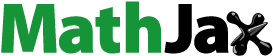
ABSTRACT
The capability of continuous eddy tracking has been significantly advanced over the past decades, thanks to the availability of sea surface height measurements routinely collected by satellite altimeters. However, due to the complexity of eddy kinematic mechanisms and underlying oceanic processes, a medium-range forecast of individual eddy trajectories is a challenging task. This study develops an algorithm to effectively achieve a precise prediction of eddy propagation with a ten-day lead time by building a physics-embedded temporal evolution model. The theoretical eddy phase speed, current field, and eddy properties are incorporated as physical constraints. Compared to only considering eddy past/climatological velocities, the forecast accuracy of embedded physics constraints is improved by 21%. Forecasting of 2.3 million altimeter-derived eddy tracks globally shows that trajectory predictions are valid for eddies moving towards any direction, and the forecast error achieves 9.9 km on a ten-day average. After systematically evaluating the global forecast error, we conclude that the forecasting accuracy is geographically correlated and affected by external forcings (topography and wind) and eddy properties (geostrophy and life stage). Achieving accurate eddy trajectory prediction is expected to provide critical guidance for in-situ eddy observations, and bring substantial improvements for understanding oceanic substance transportations and biogeochemical processes.
1. Introduction
The generic term ‘eddy’ refers to a kind of oceanic storm, with rotational and turbulent flows of vortices, swirls, rings, meanders, and some filaments and wakes (Robinson Citation1983). Oceanic eddies with characteristic spatial scales of tens to hundreds of kilometers, and life spans of weeks to several years while propagating hundreds to thousands of kilometers across the ocean, playing a significant role in transporting climatically important properties such as mass, heat, carbon, and nutrients (e.g. Gaube et al. Citation2014; McGillicuddy Citation2016; Zhang, Wang, and Qiu Citation2014).
Eddy detection based on the sea level anomaly (SLA) became possible in the 1990s with the concurrence of two-satellite altimetry missions (e.g. Le Traon and Ogor Citation1998). The merged product of the coordinated tandem satellite mission provided high enough data resolution to enable the detection of mesoscale eddies (Chelton and Schlax Citation2003). The spatial resolution of the merging of two or more satellite products had been interpolated onto a (1/4)° × (1/4)° grid, and the temporal resolution had been increased from a 7-day interval to daily over the global ocean, allowing an oceanic eddy to be individually tracked during its lifetime (Le Traon and Dibarboure Citation2004; Pascual et al. Citation2006). Over the past decades, a growing development has been made to automatically detect and track eddies (e.g. the Okubo–Weiss parameter method, Chelton et al. Citation2007; the winding angle method, Chaigneau, Gizolme, and Grados Citation2008; the flow direction-based methods, Nencioli et al. Citation2010; the SLA-based methods, Chelton, Schlax, and Samelson Citation2011). Compared with other eddy identification methods, Chelton, Schlax, and Samelson (Citation2011) pointed out that the SLA-based method can avoid excess eddy detections and additional noise sources. In addition, some studies have illuminated the potential superiority of manual eddy tracking over automated methodologies. For example, Pilo, Mata, and Azevedo (Citation2015) pointed out that eddies can escape detection in sparsely sampled regions or after the process of merging and splitting. These events can distort the eddy, failing to satisfy the algorithm identification criteria. While manual tracking of eddies has shown promise in capturing eddies for a much longer lifetime. Hughes and Miller (Citation2017) developed a manual eddy tracking method and reported several modon eddy pairs whose propagation speeds are super-fast when geared together. Though the automatic detection of eddies in the SLA field has uncertainties and imperfections due to both the interpolation of the gridded SLA product and the detection algorithm (e.g. Amores et al. Citation2018; Chen et al. Citation2021a; Chen, Chen, and Huang Citation2021b), the SLA-based method is constantly being optimized and has been demonstrated for providing credible enough eddy recognition, and has become the dominant eddy detection and tracking algorithm (e.g. Faghmous et al. Citation2015; Liu et al. Citation2016; Mason, Pascual, and McWilliams Citation2014; Pegliasco et al. Citation2022).
Given the significant progress of SLA-based eddy tracking, eddy propagation has been individually and statistically analyzed in recent decades and highlighted as having a prominent role in transporting heat and substances across the global ocean (e.g. Chelton, Schlax, and Samelson Citation2011; Fu Citation2009; Morrow et al. Citation2004; Zhang, Wang, and Qiu Citation2014). Yet the forecast of eddy propagation is still a challenging task. It is of great scientific importance for gaining insight into eddy dynamics and transportation, and of practical significance for designing ocean observing systems, fishing programs, and probing underwater acoustics (Wang et al. Citation2021). The difficulty in precisely forecasting an eddy trajectory mainly relates to its frequent deviation from the theoretical propagation affected by coupled mechanisms (Chen, Chen, and Cao Citation2022). In addition, the complex process of splitting and merging that occurs during eddy propagation can also pose a challenge to accurately predict their trajectories. A deeper physical understanding of what controls eddy propagation and integrating it into eddy track prediction will be an important step to solving the problem. As briefly summarized below, several investigations have explored the dominant physical mechanisms that control eddy motion in both zonal and meridional propagations. The theoretical framework proposed by McWilliams and Flierl (Citation1979), and Cushman-Roisin, Chassignet, and Tang (Citation1990) documented that nonlinear mesoscale eddies are expected to propagate westward at approximately the long Rossby wave speed driven by the planetary gradient of the Coriolis effect. The advent of TOPEX/Poseidon (T/P) sea surface height (SSH) data prompted Chelton and Schlax (Citation1996) to employ a Radon transform to characterize phase speeds of SSH and firstly noted that the standard theory for freely propagating linear baroclinic Rossby waves may significantly underestimate the observed eddy phase speeds. In parallel, Killworth, Chelton, and Szoeke (Citation1997) pointed out that this major change would be caused by the presence of baroclinic mean flows. Consequently, endeavors were undertaken to reconcile the theoretical predictions of eddy phase speed with observational estimates by incorporating background flow effects (e.g. de Szoeke and Chelton Citation1999). Several recent studies based on both observations and theoretical arguments have demonstrated that eddy propagation characteristics can be well-approximated by the long-wave limit of the baroclinic mode relative to the background mean flow (e.g. Klocker and Abernathey Citation2014; Shi et al. Citation2018; Tulloch, Marshall, and Smith Citation2009; Wang, Jansen, and Abernathey Citation2016). Klocker and Marshall (Citation2014) conducted a comparison between observed zonal eddy speeds and theoretical Rossby wave phase speeds, incorporating the Doppler shift induced by the depth mean flow, revealing a substantial alignment between theoretical predictions and observed velocities. This theoretical prediction of the eddy phase speed serves as a fundamental guide for developing eddy propagation forecasts. In addition to the zonal propagation, it has been recognized that the baroclinic eddy propagation would have a large meridional component due to the rotational advection of the background potential vorticity (PV) (McWilliams and Flierl Citation1979). In subsequent decades, Vandermeirsch, Morel, and Sutyrin (Citation2001) found that besides this direct effect on eddies, a vertically sheared current associated with a baroclinic PV gradient can induce an additional eddy displacement, which could drastically modify the eddy trajectory. This effect was referred to as the ‘baroclinic β effect’ or the ‘effective β vector’ because of its similarity with the planetary β effect (Samelson Citation2010; Vandermeirsch, Morel, and Sutyrin Citation2001). In the case of a zero mean flow, the baroclinic β effect reduces to the β effect, while in the presence of a nonzero mean flow, the PV gradient vector can deviate considerably from the meridional. It is further demonstrated in subsequent studies. For instance, Early, Samelson, and Chelton (Citation2011) pointed out that eddy deflection from the westward could be the effect of vertical shear on the total PV gradient vector from ambient currents. Meanwhile, a recent study found that eddy meridional deflection is more geographically correlated rather than polarity determined, which is actually controlled by the PV gradient (Chen, Chen, and Cao Citation2022). In addition, other mechanisms exist that can affect the propagation of eddies, such as the bottom topography or wind stress (e.g. Jacob, Chassignet, and Dewar Citation2002; Wilson Citation2016). Tulloch et al. (Citation2011) have emphasized that atmospheric wind forcing is an essential and direct driver to generate mesoscale variabilities. Khani, Jansen, and Adcroft (Citation2019) diagnosed the subgrid mesoscale eddy fluxes using a spatial filtering approach and found that there are substantial differences in the characteristics of eddy fluxes between regions of westerly and easterly winds in the presence of topography. These studies have highlighted the important influence of wind fields and topographic effects on both eddy properties and motions. However, these physical constraints are poorly utilized in existing eddy propagating forecast models (e.g. Wang et al. Citation2021).
Several researchers have employed the predictions of eddy propagation by predicting the SLA field based on numerical/artificial intelligence (AI) ocean models (e.g. Ma et al. Citation2019; Masina and Pinardi Citation1994; Robinson et al. Citation1984; Shriver et al. Citation2007). For example, Hurlburt et al. (Citation2008) observed daily forecast errors ranging from 22.5 to 37 km for eddies in the Arabian Sea and the Gulf of Oman using the 1/32° NLOM model. Xu, Wei, and Yan (Citation2019) demonstrated that the generation and propagation of two typical anticyclonic eddies in the South China Sea (SCS) can be well predicted based on the HYCOM model when their amplitudes are greater than 8 cm. Ma et al. (Citation2019) developed a convolutional long short-term memory network (Conv-LSTM) for 7-day prediction of the eddy evolution, achieving a mean eddy center error of 11.2 km. Notably, they highlighted that Conv-LSTM network predictions exhibited closer alignment with observations compared to those generated by the HYCOM model. Nonetheless, these eddy forecasts based on projected SLA field represent an indirect approach to eddy trajectory prediction, since the predicted results were daily SLA snapshots and eddy tracking was further needed to obtain the eddy propagating trajectory. Furthermore, the predictability of dynamical models can only be increased by better initialization, sufficient data and resolution, and computing power (Oey et al. Citation2005). Recently, encouraging progress has been made in eddy trajectory prediction in the regional ocean based on the statistical/deep-learning method. For example, Li et al. (Citation2019) constructed a simple statistical model to conduct a prediction in the northern SCS using eddies’ past positions and surrounding geostrophic current fields. The mean flow advection was taken into account by incorporating the altimeter-derived absolute geostrophic flows for the first time. However, they pointed out that these predictors contributed less to the forecast equations compared to the predictors of the eddy past-day speed and the climatological speed. Based on their prediction model, eddy tracks predicted 1–2 weeks in advance coincide well with the observations, with the overall average forecast error of 27.6 km (1 week) and 42.5 km (2 weeks). Similarly, Wang et al. (Citation2020) forecasted eddy propagation for 1–4 weeks based on the long short-term memory network (LSTM) in the SCS, with zonal and meridional errors of ∼29–47 km and 24–37 km, respectively. Subsequently, Wang et al. (Citation2021) proposed a deep learning framework named MesoGRU for 7-day eddy trajectory prediction in the SCS based on 13 predictors, including the eddy velocity, radius, amplitude, and the SLA imagery information. The smallest prediction errors ranged from 8∼18 km in 1∼7 days.
Despite the certain success of the current eddy trajectory prediction algorithm, the accuracy can be improved. For a 7-day trajectory forecast in the SCS, the present error ranges from ∼18 to ∼28 km. This error represents half of the eddy scale (∼60 km in an ellipse morphology, Han et al. Citation2021), and more than one pixel in high precision numerical model gridded data (e.g. ∼13.6 km in 1/8° grid, and ∼9.1 km in 1/12° grid), which would introduce uncertainties into subsequent studies. The potential for optimizing eddy trajectory prediction remains substantial, provided that the subsequent limitations and shortcomings can be addressed. First, as previously discussed, the incorporated features in the existing algorithms lack physical mechanisms to support accurate eddy forecasts. It is far from sufficient to characterize eddy motions only by their previous velocities and properties. In-depth physical factors, such as the theoretical eddy zonal phase speed and the baroclinic PV gradient, should be considered to provide essential theoretical guidance for eddy trajectory prediction. Second, an effective prediction mechanism for individual eddy trajectories has not been proposed yet. In general, the trajectory prediction approach is to build a model based on all the historical samples in a specific region, and use this model for the prediction of every trajectory within the area (e.g. Wang et al. Citation2020; Citation2021). It is understood that eddy motion is transient, for which models trained on large samples can describe the climatological behavior of an eddy trajectory; however, they inevitably have difficulty depicting the transient state of individual trajectories. More importantly, the westward and eastward propagating eddies are regulated by different principal forcing (e.g. β effect on westward-propagating eddies, and current system on eastward-propagating eddies; Chen, Chen, and Cao Citation2022; Nof Citation1981; Peng et al. Citation2021), and considering all eddy trajectories for the forecast model training will inevitably introduce confusion. An accompanying consequence associated with this is that high accuracy forecasting of a relatively long-time span is challenging to implement. Third, the existing algorithms are limited to specific regional domains (e.g. the SCS, Li et al. Citation2019), thus lacking comprehensive and systematic analyses of the predictability and influencing factors governing global eddy trajectories.
In this study, we aim to design a new eddy trajectory forecast scheme, named the physics-embedded temporal evolution model (PTEM), to provide a highly precise prediction of eddy trajectories in the global ocean. The mesoscale eddies are often described as the ‘weather’ of the ocean, equivalent to the synoptic variability in the atmosphere (Marie et al. Citation2017). According to the World Meteorological Organization, periods of the weather forecasting classification can be divided into short-range (0–3 days), medium-range (4–10 days), and long-range (longer than 10 days), respectively. Due to the nonlinearity and instability of the weather time-scale features, the dynamical predictions would be inaccurate in a few days, making reliable predictability limited to less than 10 days even after several decades of progress (e.g. ECMWF Citation2018; Krishnamurthy Citation2019). Though studies have been reported to provide forecasts of eddy tracks for the next 30 days (e.g. Li et al. Citation2019), the results have errors of above 100 km which have exceeded mesoscale scale magnitudes of O(100 km). A qualitative assessment considers that the forecast error for mesoscale eddy tracks should be at least one order of magnitude smaller than its spatial scale, in other words, the forecast accuracy of mesoscale processes should achieve the submesoscale magnitudes (∼10 km). In this study, we focus on the medium-range forecast (i.e. 10 days) eddy trajectory prediction. It is noteworthy that this 10-day trajectory forecast aligns with the minimum repeat cycle of currently operated altimeters (e.g. T/P, Jason-1/2/3), facilitating reliable observation and validation of mesoscale eddies. The medium-range forecast based on the proposed PTEM method is effectively applied to predict over two million altimeter-derived individual trajectories from 1 January 2010 to 31 May 2020. It can avoid the aforementioned limitations as it combines both physical variables related to eddy propagation and individual track characteristics of eddy motions, and allows a comprehensive forecast verification of global eddy trajectories. The rest of the paper is organized as follows. The datasets are described in Section 2. The eddy detection algorithm and the proposed PTEM scheme for eddy trajectory forecast are presented in Section 3. Section 4 implements the algorithm for the medium-range forecast of individual eddy propagation and further validates the influence of the current field on the eddy trajectory forecast. Global verification and precision assessment of successively predicted trajectories are discussed in Section 5. The examination of eddy trajectory forecast ability on the near-real-time SLA product is discussed in Section 6. Finally, conclusions are given in Section 7. Further tests of eddy trajectory forecast derived from the numerical model and near-real-time SLA fields are provided in Appendix A.
2. Data
2.1. Merged altimeter data
The ‘all-satellite’ merged SLA data from multiple satellite altimeters (T/P, ERS-1/2, ENVISAT, JASON-1/2/3, Sentinel-3A, HY-2A, Saral/AltiKa, Cryosat-2, and GFO missions) generated by the Copernicus Marine Environment Monitoring Service (https://resources.marine.copernicus.eu/product/detail/) on a global 1/4°×1/4° grid are used in this study for a 10-yr period from January 2010 to May 2020.
2.2. Current data
Three current datasets ranging from 1 January 2007 to 31 May 2020 are included and checked for forecasting performance: (1) the three-dimensional (0–5500 m) gridded reanalysis current product from the GLORYS12V1 with a spatial resolution of 1/12°×1/12°; (2) the gridded absolute geostrophic current calculated using the geostrophic principle from the absolute dynamic topography (ADT), with a spatial resolution of 1/4°×1/4°, and (3) the subsurface current data at a depth of 15 m observed by drifting buoys. The first two current datasets were generated by the CMEMS (available at https://resources.marine.copernicus.eu), and the drifter-measured current data were released by the Drifter Data Assembly Center at the NOAA (available at ftp://ftp.aoml.noaa.gov/pub/phod/buoydata/). Detailed descriptions can be found in Amanda, Marie, and Matthieu (Citation2019), CMEMS (Citation2021), and Rick and Luca (Citation2019), respectively.
2.3. Wind and topography data
The wind and topography datasets are employed to examine the external influences on trajectory prediction accuracy. Daily gridded wind products were released by Remote Sensing Systems (http://www.remss.com/measurements/wind/) from WindSat (1 January 2010–31 December 2019, version 7.0.1; Meissner and Wentz Citation2009). The topographical data was the ETOPO1 Arc-Minute Global Relief Model obtained from the NOAA (https://www.ngdc.noaa.gov/mgg/global/), and a detailed description can be found in Amante and Eakins (Citation2009).
2.4. World ocean atlas data
The climatological temperature and salinity gridded dataset derived from the World Ocean Atlas 2018 obtained from the NOAA (available at https://www.ncei.noaa.gov/access/world-ocean-atlas-2018/) is used in this study, which is constructed at 102 standard depth levels upper 5500 m in 1°×1° resolution (Garcia et al. Citation2019).
3. Methods
3.1. Eddy identification and tracking
Mesoscale eddies are detected on gridded 1/4° delayed-time SLA maps using the algorithm developed by Tian et al. Citation2020. Individual SLA fields are firstly spatially high-pass filtered to remove coherent signals with wavelength scales larger than 20° of longitude by 10° of latitude using a Gaussian filter (Chelton, Schlax, and Samelson Citation2011). Note that the SLA fields are segmented into 40 regular blocks with a zonal/meridional spacing of 45°/36° for the parallel eddy detection, which has been proven to significantly reduce the computation complexity compared to previous SLA-based methods at the global scale (Liu et al. Citation2016). Then, we search in these SLA blocks simultaneously for eddy seeds (i.e. local extreme points in each 5 × 5 grid cell) as candidate eddies. SLA contours are calculated at 0.25 cm intervals for levels of the minimum SLA to the maximum SLA to extract eddy boundaries around the eddy seeds (Liu et al. Citation2016). The amplitude is defined as the absolute value of the difference between the SLA at the eddy center and the boundary along the eddy boundary. Only eddies with amplitude larger than 0.4 cm, and containing more than five consecutive pixels within the contour are retained (Pegliasco et al. Citation2022). It has been demonstrated that the 0.4 cm threshold of eddy amplitude is a more reasonable compromise between the time of computation and the size of the detected eddies compared to the noise and the resolution of the altimetry maps. Meanwhile, the effectivity of the 0.4 cm threshold as the amplitude criterion has been proven in the construction of various other eddy datasets (e.g. AVISO Citation2022).
In the next step, an automated tracking procedure is adopted to determine the trajectory of each eddy from the detected eddy dataset. For each eddy identified on Day 1 (t1), eddies for the next day (t2) are searched to find the closest one within a search radius of 0.5°. Note that only eddies whose amplitude (A), area (a), and kinetic energy (E) are between 0.25 and 2.75 times of the t1 eddy are considered as next-step candidate eddies (Tian et al. Citation2020). Then, a non-dimensional distance (S) is computed to assess the similarity between the t1 eddy and t2 eddy candidates (Penven et al. Citation2005):
(1)
(1) where Δd, Δa, ΔA, and ΔE are the variations of the distance, area, amplitude, and eddy kinetic energy (EKE) between eddies at times t1 and t2, and d0 = 0.25°, a0 = π×602 km2, A0 = 2 cm, and E0 = 100 cm2/s2 are the characteristic values for the eddy distance, area, amplitude, and EKE, respectively (Mason, Pascual, and McWilliams Citation2014; Penven et al. Citation2005). Finally, the eddy pair that minimizes
is selected to be the same eddy that is tracked from the first step to the second step. Ultimately, t2 eddies with no t1 counterparts are considered new ones. The tracking process is executed iteratively until all identified eddies are examined. In addition to the mentioned filters on detected eddies, we only retain eddies with a minimum lifetime of 30 days, and propagating with a speed smaller than ten times of the Rossby phase speed. The tracked eddy trajectories are visualized in , and the eddy dataset is publicly available at https://data.casearth.cn/en/sdo/detail/5fa668ad1f4600005e005ba3 .
Figure 1. Altimeter-derived mesoscale eddy trajectories tracked globally from 1993 to 2020. Only eddy trajectories that survived longer than 60 days and propagated westward (eastward) with a minimum of 6° (3°) of longitude are presented, and the westward (eastward) propagating eddies are plotted in red and blue (magenta and light blue). AE and CE are anticyclonic and cyclonic eddies, respectively.

It is worth pointing out that both the SLA gridded products and the eddy detection algorithm have limitations. The spatial (∼80–400 km) and temporal (∼10–35 days) decorrelation scales of the merged SLA product will not allow numerous small eddy signals to be captured, especially in the tropical ocean (Ballarotta et al. Citation2019; Pujol et al. Citation2016). It is believed that the accuracy of the eddy trajectory forecast proposed in this study will be further improved when better altimeter measurements become available in the future. Meanwhile, the proposed algorithm has also been validated on eddies tracked based on the model SLA fields (see Appendix A), further demonstrating its stability and robustness, and could serve as a support for eddy studies in ocean models.
3.2. Controlling factor estimates for eddy trajectory forecast
3.2.1. Eddy zonal phase speed predicted by the quasi-geostrophic theory
A physical understanding of what controls eddy propagation is an important step to achieving a credible forecast. Theoretically, eddies will intrinsically drift westward at approximately the long Rossby wave speed (Cushman-Roisin, Chassignet, and Tang Citation1990; Nof Citation1981). According to the Introduction to Geophysical Fluid Dynamics, quasi-geostrophic (QG) linear planetary waves in a continuously stratified fluid satisfy a dispersion relation of the form (Cushman-Roisin and Beckers Citation2011),
(2)
(2) where
is frequency,
is the latitudinal variation of the Coriolis parameter,
and
are the zonal and meridional wavenumber components. Considering the eddy scales, the appropriate wavenumber was found to be proportional to the average diameter of tracked nonlinear coherent eddies from the eddy census (Abernathey and Wortham Citation2015). We define the eddy length scale (Le) as the average radius of all eddies in each 1° × 1° cell. Note that the eddy radius corresponds to the eddy boundary of the closed SLA contour with the maximum average geostrophic current speed. Assuming the eddy stream function can be described by a Gaussian function with an e-folding scale of
Le (Chelton, Schlax, and Samelson Citation2011), the eddy wavenumber is defined as
. The length scale
refers to the first baroclinic Rossby radius of deformation calculated following the method by Chelton, deSzoeke, and Schlax (Citation1998):
(3)
(3)
(4)
(4) where
is the Coriolis parameter, and H is the ocean floor depth.
is the buoyancy frequency,
is the potential density calculated from the WOA 2018 temperature and salinity data using the Gibbs Seawater Oceanographic toolbox (McDougall et al. Citation2009),
is a reference density (1025 kg/m3), g is the gravitational acceleration (9.8 m/s2), and z is the vertical coordinate. It should be noted that f gradually decays to almost zero in the equatorial area, where the f-plane is transformed to the β-plane (Lagerloef et al. Citation1999). Eddy movement speeds at low latitudes are dominant by the β effect, and with increasing latitude, the beta β weakens. Considering the wavenumber of eddies, the zonal phase speed predicted by the QG theory can be expressed as,
(5)
(5) However, in the presence of the baroclinic flow, the standard theory for freely propagating linear baroclinic waves often underestimates the observed phase speeds (Chelton and Schlax Citation1996). As de Szoeke and Chelton (Citation1999) further pointed out, the second mode baroclinic structure of the background flow facilitates faster wave speeds than standard theory. They explored this in the three-layer model and found that the planetary-wave phase speed is the sum of the vertically averaged mean flow and the phase speed of Rossby waves. It is also evident by the observed reversed propagation (i.e. eastward) of mesoscale eddies over the Antarctic Circumpolar Current (ACC) and the western boundary current regions where Doppler shifting by the background mean flow is significant ((a and b)). The advection by the strong eastward mean flow here exceeds the westward propagation speed of baroclinic Rossby waves. Therefore, the linear relation of Equation (5) is considered insufficient to describe the real zonal motions of mesoscale eddies. Klocker and Marshall (Citation2014) argued that eddy phase speeds over most of the ocean are well approximated by the first baroclinic mode Rossby wave phase speed and Doppler shifted by the depth-averaged mean velocity. Shi et al. (Citation2018) pointed out that eddy phase speed is proportional to the intensity of zonal currents with meridional shear. Also, many works have added this new factor to the equation to reveal the eddy propagation relative to the background mean flow (e.g. Killworth and Blundell Citation2007; Tulloch, Marshall, and Smith Citation2009; Wang, Jansen, and Abernathey Citation2016). Therefore, considering the depth-averaged zonal flow (
), we further obtain the eddy zonal phase speeds by the inclusion of the Doppler shift of the background mean flow,
(6)
(6) where
is computed by vertically integrating the vertical variations of the geostrophic velocity related to horizontal potential density gradients based on thermal wind balance (Karsten and Marshall Citation2002):
(7)
(7) The global distributions of the altimeter-observed and theoretically-predicted eddy zonal speed (
) are shown in (c and d). It is found that, over most of the ocean, the observed eddy zonal propagation is westward except near the western boundaries and the ACC. The most prominent region for Doppler shifting is found in the core of the ACC at observed eastward speeds of ∼2 cm/s, while the theoretically-predicted speed is slightly larger (∼3 cm/s) given uncertainties in the intrinsic Rossby wave phase speed (Klocker and Marshall Citation2014). By interpreting eddy phase speed for the dispersive dynamics in the presence of instability, the overall pattern of
is quite consistent with observations for both direction and magnitude, which will provide important guidance for forecasting eddy zonal motions.
Figure 2. Global distributions of phase map of eddies (a) and depth-mean current (b); Altimeter-derived (c) and theoretically-predicted (d) zonal speed of eddy propagation; Azimuth angle of the equatorward (positive) and poleward (negative) deflections of the mesoscale eddy (e) and the PV gradient (f).

3.2.2. Eddy meridional deflection driven by the baroclinic instability of mean flow
In addition to the Doppler effect, the vertical baroclinic instability of the mean flow would alter the background PV gradient, changing the propagation of eddies, particularly for their meridional motion (Chen, Chen, and Cao Citation2022; Samelson Citation2010; Walker and Pedlosky Citation2002). The reason is that the background current changes the ambient vorticity gradient so that the eddy obtains an extra self-propagation term for the north–south motion (Early, Samelson, and Chelton Citation2011; van Leeuwen Citation2007). Therefore, the baroclinic gradient is considered as a factor to reflect eddy meridional deflection. For linear-waves on the large-scale mean flow, the QG interior PV equation is expressed as (Pedlosky Citation1987),
(8)
(8) where
is the stream function for the mean flow. PV and surface buoyancy are both conserved by the advection along the geostrophic flow
(Lapeyre and Klein Citation2006). The zonal/meridional components of the mean flow PV gradient (
,
), determined from the stratified mean vertical velocity, can be expressed as,
(9)
(9)
(10)
(10) The vertical variations of the velocity field are computed based on the thermal wind balance (
) based on the grided Argo hydrological dataset. We finally computed the total PV gradient
by integrating the PV gradient from 2000 m (z1) to the surface layer (z2),
(11)
(11)
(12)
(12) Then, the two orthogonal components are vectorized to obtain the direction and magnitude of the total PV gradient. To reflect the correlation between the meridional drift of eddy propagation and the PV gradient, the meridional deflection angle of the total PV gradient is computed and shown in (f), while (e) shows the corresponding eddy meridional deflection angle. A general similarity can be found between the deflection angle of eddies and the PV gradient, implying the primary order of the vertical shear of current fields to eddy meridional deflection. In areas where eddies are deflected (e.g. the North Pacific, the Southeast Pacific, and the North Atlantic, (e)), the total PV gradient bears a similarly enhanced deflection behavior with a stronger magnitude. Considering the result of this comparison, the magnitude of the total PV gradient (Q) is incorporated into the prediction of eddy meridional motions. It is worth noting that, besides the PVG of the background current, the eddy-induced PVG calculated based on the Ertel’s potential vorticity also plays an important role in affecting its trajectory since this PV anomaly associated with the advection flow (Wu and Emanuel Citation1995). The incorporation of eddy PVG into eddy trajectory prediction would improve the forecast accuracy to a certain extent (not shown). However, given that the dominant factor of the theoretical Rossby wave phase speed for eddy propagation is derived from linearizing the quasi-geostrophic potential vorticity equation, the combination of background PVG and eddy theoretical velocities is considered to be promising guidance for eddy trajectory prediction.
3.2.3. Observed eddy velocities, properties, and horizontal current fields
In addition to the specific physics-guided predictors described above, the prediction model considers the specific characteristics of each eddy to provide individual forecasted trajectories. First, eddy propagation speed on the previous day (), the averaged velocity during the historical modeled days (see detailed definition of the historical modeled days in Section 3.3,
), and the monthly climatological velocity at the previous location of the eddy core (
) in the zonal and meridional components are included. The speed of zonal and meridional eddy propagation is defined as the ratio of the distance traveled by the eddy over the time elapsed of 7 days, so as to reduce the sampling and accidental uncertainties (e.g. errors associated with eddy centroid identification or eddy-eddy interaction). The climatological velocity is obtained by a vector composition of eddy velocity within each 1°×1° cell, which is expected to minimize the effect of high frequency perturbations induced by instantaneous shift of individual eddies. Second, individual characteristics of eddies including amplitude (A) and eddy geostrophic ratio (G) are included. Eddy amplitude has been found to significantly affect eddy propagation for both zonal speeds and meridional deflection based on simulations and observations. Eddies with larger amplitudes tend to have faster phase speed (e.g. Chelton, Schlax, and Samelson Citation2011; Early, Samelson, and Chelton Citation2011; Shi et al. Citation2018), and show an increasing poleward deflection for both polarities (Chen, Chen, and Cao Citation2022). Early, Samelson, and Chelton (Citation2011) speculate that the ‘wave drag’ caused by the Rossby waves may be a reason that slows the propagating speed of eddy with smaller amplitude. The eddy geostrophic ratio is defined as the relative length scale of the ratio of the eddy radius and the Rossby radius of deformation, which reflects the deviation magnitude of the eddy scale relative to the Rossby scale. It is an important quantity in determining horizontal scales which characterize eddy sizes and trapping scales, indicating the scale of eddy instability (Stammer Citation1997). When the eddy geostrophic ratio is less than 1, eddies are more ageostrophic and easily undergo submesoscale instabilities (Chen and Chen Citation2023). It was found that eddies with a larger length scale propagated slightly faster with a more consistent and stable speed (Chelton, Schlax, and Samelson Citation2011; Early, Samelson, and Chelton Citation2011). Our recent work found that the meridional deflection of eddies has a certain correlation with their geostrophic ratio, as eddies with weak geostrophy tend to move poleward irrespective of their polarity. In addition, the spatiotemporal-matched subsurface current field (
) derived from the GLORYS12V1 reanalysis dataset is introduced. It should be noted that instead of using the current field at a fixed horizontal layer, the depth of the vertical eddy core (i.e. the depth corresponding to the most significant vertical potential density anomalies induced by individual eddies, see Chen, Chen, and Huang [Citation2021b] for detailed procedures of eddy core depth determination) is considered as the horizontal layer with the most significant impact on eddy dynamics.
3.3. Physics-embedded temporal evolution model for eddy trajectory forecast
In this section, we introduce the proposed eddy trajectory prediction algorithm for individual eddy tracks based on the predictors described in Section 3.2, which consists of the following three steps.
3.3.1. Optimizing window size for individual eddy tracks
To ensure better performance of the algorithm, a flexible moving window strategy is utilized to extract the most appropriate individual historical trajectories to be used for fitting the trajectory prediction regression model. To be specific, unlike the existing studies that arbitrarily use a fixed modeling period to predict the entire eddy trajectory sets, we find the appropriate window for each eddy track to minimize the predicted trajectory deviation. As an example in (A), excessively long modeling periods tend to incorporate an overload of historical trajectories, confusing the accurate prediction of both the direction and the velocity ((A) [a–b]). In contrast, fewer modeling periods may introduce errors due to insufficient samples ((A) [d]). To ensure an appropriate number of samples and avoid introducing excessively long historical tracks, the flexible modeling window size is set to a range of 5–15 days. Assuming that the prediction starts on day t + 1, the modeling period ranges from t-n to t-2 (6 ≤ n ≤ 16, orange points), and days from t-1 to t (pink points) are used as the test stages. Let and
be the zonal (x) and meridional (y) predictors on day i, the predicted zonal and meridional location,
and
, in the test stages can be expressed as,
(13)
(13)
(14)
(14) where
and
represent the zonal and meridional prediction regression function described in Equation (20), t-n is the starting point of the modeling window (i.e. n is ranged from 6 to 16 and is iterated in a one-day step, see (A)). Suppose
and
is respectively the altimeter-observed eddy zonal and meridional location in the test stages, the testing error corresponding to each window size is estimated by the great circle distance (GCD), expressed as (Baskar Citation2021),
(15)
(15) where
is the radius of the Earth. When the GCD reaches its minimum, the window size (m) is taken as the fitted window to be the typical trajectory prediction component ((A) [c]).
Figure 3. Schematic illustration of the proposed eddy trajectory forecast algorithm. (A) The process of determining an optimizing window size for individual eddy track forecasts. The grey and orange dotted lines represent the historical and modeled eddy trajectories, respectively. The blue and magenta dotted lines represent the two-day forecasted and true eddy trajectories using the corresponding window size. (B) Evolving forecast for medium-range eddy trajectory with ten-day lead time from day t + 1 (e) to day t + 10 (h). The grey and orange dotted lines are the same in (A), the purple lines are the true eddy trajectories and the yellow lines are the forecasted eddy trajectories used as modeled tracks to forecast the next-day eddy location. The green dotted lines are the forecasted eddy position of the corresponding days. (C) The result of the forecasted eddy trajectory.

3.3.2. Evolving forecast for medium-range eddy trajectory
After the modeling window size is determined, the future eddy trajectory is predicted based on the quadratic polynomial regression. Instead of employing the same functional relationship for predicting the future multi-day eddy paths, the function built on a specific historical trajectory only predicts the next eddy stage, and then the predicted eddy stage will be involved in the next model training, so as to develop a temporal evolutionary forecast ((B)). Taking advantage of the optimized modeling window size (m), an eddy trajectory can be temporally evolving forecasted for a medium range with ten days lead time from t + 1 to t + d (d = 10). For clarity, we take to represent the feature pair of the zonal and meridional components on day z. In practice, when it is required to introduce modeling features in the future moments (i.e.
), the
is obtained based on the previous predicted eddy position and the monthly means of climatological fields. For the sake of distinction, we note this part of features as
, and the predicted eddy location from day t + 1 to day t + 10 can be obtained by,
(16)
(16)
(17)
(17) … …
(18)
(18)
(19)
(19) where
is the forecasted eddy zonal and meridional location with j days lead time.
is the zonal and meridional prediction model with j days lead time obtained based on the multiple quadratic regression defined by summing the multiplication between the variables to highlight their characteristics and dynamic interactions. The regression in zonal and meridional are modeled separately for each day. Taking the zonal prediction model on day t + 1 (
) as an example, the modeling process can be described as follows. Let
be the zonal predictors for m day on day t + 1,
can be summarized by a mathematical formula,
(20)
(20) where
is the weight coefficients, q = 7 is the number of the predictors,
is a matrix with size m × 56, which contains the primary, quadratic and cross-multiplicative terms of each predictor in
, and
is the bias coefficient. It's important to highlight that an investigation into the efficacy of employing both primary and cubic functions has been conducted. The results reveal a notable reduction of 16% in the prediction error subsequent to the incorporation of the secondary nonlinear term, indicating the effectiveness of nonlinear quadratic functions in forecasting nonlinear eddy motions. Conversely, the introduction of the more intricate triple term yields negligible alterations in prediction accuracy. Then, the least-squares optimization is used to evaluate the
and b. The main idea is to minimize the squared errors between the true and predicted positions of the tracks within the modeling window. After the optimal coefficients have been determined, the eddy zonal location (
) on day t + 1 can be obtained by substituting the features of day i + 1 (
) into the model
:
(21)
(21) Likewise, the eddy meridional position on day t + 1 can be obtained from the model
based on the meridional predictor yi. Iterating the above process, the zonal and meridional position of the eddy during time t + 2 to t + 10 can be determined ((C)). presents two examples of the forecast of eddy trajectories alongside their corresponding PTEM functions. It can be clearly revealed that for each day of an individual eddy trajectory, the prediction is made by fitting the specific optimal parameters for a function from its previous adjacent historical trajectories. Consequently, the derived predictive values for the given day are seamlessly integrated into the preexisting dataset, thus engendering the formulation of the ensuing day’s predictive function. It is noteworthy to mention that the predictive trajectories in the zonal and meridional dimensions are discretely segregated, employing distinct functional representations. Admittedly, the evolving model process will increase the cost of computing. However, we consider it necessary and effective to improve the trajectory prediction accuracy, especially when eddies deflect in direction or accelerate/decelerate during the predicted path, which is a frequent occurrence in eddy motion under complex oceanic processes.
Figure 4. Example of the medium-range eddy trajectory forecast for an westward propagating AE (a) and eastward propagating CE (b). The bottom panels are the corresponding PTEM model. The is the forecasted eddy zonal (x) and meridional (y) location with j days lead time based on the function
and
. The coefficients in the prediction function are obtained by least squares fitting using the corresponding historical trajectories. The definitions of each variable in the function are detailed in Section 3.2.
![Figure 4. Example of the medium-range eddy trajectory forecast for an westward propagating AE (a) and eastward propagating CE (b). The bottom panels are the corresponding PTEM model. The Pt+j=[pt+jx,pt+jy] is the forecasted eddy zonal (x) and meridional (y) location with j days lead time based on the function ft+jx and ft+jy. The coefficients in the prediction function are obtained by least squares fitting using the corresponding historical trajectories. The definitions of each variable in the function are detailed in Section 3.2.](/cms/asset/020797aa-9577-4e9b-bceb-7d3560cf7ec0/tjde_a_2300325_f0004_oc.jpg)
3.3.3. Model evaluation
The performance of the proposed trajectory prediction algorithm is evaluated based on eddy tracks in 2018 from two perspectives: the integration of diverse physics-guided variables and a comparative analysis against two recently employed deep learning models, namely, Deep Neural Network (DNN) and LSTM (LeCun, Bengio, and Hinton Citation2015), as previously applied to eddy trajectories forecast (Wang et al. Citation2020). Note that the LSTM is built in the classic way of time-series prediction in deep learning (Zhang, Zheng, and Liu Citation2021), while the construction of the DNN model takes inspiration from the temporal evolution prediction scheme proposed in this study. Three measures are computed to provide a broader view of the forecast accuracy: the adjusted determination coefficient (), the averaged great circle distance (
) as defined in Equation (15), and the Pearson correlation coefficient between the true and predicted eddy trajectory. Considering that it is to demonstrate the effectiveness of incorporating physical constraints on track prediction, the
is employed instead of the commonly used determination coefficient (R2), given that
can effectively avoid obtaining a better model result when one includes more predictors even if the extra predictors are random noise by taking into account the sample and independent feature number (
, where n0 and p0 are the sample number and feature number, respectively). While the
and the correlation coefficient are used to assess the prediction performance in terms of the absolute distance error and the morphological similarity between the actual and predicted trajectories, respectively. As summarized in , the inclusion of physical variables progressively enhances prediction performance. The most significant improvement occurs with the incorporation of physical factors (the
increase from 0.76 to 0.88), followed by the inclusion of the current fields (the
increase from 0.76 to 0.83). A comparison between Case 0 and Case 6 suggests an improvement of approximately 7 km in prediction accuracy (the
is reduced from 17.00 km to 10.03 km). It is also evident that the PTEM provides better performance when compared with Case 7 and Case 8 predicted using DNN and LSTM based on the same predictors. Moreover, the PTEM-predicted trajectories exhibit the highest correlation with the actual paths (0.83), illustrating the high consistency between predicted and true trajectory patterns.
Table 1. Performance of the medium-range eddy trajectory forecasting under different conditions.
Then, histograms of the from day 1 to day 10 in different cases are given in (a). As expected, the PTEM can reach the smallest prediction error ranging from 3.25 to 19.93 km in 1–10 days (grey lines), while the largest error occurs when using the LSTM method, ranging from 6.67 to 37.38 km (purple line in (a)), which further demonstrates the performance enhancement of the proposed algorithm. In addition to the absolute distance error, the comparison of statistically-averaged trajectories is an important aspect to assess the model performance. As shown in (b and c), the true and predicted average trajectories of anticyclonic eddies (AEs) in the Northeast Pacific (NEP, (b)) and cyclonic eddies (CEs) in the ACC ((c)) are calculated. These two regions are chosen to reflect both the westward and eastward propagating eddies with equatorward and poleward deflections, respectively. It can be clearly found that the average predicted trajectories in case 6 almost overlap with the true trajectories for both equatorially-deflected AE and polar-deflected CE. In contrast, the average predicted trajectories significantly deviate from the true trajectory when no physical parameters are taken into account. The necessity and validity of the inclusion of the physical predictors are further demonstrated.
Figure 5. (a) Comparisons of the daily-mean distance errors in different cases; (b) Average of the normalized eddy trajectories relative to the initial location of each eddy for AEs in the Northeast Pacific for the true trajectories, forecasted trajectories in Case 0, and forecasted trajectories in Case 6. (c) as in (b), but for CEs in the ACC. Equator. = Equatorward, Pole. = Poleward.

4. Medium-range forecast of individual eddy propagation
To give an intuitive impression of the eddy propagation forecast result, the medium-range predicted tracks in the Northwest Pacific Ocean (NWP) and the Southeast Indian Ocean (SEI) with predominantly westward and eastward eddy motions are shown in (a and b). It is visible that the forecasted eddy propagation is consistent with the altimeter-observed eddy paths for both westward and eastward propagating AEs and CEs. Quantitatively, the ten days averaged GCD between the forecasted and true eddy location in the NWP and the SEI are 5.3 and 6.6 km, respectively, exhibiting slightly lower forecast accuracy in the SEI dominated by eastward propagating eddies. However, the prediction accuracy is not appreciably different for AEs and CEs (5.5 and 6.9 km for AEs, and 5.3 and 6.2 km for CEs in the NWP and the SEI respectively), indicating that the proposed algorithm has no obvious polarity preference. In addition, the scatters between the actual and predicted eddy locations of the longitudes and latitudes appear to fit well ((c–f)), suggesting that the proposed PTEM serves as an effective approach in eddy propagation forecasting.
Figure 6. (a–b) Prediction examples of the medium-range forecasts for eddy tracks in (a) the Northwest Pacific and (b) the Southern Indian Ocean. (c–f) Scatter plots of the true and predicted longitudes (yellow scatters) and latitudes (pink scatters) in the Northwest Pacific (c–d) and the Southern Indian Ocean (e–f).

It should be pointed out that the current field, which modulates eddy motions both through the vertical shear and horizontal advection, is the dominant external forcing in regulating eddy movements (Chen, Chen, and Cao Citation2022), therefore is a critical factor for eddy trajectory forecasting. To provide an in-depth insight into how the background current included in the prediction model affects the eddy track forecast, four typical cases are given in . (a–d) show the predicted trajectories before and after considering the background current for a westward AE and an eastward CE in the NEP and the ACC, respectively. When the factors of background current are absent, the predicted eddy tracks inevitably tend to follow their historical trajectory for both westward and eastward propagating eddies ((a and c)). The predicted trajectories are significantly corrected when the background PV field is incorporated, in which the predicted trajectory almost overlaps with the corresponding true trajectory and moves roughly along the PV gradient contours ((b and d)). Meanwhile, quantified error evaluations show that the mean-flow correction reduces the predicted distance error by more than double (23.42 km to 11.52 km for the AE, and 13.10 km to 5.61 km for the CE). Furthermore, the simultaneous horizontal current advection plays an important role in eddy motions. Though the surface geostrophic current derived from the altimeter has been incorporated into eddy trajectory predictions (Li et al. Citation2019), it is insufficient to characterize the eddy-current field features since eddy motion is accompanied by notable submesoscale, ageostrophic energies (Cheng et al. Citation2020; Zhang et al. Citation2019). We approach the problem by introducing the high resolution, subsurface current field in eddy trajectory forecasting: on the one hand, instead of using the geostrophic current with a spatial resolution of 1/4°, a high precision reanalysis current field with a spatial resolution of 1/12° derived from GLORYS12V1 is employed to provide a better approximation to the real flow field. On the other hand, instead of using the surface current field, the subsurface current at the eddy core depth is used considering the current mainly influences the eddy motions at the depth of their vertical core. Two cases for AE and CE in the two hemispheres that are mainly modulated by horizontal current fields are shown in (e–j), forecasted based on the altimeter-, GLORYS12V1-, and drifter-derived current fields, respectively. The drifters provide the most accurate measurements of the near-surface ocean current velocities in the global ocean (Laurindo, Mariano, and Lumpkin Citation2017), providing us a valuable opportunity to access higher accurate trajectory forecasts as long as they are trapped inside eddies ((e and f)). A visual comparison of the true and predicted trajectories ((g and h)) shows that the predicted trajectories based on the drifter-derived currents match the true trajectories significantly better than those derived by altimeter and GLORYS12V1 ones, and to a considerable extent coincide with the advection of horizontal flow at the depth of its eddy core, as shown by the grey streamlines. The most noticeable deviations are found when using the surface geostrophic current derived from the altimeter data, while the deviations are significantly corrected when using the GLORYS12V1-derived ones. As further depicted in (i and j), the drifter-derived forecasts yield systematically smaller errors than the other two from day 1 to day 10. Calculating the for these two forecasted paths, the error using the altimeter-derived current is up to 3.53 times higher than that of the drifter-derived currents. However, the forecast accuracy is improved significantly when using GLORYS12V1-derived current which is only about 1.35 times larger than the drifter, indicating the validity of improved flow field estimation for enhancing eddy trajectory prediction. Nevertheless, with the continuous progress of current observation, including improvement of drifter coverage and the development of oceanic currents reconstruction techniques (e.g. Sinha and Abernathey Citation2021), further enhancements in eddy forecasting results through the integration of more precise current field are expected.
Figure 7. Case study of the effect of current fields on the eddy trajectory forecasts. (a-b) The true and forecasted trajectories of a westward propagating AE (a) before and (b) after considering the vertical shear of the background mean flow. (c-d) as in (a-b) but an eastward propagating CE. The streamlines represent the background PV gradient contours. (e and f) Eddy trajectory (grey lines) and corresponding matched drifter trajectory (dark blue lines); (g and h) Predicted trajectories for an AE (g) and a CE (h). The black and red lines are the historically modeled and the true eddy trajectories, respectively. The purple, green, and pink lines represent the eddy trajectories forecasted based on the drifter-, the GLORYS12V1-, and the altimeter-derived currents, respectively. The streamlines represent the horizontal subsurface current fields averaged over the predicted 10-day period. (i and j) The corresponding great cycle distance between the true and predicted trajectory of (g and h).

Next, we implement the medium-range prediction for over two million individual eddy tracks from 1 January 2010 to 31 May 2020 to verify the statistical reliability of the proposed forecast algorithm. The ten-day forecasted trajectories are grouped together relative to their predicted beginning positions and are presented in data density maps ((a and b)). A striking full-angle consistency can be observed by comparing the color with the overlaid contours which correspond to the true eddy trajectories, suggesting that the performance of the proposed PTEM forecast algorithm is valid and reliable for eddies moving toward any direction. It becomes more evident by the nearly overlapping averaged predicted and true trajectories (light grey and grey lines in (a and b)). Meanwhile, slight equatorward and poleward propagation trends can be found in both true and forecasted AE and CE trajectories, conforming to the polarity-based meridional eddy propagation, i.e. the small meridional offsets of cyclones and anticyclones are polar-oriented and equatorial-oriented, respectively (Chelton, Schlax, and Samelson Citation2011), exhibiting the polarity sensitivity of the proposed algorithm. Furthermore, a comparison of the hemispherical direction probabilities of eddy propagation for AE and CE in the radar plots suggests that the predicted eddy propagation can be well matched to reality ((c and d)). Notably, the proposed algorithm’s robustness can be further verified by comparing the curves’ deviation in each direction with respect to the center. Specifically, AE has a significant southerly predominance in the northern hemisphere and a significant northerly predominance in the southern hemisphere, showing a more equatorward deflection. The eastward motion with distinct southern hemisphere preference is also evident, due to the large portion of eastward eddies in the ACC driven by the strong current system and wind stress.
Figure 8. (a–b) Density maps of the medium-range predicted eddy trajectory relative to their initial location. Overlaid contours are the corresponding data density of true eddy trajectories. The grey (light grey) lines represent the averaged true (forecasted) eddy trajectories. (c–d) Cumulative directional probability map of global eddy propagation for the predicted and true eddy trajectories in two hemispheres. The top and bottom rows are for AEs and CEs, respectively.

5. Global verifications of successively predicted eddy trajectories
Since the forecast performance of the medium-range eddy propagation has been individually and statistically proven, it is worth validating the complete trajectories of eddies over their entire lifetimes, so as to clearly display a successive, oceanwide pattern of predicted trajectories.
As an example, shows two cases of the successively predicted long-lived AE and CE (LAE and LCE) trajectories at 3-day, 5-day, and 10-day stages. Going through the eight panels, two characteristics become visible. First, the predicted trajectory with a 3-day stage (magenta and blue lines) is highly consistent with the true trajectories (grey lines) of LAE and LCE. As the day stage increases, some burr signals appear in the predicted trajectories, particularly at the eddy turning. The smoothness of the trajectory is an important prediction accuracy measure from a visualization perspective. The smoother the predicted trajectory, the smaller the deviation of the predicted motion from the true one, by which the forecasted eddy propagation always follows the correct trajectory. It is estimated that the averaged distance errors for the three types of stages are 5.6, 7.3, 11.4, and 5.2, 6.7, 9.4 km for the LAE and the LCE, respectively, indicating that short-range eddy motions can be predicted precisely based on the proposed algorithm, while their motions for the medium-range can be predicted accurately despite the relatively larger error. Second, the trajectory prediction is comparably accurate and stable during the maturity stage of the eddy, while tends to become relatively inaccurate during birth and decay. This is evident in the prediction error variations over the eddy’s life cycle ((d and h)). Previous studies have noted that the submesoscale ageostrophic energy level is relatively high in eddies’ formation and decaying phases, and is low in their mature phase. Vulnerability to external deformation and larger energy variations in their formation/decaying phases (Zhang and Qiu Citation2018), provides a theoretical explanation for the relative poor predictability of unstable, weakly geostrophic eddy trajectories, as further discussed in .
Figure 9. Case study of the successively predicted eddy trajectories in 3-day, 5-day, and 10-day steps. (a–c) An AE trajectory that survived 688 days from 16 June 2016 to 5 May 2018. Colored (grey) lines are predicted (true) eddy trajectories; (d) the corresponding distance error of (a–c). (e-h) same as (a-d) but is a CE trajectory that survived 831 days from 22 August 2016 to 1 December 2018.

exhibits the successive trajectories predicted at ten-day steps globally and regionally. Note that tracks displayed globally are randomly sampled at a ratio of 1:2 for clear presentation. A high degree of consistency is observable between the predicted and real trajectories in the global ocean, with the averaged being 9.97 km. The forecast is considered precise enough to monitor eddy activities, given that the distance error is much smaller (by an order of magnitude) than the eddy scale of O (100 km). We further estimate the prediction accuracy of the westward and eastward propagating (i.e. final positions of the eddies relative to their initial locations) eddy trajectories. The averaged
of westward and eastward propagating eddies shows 8.33 and 11.84 km, respectively, indicating that the westward eddies are a bit more predictable than the eastward eddies. In particular, it is estimated that the 7-day averaged GCD in the SCS (100°E∼125°E, 9°S∼36°N; reference to the regional coverage of Wang et al. Citation2021) is 6.63 km, which is significantly smaller than the previously reported forecast error in the SCS of more than ∼13 km (e.g. Li et al. Citation2019; Wang et al. Citation2021). Moreover, the overall pattern of the enlarged typical regions with active eddy motions in different ocean basins ((a–d)) confirms that although eddies may move randomly oceanwide (Ni et al. Citation2020), the proposed forecast algorithm is applicable to eddies in all directions and velocities. A careful comparison between the true and predicted trajectories is supported by the enlarged plots, indicating that most of the predicted trajectories smoothly match the true trajectory throughout the eddy’s lifecycle for both AEs and CEs. The estimated average errors in the four regions are 11.18, 9.36, 9.33, and 10.11 km for AE and 11.34, 9.28, 9.58, and 10.17 km for CE, respectively. The forecast errors in the NWP ((a)) are larger than in the other three ocean basins because of the relatively poor forecasting performance for the eastward propagating eddies in the Kuroshio.
Figure 10. Top panel: global distribution of the true and successively predicted eddy trajectories. The red and blue (orange and green) lines are the true (predicted) trajectories for AEs and CEs, respectively. Purple boxes mark four typical areas with active eddies in the Northwest Pacific (a), the North Atlantic (b), the Southeast Pacific (c), and the South Indian Ocean (d). Lower panels are enlargements of a-d areas.

Another way to validate the predicted trajectories is to compare the eddy propagation speed derived from the predicted tracks with the altimeter-observed ones. To fully reflect the prediction performance, eddy speeds in four directions are calculated separately and shown in . An overall impression from these four panels is that the eddy velocities calculated from the predicted trajectories are stable in all directions without much data noise, and in good agreement with the velocities derived from the real trajectories. Notably, analysis of the SSH signals in longitude–time diagrams and the observed eddy translation speed both reveal that the transition from a waves-dominated field to an eddy-dominated one occurs between latitudes ±25°∼±30° (e.g. ± 30° in Tulloch, Marshall, and Smith Citation2009; 25.5°S in Oliveira and Polito Citation2013; 27°N and 26°S in Chen, Chen, and Cao Citation2022), where eddies almost travel at the phase speed of the baroclinic Rossby waves. This happens to be the latitudinal zone that corresponds to the rapid improvement of the eddy forecast accuracy (see ). Quantitative calculations demonstrate that the difference between the eddy speed obtained from the predicted and the true trajectories is 0.11 km/day for the global average, further confirming the reliability of the trajectory prediction and its applicability to eddy kinematics.
Figure 11. Global distributions of eddy propagation speed derived from the predicted trajectories. Black contours are the corresponding eddy propagation speeds derived from the true trajectories. (a–d) represent the westward, eastward, northward, and southward propagation speed components, respectively.

Figure 12. (a–c) Global distribution of the eddy trajectory forecast error. From top to bottom, rows show the absolute distance error, zonal distance error, and meridional distance error, respectively. (d–f) are the zonal averages of the error corresponding to (a–c).

To perform a comprehensive quantitative assessment of trajectory prediction accuracy at a global scale, the geographical distributions and the corresponding zonal variations of the absolute, zonal, and meridional forecast error averaged per 3°×3° cell are given in . It is estimated that the global averaged distance error is 9.89 km. Further separating (a) into zonal and meridional components reveals the average errors of 6.47 and 6.05 km, respectively. The errors are zonally pronounced in the tropical ocean where eddies move westward without significant meridional deflections ((b)). In contrast, larger meridional errors are evident in regions characterized by strong western boundary currents ((c)). It can be concluded that the forecast error is significantly geographically correlated which is mainly attributed to the following factors. First, the most notable errors occur in the tropical ocean where the altimeter-based eddy detection is less effective due to both the weakened Coriolis force and the insufficient sampling by present-day altimetric technology. It is argued that the minimum altimetrically resolvable scale in the present stage stops at ∼40–60 km (Chelton, Schlax, and Samelson Citation2011; Pujol et al. Citation2016), and Chen, Chen, and Huang (Citation2021b) have reported that more than 40% of eddies in the tropical ocean are actually undetectable by available altimeters. Meanwhile, for those altimetrically identified eddies, an unnegligible portion is misclassified in terms of polarity which is referred to as ‘abnormal eddies’. As noted in recent literature, the abnormal eddies occur in the global ocean accounting for 20–30% (e.g. Liu, Zheng, and Li Citation2021; Ni et al. Citation2021), especially in the tropical ocean within ∼15° (Chen, Chen, and Huang Citation2021b). This limitation and error in eddy tracking induced by the current existing observation capability fundamentally affect the performance of eddy forecasting. Second, external forcings such as the strong current and the topography affect the accuracy of trajectory forecasts. Regions with larger errors of more than 10 km are observed in the active current systems, such as the Kuroshio, the Gulf Stream, the Agulhas Current, and the Brazilian Current, whereas errors are relatively smaller in the eastern Pacific and the eastern Atlantic. Although efforts have been made to incorporate the effects of the current field into the prediction process, the prediction of complex eddy trajectories due to transient current shear (e.g. the clockwise rotating eddy trajectories near the Brazilian Current, Chen, Chen, and Cao Citation2022) should see further improvement as better current measurements become available in the future. Meanwhile, the error is relatively larger in the nearshore regions. It is estimated that the average prediction error reaches ∼15 km when the seafloor depth is less than 1000 m, while for depths greater than 1000 m, the average prediction error is reduced to ∼8 km. Further analysis of the relationship between topography on prediction error can be found in (a). Third, the intrinsic eddy properties (e.g. eddy nonlinearity, eddy geostrophic ratio, lifetime) influence its predictability. Eddy motions become more challenging to predict when they are in an unstable state, with complex dynamics dominated by ageostrophic forces. For example, in the Central North Pacific, there is an unstable ‘eddy pool’ with low eddy nonlinearity (i.e. the ratio of the eddy’s maximum rotational speed and propagation speed) and high ageostrophic energy (Chelton, Schlax, and Samelson Citation2011; Zhang et al. Citation2019), making it relatively tough to forecast such unstable eddy trajectories. Therefore, although the proposed algorithm maximized the eddy forecasting accuracy, practical difficulties exist for eddy track prediction and the entire eddy remote sensing observation from identification to track. It is expected that the next-generation interferometric altimeters (e.g. SWOT, Chinese ‘Guanlan’ mission) with submesoscale ageostrophic motions resolving capability (Chen et al. Citation2019b; Morrow et al. Citation2019) will overcome such eddies’ forecasting challenges.
Figure 13. Variations of averaged eddy trajectory forecast error against (a) seafloor depth, (b) wind speed, (c) eddy geostrophic ratio, and (d) eddy normalized lifetime.

Furthermore, we examine the correspondence between the forecast error to the external forcings (seafloor topography and wind), and the eddy properties (geostrophic ratio, and life stages) (). As previously reported, the local topography affects the propagation of eddies, particularly when encountering slopes, islands, or seamounts during their travels (Jacob, Chassignet, and Dewar Citation2002). This point is partially evidenced by correlating the accuracy of eddy forecasting with the ocean depth. In shallow seas, especially within 500 m, the prediction accuracy is significantly low with an average distance error greater than 15 km ((a)). The forecast error stabilizes when depths exceed 1000 m since the influence of topography on the eddy motion becomes insignificant when the sea depth is much greater than the eddy core depth (∼226 m on the global average, Chen, Chen, and Huang Citation2021b). It has been noted that wind stress plays a nonnegligible role in eddy motions through eddy-wind interactions (e.g. Eden and Dietze Citation2009; Wilson Citation2016). To be specific, eddies are systematically energized when the wind stress shear is in the same direction as their rotation, while are damped if the wind stress shear is in the opposite direction (Xu, Zhai, and Shang Citation2016). This variation of eddy energy will inevitably affect its dynamics and propagating conditions. By estimating the averaged trajectory prediction errors at varying wind speeds, it is found that the prediction error slightly rises as the wind speed increases ((b)). In the meanwhile, the intensity of eddy geostrophic ratio has a significant effect on forecasting accuracy. The correlation between the eddy geostrophic ratio and the shows that the highest prediction accuracy is achieved when the eddy geostrophic ratio equals 1 (i.e. the eddy scale is equal to the Rossby scale, (c)). The forecast error is significant when the eddy is under strong ageostrophic energy, but rapidly decreases when it becomes a geostrophic eddy. Finally, we try to understand how the forecast error varies during eddy’s life cycle by normalizing the error against eddy’s lifetime ((d)). The forecast accuracy oscillates slightly at the beginning of the eddy life cycle. It gradually increases as the eddy goes towards its decaying phase, reflecting that the eddy becomes less stable with the energy gradually dropping, introducing a higher prediction error. In particular, this variation of the eddy trajectory prediction accuracy over the life cycle can be partially mapped to the geographical distribution of the forecast errors. Prediction errors are relatively small in frequent eddy formation regions along the eastern boundaries (e.g. the North Pacific and the North Atlantic), while with the energy dissipation during eddy propagation processes, the prediction accuracy becomes relatively low in the region where eddy decay is more frequent (see (c) in Chen and Han Citation2019a for global distribution of the originations and terminations for long-lived eddies). Further corrections are considered necessary in the future regarding the inclusion of the above-mentioned local oceanic parameters as well as eddy ageostrophic properties and the eddy’s lifecycle in the model.
6. Discussion
Given that the prediction of eddy’s future motions should be applicable to eddies tracked based on the SLA fields that are updated in near-real-time (NRT) and completely devoid of future information, which would provide a higher scientific and practical significance. In this section, we examine the capability of forecasting eddy trajectories on the NRT SLA product with a delay of a few hours to 1 d (Pujol et al. Citation2016). The NRT-derived eddy trajectories (hereafter NRT eddies) are forecasted using the proposed PETM for a medium range with ten days lead time and then compared to their corresponding true trajectories tracked from the SLA in a delayed-time (DT) mode (hereafter DT eddies). The two eddy trajectory datasets are generated and quality-controlled following the methodology developed by Mason, Pascual, and McWilliams (Citation2014) and Pegliasco et al. (Citation2022) ranging from 1 January 2018 to 31 May 2020, which is being updated and available on AVISO. By performing a spatial–temporal matching of NRT eddies and DT eddies, a total of 3611 AE trajectories and 4289 CE trajectories with a life cycle longer than 30 days can be obtained, and the average distance between NRT and DT eddy cores is 17.69 km.
The forecast errors from day 1 to day 10 are computed in two aspects: the distance between forecasted NRT eddy trajectories and true NRT eddy trajectories (GCDNRT), and the distance between forecasted NRT eddy trajectories to the corresponding true DT eddy trajectories (GCDDT). It is estimated that the ten-day averaged GCDNRT and GCDDT are 17.01 and 24.16 km, respectively. As shown in (a), the GCDNRT and GCDDT are in a range of 7.13–26.11 km and 17.05–31.59 km on 1–10 days. More importantly, as the forecast days increase, the differences between the GCDNRT and the GCDDT progressively decrease (from ∼10 km on day 1 decrease to ∼5 km on day 10), indicating that the eddy trajectories predicted by the NRT product tend to be closer to the true DT eddy trajectories. As can be further found in (b–i), several individual eddies moving westward and eastward in both hemispheres are given as examples. A careful look reveals that the predicted eddy trajectories based on the NRT product are initially close to the true NRT eddy trajectories, while appearing to gradually move closer to the true DT eddy trajectories (see (g and e) for examples). In conclusion, even when predicting eddy movement obtained from the NRT products that contain a larger intrinsic mapping error (e.g. Desai and Haines Citation2003), a promising predicted trajectory can be projected and it has a tendency to approach the more accurate true eddy trajectory tracked from the DT products, which not only further demonstrates the effectiveness of the proposed forecast algorithm but also proves from the side of the advantages of the DT SLA products.
Figure 14. (a) The daily-mean NRT eddy trajectory forecast errors from 1 January 2018 to 31 May 2020. The orange and pink lines represent the forecast error compared to the true NRT eddy trajectory and the true DT eddy trajectory, respectively. (b–i) Case studies of forecasting eddy trajectories moving westward (b–e) and eastward (f–i) in the two hemispheres. The first two rows are AEs, and the last two rows are CEs. The black (grey) lines are the historical NRT (DT) eddy trajectories, the pink (orange) lines are the true NRT (DT) eddy trajectories, and the green lines are the forecasted eddy trajectories based on the NRT product.

7. Concluding remarks
In analogy to atmospheric cyclones, the precise forecast of oceanic eddy trajectory is important for monitoring ocean ‘weather’ variability. Using the merged sea level anomaly products from a combination of altimeters from 2010 to 2020, an eddy trajectory forecast algorithm has been developed, which can be used precisely for medium-range eddy track prediction with a ten-day lead time. The results demonstrate the proposed methodology’s effectiveness, reliability, and robustness.
The physics-embedded temporal evolution model is constructed based on the altimeter-derived eddy propagation characteristics and the practical theory of physical oceanography. On the one hand, the integration of theoretical eddy phase speed, background current field, and eddy properties introduces novel constraints for eddy forecasting for the first time. The inclusion of these guiding factors provides an in-depth combination of the linear theory and background current fields on the modulation of the eddy propagating trajectory, resulting in a 21% improvement in forecasting accuracy and a 7 km reduction in great circle distance errors. On the other hand, the flexible modeling window and temporally evolving forecast for individual trajectories are critical strategies for realizing precise forecast, which is a significant solution to avoid errors due to manually selected historical modeled tracks, enabling more flexibility and generalizability of the proposed algorithm to permit trajectory forecasting at the global scale.
Millions of eddy trajectories have been forecasted for a medium range over the last decade, and the forecasts are highly reliable in individual cases and statistical comparisons. The result shows that the great circle distance between the eddy cores of the forecasted and true trajectories is a striking 9.9 km (8.3 and 11.8 km for westward and eastward propagating eddies, respectively) on the ten-day average, which is significant progress compared with the deep learning forecast models reported recently. By predicting the complete trajectories of eddies over their entire lifetimes, the successive patterns of predicted trajectories are presented and validated in the global ocean. We reveal that the trajectory forecast accuracy is significantly geographically correlated. Larger errors can be found in the tropical ocean of more than 12 km, while it is less than 8 km in the extratropical ocean. In particular, in areas with complex eddy trajectories and ageostrophic dominance, relatively large errors occur as well. The seafloor topography and local wind speed are also found to affect prediction accuracy. A more refined and long-range eddy trajectory forecast is expected when further factors are considered (e.g. wind stress, topography, eddy-eddy interactions) and better measurements become available in the future.
Further validation of the proposed eddy trajectory forecasting scheme on the NRT SLA product yields relatively high prediction accuracy. Impressively, the NRT-based forecast trajectories strikingly show a trend toward the real trajectories obtained from the DT product. It is expected to be integrated with eddy identification and tracking algorithms to form a complete closed-loop eddy remote sensing observation system. Moreover, it would hopefully provide a critical enhancement of various sea–air models by injecting future eddy conditions, and is of great benefit to the guidance of the marine fisheries, underwater navigation, and buoy observations.
Author contributions
Xiaoyan Chen performed methodology, software, validation, data curation, visualization, and writing of the original draft. Ge Chen contributed to conceptualization, investigation, writing review and editing, supervision and funding acquisition. Linyao Ge performed the model framework construction and validation. Chuanchuan Cao performed the investigation and validation. Baoxiang Huang performed the methodology and funding acquisition.
Acknowledgments
This research was jointly supported by the Laoshan Laboratory (No. LSKJ202201406), the National Natural Science Foundation of China (Grant Nos. 42030406 and 42276203) and the Laoshan Laboratory (No. LSKJ202204301). We thank the two anonymous reviewers for their valuable comments. We also thank Dr. Amores for providing us the helpful model eddy dataset.
Data availability statement
All data used in the analysis are available in public repositories. The all-satellite merged sea level anomaly gridded products can be downloaded from https://resources.marine.copernicus.eu/?option = com_csw%26view = details%26product_id = SEALEVEL_GLO_PHY_L4_REP_OBSERVATIONS_008_047. The eddy identification and tracking dataset can be obtained from https://data.casearth.cn/en/sdo/detail/5fa668ad1f4600005e005ba3. The near-real-time eddy dataset and the corresponding delayed-time eddy dataset are respectively the Mesoscale Eddy Trajectories Atlas Product Meta3.2exp NRT and the Mesoscale Eddy Trajectory Atlas Product Meta3.2 DT provided by AVISO at https://www.aviso.altimetry.fr/en/data/products/value-added-products/global-mesoscale-eddy-trajectory-product.html. The reanalysis current product from the GLORYS12V1 is available at https://resources.marine.copernicus.eu/. The WOA-2018 temperature and salinity gridded dataset are available at https://www.ncei.noaa.gov/access/world-ocean-atlas-2018/, and the wind and topography data can be obtained from http://www.remss.com/measurements/wind/ and https://www.ngdc.noaa.gov/mgg/global/, respectively.
Disclosure statement
No potential conflict of interest was reported by the author(s).
Additional information
Funding
References
- Abernathey, R., and C. Wortham. 2015. “Phase Speed Cross Spectra of Eddy Heat Fluxes in the Eastern Pacific.” Journal of Physical Oceanography 45 (5): 1285–1301. https://doi.org/10.1175/JPO-D-14-0160.1.
- Amanda, G., D. Marie, and C. Matthieu. 2019. “Product User Manual for Global Ocean Reanalysis Products GLOBAL-REANALYSIS-PHY-001-031.” https://catalogue.marine.copernicus.eu/documents/PUM/CMEMS-GLO-PUM-001-657031.pdf.
- Amante, C., and B. W. Eakins. 2009. “Etopo1 Arc-minute Global Relief Model: Procedures, Data Sources and Analysis.” NOAA Technical Memorandum NESDIS NGDC-24, National Geophysical Data Center, NOAA. https://doi.org/10.7289/V5C8276M.
- Amores, A., G. Jordà, T. Arsouze, and J. Le Sommer. 2018. “Up to What Extent can We Characterize Ocean Eddies Using Present-day Gridded Altimetric Products?” Journal of Geophysical Research: Oceans 123 (10): 7220–7236. https://doi.org/10.1029/2018JC014140.
- AVISO. 2022. “Mesoscale Eddy Trajectories Atlas Product Handbook.” Issue: 1 rev 1. https://www.aviso.altimetry.fr/fileadmin/documents/data/tools/hdbk_eddytrajectory_META3.2_DT.pdf.
- Ballarotta, M., C. Ubelmann, M.-I. Pujol, G. Taburet, F. Fournier, J.-F. Legeais, Y. Faugère, et al. 2019. “On the Resolutions of Ocean Altimetry Maps.” Ocean Science 15:1091–1109. https://doi.org/10.5194/os-15-1091-2019.
- Baskar, A. 2021. “Simple Single and Multi-facility Location Models Using Great Circle Distance.” ITM Web of Conferences 37 (7): 01001. https://doi.org/10.1051/itmconf/20213701001.
- Chaigneau, A., A. Gizolme, and C. Grados. 2008. “Mesoscale Eddies off Peru in Altimeter Records: Identification Algorithms and Eddy Spatio-temporal Patterns.” Progress in Oceanography 79 (2–4): 106–119. https://doi.org/10.1016/j.pocean.2008.10.013.
- Chelton, D. B., R. A. deSzoeke, and M. G. Schlax. 1998. “Geographical Variability of the First Baroclinic Rossby Radius of Deformation.” Journal of Physical Oceanography 11:1443–1451. https://doi.org/10.1175/1520-0485(1998)028,0433:GVOTFB.2.0.CO;2.
- Chelton, D. B., and M. G. Schlax. 1996. “Global Observations of Oceanic Rossby Waves.” Science 272 (5259): 234–238. https://doi.org/10.1126/science.272.5259.234.
- Chelton, D. B., and M. G. Schlax. 2003. “The Accuracies of Smoothed Sea Surface Height Fields Constructed from Tandem Satellite Altimeter Datasets.” Journal of Atmospheric and Oceanic Technology 20 (9): 1276–1302.https://doi.org/10.1175/1520-0426(2003)020<1276:TAOSSS>2.0.CO;2.
- Chelton, D. B., M. G. Schlax, and R. M. Samelson. 2011. “Global Observations of Nonlinear Mesoscale Eddies.” Progress in Oceanography 91 (2): 167–216. https://doi.org/10.1016/j.pocean.2011.01.002.
- Chelton, D. B., M. G. Schlax, R. M. Samelson, and R. A. de Szoeke. 2007. “Global Observations of Large Oceanic Eddies.” Geophysical Research Letters 34 (15): L15606. https://doi.org/10.1029/2007GL030812.
- Chen, X., and G. Chen. 2023. “Quantifying the Degree of Eddy Quasi-geostrophy by Generalizing Rossby Deformation.” Journal of Geophysical Research: Oceans 128 (6): e2023JC019652. https://doi.org/10.1029/2023JC019652.
- Chen, G., X. Chen, and C. Cao. 2022. “Divergence and Dispersion of Global Eddy Propagation from Satellite Altimetry.” Journal of Physical Oceanography 52:705–722. https://doi.org/10.1175/JPO-D-21-0122.1.
- Chen, X., G. Chen, L. Ge, B. Huang, and C. Cao. 2021a. “Global Oceanic Eddy Identification: A Deep Learning Method from Argo Profiles and Altimetry Data.” Frontiers in Marine Science 8:646926. https://doi.org/10.3389/fmars.2021.646926.
- Chen, G., X. Chen, and B. Huang. 2021b. “Independent Eddy Identification with Profiling Argo as Calibrated by Altimetry.” Journal of Geophysical Research: Oceans 126:e2020JC016729. https://doi.org/10.1029/2020JC016729.
- Chen, G., and G. Han. 2019a. “Contrasting Short-lived with Long-lived Mesoscale Eddies in the Global Ocean.” Journal of Geophysical Research: Oceans 124 (5): 3149–3167. https://doi.org/10.1029/2019JC014983.
- Chen, G., J. Tang, C. Zhao, S. Wu, F. Yu, C. Ma, Y. Xu, et al. 2019b. “Concept Design of the “Guanlan” Science Mission: China’s Novel Contribution to Space Oceanography.” Frontiers in Marine Science 6:194. https://doi.org/10.3389/fmars.2019.00194.
- Cheng, Y. H., M. H. Chang, D. S. Ko, S. Jan, M. Andres, A. Kirincich, Y. J. Yang, et al. 2020. “Submesoscale Eddy and Frontal Instabilities in the Kuroshio Interacting with a Cape South of Taiwan.” Journal of Geophysical Research: Oceans 125 (5): e2020JC016123. https://doi.org/10.1029/2020JC016123.
- CMEMS. 2021. “Product User Manual for Sea Level SLA Products (Issue 4.0).” https://catalogue.marine.copernicus.eu/documents/PUM/CMEMS-SL-PUM-008-032-068.pdf.
- Cushman-Roisin, B., and J. M. Beckers. 2011. Introduction to Geophysical Fluid Dynamics: Physical and Numerical Aspects. Cambridge, Massachusetts: Academic press.
- Cushman-Roisin, B., E. P. Chassignet, and B. Tang. 1990. “Westward Motion of Mesoscale Eddies.” Journal of Physical Oceanography 20 (5): 758–768.https://doi.org/10.1175/1520-0485(1990)020<0758:WMOME>2.0.CO;2.
- Desai, S., and B. Haines. 2003. “Near-Real-time GPS-based Orbit Determination and Sea Surface Height Observations from the Jason-1 Mission.” Marine Geodesy 26 (3–4): 383–397. https://doi.org/10.1080/714044528.
- de Szoeke, R. A., and D. B. Chelton. 1999. “The Modification of Long Planetary Waves by Homogeneous Potential Vorticity Layers.” Journal of Physical Oceanography 29 (3): 500–511.https://doi.org/10.1175/1520-0485(1999)029<0500:TMOLPW>2.0.CO;2.
- Early, J. J., R. M. Samelson, and D. B. Chelton. 2011. “The Evolution and Propagation of Quasigeostrophic Ocean Eddies.” Journal of Physical Oceanography 41 (8): 1535–1555. https://doi.org/10.1175/2011JPO4601.1.
- Eden, C., and H. Dietze. 2009. “Effects of Mesoscale Eddy/Wind Interactions on Biological New Production and Eddy Kinetic Energy.” Journal of Geophysical Research 114 (C5): C05023. https://doi.org/10.1029/2008JC005129.
- European Centre for Medium-Range Weather Forecast. 2018. “Annual Report 2017.” European Centre for Medium-Range Weather Forecast, 1–40.
- Faghmous, J. H., I. Frenger, Y. Yao, R. Warmka, A. Lindell, and V. Kumar. 2015. “A Daily Global Mesoscale Ocean Eddy Dataset from Satellite Altimetry.” Scientific Data 2:150028. https://doi.org/10.1038/sdata.2015.28.
- Fu, L.-L. 2009. “Pattern and Velocity of Propagation of the Global Ocean Eddy Variability.” Journal of Geophysical Research 114:C11017. https://doi.org/10.1029/2009JC005349.
- Garcia, H. E., T. P. Boyer, O. K. Baranova, R. A. Locarnini, A. V. Mishonov, A. Grodsky, C. R. Paver, et al. 2019. “World Ocean Atlas 2018: Product Documentation.” In NOAA, edited by A. Mishonov, 20. https://www.ncei.noaa.gov/data/oceans/woa/WOA18/DOC/woa18documentation.pdf.
- Gaube, P., D. McGillicuddy, D. B. Chelton, M. Behrenfeld, and P. Strutton. 2014. “Regional Variations in the Influence of Mesoscale Eddies on Near-surface Chlorophyll.” Journal of Geophysical Research: Oceans 119 (12): 8195–8220. https://doi.org/10.1002/2014JC010111.
- Han, G., F. Tian, C. Ma, and G. Chen. 2021. “The Geometry of Mesoscale Eddies in the South China Sea: Characteristics and Implications.” International Journal of Digital Earth 14 (4): 464–479. https://doi.org/10.1080/17538947.2020.1842523.
- Hughes, C. W., and P. I. Miller. 2017. “Rapid Water Transport by Long-lasting Modon Eddy Pairs in the Southern Midlatitude Oceans.” Geophysical Research Letters 44 (24): 12–375. https://doi.org/10.1002/2017GL075198.
- Hurlburt, H., E. Chassignet, J. Cummings, A. Kara, E. Metzger, J. Shriver, O. Smedstad, A. Wallcraft, and C. Barron. 2008. Eddy Resolving Global Ocean Prediction. Washington, DC: American Geophysical Union Geophysical Monograph Series, 353–381. https://doi.org/10.1029/177GM21.
- Jacob, J. P., E. P. Chassignet, and W. K. Dewar. 2002. “Influence of Topography on the Propagation of Isolated Eddies.” Journal of Physical Oceanography 32 (10): 2848–2869. https://doi.org/10.1175/1520-0485(2002)032<2848:IOTOTP>2.0.CO;2.
- Karsten, R. H., and J. Marshall. 2002. “Testing Theories of the Vertical Stratification of the ACC Against Observations.” Dynamics of Atmospheres and Oceans 36 (1–3): 233–246. https://doi.org/10.1016/S0377-0265(02)00031-3.
- Khani, S., M. F. Jansen, and A. Adcroft. 2019. “Diagnosing Subgrid Mesoscale Eddy Fluxes with and Without Topography.” Journal of Advances in Modeling Earth Systems 11 (12): 3995–4015. https://doi.org/10.1029/2019MS001721.
- Killworth, P. D., and J. R. Blundell. 2007. “Planetary Wave Response to Surface Forcing and Instability in the Presence of Mean Flow and Topography.” Journal of Physical Oceanography 37 (5): 1297–1320. https://doi.org/10.1175/JPO3055.1.
- Killworth, P. D., D. B. Chelton, and R. D. Szoeke. 1997. “The Speed of Observed and Theoretical Long Extratropical Planetary Waves.” Journal of Physical Oceanography 27 (9): 1946–1966. https://doi.org/10.1175/1520-0485(1997)027<1946:TSOOAT>2.0.CO;2.
- Klocker, A., and R. Abernathey. 2014. “Global Patterns of Mesoscale Eddy Properties and Diffusivities.” Journal of Physical Oceanography 44 (3): 1030–1046. https://doi.org/10.1175/JPO-D-13-0159.1.
- Klocker, A., and D. P. Marshall. 2014. “Advection of Baroclinic Eddies by Depth Mean Flow.” Geophysical Research Letters 41 (10): 3517–3521. https://doi.org/10.1002/2014GL060001.
- Krishnamurthy, V. 2019. “Predictability of Weather and Climate.” Earth and Space Science 6 (7): 1043–1056. https://doi.org/10.1029/2019EA000586.
- Lagerloef, G., G. T. Mitchum, R. B. Lukas, and P. P. Niiler. 1999. “Tropical Pacific Near-surface Currents Estimated from Altimeter, Wind, and Drifter Data.” Journal of Geophysical Research 104 (C10): 23313–23326. https://doi.org/10.1029/1999JC900197.
- Lapeyre, G., and P. Klein. 2006. “Dynamics of the Upper Oceanic Layers in Terms of Surface Quasigeostrophy Theory.” Journal of Physical Oceanography 36 (2): 165–176. https://doi.org/10.1175/JPO2840.1.
- Laurindo, L. C., A. J. Mariano, and R. Lumpkin. 2017. “An Improved Near-surface Velocity Climatology for the Global Ocean from Drifter Observations.” Deep Sea Research Part I: Oceanographic Research Papers 124:73–92. https://doi.org/10.1016/j.dsr.2017.04.009.
- LeCun, Y., Y. Bengio, and G. Hinton. 2015. “Deep Learning.” Nature 521 (7553): 436–444. https://doi.org/10.1038/nature14539.
- Le Traon, P. Y., and G. Dibarboure. 2004. “An Illustration of the Unique Contribution of the TOPEX/Poseidon–Jason-1 Tandem Mission to Mesoscale Variability Studies.” Marine Geodesy 27 (1–2): 3–13. https://doi.org/10.1080/01490410490489313.
- Le Traon, P.-Y., and F. Ogor. 1998. “ERS-1/2 Orbit Improvement Using TOPEX/POSEIDON: The 2 cm Challenge.” Journal of Geophysical Research: Oceans 103 (C4): 8045–8057. https://doi.org/10.1029/97JC01917.
- Li, J., G. Wang, H. Xue, and H. Wang. 2019. “A Simple Predictive Model for the Eddy Propagation Trajectory in the Northern South China Sea.” Ocean Science 15 (2): 401–412. https://doi.org/10.5194/os-15-401-2019.
- Liu, Y., G. Chen, M. Sun, S. Liu, and F. Tian. 2016. “A Parallel SLA-based Algorithm for Global Mesoscale Eddy Identification.” Journal of Atmospheric and Oceanic Technology 33:2743–2754. https://doi.org/10.1175/JTECH-D-16-0033.1.
- Liu, Y., Q. Zheng, and X. Li. 2021. “Characteristics of Global Ocean Abnormal Mesoscale Eddies Derived from the Fusion of Sea Surface Height and Temperature Data by Deep Learning.” Geophysical Research Letters 48 (17): e2021GL094772. https://doi.org/10.1029/2021GL094772.
- Ma, C., S. Li, A. Wang, J. Yang, and G. Chen. 2019. “Altimeter Observation-based Eddy Nowcasting Using an Improved Conv-LSTM Network.” Remote Sensing 11 (7): 783. https://doi.org/10.3390/rs11070783.
- Marie, T. A., E. P. Chassignet, B. A. Le, and P. Nadia. 2017. “Modeling and Forecasting the “Weather of the Ocean” at the Mesoscale.” Journal of Marine Research 75 (3): 301–329. https://doi.org/10.1357/002224017821836842.
- Masina, S., and N. Pinardi. 1994. “Mesoscale Data Assimilation Studies in the Middle Adriatic sea.” Continental Shelf Research 14 (12): 1293–1310. https://doi.org/10.1016/0278-4343(94)90049-3.
- Mason, E., A. Pascual, and J. C. McWilliams. 2014. “A New Sea Surface Height–based Code for Oceanic Mesoscale Eddy Tracking.” Journal of Atmospheric and Oceanic Technology 31 (5): 1181–1188. https://doi.org/10.1175/JTECH-D-14-00019.1.
- McDougall, T. J., Rainer Feistel, Frank J Millero, D. R. Jackett, Daniel Wright, B.-A. King, Giles Marion, Chen-Tung Arthur Chen, and Petra Spitzer. 2009. “The International Thermodynamic Equation of Seawater 2010 (TEOS-10): Calculation and Use of Thermodynamic Properties.” Global Ship-based Repeat Hydrography Manual, IOCCP Report No, 14.
- McGillicuddy, D. J., Jr. 2016. “Mechanisms of Physical-biological-biogeochemical Interaction at the Oceanic Mesoscale.” Annual Review of Marine Science 8 (1):125–159. https://doi.org/10.1146/annurev-marine-010814-015606.
- McWilliams, J. C., and G. R. Flierl. 1979. “On the Evolution of Isolated, Nonlinear Vortices.” Journal of Physical Oceanography 9 (6): 1155–1182. https://doi.org/10.1175/1520-0485(1979)009<1155:OTEOIN>2.0.CO;2.
- Meissner, T., and F. J. Wentz. 2009. “Wind Vector Retrievals under Rain with Passive Satellite Microwave Radiometers.” IEEE Transactions on Geoscience and Remote Sensing 47:3065–3083. https://doi.org/10.1109/TGRS.2009.2027012.
- Morrow, R., F. Birol, D. Griffin, and J. Sudre. 2004. “Divergent Pathways of Cyclonic and Anti-Cyclonic Ocean Eddies.” Geophysical Research Letters 31 (24): L24311. https://doi.org/10.1029/2004GL020974.
- Morrow, R., L. L. Fu, F. Ardhuin, M. Benkiran, B. Chapron, E. Cosme, F. d’Ovidio, et al. 2019. “Global Observations of Fine-scale Ocean Surface Topography with the Surface Water and Ocean Topography (SWOT) Mission.” Frontiers in Marine Science 6:232. https://doi.org/10.3389/fmars.2019.00232.
- Nencioli, F., C. Dong, T. Dickey, L. Washburn, and J. C. McWilliams. 2010. “A Vector Geometry–based Eddy Detection Algorithm and its Application to a High-resolution Numerical Model Product and High-frequency Radar Surface Velocities in the Southern California Bight.” Journal of Atmospheric and Oceanic Technology 27 (3): 564–579. https://doi.org/10.1175/2009JTECHO725.1.
- Ni, Q., X. Zhai, X. Jing, and D. Chen. 2021. “Abundant Cold Anticyclonic Eddies and Warm Cyclonic Eddies in the Global Ocean.” Journal of Physical Oceanography 51 (9): 2793–2806. https://doi.org/10.1175/JPO-D-21-0010.1.
- Ni, Q., X. Zhai, G. Wang, and D. P. Marshall. 2020. “Random Movement of Mesoscale Eddies in the Global Ocean.” Journal of Physical Oceanography 50:2341–2357. https://doi.org/10.1175/JPO-D-19-0192.1.
- Nof, D. 1981. “On the β-Induced Movement of Isolated Baroclinic Eddies.” Journal of Physical Oceanography 11 (12): 1662–1672. https://doi.org/10.1175/1520-0485(1981)011<1662:OTIMOI>2.0.CO;2.
- Oey, L.-Y., T. Ezer, G. Forristall, C. Cooper, S. DiMarco, and S. Fan. 2005. “An Exercise in Forecasting Loop Current and Eddy Frontal Positions in the Gulf of Mexico.” Geophysical Research Letters 32:L12611. https://doi.org/10.1029/2005GL023253.
- Oliveira, F. S. C., and P. S. Polito. 2013. “Characterization of Westward Propagating Signals in the South Atlantic from Altimeter and Radiometer Records.” Remote Sensing of Environment 134:367–376. https://doi.org/10.1016/j.rse.2013.03.019.
- Pascual, A., Y. Faugère, G. Larnicol, and P.-Y. Le Traon. 2006. “Improved Description of the Ocean Mesoscale Variability by Combining Four Satellite Altimeters.” Geophysical Research Letters 33 (2): L02611. https://doi.org/10.1029/2005GL024633.
- Pedlosky, J. 1987. Geophysical Fluid Dynamics. New York (NY): Springer-Verlag Press, 710.
- Pegliasco, C., A. Delepoulle, E. Mason, R. Morrow, Y. Faugère, and G. Dibarboure. 2022. “META3.1exp: A New Global Mesoscale Eddy Trajectory Atlas Derived from Altimetry.” Earth System Science Data 14:1087–1107. https://doi.org/10.5194/essd-14-1087-2022.
- Peng, L., G. Chen, L. Guan, and F. Tian. 2021. “Contrasting Westward and Eastward Propagating Mesoscale Eddies in the Global Ocean.” IEEE Transactions on Geoscience and Remote Sensing PP (99): 1–10. https://doi.org/10.1109/TGRS.2021.3095936.
- Penven, P., V. Echevin, J. Pasapera, F. Colas, and J. Tam. 2005. “Average Circulation, Seasonal Cycle, and Mesoscale Dynamics of the Peru Current System: A Modeling Approach.” Journal of Geophysical Research: Oceans 110:C10. https://doi.org/10.1029/2005JC002945.
- Pilo, G. S., M. M. Mata, and J. D. Azevedo. 2015. “Eddy Surface Properties and Propagation at Southern Hemisphere Western Boundary Current Systems.” Ocean Science 11 (4): 629–641. https://doi.org/10.5194/os-11-629-2015.
- Pujol, M.-I., Y. Faugère, G. Taburet, S. Dupuy, C. Pelloquin, M. Ablain, and N. Picot. 2016. “DUACS DT2014: The New Multi-mission Altimeter Data Set Reprocessed Over 20 Years.” Ocean Science 12:1067–1090. https://doi.org/10.5194/os-12-1067-2016.
- Rick, L., and C. Luca. 2019. Global Drifter Program Quality-controlled 6-Hour Interpolated Data from Ocean Surface Drifting Buoys. NOAA National Centers for Environmental Information. Dataset. https://doi.org/10.25921/7ntx-z961.
- Robinson, A. R. 1983. “Overview and Summary of Eddy Science.” In Eddies in Marine Science., edited by A. R. Robinson, 3–15, 609. Springer-Verlag. https://doi.org/10.1007/978-3-642-69003-7_1.
- Robinson, A. R., J. A. Carton, C. N. K. Mooers, L. J. Walstad, E. F. Carter, M. M. Rienecker, J. A. Smith, and W. G. Leslie. 1984. “A Real-time Dynamical Forecast of Ocean Synoptic/Mesoscale Eddies.” Nature 309 (5971): 781–783. https://doi.org/10.1038/309781a0.
- Samelson, R. M. 2010. “An Effective-β Vector for Linear Planetary Waves on a Weak Mean Flow.” Ocean Modelling 32 (3–4): 170–174. https://doi.org/10.1016/j.ocemod.2010.01.006.
- Shi, Y., D. Yang, X. Feng, J. Qi, H. Yang, and B. Yin. 2018. “One Possible Mechanism for Eddy Distribution in Zonal Current with Meridional Shear.” Scientific Reports 8:10106. https://doi.org/10.1038/s41598-018-28465-z.
- Shriver, J. F., H. E. Hurlburt, O. M. Smedstad, A. J. Wallcraft, and R. C. Rhodes. 2007. “1/32° Real-time Global Ocean Prediction and Value-added Over 1/16° Resolution.” Journal of Marine Systems 65 (1–4): 3–26. https://doi.org/10.1016/j.jmarsys.2005.11.021.
- Sinha, A., and R. Abernathey. 2021. “Estimating Ocean Surface Currents with Machine Learning.” Frontiers in Marine Science 8:672477. https://doi.org/10.3389/fmars.2021.672477.
- Stammer, D. 1997. “Global Characteristics of Ocean Variability Estimated from Regional Topex/Poseidon Altimeter Measurements.” Journal of Physical Oceanography 27 (8): 1743–1769. https://doi.org/10.1175/1520-0485(1997)027<1743:GCOOVE>2.0.CO;2.
- Tian, F., D. Wu, L. Yuan, and G. Chen. 2020. “Impacts of the Efficiencies of Identification and Tracking Algorithms on the Statistical Properties of Global Mesoscale Eddies Using Merged Altimeter Data.” International Journal of Remote Sensing 41 (8): 2835–2860. https://doi.org/10.1080/01431161.2019.1694724.
- Tulloch, R., J. Marshall, C. Hill, and K. S. Smith. 2011. “Scales, Growth Rates, and Spectral Fluxes of Baroclinic Instability in the Ocean.” Journal of Physical Oceanography 41 (6): 1057–1076. https://doi.org/10.1175/2011JPO4404.1.
- Tulloch, R., J. Marshall, and K. S. Smith. 2009. “Interpretation of the Propagation of Surface Altimetric Observations in Terms of Planetary Waves and Geostrophic Turbulence.” Journal of Geophysical Research: Oceans 114:C02005. https://doi.org/10.1029/2008JC005055.
- Vandermeirsch, F., Y. Morel, and G. Sutyrin. 2001. “The Net Advective Effect of a Vertically Sheared Current on a Coherent Vortex.” Journal of Physical Oceanography 31 (8): 2210–2225. https://doi.org/10.1175/1520-0485(2001)031<2210:TNAEOA>2.0.CO;2.
- van Leeuwen, P. J. 2007. “The Propagation Mechanism of a Vortex on the β Plane.” Journal of Physical Oceanography 37 (9): 2316–2330. https://doi.org/10.1175/JPO3107.1.
- Walker, A., and J. Pedlosky. 2002. “Instability of Meridional Baroclinic Currents.” Journal of Physical Oceanography 32 (3): 1075–1093. https://doi.org/10.1175/1520-0485(2002)032<1075:IOMBC>2.0.CO;2.
- Wang, L., M. Jansen, and R. Abernathey. 2016. “Eddy Phase Speeds in a Two-layer Model of Quasigeostrophic Baroclinic Turbulence with Applications to Ocean Observations.” Journal of Physical Oceanography 46 (6): 1963–1985. https://doi.org/10.1175/JPO-D-15-0192.1.
- Wang, X., H. Wang, D. Liu, and W. Wang. 2020. “The Prediction of Oceanic Mesoscale Eddy Properties and Propagation Trajectories Based on Machine Learning.” Water 12 (9): 2521. https://doi.org/10.3390/w12092521.
- Wang, X., X. Wang, M. Yu, C. Li, and J. Wu. 2021. “MesoGRU: Deep Learning Framework for Mesoscale Eddy Trajectory Prediction.” IEEE Geoscience and Remote Sensing Letters PP (99). https://doi.org/10.1109/LGRS.2021.3087835.
- Wilson, C. 2016. “Does the Wind Systematically Energize or Damp Ocean Eddies?” Geophysical Research Letters 43:12,538–12,542. https://doi.org/10.1002/2016GL072215.
- Wu, C., and K. Emanuel. 1995. “Potential vorticity Diagnostics of Hurricane Movement. Part 1: A Case Study of Hurricane Bob (1991).” Monthly Weather Review 123 (1): 69–92. http://dx.doi.org/10.1175/1520-0493(1995)123<0069:PVDOHM>2.0.CO;2.
- Xu, D., Z. Wei, and Y. Yan. 2019. “Could the Two Anticyclonic Eddies during Winter 2003/2004 be Reproduced and Predicted in the Northern South China Sea?” Ocean Science 15 (1): 97–111. https://doi.org/10.5194/os-15-97-2019.
- Xu, C., X. Zhai, and X.-D. Shang. 2016. “Work Done by Atmospheric Winds on Mesoscale Ocean Eddies.” Geophysical Research Letters 43:12 174–12 180. https://doi.org/10.1002/2016GL071275.
- Zhang, Z., and B. Qiu. 2018. “Evolution of Submesoscale Ageostrophic Motions through the Life Cycle of Oceanic Mesoscale Eddies.” Geophysical Research Letters 45 (11): 847–11,855. https://doi.org/10.1029/2018GL077852.
- Zhang, Z., B. Qiu, P. Klein, and S. Travis. 2019. “The Influence of Geostrophic Strain on Oceanic Ageostrophic Motion and Surface Chlorophyll.” Nature Communications 10 (1): 2838. https://doi.org/10.1038/s41467-019-10883-w.
- Zhang, Z., W. Wang, and B. Qiu. 2014. “Oceanic Mass Transport by Mesoscale Eddies.” Science 345 (6194): 322–324. https://doi.org/10.1126/science.1252418.
- Zhang, T., X. Zheng, and M. Liu. 2021. “Multiscale Attention-based LSTM for Ship Motion Prediction.” Ocean Engineering 230:109066. https://doi.org/10.1016/j.oceaneng.2021.109066.
Appendix A.
Further testing of the eddy trajectory forecast algorithm based on different SLA fields
A.1. Forecasting eddy trajectories derived from the numerical model SLA fields
In this Appendix, we would like to examine the eddy track forecast performance on eddies identified and tracked from the model SLA fields performed with NEMO-NATL60 model configuration during 1 July 2012–31 August 2013 in the North Atlantic Ocean. The model eddy dataset was generated by Amores et al. (Citation2018), and we predict eddy tracks with lifetimes longer than 90 days (65 AE trajectories and 119 CE trajectories in total). As shown in (a), the predicted trajectories are in good agreement with the true trajectories for both AE and CE. By calculating the distance error, it is found that the accuracy in predicting model eddies trajectories is even smaller than that obtained from the observations (∼8.7 km). Furthermore, the predicted trajectories moving toward different directions (i.e. westward-northward, westward-southward, and eastward) for AE and CE are shown in (b–g), respectively. An overall impression is that the predicted trajectories of these eddies coincide well with the true trajectories, even if a certain degree of path deflection occurs during the prediction process ((c and e)). A relatively larger prediction error is found in the eastward propagating eddies, where the predicted trajectory exhibits some degree of meridional deviation, with the forecast error of ∼9 km ((d and g)). Nevertheless, it can be concluded confidently that the proposed eddy track forecast scheme can be applied to model simulations, which would also hopefully provide a critical enhancement of ocean models by injecting future eddy conditions.
Figure A1. Eddy trajectory forecast derived from the model eddy dataset in the North Atlantic for (a) the geographical distribution of the true and successively predicted eddy trajectories, and (b-g) three examples of the predicted trajectories propagating toward different directions for AE (b-d) and CE (e-g), respectively. Detailed information can be found in the legend.

A.2. Forecasting eddy trajectories derived from the near-real-time SLA fields
Two successively predicted AE and CE trajectories at 10-day steps during their life cycle, as well as all the predicted trajectories in the global ocean, are presented in . It is observed that the NRT-based predicted trajectories are in good agreement with the true trajectories for both AE and CE in the global ocean, demonstrating that the proposed algorithm is a reliable method for real-time prediction of eddy trajectories.
Figure A2. (a–d) Case study of the successively predicted trajectories in 10-day steps for two AEs (a–b) and CEs (c–d). (e) Global distribution of the true and successively predicted eddy trajectories. The grey lines are the true NRT eddy trajectories, and the red and yellow (blue and green) lines are the true DT eddy trajectories and the NRT-based predicted eddy trajectories for AEs (CEs), respectively.
