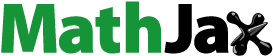
ABSTRACT
China used to have the world’s most stringent prevention policies during the COVID-19 pandemic. The strict lockdown in Xinjiang region in 2022 was considered to be the longest in the process of COVID-19 pandemic prevention. Therefore, we examined the dynamic effects of the COVID-19 lockdown in Xinjiang region using night-time light data. The findings showed that before the outbreak of COVID-19, the night-time light in Xinjiang region still maintained a significant growth. However, after the lockdown of COVID-19 in August 2022, the night-time light showed a significant decrease, especially in August. Urumqi's night-time light decreased by at least 25% compared to the non-lockdown, while that in other major cities was between 5% and 15%. After the lockdown was lifted in November 2022, the night-time light in Urumqi increased by at least 50% compared to the lockdown, while that in other major cities was generally less than 30%. The regions where night-time light decreased in Urumqi were mainly within a radius of 40 km, whereas that in other cities were mainly concentrated within a radius of 6 km. The changes in statistical data and night-time light indicated that the economic activities in Xinjiang region were significantly affected by the COVID-19 lockdown.
1. Introduction
The world economy has entered a profound recession as a result of COVID-19, which has presented a significant threat to public health globally (Beyer, Jain, and Sinha Citation2020). Since the end of 2019, there has been an alarmingly increasing number of verified and deadly COVID-19 cases worldwide (Lu et al. Citation2022). The tremendous threat to the world’s stability and security posed by COVID-19 has led to a massive public health emergency on a global scale. Governments from all over the world were forced to intervene during the early stages of the COVID-19 outbreak by limiting travel and decreasing contact to contain the pandemic (Anil and Alagha Citation2021; Lian et al. Citation2020; Teufel et al. Citation2021). Several intervention measures, which have successfully stopped the expansion of the pandemic, have also dramatically altered urban dwellers’ daily lives and social production activities, and have had a negative influence on the environment and different socioeconomic systems (Beyer, Jain, and Sinha Citation2020; Jallu et al. Citation2022; Mazhar et al. Citation2021). Effective evaluation and monitoring of the effects of pandemic containment can provide useful experience for future public safety situations.
Remote sensing is frequently used to track and assess the effects of COVID-19 on resident life because of its remarkable benefits of vast coverage, rapid return cycles, and low cost (Louw et al. Citation2022; Zheng et al. Citation2023). Previous research has mainly focused on four aspects of remote sensing. Firstly, remote sensing data and geographic information data have been used to analyse the impact of COVID-19 on human life (Ahasan et al. Citation2020; Dahu et al. Citation2023) and to explore the relationship between pandemic management and economic development (Franch-Pardo et al. Citation2020; Kanga et al. Citation2020; Xiang et al. Citation2021). Secondly, night-time light remote sensing has been used to explore the expansion and development level of urban built-up area (Huang et al. Citation2014; Yang et al. Citation2021; Zhang and Seto Citation2011; Zheng, Weng, and Wang Citation2021), estimate population and gross domestic product (GDP) scale (Beyer, Franco-Bedoya, and Galdo Citation2021; Elvidge et al. Citation2009; Zhang et al. Citation2022), and monitor the impact of natural disasters. Thirdly, night-time light remote sensing has also been used to assess the impact of pandemic transmission on economic development and provide suggestions for decision-making (Alahmadi et al. Citation2023; Hayakawa, Keola, and Urata Citation2022; Li et al. Citation2023; Zhao et al. Citation2022). Fourthly, remote sensing has been used to assess the degree of night-time light changes and restoration in different countries and cities (Dasgupta Citation2022; Deng et al. Citation2022; Roberts Citation2021; Xu et al. Citation2021), as well as the impact of lockdown on production and daily life (Alahmadi et al. Citation2023; Jiang and Liu Citation2023; Safarrad, Ghadami, and Dittmann Citation2022; Wang et al. Citation2022; Wu et al. Citation2022).
Night-time light remote sensing research based on the unique calamity of the pandemic has produced some interesting results. However, it is challenging to adequately depict the regional differences and benchmark growth of the impact of the pandemic on the urban economy because prior studies have ignored the spatial differences and temporal increase of the absolute levels of night-time light radiation. It is broadly accepted that night-time lights in cities dropped in intensity during the COVID-19 pandemic due to actions such as lockdown, but the spatiotemporal details of these changes remain unknown.
The lockdown in Xinjiang from July to November 2022 was a pandemic prevention practice with the longest duration and the widest control area among the ‘Dynamic Zero-case Policy’ in China after the outbreak of COVID-19. The goals of our current research are as follows: (1) analyse the spatiotemporal changes of night-time light in Urumqi, the core region of Xinjiang, from July to November, 2022, and examine the impact of the pandemic lockdown on human and economic activities; (2) analyse the spatiotemporal changes of night-time light in the Urumqi region prior to the outbreak of COVID-19 and examine the benchmark changes of economic development; (3) examine how the COVID-19 pandemic has influenced economic activity in the Urumqi region, as well as the resultant spatiotemporal variations in night-time light; and (4) determine whether the lockdown had a substantial impact on major cities’ economic operations by analyzing the spatiotemporal variations in night-time light in the Xinjiang region. Thus, we aim to highlight the potential of using night-time light data in the analysis of large-scale pandemic control, enrich the research on the impact of COVID-19 control in China, which is lacking, and provide experience for the handling of future sudden public safety incidents.
2. Materials and methods
2.1. Study area
Located in Northwest China, with a total size of 1.66 million km2, or approximately one-sixth of China’s total geographical area, Xinjiang is the biggest provincial-level administrative entity in China (Liu et al. Citation2023) ((a)). According to the statistics from the National Bureau of Statistics of China, Xinjiang had a permanent population of 25.89 million in 2021 ((b)) despite having a large area and a small population (Han et al. Citation2022). Urumqi City, situated in the region’s central region, is the political, economic, cultural, scientific, educational, and transportation hub of Xinjiang (Guo et al. Citation2017). Urumqi City had a GDP of approximately 389.3 billion yuan in 2022, the highest among all Xinjiang prefectures and cities, contributing 20.9% to the region’s total economic development (Li, Zhang, and Xu Citation2021). In our research area, the Urumqi region refers to Urumqi City and its closely connected surrounding counties, including Tianshan District (TSD), Shayibak District (SYD), Xinshi District (XSD), Shuimogou District (SMD), Toutunhe District (TTD), Dabancheng District (DBD), Midong District (MDD), Urumqi County (UC), Changji City (CJC), Fukang City (FKC), and Wujiaqu City (WJC).
Figure 1. Geographical location, distribution of population, and newly confirmed cases of COVID-19 in the Xinjiang region: (a) Geographical location; (b) Population density distribution; (c) Distribution of newly confirmed cases.

In 2022, Xinjiang had the longest prevention duration ever recorded in China, as well as the quickest dissemination pace, and the widest coverage of COVID-19 in China, representing a serious public health crisis. On July 30, 2022, a large-scale outbreak of COVID-19 began in Xinjiang. The initial discovery of the pandemic in Xinjiang began in the Ili Prefecture, which quickly spread to 13 provinces and cities, including Tacheng, Kashgar, Bortala, Aksu, Hami, and Urumqi ((c)). On August 10, 2022, Urumqi and even the entire Xinjiang began implementing comprehensive closed management. After more than three months of lockdown, it was lifted across the entire Xinjiang region on November 25, 2022, exceeding the 76-day lockdown of Wuhan in 2020.
2.2. Materials
In April 2012, the National Oceanic and Atmospheric Administration started disseminating NPP/VIIRS (National Polar-orbiting Operational Environmental Satellite System Preparatory Project/Visible and Infrared Imaging Suite) night-time light data to the public. The issue of oversaturated night-time light pixel digital number (DN) values in the metropolitan region is well addressed by this data. The Colorado School of Mines in the United States is the source of the monthly NPP/VIIRS products we utilized (https://eogdata,mines,edu/products/vnl/). We selected the VIIRS Cloud Mask (VCM) version data because they are not influenced by stray light. We utilized the Urumqi region’s limits to crop the matching monthly data for night-time light.
We chose and pre-processed 10 night-time light datasets from July to November 2019, and from July to November 2022 (). Despite radiometric correction for cloud cover pixels being applied to the monthly NPP-VIIRS composite data, the data still exhibited negative and extreme values due to stray light, lightning, moon illumination, and clouds. The radiation value of the image was given a threshold of 1, meaning if it was larger than 1, it was considered a night-time light pixel, and all other pixels were given a value of 0 as the background value. We changed the coordinate system of the data projection into the Asia North Alberts Equal Area Conic projection to prevent the effects of picture grid distortion and to make it easier to calculate the area of each pixel in the image. With a spatial resolution of 500 m, we used the resampling approach including the proximity method, which is appropriate for discrete data processing.
Figure 2. Raw data used in this research: (a) Raw data from July 2019 to November 2019; (b) Raw data from July 2022 to November 2022. Tianshan District (TSD), Shayibak District (SYD), Xinshi District (XSD), Shuimogou District (SMD), Toutunhe District (TTD), Dabancheng District (DBD), Midong District (MDD), Urumqi County (UC), Changji City (CJC), Fukang City (FKC), and Wujiaqu City (WJC).

Chinese provinces, municipalities, and counties comprised the majority of the data on administrative divisions, which were derived from the Resource and Environmental Science and Data Centre of the Chinese Academy of Sciences (https://www.resdc.cn). Each grid of the population spatial data showed the number of individuals living inside a square kilometre. Economic data were obtained from the National Economic Development Report of Major Cities in Xinjiang.
2.3. Methods
Our research was based on monthly data, and there were natural variations in night-time light between different months. We not only calculated the night-time light changes under pandemic control, but we also included such changes without pandemic lockdown and further eliminated the natural changes in night-time light through difference calculation. Thus, this method could not only limit night-time light changes to factors related to pandemic control, but it also eliminated the impact of relative errors in the data itself.
(1) The effects of metropolitan night-time light radiation were intuitively described by the terms total night-time light radiation (TNLR) and mean night-time light radiation (MNLR):
(1)
(1)
(2)
(2) where DNi is the DN value of the ith pixel in Equations 1 and 2, and n is the total number of pixel values in the research region.
(2) Indicators that assessed the impact of COVID-19 lockdowns on cities included night-time light radiation change (NLRC) and night-time light radiation influence (NLRI). We used NLRC to assess variations in nocturnal light radiation, which included not only changes in adjacent months of the same year but also changes in the same month of different years. Based on the basic principle of continuous changes under the rapid urbanization process in China, we used NLRI to evaluate the degree of lockdown impact on Xinjiang before and after COVID-19 lockdown. There was no significant COVID-19 pandemic from August to November 2019, which corresponded to the control period from August to November 2022. To avoid any influence from non-lockdown factors, we used the night-time light during this time as the reference light for regular alterations. In comparison to NLRC, NLRI could lessen the effects of significant variations in night-time light bases and restrict light changes to those that had an influence on pandemic control:
(3)
(3)
(4)
(4)
(5)
(5)
(6)
(6) where NLRC2019 and NLRC2022 represent the difference in monthly night-time light for 2019 and 2022, respectively; NLRCm represents the difference between the night-time light in the same months of 2019 and 2022; MNLRr+1 and MNLRr represent the night-time light from July to November 2022; MNLRj+1 and MNLRj represent the night-time light from July to November 2019, respectively, and NLRI represents the difference in NLRC between 2019 and 2022. If NLRI was less than zero, the pandemic control had a negative effect on changes in night-time light. The stronger the influence on the area during the pandemic lockdown period, the lower the NLRI; if NLRI > 0, it meant that the pandemic control had a positive impact on changes in the night-time light. Similarly, the more the region was affected during lockdown, the higher the NLRI ().
3. Results
3.1. Comparative analysis of MNLR month by month under COVID-19 lockdown
On 30 July 2022, COVID-19 began to spread throughout Xinjiang. As a result, rigorous and comprehensive control measures were put into place on 10 August 2022. Pandemic control in the Urumqi region had an impact on the economy from August to November. Therefore, performing a difference calculation on average night-time light from July to November could directly reflect the impact of pandemic control. As shown in and , when strict control measures were implemented in the Urumqi region in August 2022, MNLR significantly decreased (p < 0.01). Although MNLR in September showed a slight increase, it again experienced a decrease in October. Although Xinjiang only achieved a nationwide lifting of the lockdown on 25 November, MNLR showed an explosive increase in November. Overall, it was clear how the lockdown affected the level of activity among people in the Urumqi region. From the perspective of night-time light alone, due to the population distribution of Urumqi being mostly concentrated in the central urban area (Urumqi has a permanent population of 4 million and an urban population of 3.9 million), controlling the pandemic had a significant impact on human and economic activities.
Figure 4. Monthly NLRC spatial characteristics of the Urumqi region in 2022: (a) July to August 2022; (b) August to September 2022; (c) September to October 2022; (d) October to November 2022. Tianshan District (TSD), Shayibak District (SYD), Xinshi District (XSD), Shuimogou District (SMD), Toutunhe District (TTD), Dabancheng District (DBD), Midong District (MDD), Urumqi County (UC), Changji City (CJC), Fukang City (FKC), and Wujiaqu City (WJC). The night-time light intensities were calculated using a series of 10-km buffer rings with concentric ring analysis.

Figure 5. Monthly NLRC statistical chart of the Urumqi region in 2022. Tianshan District (TSD), Shayibak District (SYD), Xinshi District (XSD), Shuimogou District (SMD), Toutunhe District (TTD), Dabancheng District (DBD), Midong District (MDD), Urumqi County (UC), Changji City (CJC), Fukang City (FKC), and Wujiaqu City (WJC). The night-time light intensities were calculated using a series of 10-km buffer rings with concentric ring analysis.

From the perspective of counties and districts, the most affected areas were the central urban areas of Urumqi (TSD, XSD, SMD), whereas the surrounding counties and districts were less impacted (SYD, TTD, MDD, WJC). NLRC was ranked in descending order as follows: XSD, SMD, TSD, TTD, SYD, DBD, MDD, CJC, FKC, WJC, and UC, with variations ranging from −5 to −0.02. In September, NLRC increased slightly (the magnitude of the increase was between 0.5 and 2.3) in all counties and districts. In October, NLRC slightly decreased again (between −1.4 and 0.8) in various counties and districts in the Urumqi region. In November, MNLR in the Urumqi region showed a comprehensive and explosive increase. TSD, SYD, XSD, SMD, and TTD had the largest growth rates, with increases in NLRC of >7.1, whereas DBD, MDD, UC, CJC, FKC, and WJC had relatively small growth rates, with increases in NLRC between 0.2 and 0.5.
3.2. Comparative analysis of MNLR month by month before COVID-19 outbreak
The absence of a significant COVID-19 pandemic in Xinjiang from August to November 2019 is attributed to the lockdown period from August to November 2022. The variations in night-time light at this time might be seen as typical under regular circumstances. As seen in and , MNLR significantly increased (p < 0.01) before the COVID-19 pandemic in the Urumqi region from August to November 2019; the increase in MNLR was most significant from July to August, with slight increases from August to September and from September to October. MNLR showed a significant increase again from October to November, but the increase was smaller than that from July to August. Overall, the baseline changes in MNLR in the Urumqi region have increased before COVID-19 outbreak. Due to the superior resource conditions and special geographical location of the Xinjiang region, Urumqi had made great achievements in its development before the pandemic, as manifested by the stable growth of night-time light. Especially, between July and October is the peak period for tourism in Xinjiang, with particularly bright night lights.
Figure 6. Monthly NLRC spatial characteristics of the Urumqi region in 2019: (a) July to August 2022; (b) August to September 2022; (c) September to October 2022; (d) October to November 2022. Tianshan District (TSD), Shayibak District (SYD), Xinshi District (XSD), Shuimogou District (SMD), Toutunhe District (TTD), Dabancheng District (DBD), Midong District (MDD), Urumqi County (UC), Changji City (CJC), Fukang City (FKC), and Wujiaqu City (WJC). The night-time lights were calculated using a series of 10-km buffer rings with concentric ring analysis.

Figure 7. Monthly NLRC statistics of the Urumqi region in 2019. Tianshan District (TSD), Shayibak District (SYD), Xinshi District (XSD), Shuimogou District (SMD), Toutunhe District (TTD), Dabancheng District (DBD), Midong District (MDD), Urumqi County (UC), Changji City (CJC), Fukang City (FKC), and Wujiaqu City (WJC).

From the perspective of specific counties and districts, the largest increase in MNLR from July to August 2019 was in the central urban area of the Urumqi region (TSD, XSD, SMD), whereas the surrounding counties and districts were impacted relatively less (DBD, MDD, CJD, FKC, WJC). NLRC was ranked in descending order as follows: XSD, TSD, SMD, TTD, SYD, MDD, WJC, CJC, UC, FKC, and DBD, with a variation range of 0.2–12.8. From August to October 2019, the increase in MNLR was relatively small in each county (between 0.1 and 1.8). The increase in MNLR in the Urumqi region from October to November was significant but smaller than that in August. TSD, SYD, XSD, SMD, and TTD had the largest growth rates, with increases in NLRC all above 2.5, whereas DBD, MDD, UC, CJC, FKC, and WJC had relatively small growth rates, with increases in NLRC ranging from 0.1 to 0.5.
3.3. Comparative analysis of NLRC and NLRI before and after COVID-19 outbreak
3.3.1. Comparative analysis of monthly NLRC
and show that the monthly night-time light for the years 2022 and 2019 exhibited different trends. The increase in night-time light in July dominated the area, indicating that before strict lockdown, Urumqi’s night-time light showed an increasing trend (NLRCm variation = 0.4–7.8). The significant decrease in night-time light radiation in August, September, and October of 2022 indicated that the strict control resulted in Urumqi’s night-time light levels being lower than those in 2019. Compared to November, most of the region had an increase in night-time light radiation, indicating that after the lifting of lockdown in Urumqi, night-time light radiation recovered quickly and was generally developing (NLRCm variation = 0.2–19.7). Furthermore, the night-time light radiation has exceeded the pre-pandemic level in 2019.
Figure 8. Monthly comparison of NLRCm spatial characteristics in the Urumqi region in 2019 and 2022: (a) July 2019 and 2022; (b) August 2019 and 2022; (c) September 2019 and 2022; (d) October 2019 and 2022; (e) November 2019 and 2022. The night-time lights were calculated using a series of 10-km buffer rings with concentric ring analysis.

It is worth noting that three key areas of concern exhibited different characteristics of change: the north of TTD, the west of SMD, and the central part of SYD. These three regions consistently had brighter night lights in 2022 compared to 2019, and it seemed that they were not affected by pandemic control. High-resolution picture comparison showed the creation of a new urban area based on the Xinjiang Production and Construction Corps seen to the north of TTD. In addition, the new campuses of Xinjiang University, Xinjiang Medical University, and Xinjiang Normal University were observed in a newly developed urban region to the west of SMD, as well as a freshly constructed industrial park and logistical area making up the centre of SYD.
3.3.2. Comparative analysis of monthly NLRI
In our comparative analysis of NLRI, we eliminated the natural variations in monthly night-time light through the differences in monthly variations. and show that even after eliminating these natural changes, the night-time light in Urumqi still showed a significant decrease after the pandemic control. Compared to the changes from July to August, the night-time light was a generally significantly decrease, indicating that the restrictions from the strict pandemic control in August 2022 were significant. Compared to the changes from August to September, the region occupied by positive night-time light was relatively large. Night-time light in 2022 experienced a brief recovery, slightly higher than that of 2019, when the pandemic control measures were relatively loose. Compared to the changes from September to October, the area of negative night-time light was relatively large, indicating that night-time light was once again restricted, and pandemic management was once again strict in October. Compared to the changes from October to November, the night-time light was generally significantly positive, indicating that after the lifting of the lockdown in Urumqi, the night-time light had recovered quickly, far exceeding the magnitude of changes in 2019.
Figure 10. Monthly comparison of NLRI spatial characteristics of the Urumqi region: (a) July to August in 2019 and 2022; (b) August to September in 2019 and 2022; (c) September to October in 2019 and 2022; (d) October to November in 2019 and 2022. The night-time lights were calculated using a series of 10-km buffer rings with concentric ring analysis.

Based on concentric circle analysis, from a spatial perspective, we observed that the regions with the most significant changes in night-time light were distributed within a radius of 40 km. Moving away from the city centre, the decrease in night-time light began to decrease, and the impact beyond 40 km was minimal. From a time perspective, we saw that the night-time light in August, September, and October gradually decreased, with the amplitude of the decrease ranked in descending order as August, October, and September, which reflected the changes in the intensity of pandemic control.
3.4. Comparative analysis of monthly NLRI in major cities in Xinjiang region
The top-eight cities with the highest GDP in Xinjiang in 2021 were Urumqi, Korla, Karamay, Changji, Aksu, Kashgar, Yining, and Shihezi. With the exception of Urumqi and Changji, we compared the changes in NLRI in six significant urban regions to determine whether their NLRI was impacted by the lockdown.
As seen in , the night-time light in Korla, Karamay, Aksu, Kashi, and Yining decreased significantly compared to the changes from July to August; compared to the changes from August to September, the area of positive night-time light was relatively large, and compared to the changes from September to October, the area of negative night-time light was relatively large. Compared with the changes from October to November, the night-time light in Korla, Aksu, Kashi, and Yinin still showed a downward trend, and only the night-time light in Shihezi and Karamay increased. These results showed that Shihezi and Karamay recovered quickly after the lockdown was lifted, whereas Korla, Aksu, Kashi, and Yining recovered slowly. It is worth noting that during the lockdown period, Shihezi was always the city that was affected the least. Based on the concentric circle analysis, the regions with significant changes in night-time light were concentrated within a radius of 6 km. Moving away from the city centre, the decrease in night-time light gradually decreased ().
Figure 12. Analysis of NLRI changes in major cities in Xinjiang region. The area of counties and cities in Xinjiang region was generally large, so the figure only showed the city centre for clarity. The nighttime lights were calculated in a series of 1-km buffer rings with concentric ring analysis.

Figure 13. Spatial gradient distribution of nighttime light intensity. The horizontal axis represented the distance to the city centre. The vertical axis represented NLRI. Urumqi was calculated in a series of 5-km buffer rings with concentric ring analysis and the rests were calculated in a series of 1-km buffer rings with concentric ring analysis.

Compared to September 2019 and September 2022, the night-time light in Korla, Karamay, Aksu, Kashgar Yining, and Shihezi decreased by 5.12%, 13.92%, 30.07%, 1.21%, 8.88%, and 7.84%, respectively, whereas the night-time light in Urumqi decreased by 25.38%. The average night-time light in Urumqi increased by 55.72% between November 2019 and November 2022, whereas it decreased in Karamay and Kashgar by 29.42% and 13.94% and increased in Korla, Aksu, Yining, and Shihezi by 11.27%, 2.96%, 18.55%, and 37.28%, respectively.
4. Discussion
4.1. Validation of time series regression models
Even if monthly comparisons lessen the natural change influence, we still seek to improve dependability of variation trend with time series regression models. To perform time series regression analysis from July to November in 2019 and 2022, we utilized five districts in the centre metropolitan region of Urumqi as examples (). The three districts in Urumqi with the highest concentrations of people and the most obvious variations in night-time light were TSD, SYD, and XSD. We observed that the night-time light in July 2022 was still more than that in July 2019, when there was often no lockdown. The night-time light in August 2022 was clearly significantly lower than it was in August 2019 following the rigorous lockdown (Strict pandemic control in the Xinjiang region began in early August 2022 and continued until mid-November 2022). Due to the ongoing lockdown in September and October 2022, there was less night-time light than in the same months in 2019. When the lockdown period ended in mid-November 2022, we observed an instantaneous increase in night-time light radiation.
Figure 14. Regression Model of Night Light Time Series in Main Districts of Urumqi. (a) Tianshan District (TSD); (b) Shayibak District (SYD); (c) Xinshi District (XSD); (d) Shuimogou District (SMD); (e) Toutunhe District (TTD).

In contrast to other places, SMD and TTD consistently maintained the same brightness of night-time light radiation. We discovered that the stable night-time light in these places was consistent with the geographic calculation of and 9 using the time series regression model. The Xinjiang Construction Corps’ built-up area, as well as the three universities and logistics park that were just built, had less of an influence on the night-time light in these two locations. Although the night-time light in 2022 was consistently greater than that in 2019, the night-time light from August to October of 2022 was nevertheless impacted, albeit less so than in other places. These findings mutually validate the patterns of spatial changes in night-time light, and the trend of time series changes in the five districts was highly consistent.
4.2. The relationship between night-time light and economic activities
Since the 1990s, many studies have confirmed that night-time light data have the ability to reflect human socioeconomic activities and can be used as a substitute indicator for the intensity of urban socioeconomic activities (Kim Citation2022; Li et al. Citation2022; Li, Li, and Zhu Citation2022). Due to the spatial information contained in remote sensing data, night-time light remote sensing data have become a link between the physical spatial and socioeconomic aspects of urban development research, providing a good data foundation (Anand and Kim Citation2021; Hayakawa, Keola, and Urata Citation2022; Li et al. Citation2022; Li, Li, and Zhu Citation2022; Ma et al. Citation2023; Straka et al. Citation2020; Tian et al. Citation2021; Xue et al. Citation2022; Yang et al. Citation2021; Zhao et al. Citation2021; Zheng, Weng, and Wang Citation2021). The COVID-19 lockdown restricted residents in walking and driving, and prohibited the operation of public places such as factories, malls, and shops. These actions were thus affected by the lockdown, as reflected by changes in night-time light.
To guarantee the validity of our results and the dependability of the data, we compared them with changes in economic statistics. The statistics included retail sales of consumer goods, accommodation and catering, total tourist volume, and total tourism revenue. There are still no economic statistics available for the Xinjiang region from July to November 2022 due to the delay of gathering statistical data. However, the National Economic and Social Development Statistical Bulletin provided some relevant information. The peak travel season in the Xinjiang region coincided with the lockdown time, which had a direct impact on the services sector. We collected the change rates of economic indicators in major cities in Xinjiang in 2021 and 2022 for comparison before and after the lockdown. shows that the economic indicators in 2022 decreased comprehensively compared to 2021, whereas the economic indicators in 2021 still showed significant growth compared to 2020. In 2022, in Urumqi, Korla, Karamay, Aksu, Kashgar, Yining, and Shihezi, the average decreases in retail sales of consumer goods, accommodation and catering, total tourist volume, and total tourism revenue were −9.8%, −24.1%, −27.4%, and −29.1%, respectively; the average growth rates of the same four indicators in 2021 were 48.6%, 18.9%, 34.5%, and 41.5%, respectively. These differences demonstrate how the massive lockdown in Xinjiang significantly affected economic activity.
Table 1. Change rate of economic indicators in major cities of Xinjiang region %.
4.3. Limitations and prospect
There are many uncertainties regarding the use of night-time light data in pandemic research. For example, the method used to produce night-time light data can overestimate or underestimate the threshold (mean, median, minimum, or maximum) to a certain extent. Most scholars implement denoising, which was also done in this work. In general, our research on large-scale pandemic control using night-time light data is novel. It can quickly, intuitively, objectively, and easily access changes in economic activities, providing a new perspective for the study of public health and safety emergencies. The main goal of this study was to analyse the spatial variations in urban night-time light brightness as affected by the COVID-19 pandemic. However, the quantitative association between changes in night-time light brightness and economic data require further examination in the future, considering the lag in gathering statistical data, in order to inform accurate pandemic prevention and control and lessen societal damage.
5. Conclusions
To determine the extent to which controlling the COVID-19 pandemic influenced economic activity, we aimed to utilize monthly night-time light data to examine the temporal and geographical variations in night-time light radiation in the Xinjiang region. From July 2022 to November 2022, the strict lockdown in Xinjiang region were considered to be the longest in the process of COVID-19 pandemic prevention in China. Spatiotemporal analysis based on night-time light changes showed that before the outbreak of COVID-19, the night-time light in Xinjiang region still maintained a significant growth trend. However, after the lockdown of COVID-19 in August 2022, the night-time light in Xinjiang showed a significant decrease, especially in August. As an important indicator of human activity intensity, Urumqi's night-time light decreased by at least 25% compared to before the outbreak of COVID-19, while that in other major cities was between 5% and 15%. After the lockdown was lifted in November 2022, the night-time light in Urumqi increased by at least 50% compared to the lockdown, while that in other major cities was generally less than 30%.
Based on concentric circle analysis, moving away from the city centre, the decrease in night-time light gradually decreased, indicating that the night-time light in the city centre area had a more significant response to lockdown. The urban area of Urumqi was relatively large, and the regions where night-time light decreased were mainly within a radius of 40 km, whereas that in other cities were mainly concentrated within a radius of 6 km.
The social consumer goods retail, accommodation and catering, total tourist volume, and total tourism income of Urumqi, Korla, Karamay, Aksu, Kashgar, Yining, and Shihezi decreased overall in 2022 compared to 2021 by −9.8%, −24.1%, −27.4%, and −29.1%, while these same indicators still showed significant growth in 2021 compared to 2020, with increases of 48.6%, 18.9%, 34.5%, and 41.5%, respectively. Thus, the changes in statistical data and night-time light indicated that the economic activities in the Xinjiang region were significantly affected by the COVID-19 lockdown.
Acknowledgement
The authors thank the editors and anonymous referees for their valuable comments on an earlier version of this study. The authors thank the Third Xinjiang Comprehensive Scientific Expedition Project and the National Natural Science Foundation of China for their support to this study.
Disclosure statement
No potential conflict of interest was reported by the author(s).
Data availability statement
The NPP/VIIRS monthly products that support the findings of this study are openly available in Earth Observation Group (EOG) at https://eogdata.mines.edu/products/vnl/.
Additional information
Funding
References
- Ahasan, R., S. Alam, T. Chakraborty, and M. M. Hossain. 2020. “Applications of GIS and Geospatial Analyses in COVID-19 Research: A Systematic Review.” F1000Research 9: 1379. https://doi.org/10.12688/f1000research.27544.1.
- Alahmadi, M., S. Mansour, N. Dasgupta, and D. J. Martin. 2023. “Using Nighttime Lights Data to Assess the Resumption of Religious and Socioeconomic Activities Post-COVID-19.” Remote Sensing 15 (4): 1064. https://doi.org/10.3390/rs15041064.
- Anand, A., and D. Kim. 2021. “Pandemic Induced Changes in Economic Activity Around African Protected Areas Captured Through Night-Time Light Data.” Remote Sensing 13 (2): 314. https://doi.org/10.3390/rs13020314.
- Anil, I., and O. Alagha. 2021. “The Impact of COVID-19 Lockdown on the air Quality of Eastern Province, Saudi Arabia.” Air Quality, Atmosphere & Health 14 (1): 117–128. https://doi.org/10.1007/s11869-020-00918-3.
- Beyer, R. C. M., S. Franco-Bedoya, and V. Galdo. 2021. “Examining the Economic Impact of COVID-19 in India Through Daily Electricity Consumption and Nighttime Light Intensity.” World Development 140: 105287. https://doi.org/10.1016/j.worlddev.2020.105287.
- Beyer, R. C. M., T. Jain, and S. J. P. R. W. P. S. Sinha. 2020. “Lights Out? COVID-19 Containment Policies and Economic Activity.” Journal of Asian Economics 85: 101589. https://doi.org/10.1016/j.asieco.2023.101589.
- Dahu, B. M., K. Alaboud, A. A. Nowbuth, H. M. Puckett, G. J. Scott, and L. R. Sheets. 2023. “The Role of Remote Sensing and Geospatial Analysis for Understanding COVID-19 Population Severity: A Systematic Review.” International Journal of Environmental Research and Public Health 20 (5): 4298. https://doi.org/10.3390/ijerph20054298.
- Dasgupta, N. 2022. “Using Satellite Images of Nighttime Lights to Predict the Economic Impact of COVID-19 in India.” Advances in Space Research 70 (4): 863–879. https://doi.org/10.1016/j.asr.2022.05.039.
- Deng, M., G. Lai, Q. Li, W. Li, Y. Pan, and K. Li. 2022. “Impact Analysis of COVID-19 Pandemic Control Measures on Nighttime Light and air Quality in Cities.” Remote Sensing Applications: Society and Environment 27: 100806. https://doi.org/10.1016/j.rsase.2022.100806.
- Elvidge, C. D., P. C. Sutton, T. Ghosh, B. T. Tuttle, K. E. Baugh, B. Bhaduri, and E. Bright. 2009. “A Global Poverty map Derived from Satellite Data.” Computers & Geosciences 35 (8): 1652–1660. https://doi.org/10.1016/j.cageo.2009.01.009.
- Franch-Pardo, I., B. M. Napoletano, F. Rosete-Verges and L. J. Billa. 2020. Spatial Analysis and GIS in the Study of COVID-19. A Review.” Science of The Total Environment 739: 140033. https://doi.org/10.1016/j.scitotenv.2020.140033.
- Guo, B., Y. Geng, J. Ren, L. Zhu, Y. Liu, and T. Sterr. 2017. “Comparative Assessment of Circular Economy Development in China’s Four Megacities: The Case of Beijing, Chongqing, Shanghai and Urumqi.” Journal of Cleaner Production 162: 234–246. https://doi.org/10.1016/j.jclepro.2017.06.061.
- Han, C., J. Zheng, J. Guan, D. Yu, and B. Lu. 2022. “Evaluating and Simulating Resource and Environmental Carrying Capacity in Arid and Semiarid Regions: A Case Study of Xinjiang, China.” Journal of Cleaner Production 338: 130646. https://doi.org/10.1016/j.jclepro.2022.130646.
- Hayakawa, K., S. Keola, and S. Urata. 2022. “How Effective was the Restaurant Restraining Order Against COVID-19? A Nighttime Light Study in Japan.” Japan and the World Economy 63: 101136. https://doi.org/10.1016/j.japwor.2022.101136.
- Huang, Q., X. Yang, B. Gao, Y. Yang, and Y. Zhao. 2014. “Application of DMSP/OLS Nighttime Light Images: A Meta-Analysis and a Systematic Literature Review.” Remote Sensing 6 (8): 6844–6866. https://doi.org/10.3390/rs6086844.
- Jallu, S. B., R. U. Shaik, R. Srivastav, C. G. Pignatta. 2022. “Assessing the Effect of Covid-19 Lockdown on Surface Urban Heat Island for Different Land use/Cover Types Using Remote Sensing” Energy Nexus 5: 100056. https://doi.org/10.1016/j.nexus.2022.100056.
- Jiang, L., and Y. Liu. 2023. “China’s Largest City-Wide Lockdown: How Extensively Did Shanghai COVID-19 Affect Intensity of Human Activities in the Yangtze River Delta?” Remote Sensing 15 (8): 1989. https://doi.org/10.3390/rs15081989.
- Kanga, S., G. Meraj, M. Farooq, M. S. Nathawat, and S. K. J. Singh. 2020. “Reporting the Management of COVID-19 Threat in India Using Remote Sensing and GIS Based Approach” Geocarto International 37 (5): 1337–1344. https://doi.org/10.1080/10106049.2020.1778106.
- Kim, D. 2022. “Assessing Regional Economy in North Korea Using Nighttime Light.” Asia and the Global Economy 2 (3): 100046. https://doi.org/10.1016/j.aglobe.2022.100046.
- Li, J., S. He, J. Wang, W. Ma, and H. Ye. 2022a. “Investigating the Spatiotemporal Changes and Driving Factors of Nighttime Light Patterns in RCEP Countries Based on Remote Sensed Satellite Images.” Journal of Cleaner Production 359: 131944. https://doi.org/10.1016/j.jclepro.2022.131944.
- Li, C., X. Li, and C. Zhu. 2022b. “Night-Time Skyglow Dynamics During the COVID-19 Epidemic in Guangbutun Region of Wuhan City.” Remote Sensing 14 (18): 4451. https://doi.org/10.3390/rs14184451.
- Li, F., J. Liu, M. Zhang, S. Liao, and W. Hu. 2023. “Assessment of Economic Recovery in Hebei Province, China, Under the COVID-19 Pandemic Using Nighttime Light Data.” Remote Sensing 15 (1): 22. https://doi.org/10.3390/rs15010022.
- Li, P., R. Zhang, and L. Xu. 2021. “Three-Dimensional Ecological Footprint Based on Ecosystem Service Value and Their Drivers: A Case Study of Urumqi.” Ecological Indicators 131: 108117. https://doi.org/10.1016/j.ecolind.2021.108117.
- Lian, X., J. Huang, R. Huang, C. Liu, and T. Zhang. 2020. “Impact of City Lockdown on the Air Quality of COVID-19-hit of Wuhan City.” Science of The Total Environment 742: 140556. https://doi.org/10.1016/j.scitotenv.2020.140556.
- Liu, Y., X. Yuan, J. Li, K. Qian, W. Yan, X. Yang, and X. Ma. 2023. “Trade-Offs and Synergistic Relationships of Ecosystem Services Under Land Use Change in Xinjiang from 1990 to 2020: A Bayesian Network Analysis.” Science of The Total Environment 858: 160015. https://doi.org/10.1016/j.scitotenv.2022.160015.
- Louw, A. S., J. Fu, A. Raut, A. Zulhilmi, S. Yao, M. McAlinn, A. Fujikawa, et al. 2022. “The Role of Remote Sensing During a Global Disaster: COVID-19 Pandemic as Case Study.” Remote Sensing Applications: Society and Environment 27: 100789. https://doi.org/10.1016/j.rsase.2022.100789.
- Lu, H., R. Guan, M. Xia, C. Zhang, C. Miao, Y. Ge, and X. Wu. 2022. “Very High-Resolution Remote Sensing-Based Mapping of Urban Residential Districts to Help Combat COVID-19.” Cities 126: 103696. https://doi.org/10.1016/j.cities.2022.103696.
- Ma, Q., J. Wang, M. Xiong and L. Zhu. 2023. “Air Quality Index (AQI) Did Not Improve During the COVID-19 Lockdown in Shanghai, China, in 2022, Based on Ground and TROPOMI Observations.” Remote Sensing 15 (5): 1295.
- Mazhar, U., S. Jin, M. Bilal, M. A. Ali, and R. J. Khan. 2021. “Reduction of Surface Radiative Forcing Observed from Remote Sensing Data During Global COVID-19 Lockdown.” Atmospheric Research 261 (3): 105729. https://doi.org/10.1016/j.atmosres.2021.105729.
- Roberts, M. 2021. “Tracking Economic Activity in Response to the COVID-19 Crisis Using Nighttime Lights – The Case of Morocco.” Development Engineering 6: 100067. https://doi.org/10.1016/j.deveng.2021.100067.
- Safarrad, T., M. Ghadami, and A. Dittmann. 2022. “Effects of COVID-19 Restriction Policies on Urban Heat Islands in Some European Cities: Berlin, London, Paris, Madrid, and Frankfurt.” International Journal of Environmental Research and Public Health 19 (11): 6579. https://doi.org/10.3390/ijerph19116579.
- Straka, W., S. Kondragunta, Z. Wei, H. Zhang, S. D. Miller, and A. J. R. S. Watts. 2020. “Examining the Economic and Environmental Impacts of COVID-19 Using Earth Observation Data.” Remote Sensing 13 (1): 5. https://doi.org/10.3390/rs13010005.
- Teufel, B., L. Sushama, V. Poitras, T. Dukhan, and G. J. A. Bitsuamlak. 2021. “Impact of COVID-19-Related Traffic Slowdown on Urban Heat Characteristics.” Atmosphere 12 (2): 243. https://doi.org/10.3390/atmos12020243.
- Tian, S., R. Feng, J. Zhao, and L. Wang. 2021. “An Analysis of the Work Resumption in China Under the COVID-19 Epidemic Based on Night Time Lights Data.” ISPRS International Journal of Geo-Information 10 (9): 614. https://doi.org/10.3390/ijgi10090614.
- Wang, Y., F. Teng, M. Wang, S. Li, Y. Lin, and H. Cai. 2022. “Monitoring Spatiotemporal Distribution of the GDP of Major Cities in China During the COVID-19 Pandemic.” International Journal of Environmental Research and Public Health 19 (13): 8048. https://doi.org/10.3390/ijerph19138048.
- Wu, M., H. Ye, Z. Niu, W. Huang, P. Hao, W. Li, and B. Yu. 2022. “Operation Status Comparison Monitoring of China’s Southeast Asian Industrial Parks Before and After COVID-19 Using Nighttime Lights Data.” ISPRS International Journal of Geo-Information 11 (2): 122. https://doi.org/10.3390/ijgi11020122.
- Xiang, L., M. Tang, Z. Yin, and H. Lu. 2021. “The COVID-19 Pandemic and Economic Growth: Theory and Simulation.” Frontiers in Public Health 9: 741525. https://doi.org/10.3389/fpubh.2021.741525.
- Xu, G., T. Xiu, X. Li, X. Liang, and L. Jiao. 2021. “Lockdown Induced Night-Time Light Dynamics During the COVID-19 Epidemic in Global Megacities.” International Journal of Applied Earth Observation and Geoinformation 102: 102421. https://doi.org/10.1016/j.jag.2021.102421.
- Xue, R., S. Wang, S. Zhang, J. Zhan, J. Zhu, C. Gu, and B. Zhou. 2022. “Ozone Pollution of Megacity Shanghai During City-Wide Lockdown Assessed Using TROPOMI Observations of NO2 and HCHO.” Remote Sensing 14 (24): 6344. https://doi.org/10.3390/rs14246344.
- Yang, Y., J. Wu, Y. Wang, Q. Huang, and C. He. 2021. “Quantifying Spatiotemporal Patterns of Shrinking Cities in Urbanizing China: A Novel Approach Based on Time-Series Nighttime Light Data.” Cities 118: 103346. https://doi.org/10.1016/j.cities.2021.103346.
- Zhang, Y., N. Peng, S. Yang, and P. Jia. 2022. “Associations Between Nighttime Light and COVID-19 Incidence and Mortality in the United States.” International Journal of Applied Earth Observation and Geoinformation 112: 102855. https://doi.org/10.1016/j.jag.2022.102855.
- Zhang, Q., and K. C. Seto. 2011. “Mapping Urbanization Dynamics at Regional and Global Scales Using Multi-Temporal DMSP/OLS Nighttime Light Data.” Remote Sensing of Environment 115 (9): 2320–2329. https://doi.org/10.1016/j.rse.2011.04.032.
- Zhao, F., S. Zhang, Q. Du, J. Ding, G. Luan, and Z. Xie. 2021. “Assessment of the Sustainable Development of Rural Minority Settlements Based on Multidimensional Data and Geographical Detector Method: A Case Study in Dehong, China.” Socio-Economic Planning Sciences 78: 101066. https://doi.org/10.1016/j.seps.2021.101066.
- Zhao, F., S. Zhang, D. Zhang, Z. Peng, H. Zeng, Z. Zhao, W. Jin, W. Shen, and W. Liu. 2022. “Illuminated Border: Spatiotemporal Analysis of COVID-19 Pressure in the Sino-Burma Border from the Perspective of Nighttime Light.” International Journal of Applied Earth Observation and Geoinformation 109: 102774. https://doi.org/10.1016/j.jag.2022.102774.
- Zheng, Q., K. C. Seto, Y. Zhou, S. You, and Q. Weng. 2023. “Nighttime Light Remote Sensing for Urban Applications: Progress, Challenges, and Prospects.” ISPRS Journal of Photogrammetry and Remote Sensing 202: 125–141. https://doi.org/10.1016/j.isprsjprs.2023.05.028.
- Zheng, Q., Q. Weng, and K. Wang. 2021. “Characterizing Urban Land Changes of 30 Global Megacities Using Nighttime Light Time Series Stacks.” ISPRS Journal of Photogrammetry and Remote Sensing 173: 10–23. https://doi.org/10.1016/j.isprsjprs.2021.01.002.