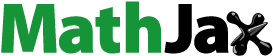
ABSTRACT
Vegetation phenological metrics have attracted increasing attentions during recent years. However, compared with the peak greenness timing (PGT), the peak photosynthesis timing (PPT) has been overlooked until now. As the world's third pole, the Tibetan Plateau is one of the most sensitive areas to global climatic change. In this study, long-term time-series solar-induced chlorophyll fluorescence (SIF) data were used to identify the PPT patterns, trends, and responses in the Tibetan Plateau over the past two decades. Our findings indicated that the SIF-derived PPT can better capture the characteristics from flux-measured gross primary productivity compared with the MODIS EVI-based PGT, exhibiting great potential to characterize large-scale vegetation PPT dynamics. The multi-year mean PPT mainly appeared in late July and early August with a gradually delayed trend from southeast to northwest. Meanwhile, early PPT occurred in many areas of the Tibetan Plateau (67.36%), with the overall trend advanced by about 0.89 day decade−1. Interestingly, opposite trends of PPT were found with elevation below and above 2500 m asl. Preseason temperature, shortwave radiation, and precipitation jointly explained the strong spatial heterogeneity of PPT trend. All analyses help clarify the role of the ‘third pole’ in responding to environmental change and regional carbon cycles.
1. Introduction
Vegetation phenology refers to the annually recurring stages of vegetation development affected by climatic and other environmental factors, including the start, end, and length of the growing season. Shifts in vegetation phenology indicate the adaptation of vegetation to climate change, which can exert a substantial influence on terrestrial carbon, water cycles, and energy flow (Richardson et al. Citation2013). Therefore, a thorough understanding of the spatiotemporal dynamics of vegetation phenology and its response mechanism to global climate change is helpful to evaluate the terrestrial carbon sequestration potential (Berra and Gaulton Citation2021; Piao et al. Citation2019).
Traditionally, the observation of plant phenology relies on manual records in the field, providing long-term and accurate information on individual plant species (Zeng et al. Citation2020). Several observation networks have been established in parts of the Earth such as the USA National Phenology Network (Crimmins Citation2021), the Pan European Phenology Network (Templ et al. Citation2018), and the Chinese Phenological Observation Network (Wu et al. Citation2022). With the development of the Internet of Things in recent years, digital cameras as near-ground remote sensing sensors can track seasonal transitions of vegetation growth automatically and continuously with high frequency; thus, they have significant advantages in small-scale vegetation phenology observations. At present, a regional and global phenological camera network (the PhenoCam network) has been formed (Katal et al. Citation2022). However, the representativeness of such observations remains limited and spatially sparse, thereby constraining our understanding of spatial patterns in vegetation phenology.
Satellite-based remote sensing provides a feasible way to monitor vegetation dynamics at different spatial and temporal scales. A series of vegetation indices (VIs) that reflect the growth information via canopy greenness, such as the normalized difference vegetation index (NDVI), has been widely used to monitor phenological parameters. Several studies used MODIS-based VIs to map the peak photosynthesis timing (PPT) from regional (Wang and Wu Citation2019) to global scales (Gonsamo, Chen, and Ooi Citation2018). However, the discrepancy between these phenological metrics derived from greenness-based VIs and vegetation photosynthetic activity is large, such as the lag between the start of photosynthesis and leaf emergence, which reflects the shifts in canopy structure and ecosystem function, respectively (Jeong et al. Citation2017; Li et al. Citation2023).
The photosynthetic phenology indicates temporal dynamics of vegetation photosynthetic intensity, and it can be captured from time-series gross primary productivity (GPP) data. At the site level, GPP can be partitioned from flux-measured net ecosystem carbon exchange (NEE) based on the eddy covariance (EC) technique. At present, the global network of EC flux towers (FLUXNET) has more than 1000 sites across various ecosystems (Chu et al. Citation2017). Under the support of remote sensing data and ecosystem models, several satellite-based GPP products including MODIS (Running et al. Citation2004), BESS (Jiang and Ryu Citation2016), and VPM (Xiao et al. Citation2004) have been developed with long-term records, which provide a method to characterize vegetation photosynthetic activity. Although Yang et al. (Citation2019) have used VPM-based GPP product to monitor PPT of temperate and alpine grasslands in China, great uncertainty remains in the accuracy of relevant satellite-based GPP products due to the algorithm, model structure, and input data, which restrict the phenological metrics of canopy photosynthesis (Wang et al. Citation2020). The validation results from FLUXNET showed that remote sensing-based GPP models can explain about 70% (53–78%) of site-level GPP changes (Lin et al. Citation2021).
Solar-induced chlorophyll fluorescence (SIF), as a proxy for vegetation photosynthesis, has been proven to be strongly correlated with GPP (Wang et al. Citation2023). Previous studies have shown that the SIF-derived photosynthetic phenology metrics are generally closer to phenology metrics extracted from flux-measured GPP than VI-based greenness phenology metrics (Liu et al. Citation2018; Zhang et al. Citation2020). In recent years, the emergence of global-scale SIF products with high temporal and spatial resolution based on discrete SIF soundings from satellites, such as OCO-2 and auxiliary data sets (Li and Xiao Citation2019; Zhang et al. Citation2018), has provided new insight into the extraction of large-scale photosynthetic phenology, which is significantly different from the conventional greenness phenology (Zhang et al. Citation2019). Previous studies about SIF-derived vegetation phenology generally focused on the start and end of growing season (Chang et al. Citation2019; Meng et al. Citation2021), whereas limited attention has been paid to PPT. As the change point of vegetation growth shifting from prosperity to decline, PPT represents the timing of maximum resource availability of vegetation and is closely linked with the transition stage in annual carbon uptake (Wang et al. Citation2020). Its variations including advance and delay are the response of vegetation to preseason hydrothermal conditions (preseason refers to the period prior to the timing of the phenological event) and will further affect ecosystem functions such as carbon, water, and energy balance between land surface and atmosphere (Zhang et al. Citation2023).
The Tibetan Plateau, known as the world's third pole, is one of the most sensitive areas to global climate change because of its unique terrain. The understanding of spatiotemporal dynamics of PPT, which reflects maximum vegetation photosynthetic activity and its environmental drivers, must be improved (Li et al. Citation2020). Here, we used a new global and fine-resolution SIF dataset (GOSIF, V2), along with the flux-measured GPP and MODIS EVI-based land phenology product. The goals of this study were as follows: (1) to examine the relationship between global reconstructed SIF and flux-measured GPP for different vegetation types; (2) to compare the consistency between site-level PPT from EC-based GPP (PPTGPP) and SIF (PPTSIF) or MODIS EVI (peak greenness timing, PGTEVI), respectively; and (3) to evaluate the spatiotemporal dynamics of PPT in the Tibetan Plateau region and determine their response to climate factors during the past two decades. All analyses will improve our understanding and predictions of the effects of global climate change on ecosystem functions in alpine regions.
2. Methods and materials
2.1. Description of the study area
The Tibetan Plateau, located in southwestern China (), covers a vast territory accounting for 26.8% of China's total land area, extending from the Pamir Plateau in the west, the Hengduan Mountains in the east, the southern margin of the Himalayas in the south, and the Kunlun Mountains to the north of the Qilian Mountains in the north (Yao et al. Citation2012). The terrain is quite complicated with an average altitude over 4000 m and a declining trend from west to east. The special geographical location at low-middle latitude and high altitude generates unique alpine climate and natural conditions. The climate is cold with multi-year mean temperature in most regions below 0°C (Chen et al. Citation2020). Influenced by the westerlies and the monsoons, annual precipitation ranges from over 1000 mm to below 100 mm, which decreases progressively from southeast to northwest (Wang, Pang, and Yang Citation2018). Solar radiation in the Tibetan Plateau is intense, which is far higher than that in other regions at similar latitudes in the mainland of China (Liu et al. Citation2012). In general, the vegetation types alter from forest in the southeast, to meadow and steppe in the central area, and desert in the northwest.
2.2. Global reconstructed fluorescence data
The advent of global reconstructed SIF measurement provides an opportunity to monitor large-scale photosynthesis dynamics. Previous studies have shown a strong relationship between satellite-observed SIF from the Orbiting Carbon Observatory-2 (OCO-2) and terrestrial GPP (Li et al. Citation2018). However, the application of space–time discontinuous OCO-2 SIF retrievals at regional and even global scales remains a challenge. Fortunately, a global SIF product (GOSIF, V2) at a 0.05° spatial resolution and an 8-day interval is derived from individual OCO-2 SIF soundings, MODIS data, and meteorological reanalysis data (http://data.globalecology.unh.edu/). These SIF estimates from GOSIF performed well for GPP estimation at 91 EC-based flux sites globally (R2 = 0.73, p < 0.001) (Li and Xiao Citation2019). Besides, the fine spatial resolution, globally continuous coverage, and long-term records make GOSIF useful to characterize terrestrial photosynthesis. This study used the time-series GOSIF dataset from 2001 to 2022 to extract PPT in the Tibetan Plateau region and analyze its spatiotemporal characteristics.
2.3. Eddy covariance-based GPP data
The EC technique directly measures NEE, which can then separate into GPP and ecosystem respiration (Re). Therefore, terrestrial GPP can be used to generate photosynthetic phenology metrics and evaluate the performance of SIF-derived PPT. This study used GPP data from five EC flux sites in the Tibetan Plateau region covering three vegetation types (). GPP of each site was estimated from EC measurements by a standard procedure, including data quality control and gap filling (Li et al. Citation2023). The study used the night-time based flux-partitioning algorithm to separate GPP. Finally, we calculated the 8-day GPP to match the temporal interval of GOSIF and then detected the peak timing from GPP (PPTGPP).
Table 1. Description of the flux sites used in the study.
There are two alpine meadow flux sites (Damxung site [DX] and Naqu site [NQ]), two alpine swamp flux sites (Haibei swamp site [HB2] and Shenzha site [SZ]), and one alpine shrub flux site (Haibei shrub site [HB1]). The DX site and NQ site are situated in the hinterland of the Tibetan Plateau. The DX site is categorized as a region with plateau monsoon climate, mean annual temperature of 1.3°C, and annual mean precipitation of 476.8 mm. The NQ site has a sub-frigid monsoon climate and mean annual temperature of −1.9°C, which is the lowest among the five sites. Vegetation at the two sites is typical of alpine meadow. The HB2 site and SZ site are covered by alpine swamp. The HB2 site is located at the northeastern Tibetan Plateau, and the SZ site was built in the middle of Tibetan Plateau with an altitude of 4760 m, which is the highest among these sites. The HB1 site is close to HB2, which is covered by alpine shrub with characteristics of highland continental climate. Further details are available at the ChinaFLUX website (https://www.chinaflux.org) and Chinese Wetland Ecosystem Research Station Alliance website (https://wetland.ihb.cas.cn).
2.4. EVI-based phenology data
The EVI-based phenology data were obtained from the MODIS Land Cover Dynamics (MCD12Q2, Collection 6). Such MODIS land surface phenology product included the peak timing at 500 m spatial resolution and annual time step. The phenological events of vegetation within each year were estimated by modeling valid vegetation phases with a series of logistic functions based on the curvature change rate of smoothed EVI time series (Begue et al. Citation2014). Thus, this study extracted the peak layer of MCD12Q2 (https://lpdaacsvc.cr.usgs.gov/appeears/) as the MODIS-based peak greenness timing (PGTEVI) according to the location of flux sites and relevant period of flux-measured GPP data and then aggregated it to 0.05° spatial resolution.
2.5. Auxiliary data
The climate data were obtained from China Meteorological Forcing Dataset (CMFD), which was developed from satellite-based products, reanalysis datasets, and ground-based observations (He et al. Citation2020). The CMFD was at high spatiotemporal resolution covering 1979 to 2018 with a spatial resolution of 0.1° and a temporal resolution of 3 h. Seven climatic factors are provided in the CMFD, including air temperature, downward shortwave radiation, and precipitation rate. This study interpolated these variables to the spatial resolution of 0.05° to match GOSIF data. We calculated monthly mean temperature, precipitation, and solar radiation to examine the response of PPT to climate dynamics.
To analyze the response of PPT to the variability of altitude in this area, the study also used the digital elevation model (DEM) data from the Shuttle Radar Topography Mission (SRTM; 90 m; https://srtm.csi.cgiar.org/).
2.6. Extraction method of PPT
We extracted PPT at two different scales, namely, site level and regional level, relying on TIMESAT software (v3.3) developed by Eklundh and Jönsson (Citation2016). The adaptive Savitzky–Golay filter was used to lessen the noise in input data. The data were then smoothed by a least-squares fit to a quadratic polynomial, which could iteratively tighten the search window to capture rapid variations. The smoothing window was set to 5. According to a previous study (Li et al. Citation2023), the regional PPT was defined as a day of year halfway between the right 80% and left 80% of the input curve ().
2.7. Statistical analysis
To assess the consistency between PPTGPP and PPTSIF or PGTEVI, we calculated the root mean square error (RMSE) as follows:
(1)
(1) where
or
refers to the PPTGPP and PPTSIF or PGTEVI at relevant year
, respectively; and
refers to the number of data.
To analyze the long-term trend in PPT, we used the Mann–Kendall test and Theil–Sen slope at each pixel. If slope > 0, the PPT shifted toward autumn, and vice versa. The larger the absolute value of slope, the greater the intensity of change. Compared with the least squares regression, the Theil–Sen estimation is a non-parametric median-based slope estimator that is robust against outliers and can be computed as
(2)
(2) where
and
are the data values at times
and
(
), respectively.
The Mann–Kendall test was implemented to evaluate the significance of the trend. As a non-parametric statistical method, the Mann–Kendall test has been widely used for trend analysis (Zhao et al. Citation2023). The calculation of the Mann–Kendall statistic (S) is as follows:
(3)
(3) where
and
represent values at time
and
(
) in a time series of length
, respectively. The
is computed as
(4)
(4)
The variance of S was calculated using the following equation:
(5)
(5)
The standard test statistic (Z) was determined as follows:
(6)
(6)
We calculated the significance of 22 years of data and set the significance level at p < 0.1. The trend of PPT was considered significant with a 90% level of confidence when the absolute value of Z was greater than 1.64.
Wang and Wu (Citation2019) revealed that the peak timing of China's terrestrial ecosystems was mostly determined by climatic factors within 0–1 month. Here, we first confirmed which month that vegetation photosynthetic activity in the Tibetan Plateau region peaked at and then set preseason as the month. Partial correlation analysis was performed to reveal the dominant role of climate factors during preseason (i.e. temperature, precipitation, and radiation) in the inter-annual PPT dynamics. Compared with correlation analysis, partial correlation analysis can measure the degree of association between two variables with other potential controlling variables removed. We further examined the most important environmental factor that determined PPT according to the significance level (p < 0.1).
For example, the calculation of the partial correlation coefficient of PPT to temperature when controlling precipitation and radiation is as follows:
(7)
(7) where variables 1–4 refer to PPT, temperature, precipitation, and radiation, respectively.
or
refers to the determination coefficient of the regression analysis of variable 1 (PPT) and variables 2–4 (temperature, precipitation, and radiation, respectively) or variables 3 and 4 (precipitation and radiation, respectively). The other partial correlation coefficients were calculated in a similar way.
3. Results
3.1. Relationships between SIF and site-level GPP
The scatter plots in showed a strong positive correlation between global reconstructed SIF and flux-measured GPP at these EC-based sites in the Tibetan Plateau region (p < 0.001). For each vegetation type, the correlation coefficient (r) ranged from 0.942 at the alpine meadow (a) to 0.956 at the alpine swamp (b), with the alpine shrub falling in between (c). Furthermore, when mixing all vegetation types together, the correlation reached up to 0.877 (p < 0.001; d). This result confirmed the strong potential of SIF in capturing photosynthetic activity for a variety of vegetation types.
Figure 3. Relationship between flux-measured GPP and global reconstructed SIF for different vegetation types in the Tibetan Plateau region at an 8-day interval: (a) alpine meadow, (b) alpine swamp, (c) alpine shrub, and (d) all vegetation types.

As illustrated in , the seasonal dynamics of global reconstructed SIF generally agreed well with the flux-measured GPP at an 8-day interval. Both SIF and GPP exhibited similar climatology, with a single peak in the summertime. Specifically, the variability in SIF and GPP indicated that vegetation photosynthesis started around late March or April and ended in late October or early November. The PPT fell in approximately middle to late July, which was broadly consistent with the growth status of vegetation. Although a certain amount of discrepancies was observed between the global reconstructed SIF and the GPP observations at five flux sites, the time-series SIF showed highly consistent seasonality, especially in peak timing with GPP. Thus, the photosynthetic phenology in the Tibetan Plateau region could be feasibly monitored.
Figure 4. Climatology of global reconstructed SIF and the GPP observations at five flux sites in the Tibetan Plateau region. Each dot represents multi-year averaged SIF or GPP at an 8-day interval. Each curve represents fitted 8-day SIF or GPP. The vertical line represents the peak photosynthesis timing (PPT) from multi-year averaged SIF or GPP.

3.2. Site-level evaluation of PPTSIF and PGTEVI
To evaluate the accuracy of peak timing from global reconstructed SIF (PPTSIF) and EVI (PGTEVI) at the site level, we compared the consistency between PPTSIF and PGTEVI with PPT from the EC-based GPP (PPTGPP) for all sites across different vegetation types (). In comparison with PGTEVI, PPTSIF performed better with site-level PPTGPP observations. The scatter plots between PPTSIF and PPTGPP were distributed close to the 1:1 line, with R2 ranging from 0.52 to 0.90 and RMSE of 2.04 to 6.13 days, respectively. By contrast, PGTEVI almost lagged behind PPTGPP over all three vegetation types with relatively lower R2 and higher RMSE values. Overall, PPTSIF exhibited the best performance at the alpine shrub and swamp (RMSE = 2.04 and 2.64 days, respectively), followed by the alpine meadow ecosystem (RMSE = 6.13 days).
Figure 5. Comparisons of the peak photosynthesis timing from site-level GPP (PPTGPP), global reconstructed SIF (PPTSIF), and MODIS EVI (PGTEVI) at the five flux sites in the Tibetan Plateau region: (a) and (b) alpine meadow; (c) and (d) alpine swamp; (e) and (f) alpine shrub. Solid line is the regression analysis between PPTGPP and PGTEVI or PPTSIF. Dashed line represents the 1:1 line.

As shown in , this study further compared multi-year mean PPTs including flux-measured PPTGPP, global reconstructed PPTSIF, and MODIS-based PGTEVI at the five flux sites. In general, PPTSIF exhibited good consistency with PPTGPP at more sites than PGTEVI. The average difference between multi-year mean PPTGPP and PPTSIF was 2.28 days (ranging from 0.81 to 5.08 days), which was far lower than that between PGTEVI and PPTGPP of 6.65 days (ranging from 1.37 to 13.56 days). Except for the SZ and NQ sites with limited site years of data, the biases between PPTSIF and PPTGPP were generally less than 2 days at the other sites, including the DX, HB2, and HB1 sites. However, such discrepancies were considerably larger between PGTEVI and PPTGPP. Therefore, the study used PPTSIF to characterize the spatial and temporal patterns of PPT across the Tibetan Plateau region.
3.3. Spatial pattern of PPT
As a result of complicated terrain factors in the Tibetan Plateau, the pattern of multi-year mean PPT exhibited strong spatial heterogeneity (a). In general, we observed an increasing trend in PPT along the southeast–northwest direction. Early PPT was mainly located in the eastern borders of the Tibetan Plateau with low elevation, and it occurred in early July. However, PPT arrived in late July or even early August in the vast hinterland of the northwestern Tibetan Plateau area. Late PPT (>215) also occurred in the southwestern part of the Tibetan Plateau because of the effect of high elevation. An exception was a small area at the northwestern edge with low altitude where PPT arrived in mid-July. Overall, during the past two decades, the regional multi-year mean PPT mainly occurred on DOY 205–215, accounting for 56.91% of the Tibetan Plateau region. Thus, vegetation photosynthetic activity reached a peak at the end of July to the beginning of August.
Figure 7. Spatial pattern (a), latitudinal trend (b), and longitudinal trend (c) of multi-year mean peak photosynthesis timing (PPT) in the Tibetan Plateau region during 2001–2022. (d) and (e) Variations in elevation along latitude and longitude. (f) Distribution of PPT.

This study also investigated the latitudinal and longitudinal trends of PPT (b,c) jointly with elevation (d,e). We found an elevation-dependent spatial pattern of PPT. Overall, the variation in PPT along latitude and longitude was similar to elevation in the Tibetan Plateau area. PPT showed an obviously eastward-delayed trend in the longitude zone ranging from 82°E to 105°E, which was ascribed to the decreasing elevation. However, the variation in PPT in latitude was relatively complicated. PPT reached the earliest (during about DOY 201) at the lower and higher latitudes, which could be explained by the effect of relatively low elevation. By contrast, the lagging PPT was mainly concentrated between 28°N and 30°N with the highest elevation. It then fluctuated slightly from 30°N and 35.4°N around DOY 210. The study also found that PPT reached the latest on approximately DOY 214 at about 28.7°N.
3.4. Long-term trend in PPT
illustrates the long-term spatial trend in PPT over the past two decades in the Tibetan Plateau region, which also exhibited strong spatial heterogeneity. Overall, the regional PPT was advanced by 0.89 day decade−1. The percentage of the advanced area was about 67.36% of the total area (c), of which 11.37% passed the significance level (d; p < 0.1). The advanced PPT was mainly distributed in the central hinterland of the region with high elevation. However, 32.64% of the total area experienced delayed PPT (c), of which 2.21% passed the significance level (d; p < 0.1), because of the sparse location in the borders of the area.
3.5. Effect of preseason climatic factors on PPT
Given the crucial role of preseason hydrothermal conditions to vegetation development, we evaluated the effects of preseason climatic factors including mean temperature, total precipitation, and total solar shortwave radiation on PPT by using spatial partial correlation analysis to account for the long-term trend in PPT during the past two decades. As illustrated in , the dominant factors of PPT in the Tibetan Plateau area exhibited strong spatial heterogeneity. Among the three climate factors, preseason temperature was the most important factor dominating about 8.90% of all pixels, followed by solar shortwave radiation (8.15%) and precipitation (7.01%). PPT showed positive partial correlations with preseason temperature in 52.56% of all significant pixels (p < 0.1, a), and it was mainly distributed in southwestern part of the Tibetan Plateau with high elevation. Pixels with significant negative partial correlations were much less, and they appeared in the central Tibetan Plateau area. Significant positive correlations between PPT and precipitation were mainly found in the central and northeastern Tibetan Plateau (about 61.78% of all significant pixels), indicating that additional precipitation would delay PPT (b). The pixels with significant partial correlations between PPT and radiation were about 10.09% of the Tibetan Plateau region (c), 53.61% of which exhibited negative effects (e.g. northeastern and central Tibetan Plateau). Thus, PPT coincided with the changes in radiation, and high levels of radiation led to the advancement in PPT. However, strong radiation may also delay PPT, which mainly occurred in the northeast low-elevation Tibetan Plateau area.
Figure 9. Spatial partial correlations between the peak photosynthesis timing (PPT) and climatic factors including (a) monthly mean temperature (Temp); (b) monthly total precipitation (Prec); (c) monthly total shortwave radiation (Srad); and (d) dominant factor in the Tibetan Plateau during 2001–2018. The significance level was set at p < 0.1.

4. Discussion
4.1. Differences among SIF, reconstructed SIF, and VIs in monitoring GPP
The study revealed a strong linear GOSIF–GPP relationship at the EC-based flux sites in the Tibetan Plateau area (), which was confirmed by previous studies (Gao et al. Citation2021). The complicated relationships between SIF and other physiological processes, as well as the uncertainties in various SIF products, cannot be neglected. Although GOSIF is effective for assessing terrestrial photosynthesis (Tang et al. Citation2022), GOSIF is not a true SIF observation but reconstructed SIF product derived from the discrete OCO-2 SIF soundings and other auxiliary data. With the increasing demand of terrestrial vegetation photosynthesis monitoring, several reconstructed SIF data including gap-filled products, such as CSIF (Zhang et al. Citation2018), (Yu et al. Citation2019), and GOSIF (Li and Xiao Citation2019), and downscaled products like RSIF (Gentine and Alemohammad Citation2018),
(Duveiller et al. Citation2020), and
(Wen et al. Citation2020) have been developed. The different input data and algorithm lead to uncertainties among such reconstructed SIF products, especially for the SIF signals derived from various satellite platforms. Bacour et al. (Citation2019) compared the daily mean SIF products from OCO-2 and GOME-2, and they found disagreements in annual mean values and seasonal amplitude. Gao et al. (Citation2021) found that the relative biases for GOSIF and CSIF are 7.14% and 11.06% in the estimated cumulative GPP across all EC towers, respectively. Moreover, uncertainty from the footprint mismatch between satellite-based reconstructed SIF product and EC-based GPP measurements still exists. However, a considerable proportion of spatial homogeneity of vegetation types in the Tibetan Plateau region could reduce the impact of such uncertainty to a certain extent. The strong linear relationship between SIF and GPP, as well as the consistent seasonal dynamics ( and ), also certified the feasibility to monitor regional photosynthetic phenology.
Compared with VIs, SIF is unique in capturing some physiological responses to stress that might not be reflected in VIs (Martini et al. Citation2022). Li et al. (Citation2018) reported that OCO-2 SIF is more strongly correlated with tower GPP than conventional NDVI and EVI, EVIBRDF, and the recently proposed NIRv. Zhang et al. (Citation2021) found that EVI is not an accurate indicator of peak phenology in a warm-temperate mixed plantation. The notable separation between greenness-related VIs and photosynthesis-related SIF in a high-latitude ecosystem has been confirmed by Walther et al. (Citation2018). Kong et al. (Citation2020) also described asynchronization between VI-indicated greenness phenology and GPP-indicated photosynthetic phenology at 95 flux sites around the world. Yang et al. (Citation2022) revealed the systemic inconsistency in phenological metrics between TROPOMI SIF and VIs in Tibetan Plateau grasslands. Recently, Dechant et al. (Citation2022) found that canopy structure-related near-infrared reflectance of vegetation multiplied by incoming sunlight (NIRVP) is a robust structural proxy for SIF and photosynthesis across scales.
Our study found that PPTSIF was more consistent with PPTGPP than PGTEVI in alpine shrub, swamp, and meadow ecosystems (), which could contribute to the mismatch between the timing of physiological parameter and timing of ecological functions, such as photosynthesis (D’Odorico et al. Citation2015; Jeong et al. Citation2017). Compared with the reflectance-based VIs, the global reconstructed SIF is more related to vegetation photosynthesis and not sensitive to cloud and atmospheric scattering, which accounted for the differences in accuracy. For large-area estimation, the remote sensing technique can only provide relatively accurate results of terrestrial ecosystems, which does not affect the long-term trend analysis. Similar to previous studies (Gao et al. Citation2021), the SIF exhibited strong potential in capturing the variability of GPP at the flux sites in the Tibetan Plateau region (). Additionally, peak greenness emerged later than peak photosynthesis across years and vegetation types in circumpolar regions (Walther et al. Citation2018), which was also confirmed in our work. At the time when greenness is at a maximum, photosynthetic rates are decreasing. Therefore, our study provided an alternative framework to monitor global photosynthetic phenology by solely using the global reconstructed SIF dataset.
4.2. Responses of PPT to 3D environment and climate factors
Previous studies and Hopkins’ Bioclimatic Law reported the influence of 3D terrain on the spatial pattern of vegetation phenological events (Dai, Wang, and Ge Citation2014; Gao et al. Citation2020). In this study, we revealed the strong spatial heterogeneity and a northwestward delaying trend in PPT across the Tibetan Plateau region (). Thus, our work investigated the responses of multi-year mean PPT during the past two decades to the variations in latitude, longitude, and elevation (). Insignificant correlations were found between PPT and latitude (0.1°) with a low correlation coefficient (r = 0.121) and partial correlation coefficient (pr) lower than 0.01. However, we observed relatively stronger correlations between elevation (r = 0.568, p < 0.001) or longitude (r = −0.551, p < 0.001) and multi-year averaged PPT. When controlling the two other terrain factors, the correlation between multi-year averaged PPT and longitude weakened (pr = −0.373, p < 0.001), whereas elevation and multi-year averaged PPT still had a strong correlation (pr = 0.407, p < 0.001). Thus, the relatively strong correlation between longitude and multi-year averaged PPT was derived from the regional strong correlation between longitude and elevation. Despite complex effects exerted by longitude and elevation, our study demonstrated that these terrain factors mostly contributed to the spatial heterogeneity of PPT in the Tibetan Plateau region.
Figure 10. Distribution of multi-year averaged PPT across latitude, longitude, and elevation in the Tibetan Plateau.

Given the significant role of altitude, this study further discussed the response of multi-year averaged PPT to elevation with an interval of 100 m. As shown in , an apparent inflection point was observed at about 2500 m asl. Specifically, when the elevation exceeded 2500 m in the Tibetan Plateau region, PPT exhibited a significant increase, which meant that peak timing was delayed by 4.99 days per 1000 m (R2 = 0.87, p < 0.001). By contrast, a negative correlation between PPT and elevation was revealed when the altitude was below 2500 m, which meant that peak timing advanced by 4.49 days per 1000 m (R2 = 0.80, p < 0.001). Ding et al. (Citation2013) also reported the relationship between elevation from 2500 to 5500 m and associated phenological metrics including the start of growing season (−9 days per 1000 m), end of growing season (+1 day per 1000 m), and shortened length of growing period (−9 days per 1000 m) in the Tibetan Plateau. The opposite trend of PPT with elevation below 2500 m could be related to extensive human-induced activities (such as land management and land use difference) at the edges of the Tibetan Plateau (Ding et al. Citation2013).
Figure 11. Variations in averaged PPT along with the elevation gradients from the SRTM DEM dataset in the Tibetan Plateau. The averaged PPT values were calculated from multi-year PPT during 2001–2022 within each 100 m elevation belt.

In addition, climatic conditions are the direct factors affecting changes in vegetation phenology (Gong et al. Citation2023; Park et al. Citation2019; Shen et al. Citation2015). In this study, we systematically analyzed the influence of temperature, precipitation, and radiation on long-term PPT trends across various ecosystems. Our study revealed an overall advanced trend of PPT by approximately 0.89 day decade−1 in the area (). However, the long-term dynamics in vegetation PPT were eventually controlled by the alterations in the climate system (Wang et al. Citation2021). Zhang et al. (Citation2018) also found that temperature is the dominant climatic variable driving the changes in vegetation phenology. Park et al. (Citation2019) found a widespread warming-induced advance in PPT (−1.66 ± 0.30 day decade−1, p < 0.001) across northern lands, and PPT in the boreal ecosystems showed a much closer alignment with peak radiation. Similar to the findings of Wang et al. (Citation2015), our work also confirmed the influence exerted by precipitation on the peak timing. Indeed, dominant climate factors vary across large areas, and the impact of these climate factors on PPT can differ in various regions due to their varying sensitivity to environmental stress. For example, in the southwestern Tibetan Plateau, where spring is typically cold, a high summer temperature will increase the chance of drought and then lead to a delayed PPT (Wang and Wu Citation2019). Although the study explored the response of climatic factors to PPT, other environmental effects such as CO2 fertilization and snow cover should be considered in the future.
5. Conclusion
PPT is an important but neglected phenological metric under the context of global climate change. Our study compared the global reconstructed SIF and flux-measured GPP at different EC-based flux sites in the Tibetan Plateau region. We found strong consistencies, which revealed the potential of SIF to characterize vegetation productivity. Moreover, PPTSIF performed better than PGTEVI in capturing the variability of PPT (PPTGPP) at the site level. Thus, we used PPTSIF to examine the spatiotemporal dynamics of PPT in the Tibetan Plateau region. Multi-year mean PPT mainly appeared in late July to early August, and a gradually delayed trend was revealed from southeast to northwest. This study also found that PPT over the Tibetan Plateau region experienced apparent advancement since 2000. Furthermore, the elevation exhibited a substantial effect on PPT from the perspective of 3D environment. Overall, the strong spatial heterogeneity of PPT was constrained by different climatic factors. Future work can focus on the regulatory mechanisms that underlie vegetation photosynthetic phenology. Our study contributes to the realization of interactions between global climate change and ecosystem responses in alpine regions.
Acknowledgements
We would like to express our gratitude to all scientists that have contributed to the multi-source datasets used in the study.
Disclosure statement
No potential conflict of interest was reported by the author(s).
Data availability statement
All data can be accessed via the corresponding author upon request.
Additional information
Funding
References
- Bacour, C., F. Maignan, P. Peylin, N. MacBean, V. Bastrikov, J. Joiner, P. Köhler, L. Guanter, and C. Frankenberg. 2019. “Differences Between OCO-2 and GOME-2 SIF Products from a Model-Data Fusion Perspective.” Journal of Geophysical Research: Biogeosciences 124 (10): 3143–3157. https://doi.org/10.1029/2018JG004938.
- Begue, A., E. Vintrou, A. Saad, and P. Hiernaux. 2014. “Differences Between Cropland and Rangeland MODIS Phenology (Start-of-Season) in Mali.” International Journal of Applied Earth Observation and Geoinformation 31: 167–170. https://doi.org/10.1016/j.jag.2014.03.024.
- Berra, E. F., and R. Gaulton. 2021. “Remote Sensing of Temperate and Boreal Forest Phenology: A Review of Progress, Challenges and Opportunities in the Intercomparison of In-Situ and Satellite Phenological Metrics.” Forest Ecology and Management 480: 118663. https://doi.org/10.1016/j.foreco.2020.118663.
- Chang, Q., X. Xiao, W. Jiao, X. Wu, R. Doughty, J. Wang, L. Du, Z. Zou, and Y. Qin. 2019. “Assessing Consistency of Spring Phenology of Snow-Covered Forests as Estimated by Vegetation Indices, Gross Primary Production, and Solar-Induced Chlorophyll Fluorescence.” Agricultural and Forest Meteorology 275: 305–316. https://doi.org/10.1016/j.agrformet.2019.06.002.
- Chen, F., J. Zhang, J. Liu, X. Cao, J. Hou, L. Zhu, X. Xu, et al. 2020. “Climate Change, Vegetation History, and Landscape Responses on the Tibetan Plateau During the Holocene: A Comprehensive Review.” Quaternary Science Reviews 243: 106444. https://doi.org/10.1016/j.quascirev.2020.106444.
- Chu, H., D. D. Baldocchi, R. John, S. Wolf, and M. Reichstein. 2017. “Fluxes All of the Time? A Primer on the Temporal Representativeness of FLUXNET.” Journal of Geophysical Research: Biogeosciences 122 (2): 289–307. https://doi.org/10.1002/2016JG003576.
- Crimmins, T. M. 2021. “The USA National Phenology Network: Big Idea, Productivity, and Potential—and Now, at Big Risk.” Bulletin of the Ecological Society of America 102: 1–3. https://doi.org/10.1002/bes2.1802.
- Dai, J., H. Wang, and Q. Ge. 2014. “The Spatial Pattern of Leaf Phenology and Its Response to Climate Change in China.” International Journal of Biometeorology 58 (4): 521–528. https://doi.org/10.1007/s00484-013-0679-2.
- Dechant, B., Y. Ryu, G. Badgley, P. Köhler, U. Rascher, M. Migliavacca, Y. Zhang, et al. 2022. “NIRVP: A Robust Structural Proxy for Sun-Induced Chlorophyll Fluorescence and Photosynthesis Across Scales.” Remote Sensing of Environment 268: 112763. https://doi.org/10.1016/j.rse.2021.112763.
- Ding, M., Y. Zhang, X. Sun, L. Liu, Z. Wang, and W. Bai. 2013. “Spatiotemporal Variation in Alpine Grassland Phenology in the Qinghai-Tibetan Plateau from 1999 to 2009.” Chinese Science Bulletin 58 (3): 396–405. https://doi.org/10.1007/s11434-012-5407-5.
- D’Odorico, P., A. Gonsamo, C. M. Gough, G. Bohrer, J. Morison, M. Wilkinson, P. J. Hanson, D. Gianelle, J. D. Fuentes, and N. Buchmann. 2015. “The Match and Mismatch Between Photosynthesis and Land Surface Phenology of Deciduous Forests.” Agricultural and Forest Meteorology 214-215: 25–38. https://doi.org/10.1016/j.agrformet.2015.07.005.
- Duveiller, G., F. Filipponi, S. Walther, P. Köhler, C. Frankenberg, L. Guanter, and A. Cescatti. 2020. “A spatially downscaled sun-induced fluorescence global product for enhanced monitoring of vegetation productivity.” Earth System Science Data 12 (2): 1101–1116. https://doi.org/10.5194/essd-12-1101-2020.
- Eklundh, L., and P. Jönsson. 2016. “TIMESAT for Processing Time-Series Data from Satellite Sensors for Land Surface Monitoring.” In Remote Sensing and Digital Image Processing. Multitemporal Remote Sensing, edited by Y. Ban, 177–194. Cham: Springer International Publishing.
- Gao, H., S. Liu, W. Lu, A. R. Smith, R. Valbuena, W. Yan, Z. Wang, et al. 2021. “Global Analysis of the Relationship Between Reconstructed Solar-Induced Chlorophyll Fluorescence (SIF) and Gross Primary Production (GPP).” Remote Sensing 13: 2824. https://doi.org/10.3390/rs13142824.
- Gao, M., X. Wang, F. Meng, Q. Liu, X. Li, Y. Zhang, and S. Piao. 2020. “Three-dimensional Change in Temperature Sensitivity of Northern Vegetation Phenology.” Global Change Biology 26 (9): 5189–5201. https://doi.org/10.1111/gcb.15200.
- Gentine, P., and S. H. Alemohammad. 2018. “Reconstructed Solar-Induced Fluorescence: A Machine Learning Vegetation Product Based on MODIS Surface Reflectance to Reproduce GOME-2 Solar-Induced Fluorescence.” Geophysical Research Letters 45 (7): 3136–3146. https://doi.org/10.1002/2017GL076294.
- Gong, H., L. Cao, Y. Duan, F. Jiao, X. Xu, M. Zhang, K. Wang, and H. Liu. 2023. “Multiple Effects of Climate Changes and Human Activities on NPP Increase in the Three-North Shelter Forest Program Area.” Forest Ecology and Management 529: 120732. https://doi.org/10.1016/j.foreco.2022.120732.
- Gonsamo, A., J. M. Chen, and Y. W. Ooi. 2018. “Peak Season Plant Activity Shift Towards Spring is Reflected by Increasing Carbon Uptake by Extratropical Ecosystems.” Global Change Biology 24 (5): 2117–2128. https://doi.org/10.1111/gcb.14001.
- He, J., K. Yang, W. Tang, H. Lu, J. Qin, Y. Chen, and X. Li. 2020. “The First High-Resolution Meteorological Forcing Dataset for Land Process Studies Over China.” Scientific Data 7: 25. https://doi.org/10.1038/s41597-020-0369-y.
- Jeong, S.-J., D. Schimel, C. Frankenberg, D. T. Drewry, J. B. Fisher, M. Verma, J. A. Berry, J.-E. Lee, and J. Joiner. 2017. “Application of Satellite Solar-Induced Chlorophyll Fluorescence to Understanding Large-Scale Variations in Vegetation Phenology and Function Over Northern High Latitude Forests.” Remote Sensing of Environment 190: 178–187. https://doi.org/10.1016/j.rse.2016.11.021.
- Jiang, C., and Y. Ryu. 2016. “Multi-scale Evaluation of Global Gross Primary Productivity and Evapotranspiration Products Derived from Breathing Earth System Simulator (BESS).” Remote Sensing of Environment 186: 528–547. https://doi.org/10.1016/j.rse.2016.08.030.
- Katal, N., M. Rzanny, P. Mäder, and J. Wäldchen. 2022. “Deep Learning in Plant Phenological Research: A Systematic Literature Review.” Frontiers in Plant Science 13: 805738. https://doi.org/10.3389/fpls.2022.805738.
- Kong, D., Y. Zhang, D. Wang, J. Chen, and X. Gu. 2020. “Photoperiod Explains the Asynchronization Between Vegetation Carbon Phenology and Vegetation Greenness Phenology.” Journal of Geophysical Research: Biogeosciences 125 (8): e2020JG005636. https://doi.org/10.1029/2020JG005636.
- Li, G., C. Wu, Y. Chen, C. Huang, Y. Zhao, Y. Wang, M. Ma, Z. Ding, P. Yu, and X. Tang. 2023. “Increasing Temperature Regulates the Advance of Peak Photosynthesis Timing in the Boreal Ecosystem.” Science of The Total Environment 882: 163587. https://doi.org/10.1016/j.scitotenv.2023.163587.
- Li, J., C. Wu, X. Wang, J. Peng, D. Dong, G. Lin, and A. Gonsamo. 2020. “Satellite Observed Indicators of the Maximum Plant Growth Potential and Their Responses to Drought Over Tibetan Plateau (1982–2015).” Ecological Indicators 108: 105732. https://doi.org/10.1016/j.ecolind.2019.105732.
- Li, X., and J. Xiao. 2019. “Mapping Photosynthesis Solely from Solar-Induced Chlorophyll Fluorescence: A Global, Fine-Resolution Dataset of Gross Primary Production Derived from OCO-2.” Remote Sensing 11: 2563. https://doi.org/10.3390/rs11212563.
- Li, X., J. Xiao, B. He, M. A. Arain, J. Beringer, A. R. Desai, C. Emmel, et al. 2018. “Solar-Induced Chlorophyll Fluorescence is Strongly Correlated with Terrestrial Photosynthesis for a Wide Variety of Biomes: First Global Analysis Based on OCO-2 and Flux Tower Observations.” Global Change Biology 24 (9): 3990–4008. https://doi.org/10.1111/gcb.14297.
- Lin, S., J. Li, Q. Liu, B. Gioli, E. Paul-Limoges, N. Buchmann, M. Gharun, et al. 2021. “Improved Global Estimations of Gross Primary Productivity of Natural Vegetation Types by Incorporating Plant Functional Type.” International Journal of Applied Earth Observation and Geoinformation 100: 102328. https://doi.org/10.1016/j.jag.2021.102328.
- Liu, J., J. Liu, H. W. Linderholm, D. Chen, Q. Yu, D. Wu, and S. Haginoya. 2012. “Observation and Calculation of the Solar Radiation on the Tibetan Plateau.” Energy Conversion and Management 57: 23–32. https://doi.org/10.1016/j.enconman.2011.12.007.
- Liu, X., L. Zhou, H. Shi, S. Wang, and Y. Chi. 2018. “Phenological Characteristics of Temperate Coniferous and Broad-Leaved Mixed Forests Based on Multiple Remote Sensing Vegetation Indices, Chlorophyll Fluorescence and CO2 Flux Data.” Acta Ecologica Sinica 38: 3482–3494. https://doi.org/10.5846/stxb201708211508.
- Martini, D., K. Sakowska, G. Wohlfahrt, J. Pacheco-Labrador, C. van der Tol, A. Porcar-Castell, T. S. Magney, et al. 2022. “Heatwave Breaks Down the Linearity Between sun-Induced Fluorescence and Gross Primary Production.” New Phytologist 233 (6): 2415–2428. https://doi.org/10.1111/nph.17920.
- Meng, F., L. Huang, A. Chen, Y. Zhang, and S. Piao. 2021. “Spring and Autumn Phenology Across the Tibetan Plateau Inferred from Normalized Difference Vegetation Index and Solar-Induced Chlorophyll Fluorescence.” Big Earth Data 5 (2): 182–200. https://doi.org/10.1080/20964471.2021.1920661.
- Park, T., C. Chen, M. Macias-Fauria, H. Tømmervik, S. Choi, A. Winkler, U. S. Bhatt, et al. 2019. “Changes in Timing of Seasonal Peak Photosynthetic Activity in Northern Ecosystems.” Global Change Biology 25 (7): 2382–2395. https://doi.org/10.1111/gcb.14638.
- Piao, S., Q. Liu, A. Chen, I. A. Janssens, Y. Fu, J. Dai, L. Liu, X. Lian, M. Shen, and X. Zhu. 2019. “Plant Phenology and Global Climate Change: Current Progresses and Challenges.” Global Change Biology 25 (6): 1922–1940. https://doi.org/10.1111/gcb.14619.
- Qi, Y., D. Wei, H. Zhao, and X. Wang. 2021. “Carbon Sink of a Very High Marshland on the Tibetan Plateau.” Journal of Geophysical Research: Biogeosciences 126 (4): e2020JG006235. https://doi.org/10.1029/2020JG006235.
- Richardson, A. D., T. F. Keenan, M. Migliavacca, Y. Ryu, O. Sonnentag, and M. Toomey. 2013. “Climate Change, Phenology, and Phenological Control of Vegetation Feedbacks to the Climate System.” Agricultural and Forest Meteorology 169: 156–173. https://doi.org/10.1016/j.agrformet.2012.09.012.
- Running, S. W., R. R. Nemani, F. A. Heinsch, M. Zhao, M. Reeves, and H. Hashimoto. 2004. “A Continuous Satellite-Derived Measure of Global Terrestrial Primary Production.” BioScience 54 (6): 547–560. https://doi.org/10.1641/0006-3568(2004)054[0547:ACSMOG]2.0.CO;2
- Shen, M., S. Piao, N. Cong, G. Zhang, and I. A. Jassens. 2015. “Precipitation Impacts on Vegetation Spring Phenology on the Tibetan Plateau.” Global Change Biology 21 (10): 3647–3656. https://doi.org/10.1111/gcb.12961
- Shi, P., X. Sun, L. Xu, X. Zhang, Y. He, D. Zhang, and G. Yu. 2006. “Net Ecosystem CO2 Exchange and Controlling Factors in a Steppe—Kobresia Meadow on the Tibetan Plateau.” Science in China Series D: Earth Sciences 49 (S2): 207–218. https://doi.org/10.1007/s11430-006-8207-4.
- Tang, X., J. Xiao, M. Ma, H. Yang, X. Li, Z. Ding, P. Yu, et al. 2022. “Satellite Evidence for China’s Leading Role in Restoring Vegetation Productivity Over Global Karst Ecosystems.” Forest Ecology and Management 507: 120000. https://doi.org/10.1016/j.foreco.2021.120000.
- Templ, B., E. Koch, K. Bolmgren, M. Ungersböck, A. Paul, H. Scheifinger, T. Rutishauser, et al. 2018. “Pan European Phenological Database (PEP725): A Single Point of Access for European Data.” International Journal of Biometeorology 62 (6): 1109–1113. https://doi.org/10.1007/s00484-018-1512-8.
- Walther, S., L. Guanter, B. Heim, M. Jung, A. Duveiller, A. Wolanin, and T. Sachs. 2018. “Assessing the dynamics of vegetation productivity in circumpolar regions with different satellite indicators of greenness and photosynthesis.” Biogeosciences 15 (20): 6221–6256. https://doi.org/10.5194/bg-15-6221-2018.
- Wang, M., Z. Ding, C. Wu, L. Song, M. Ma, P. Yu, B. Lu, and X. Tang. 2021. “Divergent Responses of Ecosystem Water-Use Efficiency to Extreme Seasonal Droughts in Southwest China.” Science of the Total Environment 760: 143427. https://doi.org/10.1016/j.scitotenv.2020.143427.
- Wang, C., H. Guo, L. Zhang, S. Liu, Y. Qiu, and Z. Sun. 2015. “Assessing Phenological Change and Climatic Control of Alpine Grasslands in the Tibetan Plateau with MODIS Time Series.” International Journal of Biometeorology 59 (1): 11–23. https://doi.org/10.1007/s00484-014-0817-5.
- Wang, X., G. Pang, and M. Yang. 2018. “Precipitation Over the Tibetan Plateau During Recent Decades: A Review Based on Observations and Simulations.” International Journal of Climatology 38 (3): 1116–1131. https://doi.org/10.1002/joc.5246.
- Wang, Y., Y. Sun, Y. Chen, C. Wu, C. Huang, C. Li, and X. Tang. 2023. “Non-linear correlations exist between solar-induced chlorophyll fluorescence and canopy photosynthesis in a subtropical evergreen forest in Southwest China.” Ecological Indicators 157: 111311. https://doi.org/10.1016/j.ecolind.2023.111311.
- Wang, Y., J. Wei, X. Tang, X. Han, and M. Ma. 2020. “Progress of Using the Chlorophyll Fluorescence to Estimate Terrestrial Gross Primary Production.” Remote Sensing Technology and Application 35: 975–989. https://doi.org/10.11873/j.issn.1004-0323.2020.5.0975.
- Wang, X., and C. Wu. 2019. “Estimating the Peak of Growing Season (POS) of China’s Terrestrial Ecosystems.” Agricultural and Forest Meteorology 278: 107639. https://doi.org/10.1016/j.agrformet.2019.107639.
- Wen, J., P. Köhler, G. Duveiller, N. C. Parazoo, T. S. Magney, G. Hooker, L. Yu, C. Y. Chang, and Y. Sun. 2020. “A Framework for Harmonizing Multiple Satellite Instruments to Generate a Long-Term Global High Spatial-Resolution Solar-Induced Chlorophyll Fluorescence (SIF).” Remote Sensing of Environment 239: 111644. https://doi.org/10.1016/j.rse.2020.111644.
- Wu, C., J. Peng, P. Ciais, J. Peñuelas, H. Wang, S. Beguería, T. Andrew Black, et al. 2022. “Increased Drought Effects on the Phenology of Autumn Leaf Senescence.” Nature Climate Change 12 (10): 943–949. https://doi.org/10.1038/s41558-022-01464-9.
- Xiao, X., D. Hollinger, J. Aber, M. Goltz, E. A. Davidson, Q. Zhang, and B. Moore. 2004. “Satellite-Based Modeling of Gross Primary Production in an Evergreen Needleleaf Forest.” Remote Sensing of Environment 89 (4): 519–534. https://doi.org/10.1016/j.rse.2003.11.008.
- Yang, J., J. Dong, X. Xiao, J. Dai, C. Wu, J. Xia, G. Zhao, et al. 2019. “Divergent Shifts in Peak Photosynthesis Timing of Temperate and Alpine Grasslands in China.” Remote Sensing of Environment 233: 111395. https://doi.org/10.1016/j.rse.2019.111395.
- Yang, J., X. Xiao, R. Doughty, M. Zhao, Y. Zhang, P. Köhler, X. Wu, C. Frankenberg, and J. Dong. 2022. “TROPOMI SIF Reveals Large Uncertainty in Estimating the end of Plant Growing Season from Vegetation Indices Data in the Tibetan Plateau.” Remote Sensing of Environment 280: 113209. https://doi.org/10.1016/j.rse.2022.113209.
- Yao, T., L. G. Thompson, V. Mosbrugger, F. Zhang, Y. Ma, T. Luo, B. Xu, et al. 2012. “Third Pole Environment (TPE).” Environmental Development 3: 52–64. https://doi.org/10.1016/j.envdev.2012.04.002.
- Yu, L., J. Wen, C. Y. Chang, C. Frankenberg, and Y. Sun. 2019. “High-Resolution Global Contiguous SIF of OCO-2.” Geophysical Research Letters 46 (3): 1449–1458. https://doi.org/10.1029/2018GL081109.
- Zeng, L., B. D. Wardlow, D. Xiang, S. Hu, and D. Li. 2020. “A Review of Vegetation Phenological Metrics Extraction Using Time-Series, Multispectral Satellite Data.” Remote Sensing of Environment 237: 111511. https://doi.org/10.1016/j.rse.2019.111511.
- Zhang, J., A. Gonsamo, X. Tong, J. Xiao, C. Rogers, S. Qin, P. Liu, and P. Ma. 2023. “Solar-Induced Chlorophyll Fluorescence Captures Photosynthetic Phenology Better Than Traditional Vegetation Indices.” ISPRS Journal of Photogrammetry and Remote Sensing 203: 183–198. https://doi.org/10.1016/j.isprsjprs.2023.07.021.
- Zhang, Q., D. Kong, P. Shi, V. P. Singh, and P. Sun. 2018. “Vegetation Phenology on the Qinghai-Tibetan Plateau and Its Response to Climate Change (1982–2013).” Agricultural and Forest Meteorology 248: 408–417. https://doi.org/10.1016/j.agrformet.2017.10.026.
- Zhang, J., X. Tong, P. Meng, J. Zhang, and P. Liu. 2020. “Comparative Study on Phenology in a Mountainous Plantation in Northern China Based on Vegetation Index, Chlorophyll Fluorescence and Carbon Flux.” Journal of Beijing Forestry University 42: 17–26. https://doi.org/10.12171/j.1000-1522.20200113.
- Zhang, J., X. Tong, J. Zhang, P. Meng, J. Li, and P. Liu. 2021. “Dynamics of Phenology and its Response to Climatic Variables in a Warm-Temperate Mixed Plantation.” Forest Ecology and Management 483: 118785. https://doi.org/10.1016/j.foreco.2020.118785.
- Zhang, Z., S. Wang, B. Qiu, L. Song, and Y. Zhang. 2019. “Retrieval of Sun-Induced Chlorophyll Fluorescence and Advancements in Carbon Cycle Application.” National Remote Sensing Bulletin 23: 37–52. https://doi.org/10.11834/jrs.20197485.
- Zhang, T., M. Xu, Y. Zhang, T. Zhao, T. An, Y. Li, Y. Sun, et al. 2019. “Grazing-Induced Increases in Soil Moisture Maintain Higher Productivity During Droughts in Alpine Meadows on the Tibetan Plateau.” Agricultural and Forest Meteorology 269-270: 249–256. https://doi.org/10.1016/j.agrformet.2019.02.022.
- Zhao, Y., Y. Chen, C. Wu, G. Li, M. Ma, L. Fan, H. Zheng, L. Song, and X. Tang. 2023. “Exploring the Contribution of Environmental Factors to Evapotranspiration Dynamics in the Three-River-Source Region, China.” Journal of Hydrology 626: 130222. https://doi.org/10.1016/j.jhydrol.2023.130222.
- Zhao, L., J. Li, S. Xu, H. Zhou, Y. Li, S. Gu, and X. Zhao. 2010. “Seasonal Variations in Carbon Dioxide Exchange in an Alpine Wetland Meadow on the Qinghai-Tibetan Plateau.” Biogeosciences 7: 1207–1221. https://doi.org/10.5194/bg-7-1207-2010.
- Zheng, H., Q. Wang, Y. Li, and X. Zhu. 2013. “Characteristics of Evapotranspiration in an Alpine Shrub Meadow in Haibei, Qinghai of Northwest China.” Chinese Journal of Applied Ecology 24: 3221–3228. https://doi.org/10.13287/j.1001-9332.2013.0549.