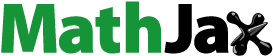
ABSTRACT
Artemia has high commercial and ecological value, but few studies have considered the spectral, spatial and temporal requirements of Artemia multisatellite observations. Adopting Landsat-8 OLI and Sentinel-2 MSI as examples, we mainly analyzed and compared their Artemia slick detection performance considering spectral sensitivity, spatial resolution and revisit cycle. First, the NIR bands of both sensors were most sensitive in Artemia slick detection. Second, compared to that of 2 m GF-6 PMS fusion images, the spatial uncertainty of MSI images was approximately ±16% using the brine shrimp index (BSI) to extract Artemia slicks, better than that of OLI images. The OLI and MSI detection limits under ideal conditions were 0.77% and 1.69%, corresponding to the smallest detectable Artemia slick sizes of approximately 6.93 and 1.69 m2. Third, multisatellite observations could improve dynamic Artemia slick monitoring ability. The OLI and MSI combination could enhance the monthly average observation frequency to 6.58 times. Finally, we examined the influences of the selected near-infrared (or red-edge) bands and Artemia slick width on the extraction results and analyzed detection limit changes and sensor consistency through practical considerations. Overall, these findings could provide support for remote Artemia observation, aiding in fishery management, global mapping and biomass estimation.
1. Introduction
Artemia, also known as brine shrimp, is a genus of aquatic crustaceans that generally occurs in inland salt lakes and coastal saltworks globally, such as the Great Salt Lake in Utah (USA), San Francisco Bay (USA), Chaplin Lake (Canada), Ebinur Lake (China), and Urmia Lake (Iran) (Asem et al. Citation2020; Vanhaecke, Tackaert, and Sorgeloos Citation1987). Artemia has high commercial and ecological value. It is rich in protein, lipids, pigments and other active substances. Artemia cysts can be stored for long periods, making it a leading live food for aquaculture worldwide (Bengtson, Léger, and Sorgeloos Citation2018; Seale Citation1933). With the expansion of aquaculture and hatchery production over the last century, the demand for Artemia has continued to increase (Van Stappen Citation1996). The global output of Artemia dry products is estimated at 3050–4050 t annually (Litvinenko et al. Citation2015), involving a hatchery industry valued at more than $2 billion (Sorgeloos and Roubach Citation2021). What’s more, as a crucial ecological component in salt lakes or coastal waters, Artemia provides rich, high-energy food for birds during their migration and growth (Brown, Bosteels, and Marden Citation2023). Artemia can also filter and feed on microalgae, halophilic bacteria, and other suspended organic matter, thereby purifying water and accelerating the evaporation speed for salt production (Marden, Brown, and Bosteels Citation2020; Veeramani et al. Citation2019). In addition, benefiting from its easy cultivation and small size, Artemia is widely utilized as a model organism in biochemical, physiological, genetic, and toxicology studies (Charoeythornkhajhornchai et al. Citation2023; Dey, Bradley, and Boymelgreen Citation2023). Nevertheless, the population dynamics and abundance of Artemia are greatly influenced by environmental factors, especially salinity (5-200 ppt), oxygen content (low level), and temperature (6-35 °C) (Islam, Kibria, and Bhuyan Citation2019; Van Stappen Citation1996). Due to the unstable annual production of Artemia, the global market is vulnerable to anomalous climate or ecological changes (Vinh et al. Citation2020), leading to a risk of overharvesting (Belovsky and Perschon Citation2019) and a shortage of Artemia resources (Van Stappen et al. Citation2020). Therefore, timely and accurate detection of Artemia growth and its spatial distribution are essential to guide harvest and stabilize the market.
Artemia growth exhibits extremely high spatiotemporal dynamics. Generally, from March to October each year, Artemia rapidly grows in Great Salt Lake and Ebinur Lake (Marden, Brown, and Bosteels Citation2020). It takes 24–48 h for Artemia to hatch from cysts to nauplii (Van Stappen Citation1996), and then the period from nauplii to sexual maturity adult is only 8–14 days (Ogburn et al. Citation2023). Affected by winds, waves and currents, they typically form slender ribbon-like patterns (slicks) several meters in width and hundreds of meters in length ((b)) on the water surface (∼5 cm) and sometimes wash ashore (Van Stappen Citation1996). Moreover, Artemia slicks are reddish brown, with a spectral reflectance significantly different from that of water since they are rich in carotene, heme and other pigments (Gilchrist and Green Citation1960). Therefore, Artemia slicks can be detected by remote sensing.
Figure 1. Partial OLI RGB images on 10 June 2019 and 12 April 2019, showing Artemia features in the Great Salt Lake and Ebinur Lake, respectively. (a) Location of Great Salt Lake and Ebinur Lake; (b) Artemia slicks; (c) Artemia collection by dip nets during the harvest season (∼ October).

According to the Fisheries and Aquaculture Department of the Food and Agriculture Organization of the United Nations (FAO), remote sensing-based Artemia production statistics are currently unavailable for most areas. The absence of public monitoring agencies has also resulted in a lack of production data completeness and reliability (https://www.fao.org/fishery/en/culturedspecies/artemia_spp/en). Previous studies have mainly focused on the habits, density and feeding methods of Artemia but have not quantified these factors macroscopically (e.g. the Artemia slick area). Limited by the coverage of sampling points, traditional manual surveys (Liu et al. Citation2014; Van Stappen, Fayazi, and Sorgeloos Citation2001), which require considerable manpower and material resources, are insufficient to support wide-range Artemia slick detection. With the advantages of large-scale observation, surface spectral acquisition, and high sampling frequency, remote sensing plays an important role in water environment assessment (Wang et al. Citation2018), water-area change analysis (Yu et al. Citation2018), and water-surface floating objects detection (e.g. algae (Hu et al. Citation2019; Huang et al. Citation2015; Qi et al. Citation2019; Wang and Hu Citation2021; Xing et al. Citation2018), plankton (Yamano, Sakuma, and Harii Citation2020), oil (Lu et al. Citation2020; Shi et al. Citation2018), sea snot (Hu et al. Citation2022), plastic litter (Topouzelis, Papakonstantinou, and Garaba Citation2019), and other materials). In addition, the spectral, spatial and temporal requirements for remote floating object detection have also been analyzed (Hu et al. Citation2023; Zhang et al. Citation2022). Therefore, remote sensing could represent a feasible tool for Artemia slick detection and biomass estimation.
Studies on Artemia slick detection in salt lakes by moderate- to high-resolution remote sensors are scarce. Qi et al. (Citation2020) found large red Artemia slicks in Great Salt Lake based on Visible Infrared Imaging Radiometer Suite (VIIRS) (375 m) images and analyzed their spectral shapes. Qi et al. (Citation2021) extracted long-term Artemia cysts in the Great Salt Lake to quantify their abundance in Medium Resolution Imaging Spectrometer (MERIS) (300 m) and Ocean and Land Color Instrument (OLCI) (300 m) images through deep learning. However, most cyst pixels in images were mixed with adjacent water endmembers, resulting in detection difficulty and loss of small cyst features corresponding to a low signal proportion in pixels. Wang et al. (Citation2023) constructed an Artemia-water dataset for Ebinur Lake by HY-1C Coastal Zone Imager (CZI) images and extracted Artemia slicks using U-Net. Tian et al. (Citation2023) proposed the brine shrimp index (BSI) via analogy with the floating algae index (FAI) (Hu Citation2009) based on Landsat-8 Operational Land Imager (OLI) (30 m) images and measured Artemia spectra. They successfully extracted Artemia slicks in Great Salt Lake (USA), Ebinur Lake (China), and Qixiang Cuo (China) using BSI, which was a new pioneering attempt at the remote detection of Artemia slicks. However, it remains uncertain how applicable the BSI is to Sentinel-2 Multispectral Imager (MSI) (10-60 m) image data, and there is no research on Artemia slick detection using multisatellite observations from spectral, spatial, and temporal perspectives.
Accordingly, the objectives of this paper were as follows: 1) analyze Landsat-8 OLI and Sentinel-2 MSI multiband radiometric sensitivity and provide spectral suggestions for Artemia slick detection; 2) evaluate the spatial uncertainty of OLI and MSI based on the area extracted by the BSI algorithm and analyze the spatial detection limit to predict the smallest detectable slick size; and 3) consider the temporal enhancements in time series formation (e.g. effective observation frequency) and slick movement tracking using multiple satellites under highly dynamic Artemia slick variation.
2. Materials and data
2.1. Study area
In this study, Great Salt Lake (112°31′W, 41°08′N) and Ebinur Lake (82°48′E, 45°02′N) were selected as study areas (), both highly representative of the world's major Artemia production sites. Great Salt Lake supplies approximately 90% of global commercial Artemia cyst production (Islam, Kibria, and Bhuyan Citation2019). Ebinur Lake contains the richest Artemia resources in China and serves as a pillar of the local economy. Both lakes occur in the temperate zone with a temperate continental climate. Regarding the Great Salt Lake, our study focused only on its southern half. Since the construction of a rock-filled railroad causeway that bisected Great Salt Lake in 1959, the southern arm is less saline (11-14% salinity), while the northern arm away from freshwater input has become more saline (24–31% salinity) and is unsuitable for Artemia survival (Baxter and Butler Citation2020; Lindsay et al. Citation2017). Moreover, according to the Utah Division of Wildlife Resources (UDWR), most Artemia fishing activities are concentrated in the southern arm.
2.2. Artemia in situ reflectance spectra
Artemia in situ reflectance spectra at different densities were obtained by Tian et al. (Citation2023) through water tank experiments. Since the water surface was nearly completely covered with Artemia at a density of 56 g/L, continued addition of Artemia imposed a negligible impact on the surface reflectance but increased the thickness of the vertical column. The measured reflectance varied slowly at approximately 56 g/L and reached saturation at approximately 120 g/L (). In the end, we collected 19 spectra at different Artemia densities ranging from 0 to 120 g/L (). In this study, the obtained 0 and 120 g/L reflectance spectra were used to represent the typical spectral endmembers of water and Artemia with 0% and 100% Artemia subpixel coverage levels (i.e. χ = 0% and 100%), respectively. Considering the low signal-to-noise ratio (SNR) of the SVC HR-1024 spectrometer beyond 1700 nm, we only retained the data between 400 and 1700 nm.
Figure 2. Typical reflectance spectra and digital photographs of Artemia and water at densities ranging from 0–120 g/L (400-1700 nm) obtained via water tank experiments (Tian et al. Citation2023). The 0 and 120 g/L spectra represent the endmembers of water and Artemia, respectively. Note that, because of the tiled-grating system of the SVC spectrometer, breakpoints occur at 1000 nm, but these exert a negligible effect on the reflectance features of Artemia. In addition, the reflectance of Artemia at high density exceeds 0 near 1600 nm. This phenomenon may be attributed to the high density of Artemia causing them to float slightly above the water surface in the tank experiment, resulting in a weakened water absorption characteristic.

Figure 3. Extraction result comparison of the BSI threshold and BSI gradient. By comparison, the BSI gradient enhances the difference between water and Artemia slicks, ensuring more homogenous water background information. Within the small scope of an image, the BSI gradient value of most water pixels is approximately 0, while the gradient value of Artemia pixels is greater than 0.

2.3. Satellite images
The measured spectra revealed that variations in Artemia reflectance primarily concentrated in the visible and near-infrared (VNIR) bands. The Artemia characteristic peaks almost coincided with the band settings of Landsat-8 OLI and Sentinel-2 MSI. In addition, regarding water surface floating features, such as floating algae, oil slicks, and Artemia slicks, strong backscattering could significantly enhance their radiometric signal in the VNIR bands so that they could be treated as ‘land objects’. Under such conditions, Landsat-8 and Sentinel-2 surface reflectance (SR) products could still perform well in aquatic monitoring (Chen et al. Citation2020; Kuhn et al. Citation2019; Sòria-Perpinyà et al. Citation2020).
Therefore, based on the Google Earth Engine (GEE) platform (Gorelick et al. Citation2017) (https://code.earthengine.google.com/), we collected and preprocessed Landsat-8 OLI Level-2 surface reflectance products and Sentinel-2 MSI Level-2A bottom-of-the-atmosphere products (also surface reflectance (SR)) covering Great Salt Lake and Ebinur Lake from January 2019 to December 2019 through a JavaScript (JS) procedure. lists the attributes of the above two satellites. MSI data with mixed band resolutions (10, 20, and 60 m) across 13 bands (443∼2202 nm) were resampled to a uniform 10 m by the nearest neighbor method. Subsequently, we initially screened images with cloud cover below 70% and masked land based on the Global Surface Water (GSW) dataset (Pekel et al. Citation2016). Finally, a total of 74 Landsat-8 SR images and 224 Sentinel-2 SR images of Great Salt Lake and Ebinur Lake were obtained for subsequent analysis ().
Table 1. Attributes of the Sentinel-2A MSI and Landsat-8 OLI satellite data used in this study.
Table 2. Information statistics of the satellite images used in this study.
In addition, Chinese high-resolution Earth observation satellite GF-6 panchromatic and multispectral (PMS) images (https://data.cresda.cn/#/home) were collected to verify the spatial uncertainty of Artemia slick extraction based on accurate geometric registration. The GF-6 PMS sensor comprises a 2-m panchromatic band and 4 VNIR bands with an 8-m spatial resolution (blue (485 nm), green (555 nm), red (660 nm), and NIR (830 nm)). PMS images were fused into 2 m images to ensure better visual effects. Then, through visual interpretation, we manually identified and extracted Artemia slicks, which were regarded as true samples. However, limited by the swath width, cloud coverage and data accessibility, our acquisition of PMS synchronized images matching Landsat-8 OLI and Sentinel-2 MSI was only 2 ().
3. Methods
3.1. Spectral sensitivity analysis
To simulate Artemia reflectance in discrete OLI and MSI bands, the continuous Artemia spectra acquired through the water tank experiment were convolved with the relative spectral response (RSR(λ)) in specific sensor channels (EquationEq. (1(1)
(1) )). The SWIR-2 bands of both sensors with a wavelength of ∼2200 nm were not considered in this study, as the Artemia in situ spectra only reached 1700 nm. The cirrus band (∼1300 nm) and water vapor band (∼945 nm) were also excluded, as they seldom contain Earth surface signals and are usually employed for atmospheric correction (Richter et al. Citation2011; Xu, Jia, and Pickering Citation2014).
(1)
(1) where Rband represents the simulated band-specific reflectance, and Rhyp signifies the Artemia in situ hyperspectral reflectance.
Then, an index of sensitivity (EquationEq. (2(2)
(2) )) can be defined as the ratio of the variation in band-specific reflectance at different Artemia densities to the noise-equivalent reflectance.
(2)
(2) where Rcon=i is the band-specific reflectance at an Artemia density of i g/L (0 ≤ i ≤ 18), and Rcon=0 is the reflectance of water, which is defined as the reference density. Rnoise is the noise-equivalent reflectance, which can be calculated by Equation (3) and needs adjustment based on the reference density (Cao et al. Citation2018; Li, Chen, Tian et al. Citation2015).
(3)
(3) where F0 is the extraterrestrial solar irradiance, θ (set to 45°) is the solar zenith angle, and d is the Earth-Sun distance in astronomical units. Additionally, Lnoise, as defined in Equation (4), is the noise-equivalent radiance determined by the sensor-specific input radiance (Ltypical) and SNR (Qi et al. Citation2017).
(4)
(4) The SNR and relevant Ltypical values of each band for OLI and MSI can be obtained from the United States Geological Survey (USGS) and European Space Agency (ESA) websites ().
Following Equations (2)-(4), the sensitivity degree (Ratio) of each sensor band to Artemia density variation can be estimated. The higher the Ratio value is, the higher the sensitivity. Furthermore, with increasing density, whether the Ratio value monotonically increases is critical to determine whether a given band can be used for Artemia density retrieval.
3.2. Artemia slick extraction algorithm
To analyze and compare the spatial uncertainty of different satellites and form a time series, an accurate and reliable Artemia extraction algorithm is needed. Artemia pixels exhibit a much higher reflectance than those of the surrounding water pixels in the NIR bands. According to this distinct feature, the BSI was developed and was defined as the difference between the NIR reflectance and the baseline constructed by the green and shortwave infrared (SWIR) reflectance (Tian et al. Citation2023). Specifically, the BSI can be expressed as:
(5)
(5) where R is the reflectance (unitless) in specific bands (indicated by the subscripts green, NIR, and SWIR). Regarding Landsat-8 OLI, the wavelengths are λgreen = 560 nm, λNIR = 865 nm, and λSWIR = 1610 nm. Regarding Sentinel-2 MSI, the wavelengths are λgreen = 560 nm and λSWIR = 1610 nm, given that the MSI sensor offers multiple red edge bands and that the ranges of the red edge 3 and 4 and NIR bands are very close and partly overlapping, more alternatives are available within the NIR wavelength ranges. The impact of NIR band selection on the BSI results was discussed in Section 5.1.
However, influenced by complex water backgrounds, atmospheric conditions, bottom materials under shallow water, and other factors, it is difficult to use a fixed threshold in Artemia slick extraction with high accuracy, even in the same lake. Hence, it is necessary to optimize the algorithm by combining the edge detector and introducing the BSI gradient through convolution (Tian et al. Citation2023). Finding suitable thresholds becomes easier since most BSI gradient values of Artemia-containing pixels are greater than 0 (). The extracted area is defined as the product of pixel number and pixel size. Next, we can quantify spatial uncertainty based on the ratio of the extracted area to the reference area.
3.3. Spatial detection limit analysis
Detecting and quantifying small Artemia features in satellite images are very important steps for estimating Artemia areal coverage and tracing its origins. Obviously, the higher the spatial resolution is, the more accurate the detection of Artemia features in visual interpretation. However, obtaining the average or minimum size of Artemia slicks requires long-term observation. Determining the optimal sensor spatial resolution for Artemia slick detection is also challenging due to the lack of reliable fishery statistics. Through mixed pixel decomposition and spectral differencing techniques (Equations (6)- (8)), the lowest detectable Artemia percentage in pixels (χmin) can be defined, thereby obtaining the sensor spatial detection limit (Hu et al. Citation2015). Assuming that the percentages of Artemia and water in mixed pixels are χ and (1- χ), respectively, the reflectance of mixed pixels can be obtained as:
(6)
(6) where RA is the reflectance of the Artemia endmember and RW is the reflectance of the Artemia-free water endmember. For simplicity, their dependence on the wavelength is omitted here.
In Artemia slick detection, the reflectance of Artemia-containing pixels must be significantly different from that of nearby Artemia-free water pixels. Qi and Hu (Citation2021) proposed that their difference (i.e. spatial contrast (ΔR)) must be at least twice the noise level (statistical 2σ) to achieve a 95% confidence level, which can be expressed as follows:
(7)
(7) where σ is the sensor noise Rnoise and √2 is the noise propagation between the target and nearby reference pixels (Hu Citation2021). The subpixel detection limit (χmin) of the sensor can be derived from the above, as follows:
(8)
(8)
Given that baseline algorithms like the maximum chlorophyll index (MCI) (Gower et al. Citation2005), FAI, and BSI, which effectively highlighting the contrast between the target and water and are highly robust (Zhao, Liu, and Wei Citation2020), have been more widely applied than single-band algorithms, we proposed the BSI for Artemia slick extraction. Similarly, for the BSI, the detection limit (χdet) can be obtained as:
(9)
(9)
(10)
(10)
(11)
(11)
Then, the lower spatial detection limit of the sensor can be converted into the product of χdet and the pixel size (Qi and Hu Citation2021).
4. Results
4.1. Spectral simulation and sensitivity comparison
By convolving the measured continuous Artemia spectra with the sensor-specific RSR, simulated OLI and MSI Artemia reflectance data were obtained ((a), (b)). Referring to in situ spectra, the two sensors did not show the characteristic trough of Artemia at ∼675 nm, while OLI also did not exhibit the characteristic peak at ∼710 nm (high density of ∼725 nm) and trough at ∼755 nm. In contrast, MSI exhibited more spectral details in the NIR bands. Since the narrow peak at ∼710 nm generated a bathochromic shift with increasing Artemia density (), at high densities (> 56 g/L), MSI did not actually detect the reflectance peak at ∼725 nm, resulting in the trough at ∼755 nm seemingly disappearing, as shown in (b).
Figure 4. Spectral sensitivity analysis based on simulated Landsat-8 OLI and Sentinel-2 MSI spectra with Artemia density variation, where C, B, G, and R respectively refer to the coastal aerosol, blue, green, and red bands, while RE-1 (or −2, −3, −4) denote the red edge bands. (a) simulated OLI spectra (b) simulated MSI spectra (c) OLI sensitivity index (Ratio) analysis (d) MSI sensitivity index (Ratio) analysis

The bands showing the highest spectral contrast between floating objects and water are predominantly the NIR bands (Biermann et al. Citation2020). (c) and (d) show an analogous conclusion. The red to NIR bands exhibited a high sensitivity to Artemia for both OLI and MSI. Specifically, the density of 32 g/L represented a turning point. The red (655 nm) band of OLI was most sensitive for medium- to low-density Artemia slick detection (≤ 32 g/L). The Ratio value was 310 and tended to remain stable at 20 g/L. The most sensitive band at high densities (> 32 g/L) was the NIR band (865 nm). The Ratio value rapidly increased with increasing Artemia density and was 758 at 120 g/L. The most sensitive band of MSI for medium- to low-density detection was red edge 1 (705 nm). The Ratio value monotonically increased with the Artemia density variation, and the growth rate did not stabilize until 20 g/L. At high densities, the most sensitive band was still the NIR band (842 nm) and exhibited a similar change pattern to that of OLI. The similarity in the curve patterns of MSI NIR and OLI NIR, despite them not being equivalent bands, may be attributed to the ‘plateau’ at near-infrared wavelengths (750-880 nm) observed in high-density Artemia spectra. This could also explain why multiple MSI red edge bands within this range exhibited similar curve shapes. Interestingly, the MSI red edge 1 band, which is closest to the red band, exhibited a trend between those of the red and NIR curves but maintained sensitivity to low-density Artemia. Obviously, the lack of red-edge band settings for OLI made it difficult to accurately obtain optimal Artemia detection wavelength just like MSI. However, due to sensor design differences, the Ratio value of MSI red edge 4 (equivalent to OLI NIR (865 nm)) was only 175 at an Artemia density of 120 g/L, accounting for approximately 0.23 of that of OLI. Furthermore, the most sensitive MSI NIR band (842 nm) had a Ratio value of 313, approximately 0.41 times of that of OLI. It was reasonable to conclude that OLI provided better radiometric performance than MSI in Artemia slick detection. According to theoretical research, the SNR is proportional to the square root of the pixel area (Li, Ren, Wan et al. Citation2015; Schott Citation2007; Zhang et al. Citation2023). Therefore, spatial aggregation could improve the SNR and reduce noise levels within pixels (Equations (2)-(4)) while reducing image resolution. We can thus infer that the spectral sensitivity of 30-40 m aggregated MSI VNIR bands could potentially reach or exceed that of OLI (Ouma, Noor, and Herbert Citation2020).
In addition, the Ratio value did not change monotonically with density in the blue and green bands, instead showing low peaks at 24 and 56 g/L. At a density of 56 g/L, the Ratio values in the NIR and green bands were 732 and 82 for OLI, and 309 and 18 for MSI, respectively. In other words, the sensitivity of the NIR band was approximately 9 times or even higher than that of the blue and green bands at high densities. We generally do not apply the blue and green bands alone in Artemia abundance retrieval.
In summary, the sensitive OLI and MSI bands for Artemia slick detection were not unique at the various densities. In addition to the conventional settings of visible spectra, we expect sensors for Artemia slick detection to provide more bands in the near-infrared wavelength range, which should include at least the 820–850 nm band (or the 700–725 nm and 820–850 nm bands). The SNR and bandwidth of the bands could be set based on OLI guidelines.
4.2. Spatial inspection and interpretation
4.2.1. Spatial uncertainty of Artemia slick extraction areas
Different spatial resolutions may cause a scale effect and is one of the major limiting factors of Artemia slick detection and characterization (Wu and Li Citation2009). We regarded the manual interpretation results from GF-6 PMS fusion images (2 m) as true values and analyzed the spatial uncertainty of the Artemia slick area based on OLI and MSI images for 12 July and 18 August 2019, respectively ((a) and (b)). These images were subjected to strict geometric correction, ensuring registration accuracy within 2 pixels (∼15–25 m). Artemia slicks are light and susceptible to displacement caused by water flows and strong winds. Since the slick shape generally remains unchanged over short periods of drifting, the corresponding offset in images can usually be ignored. In Great Salt Lake ((a)), the Artemia slick area estimated from the PMS images was 3.297 km2. In comparison, the slick areas estimated from the OLI and MSI images were 4.523 and 3.172 km2, respectively, and the ratios to the true value were 137% and 96%, respectively. Overall, OLI overestimated the Artemia slick area by 37%, while MSI underestimated it by 4%. Local samplings of Artemia slicks were shown in regions d and e. OLI still showed overestimations of 48% and 20%, while MSI indicated overestimations of 12% and 14%. In Ebinur Lake ((b)), the Artemia slick area estimated by the PMS images was 1.350 km2, while those estimated by the OLI and MSI images were 2.440 and 1.552 km2, respectively, showing overestimation degrees of 81% and 15%, respectively. The partial areas in regions f and g showed a similar tendency. Notably, OLI produced overestimations of 83% and 78%, while MSI only yielded overestimations of 13% and 16%.
Figure 5. GF-6 PMS, Landsat-8 OLI, and Sentinel-2 MSI Artemia slick extraction results and corresponding uncertainty statistics for different regions. (a) Extraction results and local magnification for Great Salt Lake on 12 July 2019. (b) Extraction results and local magnification for Ebinur Lake on 18 August 2019. (c) Area, uncertainty and slick width statistics of the different regions. Among them, regions a and b represent the complete ranges of Great Salt Lake and Ebinur Lake, respectively, and regions d–g are marked in the figure.

The above two examples were the only synchronized cases within the weather restrictions. Based on the limited statistical data, the Sentinel-2 MSI Artemia slick extraction results were smooth and detailed with an approximately acceptable ±16% estimated spatial uncertainty, indicating a favorable detection ability. Landsat-8 OLI significantly overestimated Artemia slicks with an uncertainty of ± 83% due to the coarser spatial resolution. In comparison, MSI is more instrumental in the detection of finer Artemia slicks. It is anticipated that for high-precision Artemia slick detection, the sensor spatial resolution should reach at least 10 m or higher.
4.2.2. Sensor spatial detection limit
The calculated detection limits for the OLI and MSI bands using the equations detailed in Section 3.3 were listed in . Then, the detection limit for the BSI images was calculated. Accordingly, the detection limits of the two sensors were 0.77% and 1.69%, respectively. These results suggest that for clear water under ideal atmospheric conditions, if an Artemia-containing OLI pixel exhibits χ > 0.77%, the detection probability is likely > 95%. For MSI, the same applies. In other words, if the proportion of MSI pixels is χ > 1.69%, the likelihood of false positive detection is very low (< 5%). Although OLI is more sensitive to Artemia features, considering the spatial resolution, surface Artemia slicks larger than 6.93 m2 (i.e. 0.77% × 30 m × 30 m) can be observed. Similarly, Artemia slicks with an area > 1.69 m2 (i.e. 1.69% × 10 m × 10 m) are detectable by MSI.
Table 3. Detection limits and relevant calculation parameters derived from the OLI or MSI spectral bands. Please refer to Section 3.1 for Rnoise calculation details.
4.3. Temporal enhancements using multisatellite observations
The combination of MSI and OLI enables nearly weekly observations of Artemia slicks, and occasionally allows for continuous daily series over the short term. For example, (a) to 6(c) showed the extracted Artemia slicks in Great Salt Lake from 7 to 9 November using MSI and OLI, which was a short-term series over three consecutive days. Since the image on 9 November lacked the northwestern part of the Great Salt Lake, we masked images from 7 and 8 November to maintain the same geographical range. To ensure consistency in the time series, we also converted the extracted areas from OLI images on 8 November into MSI-extracted areas according to equations y1 and y2 in (f). The consistency analysis and area conversion were detailed in Section 5.4. Apparently, Artemia slicks exhibited extremely high dynamic variations in their position, shape and areal coverage within a day. A single satellite can hardly meet these high-frequency detection requirements. Multisatellite joint observations to achieve a higher observation frequency may become a developing trend of Artemia slick monitoring. Specifically, when selecting cloud-free, sun-glint-free, and shadow-free images with complete lake coverage and clear Artemia slicks as effective observation data, OLI yielded 1.71 observations per month on average, while MSI yielded 4.42 monthly average observations. OLI and MSI could fill any no-image gaps between each other, thus significantly enhancing the Artemia slick observation capacity to an average frequency of 6.58 times per month.
Figure 6. (a) MSI extraction results for Great Salt Lake on 7 November 2019, with an area of 11.72 km2. (b) OLI extraction results for Great Salt Lake on 8 November 2019, with an area of 13.03 km2. (c) MSI extraction results for Great Salt Lake on 9 November 2019, with an area of 16.32 km2. (d) Partial Artemia slick extraction results for Great Salt Lake on 17 November 2019. (e) Partial Artemia slick extraction results for Ebinur Lake on 30 May 2019. (f) Consistency analysis of the Artemia slick areas extracted from 9 simultaneous OLI and MSI images.

Moreover, synchronous images may help to track Artemia slick movement. (d) showed the OLI and MSI extracted Artemia slicks in the Great Salt Lake on 17 November 2019, where the OLI results were marked in red, while the MSI results were marked in blue. At an interval of 18 min, the spatial distribution and shapes of some Artemia features were maintained, supporting their southeast movement direction. The displacement distance was approximately 210–380 m at a geometric positioning accuracy of 25 m. In (e), Artemia slicks were detected in both OLI and MSI images of Ebinur Lake on 30 May 2019, 12 min apart with a northwest drift distance of approximately 120–260 m. Therefore, for accurate monitoring, we propose realizing repeated daily observations via multisatellite joint efforts.
5. Discussion
5.1. Usage comparison of red-edge and near-infrared wavelengths in BSI calculations
The near-infrared wavelength is most sensitive to spectral changes in Artemia slicks. With sensors like MSI that have multiple bands in the near-infrared wavelength range, it is possible to obtain various BSI combinations. According to BSI design principles (Tian et al. Citation2023) and Artemia spectral features (), the red-edge wavelength was also considered here. The NIR and red edge 1–4 bands of MSI can be substituted as λNIR into Equation (5) to calculate BSI, and the extracted Artemia slick areas can be successively compared. For variable control, the MSI NIR (842 nm) band was downsampled to a 20 m spatial resolution. Within the same display range, the BSI value based on red edge 1 (705 nm) showed a certain advantage in low-density and scattered Artemia slick detection, with a raised threshold (). However, this high sensitivity might inevitably result in overextraction, requiring more rigorous threshold selection (generally by raising thresholds) or the exploration of correction (such as linear or nonlinear) methods when used. The BSI value based on red edge 2 (740 nm) was relatively low and could not fully extract all Artemia slicks. The extraction results based on red edge 3 (783 nm) were similar to those based on NIR (842 nm), both of which can be used to comprehensively detect Artemia slicks. Moreover, (b) showed obvious gradient stratification for red, yellow, and green, indicating that the MSI NIR (842 nm) band was sensitive to the density variation (especially at moderate and high densities), which was beneficial for Artemia biomass inversion. The extracted BSI area based on red edge 4 (865 nm) was the smallest. The water background in (f) showed obvious blue-purple separation, suggesting that this calculation method might be influenced by an inhomogeneous water background or image noise.
Figure 7. Partial MSI image of the Great Salt Lake and performance of 5 BSI calculation methods on 9 November 2019. (a) Partial MSI image (R, G, B = 4, 3, 2) of Great Salt Lake on 9 November 2019. (b) The BSI extraction result using the MSI NIR (842 nm) band, which was downsampled to a spatial resolution of 20 m. (c) The BSI extraction result using the MSI red edge 1 (705 nm) band. (d) The BSI extraction result using the MSI red edge 2 (740 nm) band. (e) The BSI extraction result using the MSI red edge 3 (783 nm) band. (f) The BSI extraction result using the MSI red edge 4 (865 nm) band.

Table 4. Artemia slick areas and thresholds based on the 5 BSI calculation methods with different λNIR values and their deviation from the reference area. Note that the reference area was obtained via manual interpretation.
The above BSI calculation results were generally consistent with those of the sensitivity analysis in Section 4.1. In addition, since the deviations () between the extracted areas of three BSI calculation methods using NIR, red edge 1, or red edge 3 were small (<±1%), it did not matter which band was used. For the MSI sensor, its NIR band has the highest spatial resolution (10 m), and it clearly achieves the best results when the corresponding data are applied in BSI calculations. For other sensors with the same band settings and a consistent spatial resolution in the future, we prefer wavelengths suitable for Artemia biomass inversion to support Artemia yield estimation, provide fishing guidance, and enhance resource-sustainable utilization. Therefore, a wavelength of ∼840 nm yields the best BSI calculation results.
5.2. Slick width and sensor detection capacity
With the example cases in Section 4.2.1, the spatial uncertainty of Sentinel-2 MSI and Landsat-8 OLI images was analyzed based on the extracted Artemia slick areas from the GF-6 PMS fusion images. As a commonly used sensor for detecting floating objects, Landsat provides high application value, offering a higher SNR value and a longer time series compared to Sentinel. Landsat data plays a vital role in the future production of Artemia datasets and the exploration of the growth-disappearance pattern of Artemia slicks. Although the uncertainty of OLI was ± 83%, we could not simply conclude that OLI was unsuitable for Artemia slick detection. Moreover, the Artemia slick width could affect the detection accuracy, especially when the width is less than the sensor spatial resolution. In (c), the spatial uncertainty of MSI for Artemia slick detection remained relatively stable (< 16%) with slick width variation. However, the spatial uncertainty of OLI (%) demonstrated a significant inverse relationship with slick width (m), as indicated by the formula: Uncertainty = −2.457· Width + 116.83 (R2 = 0.936). This may occur because the slick width was generally close to or greater than the spatial resolution of MSI but mostly less than that of OLI. Therefore, we could roughly infer that for a slick width > 30 m, the spatial uncertainty of OLI in Artemia slick detection should be < 43%. The OLI spatial uncertainty could be reduced to less than 15% for a slick width > 42 m. This indicates that if the statistical data regarding the width of Artemia slicks are comprehensive for a particular salt lake, it will be conducive to the sensor selection with an appropriate spatial resolution (preferably less than average slick width), to best mitigate spatial uncertainty.
Due to the lack of reliable Artemia slick in situ statistical data, we collected the width of Artemia slicks in 2019 from 104 cloud-free Sentinel-2 MSI images, including 47 Ebinur Lake images and 57 Great Salt Lake images. Based on statistics, nearly 68% of the Artemia slicks in Ebinur Lake exhibited a width of up to 20 m, with 22% exhibiting a width greater than 30 m. More than half of the slicks in Great Salt Lake had a width > 35 m, of which 21% had a width > 45 m. OLI may perform better than illustrated in the example for most cases, particularly in the Great Salt Lake.
5.3. Influencing factors of the detection limit
The analysis of the spatial detection limit in this study was based solely on ideal situations, assuming that the spectral shapes of both Artemia and water endmembers remain stable, and image noise originated solely from sensor noise under typical conditions (clear-sky and clear-water). However, the detection limit χmin may be further improved in practical applications. For example, Tian et al. (Citation2023) indicated that Chl-a-dominated or SPM-dominated water may enhance the detection limit, even reaching 1.5-3.8 times that under clear-water conditions. The category assignment of pixels with values higher than χmin must also be carefully considered. A single pixel exceeding the detection limit may be an outlier and is often difficult to interpret. Instead, spatial continuity of several pixels is needed, potentially leading to higher (2-3 times) detection limits (Hu Citation2021). On the other hand, Artemia slicks, especially at low densities, may be submerged slightly below the water surface (∼1-5 cm) and cause a decrease in the NIR reflectance, leading to overestimations of the detection limit (Hu et al. Citation2015). Considering all the above potential factors, we conclude that Artemia slicks can be detected based on the BSI when Artemia features constitute more than 2-5% of subpixels.
5.4. Consistency analysis and correction
When combining multiple sensors to improve the effective observation frequency for Artemia slick monitoring, advanced sensor consistency correction is necessary. Taking 9 synchronous MSI and OLI image pairs as examples, they were sequentially cropped into the same coverage area to compare the two sensors’ consistency by simply calculating the number of Artemia-containing pixels. The extracted Artemia slick area was estimated as the direct product of the Artemia-containing pixel number and sensor pixel size. This was a nonweighted calculation. The area calculations mentioned in Sections 4.2 and 4.3 were based on this method. Using the extracted OLI slick area (x) as the independent variable and the MSI area (y) as the dependent variable, we plotted the coordinate axis on a logarithmic scale to stretch the image pair distribution, imitating the work in Qi et al. (Citation2022), and generated (f). (f) showed a favorable consistency between MSI (y0) and OLI (x0), with R2 = 0.957 across all 9 image pairs. Among them, the OLI extracted area (x1) was approximately 2.1 times that of MSI (y1) given a slick area < 8.5 km2, with R2 = 0.807 for 6 image pairs. The extracted results for 3 image pairs from both sensors (x2 and y2) were close to 1:1, with R2 = 0.988 given a slick area ≥ 8.5 km2. This indicated that the Artemia slick size affects the coefficient in sensor consistency correction.
By denoting ‘x’ as AreaOLI and ‘y’ as AreaMSI, respectively, and assigning superscripts and subscripts according to different conditions, the nonlinear fitting formulas in (f) can be reformed into Equations (12)-(14). Using nonlinear regression relationships, the extracted OLI area can be transformed into the MSI area, ensuring the consistency and accuracy of joint observations.
(12)
(12)
(13)
(13)
(14)
(14)
In addition, there is a certain correlation between the Artemia biomass (density) and its slick scale. To support further biomass inversion, mixed pixel decomposition by weighting each Artemia-containing pixel (EquationEq. (7(7)
(7) )) is needed. This can not only control the effect of Artemia slick size on consistency correction but also eliminate the spatial uncertainty caused by sensor differences. In other words, different sensors can obtain approximate weighted area estimation results. Via the same record form, the nonlinear regression relationship (R2 = 0.982) between OLI (x3) and MSI (y3) can be expressed as:
(15)
(15)
6. Conclusion
In this study, Landsat-8 OLI and Sentinel-2 MSI images were adopted as examples to compare their performance in terms of spectral sensitivity, spatial resolution, and temporal dynamics in Artemia slick detection. First, the following spectral sensitivities were identified: 1) the MSI NIR band (∼842 nm), which has advantages in Artemia biomass inversion, displayed the highest sensitivity for Artemia detection, and the most sensitive band for detecting Artemia in OLI was also the NIR band (∼865 nm) owing to the lack of other comparable near-infrared bands; 2) for scattered Artemia at low densities, the MSI red edge 1 band (∼705 nm) and the OLI red band (∼655 nm) showed relatively stronger sensitivity; 3) the radiometric performance of OLI was better than that of MSI, and this difference can be offset by the spatial aggregation of pixels; and 4) the blue and green bands were not used alone for detecting or retrieving Artemia abundance. Second, using the BSI index for Artemia slick extraction, MSI attained an uncertainty of approximately ±16%, while OLI showed a higher uncertainty (±83%) based on the reference 2-m GF-6 fusion images. The detection limits of OLI and MSI for Artemia slicks were 0.77% and 1.69%, respectively, i.e. Artemia slicks with areas larger than 6.93 and 1.69 m2, respectively, could be detected and quantified. Third, OLI yielded 1.71 average effective observations per month, approximately 0.39 times that of MSI (4.42 observations monthly). The combination of OLI and MSI could enhance the monthly average observation frequency to 6.58 times. Multisatellite observations could facilitate Artemia slick tracking with highly dynamic changes in position, shape, and area within a day. According to the above analysis, we expect sensors for Artemia slick detection to provide at least 4–6 spectral bands, including visible bands (480, 560, and 650 nm) and the NIR band (820-850 nm), while the red edge band (700-725 nm) could be added for low-density scattered Artemia detection, employing the 1610 nm SWIR band for BSI calculation. The SNR and bandwidth of the bands could be determined by referencing OLI’s settings. A 10 m (or even higher) spatial resolution and 1 revisit observation per day through multisatellite joint observations could be considered for accurate monitoring.
Furthermore, more factors need to be considered in practical applications. For instance, the selection of specific bands within the red-edge and near-infrared wavelength ranges may affect the BSI results. The Artemia slick width may impact the sensor’s detection capacity, especially for sensors with spatial resolutions lower than the average slick width. Slick width is inversely proportional to the spatial uncertainty within a certain range in the above cases but remains stable otherwise. Affected by environmental and imaging factors, the sensor detection limit may be increased to approximately 2–5%. MSI and OLI achieved satisfactory consistency, especially given an area ≥ 8.5 km2. The spatial uncertainty due to sensor differences could be eliminated via mixed pixel decomposition. The spectral, spatial and temporal considerations detailed in this study could provide suggestions or inspiration for remote Artemia slick observation, thus improving global resource investigation and future biomass dynamic monitoring of Artemia slicks. In the future, we can also develop detection algorithms with higher precision by studying the spectral features of Artemia and constructing global datasets to analyze long-term biomass variations.
Acknowledgements
The authors thank the USGS, ESA and China Center for Resources Satellite Data Application (CCRSDA) for providing satellite data, and thank Dr. Jian Li from Nanjing University of Information Science and Technology for his assistance and suggestions in experimental design and paper revising.
Disclosure statement
No potential conflict of interest was reported by the author(s).
Data availability statement
The Landsat-8 OLI and Sentinel-2 MSI images are openly available in Google Earth Engine (GEE, https://code.earthengine.google.com/). The relative spectral response (RSR(λ)) and SNR with relevant Ltypical values for OLI and MSI can be acquired from official websites, respectively (National Aeronautics and Space Administration (NASA), https://landsat.gsfc.nasa.gov/satellites/landsat-8/spacecraft-instruments/operational-land-imager/; European Space Agency (ESA) https://sentinels.copernicus.eu/web/sentinel/user-guides/sentinel-2-msi/). The GF-6 PMS data, Artemia in-suit data and the results of this paper can be asked from the corresponding author upon reasonable request.
Additional information
Funding
References
- Asem, Alireza, Amin Eimanifar, Nasrullah Rastegar-Pouyani, Francisco Hontoria, Stephanie De Vos, Gilbert Van Stappen, and Shi-Chun Sun. 2020. “An Overview on the Nomenclatural and Phylogenetic Problems of Native Asian Brine Shrimps of the Genus Artemia Leach, 1819 (Crustacea, Anostraca).” ZooKeys 902: 1–15. https://doi.org/10.3897/zookeys.902.34593.
- Baxter, Bonnie K., and Jaimi K. Butler. 2020. “Climate Change and Great Salt Lake.” In Great Salt Lake Biology, 23–52. Cham: Springer. https://doi.org/10.1007/978-3-030-40352-2_2.
- Belovsky, Gary E., and W. Clay Perschon. 2019. “A Management Case Study for a New Commercial Fishery: Brine Shrimp Harvesting in Great Salt Lake, Utah, USA.” Ecological Applications 29 (3): e01864. https://doi.org/10.1002/eap.1864.
- Bengtson, David A, Philippe Léger, and Patrick Sorgeloos. 2018. “Use of Artemia as a Food Source for Aquaculture.” In Artemia Biology, edited by Robert A. Browne, Patrick Sorgeloos, and Clive N. A. Trotman, 255–286. Boca Raton, USA: CRC Press. https://doi.org/10.1201/9781351069892.
- Biermann, Lauren, Daniel Clewley, Victor Martinez-Vicente, and Konstantinos Topouzelis. 2020. “Finding Plastic Patches in Coastal Waters Using Optical Satellite Data.” Scientific Reports 10 (1): 1–10. https://doi.org/10.1038/s41598-020-62298-z.
- Brown, Phil D., Thomas Bosteels, and Brad T. Marden. 2023. “Salt Load Transfer and Changing Salinities Across a new Causeway Breach in Great Salt Lake: Implications for Adaptive Management.” Lakes & Reservoirs: Science, Policy and Management for Sustainable Use 28 (1): e12421. https://doi.org/10.1111/lre.12421.
- Cao, Zhigang, Hongtao Duan, Qingjun Song, Ming Shen, Ronghua Ma, and Dong Liu. 2018. “Evaluation of the Sensitivity of China’s Next-Generation Ocean Satellite Sensor MWI Onboard the Tiangong-2 Space lab Over Inland Waters.” International Journal of Applied Earth Observation and Geoinformation 71: 109–120. https://doi.org/10.1016/j.jag.2018.05.012.
- Charoeythornkhajhornchai, Pollawat, Tikumporn Kunjiek, Sukanya Chaipayang, and Santi Phosri. 2023. “Toxicity Assessment of Bioplastics on Brine Shrimp (Artemia Franciscana) and Cell Lines.” Emerging Contaminants 9 (4): 100253. https://doi.org/10.1016/j.emcon.2023.100253.
- Chen, Jiang, Weining Zhu, Yong Q. Tian, and Qian Yu. 2020. “Monitoring Dissolved Organic Carbon by Combining Landsat-8 and Sentinel-2 Satellites: Case Study in Saginaw River Estuary, Lake Huron.” Science of the Total Environment 718: 137374. https://doi.org/10.1016/j.scitotenv.2020.137374.
- Dey, Preyojon, Terence M. Bradley, and Alicia Boymelgreen. 2023. “The Impact of Selected Abiotic Factors on Artemia Hatching Process Through Real-Time Observation of Oxygen Changes in a Microfluidic Platform.” Scientific Reports 13 (1): 6370. https://doi.org/10.1038/s41598-023-32873-1.
- Gilchrist, Barbara M., and Jeffrey Green. 1960. “The Pigments of Artemia.” Proceedings of the Royal Society of London. Series B. Biological Sciences 152 (946): 118–136. https://doi.org/10.1098/rspb.1960.0028.
- Gorelick, Noel, Matt Hancher, Mike Dixon, Simon Ilyushchenko, David Thau, and Rebecca Moore. 2017. “Google Earth Engine: Planetary-Scale Geospatial Analysis for Everyone.” Remote Sensing of Environment 202: 18–27. https://doi.org/10.1016/j.rse.2017.06.031.
- Gower, J., S. King, G. Borstad, and L. Brown. 2005. “Detection of Intense Plankton Blooms Using the 709 nm Band of the MERIS Imaging Spectrometer.” International Journal of Remote Sensing 26 (9): 2005–2012. https://doi.org/10.1080/01431160500075857.
- Hu, Chuanmin. 2009. “A Novel Ocean Color Index to Detect Floating Algae in the Global Oceans.” Remote Sensing of Environment 113 (10): 2118–2129. https://doi.org/10.1016/j.rse.2009.05.012.
- Hu, Chuanmin. 2021. “Remote Detection of Marine Debris Using Satellite Observations in the Visible and Near Infrared Spectral Range: Challenges and Potentials.” Remote Sensing of Environment 259: 112414. https://doi.org/10.1016/j.rse.2021.112414.
- Hu, Chuanmin, Lian Feng, Robert F Hardy, and Eric J Hochberg. 2015. “Spectral and Spatial Requirements of Remote Measurements of Pelagic Sargassum Macroalgae.” Remote Sensing of Environment 167: 229–246. https://doi.org/10.1016/j.rse.2015.05.022.
- Hu, Chuanmin, Lin Qi, David C. English, Menghua Wang, Karlis Mikelsons, Brian B. Barnes, Magdalena M. Pawlik, and Dariusz Fick. 2023. “Pollen in the Baltic Sea as Viewed from Space.” Remote Sensing of Environment 284: 113337. https://doi.org/10.1016/j.rse.2022.113337.
- Hu, Chuanmin, Lin Qi, Yuyuan Xie, Shuai Zhang, and Brian B. Barnes. 2022. “Spectral Characteristics of sea Snot Reflectance Observed from Satellites: Implications for Remote Sensing of Marine Debris.” Remote Sensing of Environment 269: 112842. https://doi.org/10.1016/j.rse.2021.112842.
- Hu, Lianbo, Kan Zeng, Chuanmin Hu, and Ming-Xia He. 2019. “On the Remote Estimation of Ulva Prolifera Areal Coverage and Biomass.” Remote Sensing of Environment 223: 194–207. https://doi.org/10.1016/j.rse.2019.01.014.
- Huang, Changchun, Kun Shi, Hao Yang, Yunmei Li, A-xing Zhu, Deyong Sun, Liangjiang Xu, Jun Zou, and Xia Chen. 2015. “Satellite Observation of Hourly Dynamic Characteristics of Algae with Geostationary Ocean Color Imager (GOCI) Data in Lake Taihu.” Remote Sensing of Environment 159: 278–287. https://doi.org/10.1016/j.rse.2014.12.016.
- Islam, M. S., M. M. Kibria, and S. Bhuyan. 2019. “Production of Artemia Biomass in Indoor Culture Tank in Bangladesh.” Journal of Scientific Research 11 (1): 101–110. https://doi.org/10.3329/jsr.v11i1.36467.
- Kuhn, Catherine, Aline de Matos Valerio, Nick Ward, Luke Loken, Henrique Oliveira Sawakuchi, Milton Kampel, Jeffrey Richey, Philipp Stadler, John Crawford, and Rob Striegl. 2019. “Performance of Landsat-8 and Sentinel-2 Surface Reflectance Products for River Remote Sensing Retrievals of Chlorophyll-a and Turbidity.” Remote Sensing of Environment 224: 104–118. https://doi.org/10.1016/j.rse.2019.01.023.
- Li, Jian, Xiaoling Chen, Liqiao Tian, Jue Huang, and Lian Feng. 2015. “Improved Capabilities of the Chinese High-Resolution Remote Sensing Satellite GF-1 for Monitoring Suspended Particulate Matter (SPM) in Inland Waters: Radiometric and Spatial Considerations.” ISPRS Journal of Photogrammetry and Remote Sensing 106: 145–156. https://doi.org/10.1016/j.isprsjprs.2015.05.009.
- Li, Xiansheng, Jianwei Ren, Zhi Wan, Hongxing Liu, and Changxi Xue. 2015. “Improving Signal-to-Noise Ratio and Reducing Noise Equivalent Radiance of Electro-Optical Systems Sensor by Binning Image Pixels.” Ninth International Symposium on Precision Engineering Measurement and Instrumentation 9446: 693–697. https://doi.org/10.1117/12.2181195.
- Lindsay, M. R., C. Anderson, N. Fox, G. Scofield, J. Allen, E. Anderson, L. Bueter, S. Poudel, K. Sutherland, and J. H. Munson-McGee. 2017. “Microbialite Response to an Anthropogenic Salinity Gradient in Great Salt Lake, Utah.” Geobiology 15 (1): 131–145. https://doi.org/10.1111/gbi.12201.
- Litvinenko, Liudmila I., Aleksandr I. Litvinenko, Elena G Boiko, and Kirill Kutsanov. 2015. “Artemia Cyst Production in Russia.” Chinese Journal of Oceanology and Limnology 33 (6): 1436–1450. https://doi.org/10.1007/s00343-015-4381-6.
- Liu, Shasha, Xifang Liu, Qinxian Jia, Fanjing Kong, Mianping Zheng, and Guangjun Lv. 2014. “Assessment of Spatial Distribution and Cysts Resources of Artemia in Late Autumn in Dangxiong Co Salt Lake.” Acta Ecologica Sinica 34 (1): 26–33. https://doi.org/10.1016/j.chnaes.2013.11.004.
- Lu, Yingcheng, Jing Shi, Chuanmin Hu, Minwei Zhang, Shaojie Sun, and Yongxue Liu. 2020. “Optical Interpretation of Oil Emulsions in the Ocean–Part II: Applications to Multi-Band Coarse-Resolution Imagery.” Remote Sensing of Environment 242: 111778. https://doi.org/10.1016/j.rse.2020.111778.
- Marden, Brad, Phil Brown, and Thomas Bosteels. 2020. “Great Salt Lake Artemia: Ecosystem Functions and Services with a Global Reach.” In Great Salt Lake Biology, 175–237. Springer. https://doi.org/10.1007/978-3-030-40352-2_7.
- Ogburn, Nepheronia Jumalon, Luchun Duan, Suresh Ramraj Subashchandrabose, Patrick Sorgeloos, Wayne O'Connor, Mallavarapu Megharaj, and Ravi Naidu. 2023. “Agricultural Wastes for Brine Shrimp Artemia Production: A Review.” Reviews in Aquaculture 15: 1159–1178. https://doi.org/10.1111/raq.12784.
- Ouma, Yashon O., Kimutai Noor, and Kipkemoi Herbert. 2020. “Modelling Reservoir Chlorophyll-a, TSS, and Turbidity Using Sentinel-2A MSI and Landsat-8 OLI Satellite Sensors with Empirical Multivariate Regression.” Journal of Sensors 2020: 1–21. https://doi.org/10.1155/2020/8858408.
- Pekel, Jean-François, Andrew Cottam, Noel Gorelick, and Alan S Belward. 2016. “High-Resolution Mapping of Global Surface Water and its Long-Term Changes.” Nature 540 (7633): 418–422. https://doi.org/10.1038/nature20584.
- Qi, Lin, and Chuanmin Hu. 2021. “To What Extent Can Ulva and Sargassum be Detected and Separated in Satellite Imagery?” Harmful Algae 103: 102001. https://doi.org/10.1016/j.hal.2021.102001.
- Qi, Lin, Chuanmin Hu, Karlis Mikelsons, Menghua Wang, Veronica Lance, Shaojie Sun, Brian B Barnes, Jun Zhao, and Dimitry Van der Zande. 2020. “In Search of Floating Algae and Other Organisms in Global Oceans and Lakes.” Remote Sensing of Environment 239: 111659. https://doi.org/10.1016/j.rse.2020.111659.
- Qi, Lin, Zhongping Lee, Chuanmin Hu, and Menghua Wang. 2017. “Requirement of Minimal Signal-to-Noise Ratios of Ocean Color Sensors and Uncertainties of Ocean Color Products.” Journal of Geophysical Research: Oceans 122 (3): 2595–2611. https://doi.org/10.1002/2016JC012558.
- Qi, Lin, Sheng-Fang Tsai, Yanlong Chen, Chengfeng Le, and Chuanmin Hu. 2019. “In Search of red Noctiluca scintillans Blooms in the East China Sea.” Geophysical Research Letters 46 (11): 5997–6004. https://doi.org/10.1029/2019GL082667.
- Qi, Lin, Menghua Wang, Chuanmin Hu, and Benjamin Holt. 2022. “On the Capacity of Sentinel-1 Synthetic Aperture Radar in Detecting Floating Macroalgae and Other Floating Matters.” Remote Sensing of Environment 280: 113188. https://doi.org/10.1016/j.rse.2022.113188.
- Qi, Lin, Yao Yao, David E English, Ronghua Ma, John Luft, and Chuanmin Hu. 2021. “Remote Sensing of Brine Shrimp Cysts in Salt Lakes.” Remote Sensing of Environment 266: 112695. https://doi.org/10.1016/j.rse.2021.112695.
- Richter, Rudolf, Xingjuan Wang, Martin Bachmann, and Daniel Schläpfer. 2011. “Correction of Cirrus Effects in Sentinel-2 Type of Imagery.” International Journal of Remote Sensing 32 (10): 2931–2941. https://doi.org/10.1080/01431161.2010.520346.
- Schott, John R. 2007. Remote Sensing: The Image Chain Approach. 2nd ed. Oxford University Press. https://doi.org/10.5860/choice.45-0889.
- Seale, Alvin. 1933. “The Brine Shrimp (Artemia) as a Satisfactory Live Food for Fishes.” Transactions of the American Fisheries Society 63 (1): 129–130. https://doi.org/10.1577/1548-8659(1933)63[129:TBSAAA]2.0.CO;2.
- Shi, Jing, Junnan Jiao, Yingcheng Lu, Minwei Zhang, Zhihua Mao, and Yongxue Liu. 2018. “Determining Spectral Groups to Distinguish oil Emulsions from Sargassum Over the Gulf of Mexico Using an Airborne Imaging Spectrometer.” ISPRS Journal of Photogrammetry and Remote Sensing 146: 251–259. https://doi.org/10.1016/j.isprsjprs.2018.09.017.
- Sorgeloos, Patrick, and Rodrigo Roubach. 2021. “Past, Present and Future Scenarios for SDG-Aligned Brine Shrimp Artemia Aquaculture.” FAO Aquaculture Newsletter 63: 56–57. https://www.fao.org/3/cb4850en/cb4850en.pdf.
- Sòria-Perpinyà, Xavier, Eduardo Vicente, Patricia Urrego, Marcela Pereira-Sandoval, Antonio Ruíz-Verdú, Jesús Delegido, Juan Miguel Soria, and José Moreno. 2020. “Remote Sensing of Cyanobacterial Blooms in a Hypertrophic Lagoon (Albufera of València, Eastern Iberian Peninsula) Using Multitemporal Sentinel-2 Images.” Science of the Total Environment 698: 134305. https://doi.org/10.1016/j.scitotenv.2019.134305.
- Tian, Liqiao, Jingyi Tian, Jianru Wang, Xin Wang, and Wei Li. 2023. “A Novel Remote Sensing Index for Brine Shrimp (Artemia) Slick Detection in Salt Lakes.” Remote Sensing of Environment 286: 113428. https://doi.org/10.1016/j.rse.2022.113428.
- Topouzelis, Konstantinos, Apostolos Papakonstantinou, and Shungudzemwoyo P Garaba. 2019. “Detection of Floating Plastics from Satellite and Unmanned Aerial Systems (Plastic Litter Project 2018).” International Journal of Applied Earth Observation and Geoinformation 79: 175–183. https://doi.org/10.1016/j.jag.2019.03.011.
- Vanhaecke, Paul, Wim Tackaert, and Patrick Sorgeloos. 1987. “The Biogeography of Artemia: An Updated Review.” Artemia Research and Its Applications 1: 129–155.
- Van Stappen, Gilbert. 1996. “4.1. Introduction, Biology and Ecology of Artemia.” In Manual on the Production and use of Live Food for Aquaculture, edited by Patrick Lavens, and Patrick Sorgeloos, 361: 79–106. Rome, Italy: FAO. https://www.fao.org/3/w3732e/w3732e0m.htm.
- Van Stappen, Gilbert, Gholamreza Fayazi, and Patrick Sorgeloos. 2001. “International Study on Artemia LXIII. Field Study of the Artemia Urmiana (Günther, 1890) Population in Lake Urmiah, Iran.” Hydrobiologia 466 (1–3): 133–143. https://doi.org/10.1023/A:1014510730467.
- Van Stappen, Gilbert, Liying Sui, Van Nguyen Hoa, Montakan Tamtin, Betty Nyonje, Renato de Medeiros Rocha, Patrick Sorgeloos, and Gonzalo Gajardo. 2020. “Review on Integrated Production of the Brine Shrimp Artemia in Solar Salt Ponds.” Reviews in Aquaculture 12 (2): 1054–1071. https://doi.org/10.1111/raq.12371.
- Veeramani, T., P. Santhanam, N. Manickam, and C. Rajthilak. 2019. “Introduction to Artemia Culture.” In Basic and Applied Zooplankton Biology, 209–224. Singapore: Springer. https://doi.org/10.1007/978-981-10-7953-5_7.
- Vinh, Nguyen Phuoc, Cheng Ting Huang, Truong Khac Hieu, and Yao Jen Hsiao. 2020. “Economic Evaluation for Improving Productivity of Brine Shrimp Artemia Franciscana Culture in the Mekong Delta, Vietnam.” Aquaculture 526: 735425. https://doi.org/10.1016/j.aquaculture.2020.735425.
- Wang, Mengqiu, and Chuanmin Hu. 2021. “Satellite Remote Sensing of Pelagic Sargassum macroalgae: The Power of High Resolution and Deep Learning.” Remote Sensing of Environment 264: 112631. https://doi.org/10.1016/j.rse.2021.112631.
- Wang, Shenglei, Junsheng Li, Bing Zhang, Evangelos Spyrakos, Andrew N. Tyler, Qian Shen, Fangfang Zhang, et al. 2018. “Trophic State Assessment of Global Inland Waters Using a MODIS-Derived Forel-Ule Index.” Remote Sensing of Environment 217: 444–460. https://doi.org/10.1016/j.rse.2018.08.026.
- Wang, Xin, Jianqiang Liu, Jing Ding, Jingyi Tian, Xianghan Sun, and Liqiao Tian. 2023. “Extraction of Artemia Slicks from HY-1C CZI Images: Taking Ebinur Lake as an Example.” National Remote Sensing Bulletin 27: 104–115. https://doi.org/10.11834/jrs.20221659.
- Wu, Hua, and Zhao-Liang Li. 2009. “Scale Issues in Remote Sensing: A Review on Analysis, Processing and Modeling.” Sensors 9 (3): 1768–1793. https://doi.org/10.3390/s90301768.
- Xing, Qianguo, Lingling Wu, Liqiao Tian, Tingwei Cui, Lin Li, Fanzhou Kong, Xuelu Gao, and Mengquan Wu. 2018. “Remote Sensing of Early-Stage Green Tide in the Yellow Sea for Floating-Macroalgae Collecting Campaign.” Marine Pollution Bulletin 133: 150–156. https://doi.org/10.1016/j.marpolbul.2018.05.035.
- Xu, Meng, Xiuping Jia, and Mark Pickering. 2014. “Automatic cloud removal for Landsat 8 OLI images using cirrus band.” In 2014 IEEE Geoscience and Remote Sensing Symposium IEEE, 2511–2514. https://doi.org/10.1109/IGARSS.2014.6946983.
- Yamano, Hiroya, Asahi Sakuma, and Saki Harii. 2020. “Coral-spawn Slicks: Reflectance Spectra and Detection Using Optical Satellite Data.” Remote Sensing of Environment 251: 112058. https://doi.org/10.1016/j.rse.2020.112058.
- Yu, X. X., Yan Zhuge, G. Li, Qiang Du, D. Zhang, Haijian Tan, and S. Zhang. 2018. “A Study of the Ecological Effects of Water Supplement Conditions in Ebinur Lake.” Applied Ecology and Environmental Research 16: 7777–7790. https://doi.org/10.15666/aeer/1606_77777790.
- Zhang, Yuchao, Kun Shi, Zhen Cao, Lai Lai, Jianping Geng, Kuiting Yu, Pengfei Zhan, and Zhaomin Liu. 2022. “Effects of Satellite Temporal Resolutions on the Remote Derivation of Trends in Phytoplankton Blooms in Inland Waters.” ISPRS Journal of Photogrammetry and Remote Sensing 191: 188–202. https://doi.org/10.1016/j.isprsjprs.2022.07.017.
- Zhang, Jingwen, Lingyu Zheng, Xiaoxuan Zhou, Jian Mei, Tingliang Hu, Xinyue Ni, and Fangsheng Chen. 2023. “Signal-to-noise Analysis of Point Target Detection Using Image Pixel Binning for Space-Based Infrared Electro-Optical Systems.” Infrared Physics & Technology 133: 104757. https://doi.org/10.1016/j.infrared.2023.104757.
- Zhao, Yongquan, Desheng Liu, and Xiaofang Wei. 2020. “Monitoring Cyanobacterial Harmful Algal Blooms at High Spatiotemporal Resolution by Fusing Landsat and MODIS Imagery.” Environmental Advances 2: 100008. https://doi.org/10.1016/j.envadv.2020.100008.