016 El Niño.” Geophysical Research Letters 46 (11): 5889–5898. https://doi.org/10.1029/2019GL081946.
- Li, Xingdong, Di Long, Qi Huang, Pengfei Han, Fanyu Zhao, and Yoshihide Wada. 2019a. “High-Temporal-Resolution Water Level and Storage Change Data Sets for Lakes on the Tibetan Plateau During 2000–2017 Using Multiple Altimetric Missions and Landsat-Derived Lake Shoreline Positions.” Earth System Science Data 11 (4): 1603–1627. https://doi.org/10.5194/essd-11-1603-2019.
- Li, Lanhui, Yili Zhang, Qionghuan Liu, Mingjun Ding, and Partho Protim Mondal. 2019b. “Regional Differences in Shifts of Temperature Trends Across China between 1980 and 2017.” International Journal of Climatology 39 (3): 1157–1165. https://doi.org/10.1002/joc.5868.
- Liu, Xiangmei, Jiaqi Chen, Jiansheng Chen, and Haixia Jin. 2023b. “Shrinking Lakes of Rift Valley System in Southern Tibet: Is It the Climate?” Science of The Total Environment 858: 160016. https://doi.org/10.1016/j.scitotenv.2022.160016.
- Liu, H., and K. C. Jezek. 2004. “Automated Extraction of Coastline from Satellite Imagery by Integrating Canny Edge Detection and Locally Adaptive Thresholding Methods.” International Journal of Remote Sensing 25 (5): 937–958. https://doi.org/10.1080/0143116031000139890.
- Liu, Jianwei, Yuyan Zhou, Fan Lu, Yuanhui Yu, Denghua Yan, Yingying Hu, and Wei Xue. 2023a. “Evaluating Satellite- and Reanalysis-Based Precipitation Products Over the Qinghai-Tibetan Plateau in the Perspective of a New Error-Index System.” International Journal of Climatology 43 (5): 2200–2219. https://doi.org/10.1002/joc.7970.
- Lu, Shanlong, Li Jia, Lei Zhang, Yongping Wei, Muhammad Hasan Ali Baig, Zhaokun Zhai, Jihua Meng, Xiaosong Li, and Guifang Zhang. 2017. “Lake Water Surface Mapping in the Tibetan Plateau Using the MODIS MOD09Q1 Product.” Remote Sensing Letters 8 (3): 224–233. https://doi.org/10.1080/2150704X.2016.1260178.
- Mann, Henry B. 1945. “Nonparametric Tests Against Trend.” Econometrica: Journal of the Econometric Society, 245–259. https://doi.org/10.2307/1907187.
- Maussion, Fabien, Dieter Scherer, Thomas Mölg, Emily Collier, Julia Curio, and Roman Finkelnburg. 2014. “Precipitation Seasonality and Variability Over the Tibetan Plateau as Resolved by the High Asia Reanalysis.” Journal of Climate 27 (5): 1910–1927. https://doi.org/10.1175/JCLI-D-13-00282.1.
- McFeeters, Stuart K. 1996. “The Use of the Normalized Difference Water Index (NDWI) in the Delineation of Open Water Features.” International Journal of Remote Sensing 17 (7): 1425–1432. https://doi.org/10.1080/01431169608948714.
- Muñoz Sabater, J. 2019. “ERA5-Land Monthly Averaged Data from 1981 to Present, Copernicus Climate Change Service (C3S).” Climate Data Store (CDS). [1986–2020], https://doi.org/10.24381/cds.68d2bb30.
- Otsu, Nobuyuki. 1979. “A Threshold Selection Method from Gray-Level Histograms.” IEEE Transactions on Systems, Man, and Cybernetics 9 (1): 62–66. https://doi.org/10.1109/TSMC.1979.4310076.
- Pekel, Jean-François, Andrew Cottam, Noel Gorelick, and Alan S. Belward. 2016. “High-Resolution Mapping of Global Surface Water and Its Long-Term Changes.” Nature 540 (7633): 418–422. https://doi.org/10.1038/nature20584.
- Qiu, Jane. 2008. “China: The Third Pole.” Nature 454 (7203): 393–397. https://doi.org/10.1038/454393a.
- Sen, Pranab Kumar. 1968. “Estimates of the Regression Coefficient Based on Kendall’s Tau.” Journal of the American Statistical Association 63 (324): 1379–1389. https://doi.org/10.1080/01621459.1968.10480934.
- Setiawan, Budi Darma, Alfi Nur Rusydi, and Koko Pradityo. 2017. “Lake Edge Detection Using Canny Algorithm and Otsu Thresholding.” IEEE International symposium on geoinformatics (ISyG), 72–76. https://doi.org/10.1109/ISYG.2017.8280676.
- Shen, Jinxiang, Liao Yang, Xi Chen, Junli Li, Qingqing Peng, and Ju Hu. 2012. “A Method for Object-Oriented Automatic Extraction of Lakes in the Mountain Area from Remote Sensing Image.” Remote Sensing for Land & Resources 24 (3): 84–91.
- Singh, Kanwar Vivek, Raj Setia, Shashikanta Sahoo, Avinash Prasad, and Brijendra Pateriya. 2015. “Evaluation of NDWI and MNDWI for Assessment of Waterlogging by Integrating Digital Elevation Model and Groundwater Level.” Geocarto International 30 (6): 650–661. https://doi.org/10.1080/10106049.2014.965757.
- Sogno, Patrick, Igor Klein, and Claudia Kuenzer. 2022. “Remote Sensing of Surface Water Dynamics in the Context of Global Change—A Review.” Remote Sensing 14 (10): Multidisciplinary Digital Publishing Institute: 2475. https://doi.org/10.3390/rs14102475.
- Song, Chunqiao, Bo Huang, Keith Richards, Linghong Ke, and Vu Hien Phan. 2014. “Accelerated Lake Expansion on the Tibetan Plateau in the 2000s: Induced by Glacial Melting or Other Processes?” Water Resources Research 50 (4): 3170–3186. https://doi.org/10.1002/2013WR014724.
- Trenberth, Kevin E. 1997. “The Definition of El Nino.” Bulletin of the American Meteorological Society 78 (12): 2771–2778. https://doi.org/10.1175/1520-0477(1997)078<2771:TDOENO>2.0.CO;2.
- USGS. 2019a. “Landsat 8 Surface Reflectance Code (LASRC) Product Guide.” General Technical Report LSDS-1368 Version 2.0.
- USGS. 2019b. “Landsat 4-7 Surface Reflectance (Ledaps) Product Guide.” EROS Sioux Falls, SD, USA.
- Verbesselt, Jan, Rob Hyndman, Glenn Newnham, and Darius Culvenor. 2010. “Detecting Trend and Seasonal Changes in Satellite Image Time Series.” Remote Sensing of Environment 114 (1): 106–115. https://doi.org/10.1016/j.rse.2009.08.014.
- Wang, Binbin, Yaoming Ma, Zhongbo Su, Yan Wang, and Weiqiang Ma. 2020. “Quantifying the Evaporation Amounts of 75 High-Elevation Large Dimictic Lakes on the Tibetan Plateau.” Science Advances 6 (26): eaay8558. https://doi.org/10.1126/sciadv.aay8558.
- Wangchuk, Sonam, and Tobias Bolch. 2020. “Mapping of Glacial Lakes Using Sentinel-1 and Sentinel-2 Data and a Random Forest Classifier: Strengths and Challenges.” Science of Remote Sensing 2: 100008. https://doi.org/10.1016/j.srs.2020.100008.
- Wu, Xiaojing, Jianbin Su, Weiwei Ren, Haishen Lü, and Fei Yuan. 2023. “Statistical Comparison and Hydrological Utility Evaluation of ERA5-Land and IMERG Precipitation Products on the Tibetan Plateau.” Journal of Hydrology 620: 129384. https://doi.org/10.1016/j.jhydrol.2023.129384.
- Xu, Hanqiu. 2006. “Modification of Normalised Difference Water Index (NDWI) to Enhance Open Water Features in Remotely Sensed Imagery.” International Journal of Remote Sensing 27 (14): 3025–3033. https://doi.org/10.1080/01431160600589179.
- Xu, Jintao, Ziqiang Ma, Songkun Yan, and Jie Peng. 2022. “Do ERA5 and ERA5-Land Precipitation Estimates Outperform Satellite-Based Precipitation Products? A Comprehensive Comparison between State-of-the-Art Model-Based and Satellite-Based Precipitation Products Over Mainland China.” Journal of Hydrology 605: 127353. https://doi.org/10.1016/j.jhydrol.2021.127353.
- Yang, Ruimin, Liping Zhu, Junbo Wang, Jianting Ju, Qingfeng Ma, Falko Turner, and Yun Guo. 2017. “Spatiotemporal Variations in Volume of Closed Lakes on the Tibetan Plateau and Their Climatic Responses from 1976 to 2013.” Climatic Change 140: 621–633. https://doi.org/10.1007/s10584-016-1877-9.
- Yao, Tandong, Tobias Bolch, Deliang Chen, Jing Gao, Walter Immerzeel, Shilong Piao, Fengge Su, et al. 2022. “The Imbalance of the Asian Water Tower.” Nature Reviews Earth & Environment 3 (10): 618–632. https://doi.org/10.1038/s43017-022-00299-4.
- Yao, Tandong, Valerie Masson-Delmotte, Jing Gao, Wusheng Yu, Xiaoxin Yang, Camille Risi, Christophe Sturm, Martin Werner, Huabiao Zhao, and You He. 2013. “A Review of Climatic Controls on δ18O in Precipitation Over the Tibetan Plateau: Observations and Simulations.” Reviews of Geophysics 51 (4): 525–548. https://doi.org/10.1002/rog.20023.
- Yao, Fangfang, Jida Wang, Chao Wang, and Jean-François Crétaux. 2019. “Constructing Long-Term High-Frequency Time Series of Global Lake and Reservoir Areas Using Landsat Imagery.” Remote Sensing of Environment 232: 111210. https://doi.org/10.1016/j.rse.2019.111210.
- Yeh, Sang-Wook, Jong-Seong Kug, Boris Dewitte, Min-Ho Kwon, Ben P. Kirtman, and Fei-Fei Jin. 2009. “El Niño in a Changing Climate.” Nature 461 (7263): 511–514. https://doi.org/10.1038/nature08316.
- Zeileis, Achim. 2005. “A Unified Approach to Structural Change Tests Based on ML Scores, F Statistics, and OLS Residuals.” Econometric Reviews 24 (4): 445–466. https://doi.org/10.1080/07474930500406053.
- Zhang, Guoqing, Tobias Bolch, Wenfeng Chen, and Jean-François Crétaux. 2021a. “Comprehensive Estimation of Lake Volume Changes on the Tibetan Plateau During 1976–2019 and Basin-Wide Glacier Contribution.” Science of the Total Environment 772: 145463. https://doi.org/10.1016/j.scitotenv.2021.145463.
- Zhang, Guoqing, Wei Luo, Wenfeng Chen, and Guoxiong Zheng. 2019a. “A Robust but Variable Lake Expansion on the Tibetan Plateau.” Science Bulletin 64 (18): 1306–1309. https://doi.org/10.1016/j.scib.2019.07.018.
- Zhang, Guoqing, Youhua Ran, Wei Wan, Wei Luo, Wenfeng Chen, Fenglin Xu, and Xin Li. 2021b. “100 Years of Lake Evolution Over the Qinghai–Tibet Plateau.” Earth System Science Data 13 (8): 3951–3966. https://doi.org/10.5194/essd-13-3951-2021.
- Zhang, Guoqing, Tandong Yao, Wenfeng Chen, Guoxiong Zheng, C. K. Shum, Kun Yang, Shilong Piao, Yongwei Sheng, Shuang Yi, and Junli Li. 2019b. “Regional Differences of Lake Evolution Across China During 1960s–2015 and Its Natural and Anthropogenic Causes.” Remote Sensing of Environment 221: 386–404. https://doi.org/10.1016/j.rse.2018.11.038.
- Zhang, Guoqing, Tandong Yao, Shilong Piao, Tobias Bolch, Hongjie Xie, Deliang Chen, Yanhong Gao, Catherine M. O’Reilly, C. K. Shum, and Kun Yang. 2017a. “Extensive and Drastically Different Alpine Lake Changes on Asia’s High Plateaus During the Past Four Decades.” Geophysical Research Letters 44 (1): 252–260. https://doi.org/10.1002/2016GL072033.
- Zhang, Guoqing, Tandong Yao, Hongjie Xie, Shichang Kang, and Yanbin Lei. 2013. “Increased Mass Over the Tibetan Plateau: From Lakes or Glaciers?” Geophysical Research Letters 40 (10): 2125–2130. https://doi.org/10.1002/grl.50462.
- Zhang, Guoqing, Tandong Yao, Hongjie Xie, Jun Qin, Qinghua Ye, Yufeng Dai, and Ruifang Guo. 2014a. “Estimating Surface Temperature Changes of Lakes in the Tibetan Plateau Using MODIS LST Data.” Journal of Geophysical Research: Atmospheres 119 (14): 8552–8567. https://doi.org/10.1002/2014JD021615.
- Zhang, Guoqing, Tandong Yao, Hongjie Xie, Kun Yang, Liping Zhu, C. K. Shum, Tobias Bolch, Shuang Yi, Simon Allen, and Liguang Jiang. 2020a. “Response of Tibetan Plateau Lakes to Climate Change: Trends, Patterns, and Mechanisms.” Earth-Science Reviews 208: 103269. https://doi.org/10.1016/j.earscirev.2020.103269.
- Zhang, Guoqing, Tandong Yao, Hongjie Xie, Kexiang Zhang, and Fujing Zhu. 2014b. “Lakes’ State and Abundance Across the Tibetan Plateau.” Chinese Science Bulletin 59 (24): 3010–3021. https://doi.org/10.1007/s11434-014-0258-x.
- Zhang, Yu, Guoqing Zhang, and Tingting Zhu. 2020b. “Seasonal Cycles of Lakes on the Tibetan Plateau Detected by Sentinel-1 SAR Data.” Science of the Total Environment 703: 135563. https://doi.org/10.1016/j.scitotenv.2019.135563.
- Zhang, Guoqing, Guoxiong Zheng, Yang Gao, Yang Xiang, Yanbin Lei, and Junli Li. 2017b. “Automated Water Classification in the Tibetan Plateau Using Chinese GF-1 WFV Data.” Photogrammetric Engineering & Remote Sensing 83 (7): 509–519. https://doi.org/10.14358/PERS.83.7.509.
- Zhao, Rui, Ping Fu, Yan Zhou, Xiangming Xiao, Stephen Grebby, Guoqing Zhang, and Jinwei Dong. 2022. “Annual 30-m Big Lake Maps of the Tibetan Plateau in 1991–2018.” Scientific Data 9 (1): 164. https://doi.org/10.1038/s41597-022-01275-9.
- Zhao, Gang, and Huilin Gao. 2018. “Automatic Correction of Contaminated Images for Assessment of Reservoir Surface Area Dynamics.” Geophysical Research Letters 45 (12): 6092–6099. https://doi.org/10.1029/2018GL078343.
- Zhou, Chenghu, Yunyan Du, and Jiancheng Luo. 1996. “A Description Model Based on Knowledge for Automatically Recognizing Water from NOAA/AVHRR.” Journal of Natural Disasters 5 (3): 100–108.
- Zhu, Liping, Jianting Ju, Baojin Qiao, Ruimin Yang, Chong Liu, and Boping Han. 2019. “Recent Lake Changes of the Asia Water Tower and Their Climate Response: Progress, Problems and Prospects.” AGU Fall Meeting Abstracts 2019: GC51P–G1017. https://doi.org/10.1360/TB-2019-0185.