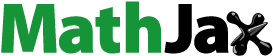
ABSTRACT
Soil erosion exacerbates land degradation, which has a detrimental impact on terrestrial ecosystems all over the world. The purpose of this study is to forecast future soil erosion in the Tumen River Basin (TRB) on the border between China and North Korea under changing land-use and climate conditions from 2030 to 2050 using the revised universal soil loss equation (RUSLE). Precipitation changes during the study period were examined under three shared socioeconomic pathway (SSP) scenarios. Future land use was simulated using a patch-generating land-use simulation (PLUS) model. As the scenario worsens, regions requiring sustained attention in the TRB are expected to rise steadily from 4% (1337 km2) in 2017 to 7.3% (2440 km2) in 2050. Under SSP5-8.5, soil erosion will reach 24.45 t ha−1yr−1 in 2050, which is 6 t ha−1yr−1 more than current soil loss. According to the land cover simulation, 2.7% (∼925 km2) of forest area is anticipated to be lost by 2050, with the majority of that area converted to built-up, cropland, and grassland areas increasing by 0.5%, 1.3%, and 0.8%, respectively. This study is expected to enhance the spatial perception of soil erosion at different scales and provide scientific basis for soil and water management.
1. Introduction
As a symptom of land degradation, soil erosion is exacerbated by the overexploitation of resources, such as mining and deforestation, which has a profound and adverse impact on global terrestrial ecosystems, directly leading to crop yield reduction, water quality deterioration, and destruction of the most basic natural resources (Yan et al. Citation2018; Mushi et al. Citation2019; Teng et al. Citation2019). Soil erosion produces enormous economic losses and is seen as a global issue (Chowdhuri et al. Citation2020), with Asia experiencing the most soil losses, amounting to 29.95 t ha−1yr−1 (Roy et al. Citation2020).
Soil loss estimation and critical area identification is particularly important for sustainable environmental management maintenance and land degradation neutrality (LDN) achievement covered by Sustainable Development Goal (SDG) 15 (Tsymbarovich et al. Citation2020). Soil erosion caused by water can lead to topsoil fertility deterioration, soil structure weakening, plant growth inhibition, and soil ability reduction in greenhouse effect mitigation (Bhattacharya, Chatterjee, and Das Citation2020). In river basins, this issue is particularly important, as it can also have far-reaching off-site impact through highly-developed river systems by increasing sediment loads and causing extreme floods (Suif et al. Citation2016; Corella et al. Citation2019). Therefore, quantifying and observing the spatiotemporal variation in soil erosion rates is essential for the sustainable development of agriculture and the environment (Rao, Shen, and Duan Citation2023).
Human-driven land-use change through agricultural practices and deforestation intensification is considered a major factor that accelerates the possibility of soil loss (Rajbanshi and Bhattacharya Citation2020). Understanding land-use activity through monitoring and forecasting is far more important than understanding the impact of rainfall intensity and topographic characteristics on soil loss (Teng et al. Citation2019; Zhang et al. Citation2022). This is because land use often negatively affects soil properties, structure, and vegetation cover (Ouyang et al. Citation2018). In the near future, irrational land-use management is likely to exacerbate soil erosion (Singh and Panda Citation2017). Cellular automata (CA) have been widely used to simulate land-use patterns based on various factors. A patch-generating land use simulation (PLUS) model (Liang et al. Citation2021) outperforms classic CA models such as the CA-Markov, CLUE S, and FLUS models in terms of capturing possible land-use change drivers and efficiency of simulation (Fang et al. Citation2022; Yang et al. Citation2022). Previously, models based on pattern analysis strategy (PAS) found it difficult to achieve land use conversion rules at particular intervals, while the PLUS model can more successfully imitate real landscapes by employing a multi-type patch generation method (Gao et al. Citation2022). While some studies explored the soil loss resulting from future land development (Getachew, Manjunatha, and Bhat Citation2021; Belay and Mengistu Citation2021; Qiao et al. Citation2023), they do not account for any aggravating variables that might exacerbate land degradation.
Climate change causes significant changes in temperature and rainfall patterns, further affecting runoff and soil erosion in river basins (Sreedevi, Eldho, and Jayasankar Citation2022). Rainfall affects soil erosion by changing its intensity, duration, and spatial pattern, whereas temperature can affect soil erosion by regulating evapotranspiration (Wu et al. Citation2018). Precipitation is highly uncertain in terms of space and time for future climate (Cook et al. Citation2020). Several climate model results from the sixth phase of the Coupled Model Intercomparison Project (CMIP6) are suitable for exploring various potential climate change pathways that are consistent with future scenarios (Su et al. Citation2021). Five Shared Socioeconomic Pathway (SSP) narratives have been presented by the Intergovernmental Panel on Climate Change (IPCC) to illustrate potential changes to global society, economy, demography, and climate in the next century (Luo et al. Citation2023). Representative Concentration Pathways (RCP)-based long-term mitigation objectives paired with SSP to create scenario matrices that can be used to assess various climate policy approaches (Doelman et al. Citation2018). Therefore, this study used three SSP-RCP scenarios for future climate observation and analysis: SSP1-1.9 (most optimistic), SSP2-4.5 (middle of the road), and SSP5-8.5 (worst-case).
The Tumen River Basin (TRB) in central Northeast Asia has received considerable international attention over the past few decades because of its great potential to become a transportation, trade, and resource development hub. The ‘Tumen River Regional Development Plan (TRADP)’ was proposed by the United Nations Development Program (UNDP) in the early 1990s. TRADP changed its name to the Greater Tumen Initiative in 2005, expanded its geographic coverage to the Greater Tumen region, and identified five priority areas (transportation, tourism, energy, investment, and the environment) for future cooperation (www.tumenprogramme.org). In addition, the current ‘One Belt One Road’ project from China underscores the region's strategic economic importance (Yang Citation2020). The TRB is a crucial habitat for many migrating waterfowl species as well as other globally threatened and endangered species including the Amur tiger and Amur leopard.
Soil erosion is expected to worsen in the next decades because of the projected increase in intense precipitation and the possible negative consequences of land-use change (Eekhout and de Vente Citation2022). Thus, in order to reduce the adverse effects of soil erosion caused by climate change and human activity, it is necessary to forecast and monitor potential soil erosion. Considering the relative stability of soil composition and topographical factors, soil erosion is mainly attributed to heavy rains, unreasonable land use, and a decline in vegetation cover (Mehri et al. Citation2018). In this context, the aims of this study are: (1) to predict land-use changes in 2030, 2040 and 2050, and identify the main drivers; (2) to examine how soil erosion is distributed both temporally and spatially under potential future climate change scenarios and land use; and (3) to assess how soil erosion may progress in China and the Democratic People's Republic of Korea in the future (DPRK). Ultimately, we used the RUSLE model to predict and track spatiotemporal changes in soil loss at different scales over the next 30 years as a result of changing land use and climate.
2. Study area and materials
2.1. Study area
The TRB is located in northeast Asia and encompasses an area of approximately 33 430 km2. China makes up over 70% of this footprint, with the DPRK making up the remaining portion (). The TRB's altitude spans from −6 to 2531 m, rising as one moves south, with an average elevation of 669 m. The average annual temperature and precipitation in the basin during the previous 30 years have been 5.3°C and 647.1 mm, respectively. According to the SoilGrids250m 2.0 dataset (Poggio et al. Citation2021), average soil organic carbon content in the area is approximately 78.8 g/kg. The Changbai Mountain is the source of the 525-km-long Tumen River, which eventually empties into the Sea of Japan (or East China Sea). Despite the region's importance as a habitat for wildlife, particularly for the Amur tiger, it has shrunk and become more fragmented in recent years as a result of inappropriate land uses like agriculture, deforestation (1900 km2 of forest lost), mining, and road construction (Yu et al. Citation2021a).
2.2. Data collection
To simulate future land-cover change, 15 potential drivers (Liang et al. Citation2021; Xu, Wang, and Rong Citation2023) collected from social and physical aspects () and land cover data (30 m × 30 m) from the past three periods were used. This study used land-cover data provided by Yu et al. (Citation2021a). The TRB land cover data for 2008 and 2017 were used for land simulation, whereas the land cover for 2014 was used for outcome evaluation. The ArcGIS 10.8 near tool was used to create a near-range raster at 30 m resolution for each vector data obtained from OpenStreetMap. Three downscaled global climate models (GCMs), EC-Earth3, GFDL-ESM4, and MRI-ESM2-0 for different SSP scenarios (SSP1-1.9, SSP2-4.5 and SSP5-8.5) obtained by National Tibetan Plateau Data Center (http://data.tpdc.ac.cn) were utilized to assess future rainfall erosivity. To ensure consistency of the raster data, we unified the resolution to 30 m × 30 m based on the resampling method. The reliability of RUSLE for soil erosion estimation was observed by comparing it with the data provided by Resource Watch (https://resourcewatch.org/) and a global soil erosion modeling platform (GloSEM) in cropland (Borrelli et al. Citation2022)
Table 1. Information on the driving factors used in this study for land use simulation.
3. Methods
The workflow of this study was mainly composed in two parts (). First, land cover projection was carried out using PLUS model based on 15 potential driving factors and two modules (LEAS and CARS). Then, future soil loss was assessed under the results of land cover simulation and different climate change scenarios.
3.1. PLUS model
The two main modules provided by the PLUS model for simulating changes in land use patches and investigating the underlying mechanisms affecting land use dynamics are the land expansion analysis strategy (LEAS) and the CA model of multi-type random patch seeds (CARS). With the help of the random forest algorithm, LEAS, a new rule mining approach, extracts and samples the growth of each form of land cover across two periods in order to assess the potential for development of various land types based on a variety of potential driving forces. The major influence mechanism and evolution of several random land-use patches are revealed by obtaining the growth probability for each land-use type and the influence weight of each driving component. LEAS not only avoids the requirement to evaluate the type of transformation, which increases exponentially with the number of categories, but it also keeps the model's capacity to analyze the mechanisms of land use change through time, resulting in enhanced interpretability. In contrast, CARS combines the technique of random seed generation and threshold reduction to dynamically simulate the automatic synthesis of patches in time and within the restrictions of development probabilities. In addition to some parameter settings, information such as neighborhood weights, transition matrix, and land demands in the target year are required to simulate future land cover. This study used the Markov chain method provided by PLUS to predict future land demand.
3.2. Soil erosion estimation
The revised universal soil loss equation (RUSLE) is a frequently used empirical model (Renard et al. Citation1997) for calculating the amount of water eroding from surfaces at different spatial scales owing to its simple structure, applicability, effectiveness, and compatibility with geographic information system (GIS) and remote sensing techniques (Fang and Sun Citation2017; Thomas, Joseph, and Thrivikramji Citation2018; Fayas et al. Citation2019; Olorunfemi et al. Citation2020). This model has been proved to offer credible soil loss information in China (Li et al. Citation2022). An approximate value for the annual average soil loss rate can be obtained using EquationEquation (1(1)
(1) ) as follows:
(1)
(1)
where A is the estimated average annual soil loss (t ha−1 yr−1), R is the rainfall erosivity factor (MJ mm ha−1 h−1 yr−1), K is the soil erodibility factor (t ha h ha−1 MJ−1 mm−1), LS is the combined slope length and steepness factor (dimensionless), C is the cover and management factor (dimensionless), and P is the conservation support practice factor (dimensionless). The RUSLE model's factors are classified as either natural or anthropogenic. R, K, and LS factors are included in the first category, whereas C and P factors are included in the second. According to the Ministry of Water Resources of China's classification standard (SL190-2007) (The Ministry of Water Resources of the People's Republic of China Citation2008), a six-level assessment of soil erosion intensity was performed: slight (<5 t ha−1 yr−1), light (5–25 t ha−1 yr−1), moderate (25–50 t ha−1 yr−1), intense (50–80 t ha−1 yr−1), very intense (80–150 t ha−1 yr−1), and severe (>150 t ha−1 yr−1).
The R factor describes the impact of climate on soil loss through the mixing of rainfall and surface runoff and expresses the capacity of rainfall to erode topsoil from unprotected surfaces. It was estimated using the following equation from monthly rainfall data (Wischmeier and Smith Citation1978):
(2)
(2) where Pi is to the total rainfall (mm) of the i-th month and P is the annual rainfall (mm). The ensemble (or overall) means of three GCMs (EC-Earth3, GFDL-ESM4, and MRI-ESM2-0) at a 1 km2 resolution was used to calculate the R factor for future climate.
The K factor, which is influenced by soil structure, texture, organic matter content, and porosity, assesses soil particle sensitivity to the segregation and transport impacts of rainfall and runoff processes. The soil information required for the K factor calculation was obtained from SoilGrids250m 2.0 (0-30cm), provided by the International Soil Reference and Information Center. The K factor was determined using the erosion/productivity impact calculator (Sharpley and Williams Citation1990):
(3)
(3) where SAN, SIL, and CLA stand for the percentages of sand, silt, and clay in the soil, respectively; C represents the percentage of organic carbon in the soil; and SN1 = 1-SAN / 100.
Slope length (L) and slope (S) make up the two subfactors of the LS factor. In general, areas with steep slopes are more susceptible to erosion than areas with gentle slopes, whereas areas with longer slopes favor erosion. The LS factor can be calculated using a digital elevation model (DEM) to assess the topography's susceptibility to erosion (Desmet and Govers Citation1996):
(4)
(4)
(5)
(5) where Ai,j-in calculated in GIS is substituted by the flow accumulation, which displays the cumulative upslope contributing area per unit cell, D refers to the size of the grid cell (m), ɑi,j represents the aspect direction of the grid cell.
The β ratio of the rill (produced by flow) to inter-rill erosion (caused by raindrops) determines the slope length exponent, m. β is the rill to inter-rill erosion ratio.
(6)
(6)
(7)
(7) where θ is the slope angle in degrees.
The S factor was calculated as a function of two types of slopes, where soil loss was more prevalent at slopes greater than 9% (McCool et al. Citation1987):
(8)
(8) where Θ is the slope steepness in radians.
The C factor denotes the proportion of soil loss under various vegetation cover types, whereas the P factor denotes the effect of land-use or tillage methods on soil erosion. The C and P variables indicate unintentional and intentional ways to prevent soil loss, respectively, by altering the flow pattern, size, or direction of surface runoff thereby lowering its volume and velocity (Kaffas et al. Citation2021). This study allocated these two values based on different types of land cover ().
Table 2. The assigned values for the C and P factors were based on different land-cover types (Wang and Dai Citation2020).
4. Results
4.1. PLUS-based projection of land-use change
Future land use was simulated based on land-cover data from 2008 to 2017 using 15 potential drivers. The test findings revealed that, when compared to the land-cover data from 2014, the PLUS model simulation's overall accuracy and kappa coefficient attained 88% and 0.65 (good agreement), respectively, which proved reliability. According to the TRB's current and projected land cover ( and ), the forest area had changed significantly, with an area reduced from 78.5% (∼26 251 km2) in 2017 to 75.8% (∼ 25 326 km2) in 2050, while built-up, cropland, and grassland areas increased by 0.5% (∼167 km2), 1.3% (∼435 km2), and 0.8% (∼271 km2), respectively, during this period. Changes in other land categories, such as bare land and water, remained relatively stable. The comparison results indicate that most areas were converted from forest to built-up, cropland, and grassland. In the near future, except for bare land and water areas, the change rate of other land-cover types will slow decrease to varying degrees. Cropland expansion on the Chinese side was concentrated in the suburbs, whereas that on the DPRK side was mainly distributed along the Tumen River.
Figure 3. Current and future spatial distributions of the six land types. The areas marked in the circles are (a) Yanji City, (b) Hunchun City, and (c) Musan County.

Table 3. Changes in land cover in six categories in the Tumen River Basin (approximately 33 430 km2) expressed as percentages (%).
The proportional changes in the six land categories in different countries from 2017 to 2050 are shown in . In both the Chinese and DPRK parts, forests were found to be the dominant class, followed by croplands and grasslands. The results show that the deforestation rates in the DPRK and China will attain 3.1% (∼318 km2) and 2.6% (∼607 km2), respectively, in 2050. Among the different land-cover types, the shift from forest to cropland was the most prominent. By continuously absorbing forest land, the cropland area could increase 1.2% (∼284 km2) in China and 1.5% (∼152 km2) in the DPRK. The increase in grassland area also contributed to forest loss, however, the increase gradually decreased over time. By 2050, the grassland area could expand from 4.5% (∼1036 km2) to 5.2% (∼1194 km2) in China and from 10.6% (∼1092 km2) to 11.7% (∼1204 km2) in the DPRK. In addition, the built-up area could increase to some extent in both China (from 312 to 439 km2) and in the DPRK (from 61 to 101 km2) during the study period.
Figure 4. Changes in the proportion and transitions between different land covers from 2017 to 2050.

The relative contributions of the various drivers to the four different land expansion probabilities (built-up, cropland, forest, and grassland) are shown in . The change in built-up area was mainly affected by the spatial patterns of nighttime light (16.5%), DEM (15.2%), and population density (11.2%). Grassland expansion was affected by DEM, distance to tertiary roads, and population density by 11.7%, 8.6%, and 8.3%, respectively. In the case of cropland, annual precipitation (11.6%), GDP (8.3%), and mean temperature (7.7%) were determined to be the top three drivers. Similarly, forest expansion was largely attributable to these factors, with 10.4% of GDP, 8.2% of average temperature, and 8.2% of annual precipitation. The first three factors accounted for 42.8% of the expansion of built-up areas. The contribution of the top five factors appears to be particularly important for other land categories, as their importance accounted for 42.6%, 42.5%, and 45.2% of agricultural land, forest, and grassland, respectively.
4.2. RUSLE model-based estimation of soil erosion
Judging from the current soil loss status in the TRB area, the area with notable loss (≥intense level) accounted for 4.6%, of which 1% was at the serious level. According to the soil erosion prevalence dataset from Resource Watch, 3% of the area (similar to the results of this study) was defined as experiencing severe soil loss. The current average soil loss in TRB, TRB–China and TRB–DPRK is 18.45, 16.27 and 23.34 t ha−1 yr−1, respectively. The soil erosion in cropland was 32.19 t/ha/yr, which is not significantly different from the 28.57 t ha−1 yr−1 provided by the GloSEM dataset. Therefore, the soil erosion assessment method used in this study is reliable.
In 2017, the R factor ranged from 406.1 to 1888.3 MJ mm ha−1 h−1 yr−1, with an average of 894.4 MJ mm ha−1 h−1 yr−1. The results of projected average R factor () under SSP1-1.9 were 905.9 (618.2 ∼ 2053.7), 784.6 (576.5 ∼ 1505.8) and 745.2 (548.5 ∼ 1291.7) MJ mm ha−1 h−1 yr−1 in 2030, 2040 and 2050, respectively. In the corresponding near future, the values could attain 764.7 (471 ∼ 1844.7), 878.8 (589.7 ∼ 1637.8) and 982.5 (699.7 ∼ 1783.8) MJ mm ha−1 h−1 yr−1 under SSP2-4.5; the values in the SSP 5-8.5 scenario were 549.6 (345.9 ∼ 1458.2), 789.4 (605.2 ∼ 1496.8) and 1005.1 (765.2 ∼ 1644.2) MJ mm ha−1 h−1 yr−1. The K and LS factors remained constant in this study: ranged from 0.197 to 0.3628 t ha h ha−1 MJ−1 mm−1 and from 0 to 79.6, respectively. The distribution of future soil losses under the different scenarios is shown in . From 2030 to 2050, except for SSP1-1.9 (20.37, 18.69 and 18.38 t ha−1 yr−1), the average rate of soil erosion has increased under both SSP2-4.5 (17.36, 21.01 and 23.71 t ha−1 yr−1) and SSP5-8.5 (12.31, 18.74 and 24.45 t ha−1 yr−1) scenarios. In the Chinese section, the value decreased from 17.5 to 15.39 t ha−1 yr−1 under SSP1.19, increased from 13.01 to 21.41 t ha−1 yr−1 under SSP2-4.5 and increased from 10.22 to 21.5 t ha−1 yr−1 under SSP5-8.5. In the same period in the DPRK, an upward trend was observed under both SSP2-4.5 (from 27.12 to 28.87 t ha−1 yr−1) and SSP5-8.5 (from 16.98 to 31.06 t ha−1 yr−1), while a slight decrease from 26.81 to 25.08 t ha−1 yr−1 was observed under SSP1.19. Compared to the slight and moderate changes in the spatial pattern under SSP1.19 and SSP2-4.5, there was a significant negative change under SSP5-8.5. The spatial development of soil loss differs between countries; the intensification of soil erosion in China is mainly distributed in the suburbs, while that on the DPRK side is mainly concentrated around the Tumen River.
Figure 6. Spatial patterns of soil erosion at different levels under the three climate change scenarios.

Table 4. Range of projected area (pixel) mean precipitation (mm) and R factor (MJ mm ha−1 h−1 year−1) in 2030, 2040 and 2050.
The changes in different soil erosion conditions under the three climate change scenarios are shown in . Under the SSP1-1.9 scenario, corresponding to the decline in the area under light level, from entire region to subregional scale, the area under slight level increased (in the TRB, China, and the DPRK side by 4.9%, 5.4%, and 3.7%, respectively), and the problem of soil loss appeared to be relieved to some extent. However, on the DPRK side, the area under very intense and severe levels could still increase from 2.2% to 2.37% and from 2.09% to 2.16%, respectively. Unlike the significant soil loss rate (≥intense level), which decreased slightly from 5.4% to 5.2% under SSP1-.1.9, this fraction increased by 2.7% (∼900 km2) under SSP 2-4.5 and increased by 4.5% (∼1,514 km2) under SSP5-8.5, attaining 7.1% (∼2,373 km2) and 7.3% (∼2,440 km2) of the TRB in 2050, respectively. In the Chinese section, from 2030 to 2050, the areas under intense and severe levels are projected to increase by 3.3% (∼763 km2) and 4.2% (∼972 km2) under SSP2-4.5 and SSP5-8.5, whereas those in the DPRK section could increase by 1.3% (∼137 km2) and 5.2% (∼542 km2), respectively.
5. Discussion
According to the projected land-cover changes, forest loss will continue, mainly because of cropland expansion. Although similar in deforestation rates between Chinese (3.1%) and DPRK (2.6%) sides from 2030 to 2050, the area involved on the DPRK side is nearly twice as large as that on the Chinese side. The continued surge in deforestation could be a sound strategy for the DPRK to secure more arable land, support daily energy needs, and increase GDP (). It is noteworthy that, in each target year, no significant differences in transfers of different land category were observed between the two countries. This is probably because they shared the same LEAS, which failed to capture differences in policy and management across countries. According to prior study (Yu et al., Citation2021a), the rate of deforestation was 1.6% between 1985 and 2000, rising to 4.1% after 2000. However, land cover simulation results () show that forest loss steadily decreases from 1.21% (2017 to 2030) to 0.68% (2040 to 2050). In addition, the built-up areas in two major cities (Yanji and Hunchun) in China and Musan County in the DPRK are expected to increase by 121%, 138%, and 146% respectively, from 2017, attaining 102.7, 74.4 and 33.2 km2 by 2050, respectively (). In the DPRK, this situation may be reasonable due to the major potential economic benefits of Musan County's large iron reserves (3–7 billion tons). In other words, the continued rise in demand for labor will drive local population growth. However, the situation would be different in China. According to the sixth and seventh national censuses, the population in Yanbian Prefecture (where Yanji and Hunchun are located) declined approximately 12.7% from 2010 to 2020. Although the population of Yanji has increased, it is mostly due to an influx from the surrounding cities. The average annual growth rate statistics showed that growth rate has declined from 6.5% in the 1990s to the current 2%. In addition, the average population per household in 2020 was 2.17, a decrease of 0.32 from 2010. In Hunchun City, the population declined by 1% over the past decade. In this context, it is difficult to expect steady population growth in both cities. Further urban expansion is probably constrained because of the nighttime lights (which in affected by population) and population density are the two main factors () that contribute to the growth of built-up area. A series of environmental protection policies and measures, such as returning farmland to wetlands (or forests) and the construction of the ‘Northeast Tiger and Leopard National Park’ will further restrict the growth of cities in the Chinese section. Therefore, future simulations are likely to overestimate the growth of built-up, agricultural, and forest areas in China.
Figure 8. Projected expansion of major urban built-up areas in the TRB region during the study period.

In this study, we found that the R-factor estimation differed in the use of the last constant, with some studies using 0.08188 (Huang et al. Citation2020; Pal et al. Citation2021; Zhang et al. Citation2021; Dou et al. Citation2022; Al-Mamari et al. Citation2023) and others using 0.8188 (Hu et al. Citation2020; Wang et al. Citation2021; Guo et al. Citation2022; Yu et al. Citation2021b; Zhu, Xiong, and Xiao Citation2021). The former constant was adopted to quantify soil erosion because of the good agreement between the final results and the global dataset. According to the test results, the much lower average value of R factor (164.09 MJ mm ha−1 h−1 yr−1) was obtained by using the latter constant. Moreover, there was a difference of 22.39 t ha−1 yr−1 (a large gap) from the average cropland soil loss provided by GloSEM. In other words, it is important to consider and determine whether a particular method or formula is appropriate for the target area to ensure accurate assessment of soil erosion (Majhi et al. Citation2022). On the other hand, we use single-year precipitation data to define the R factor and utilize three GCMs (EC-Earth3, GFDL-ESM4, and MRI-ESM2-0) to minimize potential uncertainties. Although multi-year averaging may further reduce uncertainty, our results show good agreement with different climate change scenarios from ideal to worsened. In the last 30 years, historical data have shown that the average annual precipitation in the TRB area has been 647.1 mm, with variations ranging from 551.7 mm in China to 742.5 mm in DPRK (Yu et al. Citation2019). The single-year precipitation (658.3∼745.2 mm) used in this study is within the possible range of historical data and is therefore representative to a certain extent.
indicates that areas requiring sustained attention (≥intensity) in the TRB are projected to increase gradually from 4% (∼1337 km2) in 2017 to 7.3% (∼2440 km2) in 2050, as the scenario worsens. The percentage of this section under the SSP1-1.9 scenario (most ideal) was relatively stable at approximately 5.2%. In 2050, the ratio tended to be similar under SSP2-4.5 and SSP5-8.5. However, the growth rate (≥intensity) under SSP5-8.5 was 1.7 times that under SSP2-4.5, from 2030 to 2050. In addition, the average soil loss growth rate (entire level) under SSP5-8.5 (12.14 t ha−1 yr−1) was almost twice that under SSP2-4.5 (6.35 t ha−1 yr−1) during this period. Notably, on the DPRK side, from 2030 to 2050, the increasing rate of areas identified as between intense and severe under SSP5-8.5 was 2.6% per year, almost four times greater than those under SSP2-4.5. However, in the Chinese section, the area difference between SSP2-4.5 (similar to SSP5-8.5) and SSP1-1.9 greater than the strong level is 2.1% by 2050. In other words, China should refer more to the SSP2-4.5 scenario on the issue of increasing soil erosion, whereas North Korea should try to avoid moving toward the SSP5-8.5 scenario. Furthermore, the intensification of soil erosion in the DPRK section increased in all scenarios and was mainly distributed near the main stream. Therefore, further attention should be paid to soil erosion and accompanying environmental problems in the TRB.
6. Conclusion
In this study, soil erosion in the TRB was projected for the next 30 years under the impact of climate change and land-use changes by utilizing the RUSLE model. According to the results, across the TRB region, soil loss is expected to intensify as the scenario worsens, especially with the expansion of the area between intense and severe levels. From 2030 to 2050, the average soil loss growth rate under SSP5-8.5 (12.14 t ha−1 yr−1) is nearly twice that of SSP2-4.5 (6.35 t ha−1 yr−1), with the area that suffered intensive erosion under SSP5 was 1.7 times that under SSP2-4.5. However, the most significant change on the Chinese side occurred at SSP2-4.5, rather than at SSP5-8.5. On the DPRK side, areas defined as having significant soil erosion intensification (≥ intensity) exhibited varying degrees of expansion in all the scenarios. The growth rate of this section was greatly boosted under SSP5-8.5, and was mainly distributed near the main stream. It is worth noting that sharing the same LEAS and differences in population growth (a key driver of built-up growth in this study) and environmental protection (e.g. construction of nature reserves) measures across countries may affect land-use projections and thus soil erosion assessments. However, the PLUS model's land-use simulation is helpful in understanding the effect of different driving forces on different land classes and for formulating reasonable management measures to deal with soil loss in the TRB. This study is expected to improve spatial awareness of soil erosion at various scales and provide a scientific basis for soil and water management, especially in transboundary river basins.
Author contribution
Hangnan Yu: Conceptualization, methodology, visualization, writing – original draft. Weihong Zhu: financial support, preliminary review. Ri Jin: data collection, methodology, framework recommendations.
Acknowledgments
This study was supported by the National Natural Science Foundation of China (41830643) and Natural Science Foundation of Jilin Province (YDZJ202201ZYTS478).
Disclosure statement
No potential conflict of interest was reported by the author(s).
Data availability
Data will be made available on request.
Additional information
Funding
References
- Al-Mamari, M. M., S. A. Kantoush, T. M. Al-Harrasi, A. Al-Maktoumi, K. I. Abdrabo, M. Saber, and T. Sumi. 2023. “Assessment of Sediment Yield and Deposition in a Dry Reservoir Using Field Observations, RUSLE and Remote Sensing: Wadi Assarin, Oman.” Journal of Hydrology 617: 128982. https://doi.org/10.1016/j.jhydrol.2022.128982
- Belay, T., and D. A. Mengistu. 2021. “Current and Near-Term Advances in Earth Observation for Ecological Applications.” Ecological Processes 10 (1): 1–23. https://doi.org/10.1186/s13717-020-00255-4
- Bhattacharya, R. K., N. D. Chatterjee, and K. Das. 2020. “Sub-basin Prioritization for Assessment of Soil Erosion Susceptibility in Kangsabati, a Plateau Basin: A Comparison Between MCDM and SWAT Models.” Science of The Total Environment 734: 139474. https://doi.org/10.1016/j.scitotenv.2020.139474
- Borrelli, P., C. Ballabio, J. E. Yang, D. A. Robinson, and P. Panagos. 2022. “GloSEM: High-Resolution Global Estimates of Present and Future Soil Displacement in Croplands by Water Erosion.” Scientific Data 9 (1): 406. https://doi.org/10.1038/s41597-022-01489-x
- Chowdhuri, I., S. C. Pal, A. Arabameri, A. Saha, R. Chakrabortty, T. Blaschke, B. Pradhan, and S. Band. 2020. “Implementation of Artificial Intelligence Based Ensemble Models for Gully Erosion Susceptibility Assessment.” Remote Sensing 12 (21): 3620. https://doi.org/10.3390/rs12213620.
- Cook, B. I., J. S. Mankin, K. Marvel, A. P. Williams, J. E. Smerdon, and K. J. Anchukaitis. 2020. “Twenty-First Century Drought Projections in the CMIP6 Forcing Scenarios.” Earth's Future 8 (6): e2019E–F001461.
- Corella, J. P., G. Benito, B. Wilhelm, E. Montoya, V. Rull, T. Vegas-Vilarrúbia, and B. L. Valero-Garcés. 2019. “A Millennium-Long Perspective of Flood-Related Seasonal Sediment Yield in Mediterranean Watersheds.” Global and Planetary Change 177: 127–140. https://doi.org/10.1016/j.gloplacha.2019.03.016
- Desmet, P., and G. Govers. 1996. “A GIs Procedure for Automatically Calculating the USLE LS Factor on Topographically Complex Landscape Units.” Journal of Soil and Water Conservation 51: 427–433.
- Doelman, J. C., E. Stehfest, A. Tabeau, H. van Meijl, L. Lassaletta, D. E. H. J. Gernaat, K. Hermans, et al. 2018. “Exploring SSP Land-Use Dynamics Using the IMAGE Model: Regional and Gridded Scenarios of Land-Use Change and Land-Based Climate Change Mitigation.” Global Environmental Change 48: 119–135. https://doi.org/10.1016/j.gloenvcha.2017.11.014
- Dou, X., X. Ma, C. Zhao, J. Li, Y. Yan, and J. Zhu. 2022. “Risk Assessment of Soil Erosion in Central Asia Under Global Warming.” Catena 212: 106056. https://doi.org/10.1016/j.catena.2022.106056
- Eekhout, J. P., and J. de Vente. 2022. “Global Impact of Climate Change on Soil Erosion and Potential for Adaptation Through Soil Conservation.” Earth-Science Reviews 226: 103921. https://doi.org/10.1016/j.earscirev.2022.103921
- Fang, Z., T. Ding, J. Chen, S. Xue, Q. Zhou, Y. Wang, Y. Wang, Z. Huang, and S. Yang. 2022. “Impacts of Land use/Land Cover Changes on Ecosystem Services in Ecologically Fragile Regions.” Science of The Total Environment 831: 154967. https://doi.org/10.1016/j.scitotenv.2022.154967
- Fang, H., and L. Sun. 2017. “Modelling Soil Erosion and Its Response to the Soil Conservation Measures in the Black Soil Catchment, Northeastern China.” Soil and Tillage Research 165: 23–33. https://doi.org/10.1016/j.still.2016.07.015
- Fayas, C. M., N. S. Abeysingha, K. G. S. Nirmanee, D. Samaratunga, and A. Mallawatantri. 2019. “Soil Loss Estimation Using Rusle Model to Prioritize Erosion Control in KELANI River Basin in Sri Lanka.” International Soil and Water Conservation Research 7 (2): 130–137. https://doi.org/10.1016/j.iswcr.2019.01.003
- Gao, L., F. Tao, R. Liu, Z. Wang, H. Leng, and T. Zhou. 2022. “Multi-scenario Simulation and Ecological Risk Analysis of Land Use Based on the PLUS Model: A Case Study of Nanjing.” Sustainable Cities and Society 85: 104055. https://doi.org/10.1016/j.scs.2022.104055
- Getachew, B., B. R. Manjunatha, and G. H. Bhat. 2021. “Assessing Current and Projected Soil Loss Under Changing Land use and Climate Using RUSLE with Remote Sensing and GIS in the Lake Tana Basin, Upper Blue Nile River Basin, Ethiopia.” The Egyptian Journal of Remote Sensing and Space Science 24 (3): 907–918. https://doi.org/10.1016/j.ejrs.2021.10.001
- Guo, Y., X. Du, D. Li, G. Zheng, X. Zhang, H. Chen, and J. Zheng. 2022. “How Newly Developed Shale gas Facilities Influence Soil Erosion in a Karst Region in SW China.” Science of The Total Environment 818: 151825. https://doi.org/10.1016/j.scitotenv.2021.151825
- Hu, T., J. Peng, Y. Liu, J. Wu, W. Li, and B. Zhou. 2020. “Evidence of Green Space Sparing to Ecosystem Service Improvement in Urban Regions: A Case Study of China’s Ecological Red Line Policy.” Journal of Cleaner Production 251: 119678. https://doi.org/10.1016/j.jclepro.2019.119678
- Huang, C., Z. Zhou, M. Teng, C. Wu, and P. Wang. 2020. “Effects of Climate, Land Use and Land Cover Changes on Soil Loss in the Three Gorges Reservoir Area, China.” Geography and Sustainability 1 (3): 200–208. https://doi.org/10.1016/j.geosus.2020.08.001
- Kaffas, K., V. Pisinaras, M. J. Al Sayah, S. Santopietro, and M. Righetti. 2021. “A USLE-Based Model with Modified LS-Factor Combined with Sediment Delivery Module for Alpine Basins.” Catena 207: 105655. https://doi.org/10.1016/j.catena.2021.105655.
- Li, K., L. Wang, Z. Wang, Y. Hu, Y. Zeng, H. Yan, B. Xu, et al. 2022. “Multiple Perspective Accountings of Cropland Soil Erosion in China Reveal Its Complex Connection with Socioeconomic Activities.” Agriculture, Ecosystems & Environment 337: 108083. https://doi.org/10.1016/j.agee.2022.108083
- Li, X., Y. Zhou, M. Zhao, and X. Zhao. 2020. “A Harmonized Global Nighttime Light Dataset 1992–2018.” Scientific Data 7 (1): 168. https://doi.org/10.1038/s41597-020-0510-y
- Liang, X., Q. Guan, K. C. Clarke, S. Liu, B. Wang, and Y. Yao. 2021. “Understanding the Drivers of Sustainable Land Expansion Using a Patch-Generating Land Use Simulation (PLUS) Model: A Case Study in Wuhan, China.” Computers, Environment and Urban Systems 85: 101569. https://doi.org/10.1016/j.compenvurbsys.2020.101569
- Luo, Z., X. Chen, N. Li, J. Li, W. Zhang, and T. Wang. 2023. “Spatiotemporal Foresting of Soil Erosion for SSP-RCP Scenarios Considering Local Vegetation Restoration Project: A Case Study in the Three Gorges Reservoir (TGR) Area, China.” Journal of Environmental Management 337.
- Majhi, A., P.P. Patel, R. Shaw, K. Mallick. 2022. “R, you Correct? The Curious Case of Arnoldus (1977). Response to “Comment on ‘Towards Improved USLE-Based Soil Erosion Modelling in India: A Review of Prevalent Pitfalls and Implementation of Exemplar Methods’ by Majhi et al. (2021), Earth-Science Reviews 221, 103,786” by Chen and Bezak (2022).” Earth-Science Reviews 231, 104096. https://doi.org/10.1016/j.earscirev.2022.104096
- McCool, D. K., L. C. Brown, G. R. Foster, C. K. Mutchler, and L. D. Meyer. 1987. “Revised Slope Steepness Factor for the Universal Soil Loss Equation.” Transactions of the ASAE 30: 1387–1396. https://doi.org/10.13031/2013.30576
- Mehri, A., A. Salmanmahiny, A.R.M. Tabrizi, S.H. Mirkarimi, A. Sadoddin. 2018. “Investigation of Likely Effects of Land Use Planning on Reduction of Soil Erosion Rate in River Basins: Case Study of the Gharesoo River Basin.” Catena 167: 116–129. https://doi.org/10.1016/j.catena.2018.04.026
- The Ministry of Water Resources of China. 2008. “The Chinese Standards for Classification and Gradation of Soil Erosion (SL190–2007).” 1–20. (in Chinese)
- Mushi, C. A., P. M. Ndomba, M. A. Trigg, R. M. Tshimanga, and F. Mtalo. 2019. “Assessment of Basin-Scale Soil Erosion Within the Congo River Basin: A Review.” Catena 178: 64–76. https://doi.org/10.1016/j.catena.2019.02.030
- Olorunfemi, I. E., A. A. Komolafe, J. T. Fasinmirin, A. A. Olufayo, and S. O. Akande. 2020. “A GIS-Based Assessment of the Potential Soil Erosion and Flood Hazard Zones in Ekiti State, Southwestern Nigeria Using Integrated RUSLE and HAND Models.” Catena 194: 104725. https://doi.org/10.1016/j.catena.2020.104725
- Ouyang, W., Y. Wu, Z. Hao, Q. Zhang, Q. Bu, and X. Gao. 2018. “Combined Impacts of Land Use and Soil Property Changes on Soil Erosion in a Mollisol Area Under Long-Term Agricultural Development.” Science of The Total Environment 613-614: 798–809. https://doi.org/10.1016/j.scitotenv.2017.09.173
- Pal, S. C., R. Chakrabortty, P. Roy, I. Chowdhuri, B. Das, A. Saha, and M. Shit. 2021. “Changing Climate and Land use of 21st Century Influences Soil Erosion in India.” Gondwana Research 94: 164–185. https://doi.org/10.1016/j.gr.2021.02.021
- Poggio, L., L. M. de Sousa, N. H. Batjes, G. B. M. Heuvelink, B. Kempen, E. Ribeiro, and D. Rossiter. 2021. “SoilGrids 2.0: Producing Soil Information for the Globe with Quantified Spatial Uncertainty.” Soil 7 (1): 217–240. https://doi.org/10.5194/soil-7-217-2021.
- Qiao, X., Z. Li, J. Lin, H. Wang, S. Zheng, and S. Yang. 2023. “Assessing Current and Future Soil Erosion Under Changing Land use Based on InVEST and FLUS Models in the Yihe River Basin, North China.” International Soil and Water Conservation Research.
- Rajbanshi, J., and S. Bhattacharya. 2020. “Assessment of Soil Erosion, Sediment Yield and Basin Specific Controlling Factors Using RUSLE-SDR and PLSR Approach in Konar River Basin, India.” Journal of Hydrology 587: 124935. https://doi.org/10.1016/j.jhydrol.2020.124935
- Rao, W., Z. Shen, and X. Duan. 2023. “Spatiotemporal Patterns and Drivers of Soil Erosion in Yunnan, Southwest China: RULSE Assessments for Recent 30 Years and Future Predictions Based on CMIP6” Catena 220: 106703. https://doi.org/10.1016/j.catena.2022.106703
- Renard, K. G., G. R. Foster, G. Weesies, D. McCool, and D. Yoder. 1997. Predicting Soil Erosion by Water: A Guide to Conservation Planning with the Revised Universal Soil Loss Equation (RUSLE). vol. 703. Washington, DC: United States Department of Agriculture.
- Roy, P., S. Chandra Pal, A. Arabameri, R. Chakrabortty, B. Pradhan, I. Chowdhuri, S. Lee, and D. Tien Bui. 2020. “Novel Ensemble of Multivariate Adaptive Regression Spline with Spatial Logistic Regression and Boosted Regression Tree for Gully Erosion Susceptibility.” Remote Sensing 12 (20): 3284. https://doi.org/10.3390/rs12203284
- Sharpley, A. N., and J. R. Williams. 1990. “EPIC—Erosion/Productivity Impact Calculator: Model Documentation.” US Department of Agriculture, Technical Bulletin 1768.
- Singh, G., and R. K. Panda. 2017. “Grid-cell Based Assessment of Soil Erosion Potential for Identification of Critical Erosion Prone Areas Using USLE, GIS and Remote Sensing: A Case Study in the Kapgari Watershed, India.” International Soil and Water Conservation Research 5 (3): 202–211. https://doi.org/10.1016/j.iswcr.2017.05.006
- Sreedevi, S., T. I. Eldho, and T. Jayasankar. 2022. “Physically-based Distributed Modelling of the Hydrology and Soil Erosion Under Changes in Landuse and Climate of a Humid Tropical River Basin.” Catena 217: 106427. https://doi.org/10.1016/j.catena.2022.106427
- Su, B., J. Huang, S. K. Mondal, J. Zhai, Y. Wang, S. Wen, M. Gao, et al. 2021. “Insight from CMIP6 SSP-RCP Scenarios for Future Drought Characteristics in China.” Atmospheric Research 250: 105375. https://doi.org/10.1016/j.atmosres.2020.105375
- Suif, Z., A. Fleifle, C. Yoshimura, and O. Saavedra. 2016. “Spatio-temporal Patterns of Soil Erosion and Suspended Sediment Dynamics in the Mekong River Basin.” Science of The Total Environment 568: 933–945. https://doi.org/10.1016/j.scitotenv.2015.12.134
- Teng, M., C. Huang, P. Wang, L. Zeng, Z. Zhou, W. Xiao, Z. Huang, and C. Liu. 2019. “Impacts of Forest Restoration on Soil Erosion in the Three Gorges Reservoir Area, China.” Science of The Total Environment 697: 134164. https://doi.org/10.1016/j.scitotenv.2019.134164
- Thomas, J., S. Joseph, and K. P. Thrivikramji. 2018. “Assessment of Soil Erosion in a Tropical Mountain River Basin of the Southern Western Ghats, India Using RUSLE and GIS.” Geoscience Frontiers 9 (3): 893–906. https://doi.org/10.1016/j.gsf.2017.05.011
- Tsymbarovich, P., G. Kust, M. Kumani, V. Golosov, and O. Andreeva. 2020. “Soil Erosion: An Important Indicator for the Assessment of Land Degradation Neutrality in Russia.” International Soil and Water Conservation Research 8 (4): 418–429. https://doi.org/10.1016/j.iswcr.2020.06.002
- Wang, Y., and E. Dai. 2020. “Spatial-temporal Changes in Ecosystem Services and the Trade-off Relationship in Mountain Regions: A Case Study of Hengduan Mountain Region in Southwest China.” Journal of Cleaner Production 264: 121573. https://doi.org/10.1016/j.jclepro.2020.121573
- Wang, H., L. Liu, L. Yin, J. Shen, and S. Li. 2021. “Exploring the Complex Relationships and Drivers of Ecosystem Services Across Different Geomorphological Types in the Beijing-Tianjin-Hebei Region, China (2000–2018).” Ecological Indicators 121: 107116. https://doi.org/10.1016/j.ecolind.2020.107116
- Wang, T., and F. Sun. 2022. “Global Gridded GDP Data set Consistent with the Shared Socioeconomic Pathways.” Scientific Data 9 (1): 221. https://doi.org/10.1038/s41597-022-01300-x
- Wischmeier, W.H., and D.D. Smith. 1978. “Predicting Soil Erosion Losses: A Guide to Conservation Planning.” USDA Agricultural Handbook No. 537, 58 pp
- Wu, Y., W. Ouyang, Z. Hao, C. Lin, H. Liu, and Y. Wang. 2018. “Assessment of Soil Erosion Characteristics in Response to Temperature and Precipitation in a Freeze-Thaw Watershed.” Geoderma 328: 56–65. https://doi.org/10.1016/j.geoderma.2018.05.007.
- Xu, X., S. Wang, and W. Rong. 2023. “Construction of Ecological Network in Suzhou Based on the PLUS and MSPA Models.” Ecological Indicators 154: 110740. https://doi.org/10.1016/j.ecolind.2023.110740
- Yan, R., X. Zhang, S. Yan, and H. Chen. 2018. “Estimating Soil Erosion Response to Land use/Cover Change in a Catchment of the Loess Plateau, China.” International Soil and Water Conservation Research 6 (1): 13–22. https://doi.org/10.1016/j.iswcr.2017.12.002
- Yang, X. 2020. “On the Tumen River Sub-Regional Cooperation Under “The Belt and Road": Opportunities and Future.” Northeast Asia Economic Research 4 (4): 34–45. in Chinese.
- Yang, R., H. Chen, S. Chen, and Y. Ye. 2022. “Spatiotemporal Evolution and Prediction of Land use/Land Cover Changes and Ecosystem Service Variation in the Yellow River Basin, China.” Ecological Indicators 145: 109579. https://doi.org/10.1016/j.ecolind.2022.109579
- Yu, H., W. K. Lee, L. Li, R. Jin, W. Zhu, Z. Xu, and G. Cui. 2021a. “Inferring the Potential Impact of Human Activities on Evapotranspiration in the Tumen River Basin Based on LANDSAT Imagery and Historical Statistics.” Land Degradation & Development 32 (2): 926–935. https://doi.org/10.1002/ldr.3775.
- Yu, H., L. Li, W. Zhu, D. Piao, G. Cui, M. Kim, S. W. Jeon, and W. K. Lee. 2019. “Drought Monitoring of the Wetland in the Tumen River Basin Between 1991 and 2016 Using Landsat TM/ETM+.” International Journal of Remote Sensing 40: 1445–1459. https://doi.org/10.1080/01431161.2018.1524604.
- Yu, H., W. Xie, L. Sun, and Y. Wang. 2021b. “Identifying the Regional Disparities of Ecosystem Services from a Supply-Demand Perspective.” Resources, Conservation and Recycling 169: 105557. https://doi.org/10.1016/j.resconrec.2021.105557
- Zhang, Y., X. Lu, B. Liu, D. Wu, G. Fu, Y. Zhao, and P. Sun. 2021. “Spatial Relationships Between Ecosystem Services and Socioecological Drivers Across a Large-Scale Region: A Case Study in the Yellow River Basin.” Science of The Total Environment 766: 142480. https://doi.org/10.1016/j.scitotenv.2020.142480
- Zhang, S., P. Yang, J. Xia, W. Wang, W. Cai, N. Chen, S. Hu, X. Luo, J. Li, and C. Zhan. 2022. “Land use/Land Cover Prediction and Analysis of the Middle Reaches of the Yangtze River Under Different Scenarios.” Science of The Total Environment 833: 155238. https://doi.org/10.1016/j.scitotenv.2022.155238
- Zhu, D., K. Xiong, and H. Xiao. 2021. “Multi-time Scale Variability of Rainfall Erosivity and Erosivity Density in the Karst Region of Southern China, 1960–2017.” Catena 197: 104977. https://doi.org/10.1016/j.catena.2020.104977