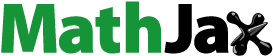
ABSTRACT
Multi-scale landscape functions play a critical role in revealing intricate functional structures within large regions. However, previous studies on landscape functions have predominantly focused on a single macro or micro scale, impeding a holistic multi-scale understanding of the spatial distribution and heterogeneity of landscape functions. To address this gap, this study proposes a framework leveraging the power of big geodata to mine multi-scale landscape functions from parcel to entire urban agglomerations, as well as non-administrative divisions. Our study focuses on the Guangdong-Hong Kong-Macao Greater Bay Area (GBA) in China. Firstly, we integrated multi-source big geodata to derive parcel-scale landscape functions. Subsequently, we employed the Normalized Revealed Comparative Advantage index to derive landscape functions at broader scales, including towns, counties and cities. The effectiveness of our approach is validated through in-field investigations and comparisons with established policy planning positions. The outcomes not only offer distinctive planning insights at various scales but also highlight the versatility of big geodata in extracting landscape functions across scales. This study demonstrates that big geodata is adept at uncovering multi-scale landscape functions irrespective of administrative boundaries, providing valuable insights for fostering multi-scale regional coordinated development.
1. Introduction
Landscape composition and configuration wield a profound influence on crucial ecological processes and functions integral to supporting human well-being (Buyantuyev, Wu, and Gries Citation2010; Cadenasso, Pickett, and Schwarz Citation2007; Sharma, Joshi, and Fürst Citation2022). Landscape function, defined as the capacity of a landscape to provide goods and services to society (De Groot Citation2006; Leemans and De Groot Citation2003; Assessment Citation2005), encompasses the provision of essential goods such as rice or timber and services ranging from residential to cultural. Understanding the spatial distribution of these functions holds immense value, given the spatial heterogeneity of landscape functions and the need for policy managers to harmonize the interests of various stakeholders (Willemen et al. Citation2008). This spatial information has become a critical parameter in most resource and environmental model systems, especially in some city growth models (Brown and Castellazzi Citation2014; Vanderhaegen and Canters Citation2017).
The spatial pattern of landscape is inherently multi-scale, mirroring the multi-scale nature of their functions (Wu and Loucks Citation1995). Mapping multi-scale spatial distribution of landscape functions is of great significance in knowing regional landscape structure, making regional planning and evaluating policies effectiveness, particularly in cities (De Groot and Hein Citation2007; Di Ludovico and D’Ascanio Citation2019; Fischer Citation2018; Ovando-Montejo, Kedron, and Frazier Citation2021). Remote sensing monitoring and pattern analysis of urban impervious surfaces reveal that city areas exhibit a multi-scale, hierarchical structure, governed by power law function (Ma et al. Citation2018; Novotný, Chakraborty, and Maity Citation2022; Ovando-Montejo, Kedron, and Frazier Citation2021). Understanding this multi-scale hierarchical structure can greatly facilitate landscape planning to enhance landscape functional capacities (Sun et al. Citation2022; Vasishth Citation2008). Spatially explicit maps showing landscape functions at multiple scales can not only illuminate the underlying principles governing urban structure and function but also serve as essential data for planning and design (Vanderhaegen and Canters Citation2017), especially as critical input and calibration parameters for models predicting results under different scenarios (Grabaum and Meyer Citation1998). Furthermore, developing cost-effective and rapid mapping strategies for multi-scale landscape functions in extensive regions can greatly facilitate tracking and evaluation of implemented policies, ensuring alignment with original intentions (Van de Voorde, Jacquet, and Canters Citation2011; Xie et al. Citation2009).
Numerous studies have identified the heterogeneity of landscape functions at different administrative scales, employing functional indicators drawn from society, economy and ecology in the form of classical statistical data. This examination spans the national scale (Angelis, Angelis-Dimakis, and Dimaki Citation2016; Egri and Tánczos Citation2015), province scale (Song et al. Citation2021), city scale (Wang et al. Citation2012); regional scale (Aumayr Citation2007; Gu et al. Citation2015; Hall Citation2006; Heffner and Gibas Citation2016), rural scale (Werner, Herrmann, and Lovett Citation2017), and beyond. While statistical data, including demographic or socioeconomic data, can support landscape function mining at aforementioned administrative scales, they availability varies across countries and often lacks the spatial details needed for analysis at micro scales. Obtaining data at more detailed levels, such as street blocks within a city, is a time-consuming process accompanied by higher field research costs. This presents a significant challenge for planners seeking to efficiently and economically undertake fine-scale landscape functionality mapping.
Over the past decade, the advent of massive big geodata, encompassing point of interest (POI), mobile phone data, social media data, high-resolution remote sensing data, and more, has ushered in new possibilities for swiftly identifying landscape functions at finer scales. Various data utilization approaches have been explored, including incorporating road network and POI data (Liu and Long Citation2016), road network and remote sensing data (Grippa et al. Citation2018; Vu et al. Citation2021), single mobile phone data (Pei et al. Citation2014), single high/very high-resolution satellite imagery (Du et al. Citation2021; Herold, Liu, and Clarke Citation2003), very high-resolution satellite imagery and POI data (Zhang, Du, and Wang Citation2017), etc. Compared to traditional statistical data, big geodata offers the advantages of low cost and high spatial resolution, facilitating the rapid mining landscape functions at finer scales. Besides, the frequent update cycles of big geodata empower planners to make timely adjustments to urban development plans amidst rapid urbanization. However, current studies leveraging big geodata predominantly focus on the method of landscape function division, often confined to a single scale within city, such as blocks or buildings (Niu et al. Citation2017). Such narrow approaches overlook the city as a holistic entity of landscape functions and hampers the exploration of the functional differences and spatial layout pattern across multiple cities. Hence, existing studies utilizing big geodata for landscape functions division still fall short in directly addressing the monitoring and analytical needs for collaborative development of landscape functions between cities at large scales, such as urban agglomerations, provinces even countries.
To bridge existing research gap, we proposed a framework for multi-scale landscape function mining, spanning from parcel to urban agglomerations, leveraging big geodata in this study. The Guangdong-Hong Kong-Macao Greater Bay Area (GBA), one of the four major bay areas in the world, is selected as the study area. The framework consists of several key steps. First, four kinds of big geodata, namely land use/cover (LULC), road network, POI and remote sensing thematic data, are integrated to obtain the parcel-scale landscape functions. The accuracy of this derivation is validated using field data collected in the GBA, employing the confusion matrix approach. Secondly, the landscape functions at town, county, city scale are explored by employing the Normalized Revealed Comparative Advantage (NRCA) index, which utilized the functional structures of different landscape functions types in administrative units. Finally, in the discussion section, the result of landscape functions mining across scale were compared with the urban functions outlined in planning documents of the GBA, providing further validation for the effectiveness of the proposed framework. In addition, outcome-based valuable landscape planning enlightenment, potential influencing factors and the applicability and limitations of the framework are thoroughly discussed. With this framework, we aspire to contribute to the growing demand for large-scale, cost-effective research on multi-scale landscape collaborative development.
2. Methods
2.1. Study area
Urban agglomerations, comprising multiple cities of varying levels and scales, serve as inherent testing grounds for multi-scale landscape function mining (Fang and Yu Citation2017). In this regard, we select the Guangdong-Hong Kong-Macao Greater Bay Area (GBA), recognized as the world's largest mega-urban agglomeration by land area (Yang et al. Citation2019), as the case study to conduct our experiment. GBA stands out with its unique administrative management and urban planning complexities, characterized by two systems, two special zones, and three tariffs (www.cnbayarea.org.cn). Additionally, the spatial distribution of the four core cities, namely Guangzhou, Shenzhen, Hong Kong and Macao, adds an extra layer of intricacy in GBA, manifesting a socioeconomic development pattern of ‘strong in the east and weak in the west’ and ‘strong in the south and weak in the north.’
Given this context of complex administrative management and imbalanced economic development in GBA, conducting cross-scale regional landscape functions mining becomes imperative. This involves systematically combing the advantage functions of various regions of the GBA, and then realizing the linkage and complementation of landscape functions have become the prerequisite for regional coordinated development of the GBA. The ‘Outline of the Development Plan for the GBA’ (www.gov.cn)introduced in 2019 offers a strategic roadmap and functional orientation for each city, providing substantial support for validating our landscape function mining results. Furthermore, as one of the three mega-urban agglomeration in China and one of the four major bay areas in the world, the GBA aspires to establish itself as a world-class bay area and urban agglomeration (Zhou Citation2023). This inherent strategic significance positions the GBA as a pivotal case study, offering insights and references for the collaborative regional planning of urban agglomerations worldwide. shows the geographical location and multi-scale administrative boundary map of the GBA.
Figure 1. Study area: the Guangdong-Hong Kong-Macao Greater Bay Area (GBA) with administrative units and the terrain information (Source: administrative boundary data comes from https://www.ngcc.cn/ngcc/; Digital elevation model (DEM) data from https://www.gscloud.cn).

2.2. Parcel-scale landscape functions and classification system
Parcel-scale landscape functions play a pivotal role in elucidating the spatial heterogeneity of social, economic and ecological functions at the parcel-scale, serving as fundamental units for comprehensive regional functional planning and the allocation of elemental resources. Within built-up areas, we adhered to the construction land types defined in the ‘Urban Land Classification and Standard for Planned Construction Land’ (GB50137-2011). In this context, we designated the street blocks divided by the road network as the analytical scale of the parcel, focusing primarily on the social and economic functions, which encompasses residential, public services, business, industry, green space and transportation. In non-built-up areas, our emphasis is on highlighting functional disparities with reference from mainstream LULC data worldwide. Here, we adopted patches as the analytical scale for parcels, emphasizing agricultural production and natural ecological functions, including waters, wetlands, forest, shrub, grass, paddy, dry land, aquaculture and unused land. shows the classification system of parcel-scale landscape functions, including specific types, abbreviations and the introduction of the functional value of each type of parcel-scale landscape functions in the region.
Table 1. Classification system of parcel-scale landscape functions.
Besides, The design of the above classification system is strategically aligned with the five major development positions for the GBA outlined by the government, aiming to discover and assess GBA’s functional development model and status with the resulting multi-scale landscape functions.
To evolve into a vibrant world-class urban agglomeration and an internationally influential hub for technological innovation, achieving multi-dimensional development in economic functions is imperative for GBA. The secondary industry, mainly comprising industries such as machinery, construction, manufacturing, and metallurgy, plays a vital role in industrial production, exemplified by cities like Dongguan and Shenzhen. We designate the spatial occupation of these industry types as industry landscape functions. Furthermore, the tertiary industry, dominated by sectors such as food and beverage services, entertainment, finance, and trade, serves as the landscape functions of business services, with cities like Guangzhou and Hong Kong as representatives. We classify the spatial occupation of these industry types as business landscape functions.
Foster the creation of a high-quality living circle that is livable, conducive to business, and enjoyable. The cornerstone of this endeavor lies in the allocation of residential and public service land to healthcare, education, and government organizations, forming the foundation for leisure and daily living. Catering to diverse moisture conditions, cultivating staple crops such as rice and vegetables on paddy and dry lands can effectively address the basic food needs of the population. Aquaculture ponds, focusing on freshwater fisheries, play a crucial role in ensuring a reliable source of fish and shrimp for higher protein intake. Furthermore, establishing a high-quality living circle requires a good ecological condition. Therefore, it is important to understand the distribution of artificial green spaces within the city, as well as the natural green areas with different ecological values outside the city, such as forests, grasslands, shrubs, and bare lands, along with the distribution and proportion of rivers, lakes, ponds, and wetlands.
Position itself into a crucial pillar supporting the Belt and Road Initiative and as a showcase zone for deep cooperation between the mainland and Hong Kong, Macao. An accessible and convenient transportation infrastructure serves as the main artery that intertwines economic development and social life. In this study, important transportation hubs such as airports, high-speed railway stations, major bus stations, ports, and terminals are considered comprehensively and classified as transportation land, in order to grasp the overall spatial pattern of transportation as a fundamental landscape function in the GBA.
2.3. Data and pre-processing
shows the data name, format, acquiring date and source. Administrative boundary is basic data to multi-scale landscape function analysis. In this study, LULC, road network, POI and remote sensing thematic data are mainly used to map the parcel-scale landscape functions and need to be introduced in detail. Among them, remote sensing thematic data needs to be acquired based on Landsat remote sensing images. The following provides a detailed introduction and pre-processing steps for each of these four data types and clear flowchart of data pre-processing ().
Table 2. Four big geodata format, acquiring date and sources.
2.3.1. LULC data
The foundational data for parcel-scale landscape function delineation in this study is derived from Land Use and Land Cover (LULC) data, sourced from Data Center for Resources and Environmental Sciences, Chinese Academy of Sciences (http://www.resdc.cn). This data is derived mainly from Landsat remote sensing image data at a spatial resolution of 30 m, obtained through visual interpretation. Notably, the data exhibits characteristics of single-type representation and homogeneity within each type of patch. The reported overall accuracy of the LULC data stands at 88.95% at the national scale. It encompasses six first-level land types and further delineates into 25 secondary-level land types.
We specifically extracted the fifth type within the first-level type, namely urban and rural industrial and mining residential land, to facilitate the subsequent road network segmentation and the POI data integration. According to the classification of parcel-scale landscape functions, the remaining land types were merged for further analysis, including paddy, dry land, grass (high, middle and low coverage grassland), forest (open forest, other forests), shrub, water (river, lake, sea, etc.), unused land (sandy land, Gobi, bare land, bare rock stony land) and wetlands (beach, marshland, etc.).
2.3.2. Road network data
The street block, serving as the basic unit of urban structure and the smallest granularity in population census, aligns with the real world boundaries. Its crucial role in depicting landscape functions within a city and supporting comprehensive planning is underscored in literature (Gong et al. Citation2020; Hermosilla et al. Citation2014; Yao et al. Citation2017). Therefore, in this study, we adopt the street block division, derived from the road network in urban and rural residential land, as the basic unit for capturing socioeconomic landscape functions.
The road network data was downloaded from OpenStreetMap (OSM) website (https://www.openstreetmap.org) for the year 2020 with 27 road types. Firstly, we extracted five types, namely expressway, main road, first-grade road, second-grade road and third-grade road. Secondly, we modified the topology of the road network using the tools of extending or trimming a polyline in ArcGIS. Third, we created buffers of varying widths based on road network to represent their actual coverage. The expressway buffers were set to 40 m, the main road buffers to 20 m, and the buffers for first-, second- and third-grade roads to 10 m. Finally, the final street block units were generated with an inverse intersection between the road network buffer zone and and the base map of the city.
2.3.3. POI data
The POI data utilized in this study is obtained from the ‘Amap’ open platform for the year 2020. It consists of 23 main types and lots of sub types, including shopping services, business residences, medical care, etc. The spatial distribution of POI was considered as an important reference for landscape functions mining within city (Yan et al. Citation2022). To ensure data quality, we removed POIs of low importance, such as telephone booths, ATMs, and bus stations. The number of remaining POIs after cleaning totaled 3,355,726. We combined the POI types with similar functions according to the classification system of parcel-scale landscape functions ().
Table 3. Merging rule of POI sub type.
We assigned a weight to each type of POI according to their importance since POIs vary in their number and importance by their types, and doing so can reduce the impact of quantity deviation on street block landscape functions mining. The Analytic Hierarchy Process (AHP) (Al-Harbi Citation2001) can construct a judgment matrix through pairwise comparison and quantitatively assign weight to each indicator based on their relative importance. In this study, we referred to the area proportion of planned urban construction land in ‘Urban Land Classification and Standard for Planned Construction Land’ (GB50137-2011) as a measure of importance for different functional types. The overall POI type served as the goal layer, with six socioeconomic POI types comprising the criterion layer. The final assigned weights are as follows: RES (0.54), ADM (0.07), BUS (0.03), IND (0.29), TRA (0.15), and GRE (0.10). The Consistency Ratio (CR = 0.04 < 0.1) passed the consistency test.
2.3.4. Remote sensing thematic data
Remote sensing enables the extraction of land use types that are not included in official LULC maps, which can provide and correct location-specific LULC information (Wang, Yang, and Zhou Citation2021). For example, aquaculture is a very important component of landscape function in the GBA, but it is not identified in the first type of LULC data mentioned above. Except for Hong Kong and Macao, the remaining nine cities in the GBA are located in Guangdong province, which is the largest province of aquaculture development in China (Ren et al. Citation2019).
Accordingly, aquaculture ponds, as a remote sensing thematic data, were extracted from intensive time-series Landsat remote sensing images in 2020 from the Google Earth Engine (GEE) platform using the method proposed by Wang et al. ( Citation2022b) and manual post-processing. The resulting 2020 aquaculture ponds data in GBA has a 30 m spatial resolution and 96.40% overall accuracy.
2.4. Operation process of multi-scale landscape functions mining
illustrates the flowchart of the study framework, which consists of three parts. Firstly (a), parcel-scale landscape functions of the GBA are divided by integrating POI, road network, land use and land cover (LULC) and remote sensing thematic data with frequency density and overlay. An accuracy assessment is conducted to verify the high consistency between the parcel-scale landscape functions and the actual land function observed in the field. Secondly (b), employing the Normalized Revealed Comparative Advantage (NRCA) index based on the area of different parcel-scale landscape functions to mine the primary and secondary landscape functions of region. Third (c), calculating the NRCA at administrative units division including town, county, city and urban agglomeration scales to realize landscape functions mining at multiple scales. Following the key methods and formula involved were shown succinctly in this flowchart.
Figure 3. Flowchart of multi-scale landscape functions mining. a. b. and c. are the first, second and third steps respectively. The full names of the abbreviations in the figure are as follows (LULC: Land Use and Land Cover; POI: Point of Interest; Urban-A: Urban agglomerations; RES: Residential; BUS: Business; IND: Industry; FOR: Forest).

2.4.1. Parcel-scale landscape functions mining by frequency density
We performed kernel density analysis (Rosenblatt Citation1956), which is a common method to explore the spatial structure of POI. It can effectively address the non-recognition of landscape functions caused by the absence of POI within the street block by considering the continuity of spatial distribution. A bandwidth of kernel density between 200 and 300 is suitable for landscape functions mining at the street block scale (Ding, Xu, and Wang Citation2020). Considering the average area of street blocks in our study area, we conducted many attempts and found that the number of street blocks with an effective kernel density value was maximized when the average influence radius of POI was set to 250 m with a pixel size of 10 m. Finally, the frequency density of the kernel density value in each street block is calculated by weighting, enabling the identification of the dominant function within the street block. This approach facilitates the completion of the division of socioeconomic functions. The formula for calculating frequency density is shown in Equation (1-2):
(1)
(1)
(2)
(2) where
is the frequency density of a single POI type kernel density value.
is the kernel density value of type
POI in a street block.
is the weight of type
POI in a street block.
stands for the number of POI types. In this study, m = 6.
is the frequency density of street block
. When
is greater than 50%, the street block is dominated by a single-type of POI function. In other cases, the comparison will be made between the top two functional types of POI with the highest frequency density, and the dominant function is determined by the POI with greater importance.
Further, we overlaid the remaining land cover types of natural ecology-agricultural production landscape functions from the LULC data, along with the aquaculture ponds thematic data. This integration process allowed us to derive the final parcel-scale landscape functions. By combining the socioeconomic functions identified at the street block level with the natural ecology-agricultural production landscape functions at the parcel-scale, we achieved a comprehensive representation of the diverse landscape functions within the study area.
2.4.2. Field data acquisition
In-field ground truthing is important to ensure a reliable accuracy assessment by providing accurate and direct examination of landscape functions at specific sampling locations. However, due to the hindrance caused by the COVID-19 pandemic, our field investigation efforts were impeded from 2020 to 2022. To address this, we strategically organized a 5-day field trip in the GBA from 13 to 18 March 2023 (). To mitigate the impact of time and phase inconsistency on accuracy assessment, we carefully selected in-field points from areas with minimal changes on the remote sensing image from 2020 to 2023. Moreover, these points were deliberately distributed evenly and encompassed all types of parcel-scale landscape functions. As a result, we successfully acquired 28 representative field collection points () and 1780 field photos ().
Table 4. Dates, cities and points of field data collection in GBA.
2.4.3. Multi-scale landscape functions mining by NRCA
Within one city or region, it is common to have several advantageous functions coexisting, with one or two functions playing a dominant role in the city's development while others contribute in a supportive capacity. For the urban agglomerations, finding the primary and secondary landscape functions of each cities is the key to realize the coordinated development of functions across cities. Normalized Revealed Comparative Advantage (NRCA) index, a valuable tool in studying regional comparative advantage in economics, is often used to reveal the extent to which a country or region has a comparative advantage in commodities (Yu, Cai, and Leung Citation2009). In this study, we employed the NRCA to quantitatively mine the comparative advantageous landscape functions of the GBA at town, county, and city scales to highlight the variations in landscape functional resource endowment across different regions. The calculation formula of NRCA is shown in EquationEquation (3(3)
(3) ):
(3)
(3) where
is the total area of type
landscape functions of
city;
represents the total area of type
landscape functions of all cities;
stands for the total area of all landscape functional types of the
city;
means the total area of all landscape functional types in all cities.
When >0, it indicates that the city has a comparative advantage in this landscape function, and the larger the value, the stronger the comparative advantage. When
<0, this landscape function has no comparative advantage. In this study, if there is only one parcel-scale landscape function type that has a positive
value, then it is considered the sole advantage function of the city. If there are two or more parcel-scale landscape function types with a
>0, then we identify the top two types with the highest
values as the primary function and secondary landscape function, respectively.
3. Results
3.1. Accuracy assessment
We employed the stratified random sampling method (Aoyama Citation1954) to validate the accuracy of parcel-scale landscape function. First, within the built-up area of LULC, 100 points were sampled randomly for each LULC type. Secondly, at the parcel-scale, we removed samples points falling outside of the parcel of their corresponding landscape functions by visual examination supported by high-resolution image in Google Earth pro, electronic map, and the rich information from the in-field data. Through repeated iterations of this process, we obtained 30 final random verification points for each type. This method was applied similarly to generate the final random verification points for non-built-up areas of parcel-scale landscape functions. The final spatial distribution of random verification points is shown in .
The accuracy assessment of the final parcel-scale landscape functions was conducted using a confusion matrix (Foody Citation2002), and the calculation results are shown in , including Overall accuracy (OA), Producer accuracy (PA), User accuracy (UA). The OA fall in the range from 0 to 100%, representing the overall accuracy of the classification results of parcel-scale landscape function, the higher the OA, the better the overall performance of our mining method. The PA measures the probability that a particular type on the ground is correctly identified. Within a value range between 0 and 1, a high PA indicates that the classification is effective in correctly identifying instances of a specific type. The UA is mainly concerned with the probability that each patch is correctly classified. Within the range of 0–1, a high UA indicates that the classification results are reliable and individual patches assigned to a certain type are likely to truly belong to that type on the grounds. The results () showed that the OA of the parcel-scale landscape function in the GBA in 2020 was 70.22%, which indicates a high level of consistency between the results of landscape functions mining at the parcel-scale and the actual situation. The UA of RES and BUS are relatively low, while the other landscape functions have better accuracy, especially, aquaculture ponds, its UA and PA were both greater than or equal to 90%.
3.2. Parcel-scale landscape functions heterogeneity of the GBA
shows the overall and localized distribution of landscape function within the GBA at the parcel-scale. present the area and proportion of socioeconomic and natural-agricultural parcel-scale landscape function types in the GBA, respectively. An extensive expansion of forest function was observed surrounding the GBA, covering the largest area of 28441.24km2 (representing 53.35% of the total coverage in parcel-scale landscape function). The socioeconomic landscape functions types are mainly distributed in the central area of the GBA. Among them, the industry function covers the largest area, spanning 2614.96km2 (constituting 5.23%), followed by the business function and residential function with coverage of 2,144.95 km2 (2.70%) and 1,377.11 km2 (3.59%), respectively. Among the natural ecology and agricultural production landscape functions types, the area of paddy, dry land, and aquaculture functions are second only to forest, with an area of 7563.23km2 (14.24%), 3345.09km2 (6.26%) and 1932.98km2 (3.86%), respectively. Therefore, from the perspective of the entire urban agglomeration, the GBA exhibits industry function and forest function as the two primary landscape functions.
Figure 8. Parcel-scale landscape functions mapping of the GBA. a. Overall distributions. b.–d. Three selected regions in GBA show the parcel-scale landscape functions in details. NON means none data.

Table 5. Area and proportion of parcel-scale landscape function in 2020.
3.3. Multi-scale landscape functions heterogeneity in administrative units division
3.3.1. Town scale
In terms of primary landscape functions (-town(a)), forest is the primary landscape function type of a large number of towns located in the northwestern and northeastern parts of the GBA. In addition to forest, paddy and dry land are the primary landscape function types of most towns in Jiangmen and Huizhou. Aquaculture is the primary landscape function type of most towns in Zhuhai, Zhongshan, and Foshan. Central Foshan and most towns in Shenzhen and Dongguan are mainly industry landscape function type. The distribution of towns with business landscape function type is relatively scattered. Concerning secondary landscape functions (-town(b)), most towns in Zhaoqing and Huizhou lack secondary landscape functions. In addition, areas with the same secondary landscape function types are less clustered spatially than those with the primary landscape function types.
3.3.2. County scale
Regarding primary landscape functions (-county(a)), the forest landscape function type remains the most widely distributed in space. The industrial landscape function type dominates the eastern part of Huizhou and the western part of Zhuhai. The eastern part of Guangzhou is dominated by the residential landscape function type. The business landscape function type prevails in Zhongshan, Macao, southwestern Guangzhou, and east and central Shenzhen. Aquaculture landscape function type is prominent in the northwest part of Shenzhen and the middle part of Foshan. Paddy is concentrated in Guangzhou and Foshan as the primary landscape function type with a strip-looking distribution. In terms of secondary landscape functions (-county(b)), paddy is the most widely distributed secondary landscape function type in space. Aquaculture is found to be the secondary landscape function type of Jiangmen in the west, Foshan in the north, and Zhuhai in the east. Business serves as the secondary landscape function type in the central and eastern parts of Guangzhou, Dongguan, and northwestern parts of Shenzhen. Water emerges as the secondary landscape function type in the northwest of Jiangmen, southwest of Foshan, and west of Guangzhou.
3.3.3. City scale
Regarding primary landscape functions (-city(a)), the primary landscape function type of Huizhou and Zhaoqing is forest. Foshan and Dongguan are dominated by industry landscape function type. Zhongshan and Zhuhai are dominated by the aquaculture landscape function type. The primary landscape function types of Jiangmen, Macao, and Hong Kong are paddy, residential, and grass, respectively. In terms of secondary landscape functions (-city(b)), the secondary landscape function types of Guangzhou, Dongguan, and Macao are business. Paddy landscape function type is secondary in Huizhou and Jiangmen. Zhongshan and Shenzhen's secondary landscape function type are industry. The secondary landscape function type of Zhuhai and Hong Kong is residence. Foshan's secondary landscape function type is aquaculture.
4. Discussion
4.1. High consistency with regional functional positioning
Regional development planning is a comprehensive decision-making process that incorporates diverse statistical indicators and data sources (Bangi Citation2012; Sowińska-Świerkosz and Soszyński Citation2022), which could effectively verify our landscape functions mining results. We conducted a thorough review of important government websites and documents related to the GBA, such as the Special page on Statistics of GBA (https://www.dsec.gov.mo/BayArea/#home) and the ‘Outline of the Development Plan for the GBA.’ We carefully examined and summarized the relevant information on the urban functions and development orientation of the GBA and each cities. Specifically, at the city scale, we compared our findings with urban functions and discovered that the landscape functions mining results based on big geodata are largely consistent with the urban function positioning outlined in government policies ().
In our research results, distinct landscape function types emerge for different cities. For instance, the major landscape function types of Zhaoqing and Jiangmen are forest-shrub and forest-paddy, respectively. This suggests that Zhaoqing is suitable to be designated as a tourism ecology city, while Jiangmen aligns with the classification of a green modern landscape city. Similarly, Zhongshan and Foshan exhibit landscape function types dominated by aquaculture ponds-industry and industry-aquaculture ponds, respectively. Therefore, they are relatively reasonable to be designated as agricultural demonstration areas and manufacturing centers. Furthermore, our comparison of landscape functions mining results with the development positioning of other cities, such as Guangzhou, Dongguan, Shenzhen, and Macau, also reveals consistent patterns. This congruence underscores the reliability and applicability of our landscape functions analysis in aligning with the intended developmental roles and characteristics of these cities ().
Table 6. Comparing result of landscape functions mining with government planning documents.
However, the comparison results for Hongkong deviated somewhat, the emphasis on being an international financial center was not prominent in our results. This disparity may be due to the fact that Hongkong is covered by 70% ecological land, with the inner city dominated by high-rise buildings. The economic value generated per unit area is relatively high, but the proportion of space resources allocated to the commercial service industry is limited (Zhao Citation2009). Therefore, exploring the functions of economically efficient cosmopolitan cities like Hong Kong solely based on the area of land functions has its limitations. In the future, it will be necessary to further improve the accuracy of mining regional functions by integrating specific socioeconomic indicators, such as GDP and trade volume, into the analysis.
At county and town scales, given the multitude of regions, we selected representative cases from the planning document for comparative description. Firstly, at the county scale, Shenzhen is one of the four central cities of the GBA, and its inner Nanshan stands out as a pivotal district, recognized for its affluence and designated as one of the first ‘regional demonstration bases’ for mass entrepreneurship and innovation in China. In 2020, Nanshan ranks first among all counties in Shenzhen with a total GDP of 650,222 million yuan (www.szns.gov.cn). Our study identifies the main and secondary landscape function types in Nanshan as forest and industry, respectively. This finding aligns with the planning objectives, where Nanshan aspires to establish itself as a green development highland and a world-class innovative coastal central city.
Moving to the town scale, Hengqin, the exclusive cross-border economic cooperation zone in Zhuhai and a key gateway to the GBA, has experienced rapid economic growth in recent years. The GDP of Hengqin New Area reached 40.699 billion yuan in 2020 (www.hengqin.gov.cn/). Our study highlights the main and secondary landscape function types in Hengqin as business and residential, respectively. In the planning vision, situated adjacent to Macau, Hengqin aims to become a trailblazing hub for scientific and technological innovation, providing a new living and working space for residents of Macao. Accordingly, the landscape function mining results, derived from big geodata, harmonize seamlessly with the urban function positioning at both town and county scales.
4.2. Implications of multi-scale analysis to landscape functional coordinated development
Compared with previous researches on landscape functions mining with administrative boundary as the main macro scale (Aumayr Citation2007; Hall Citation2006) and street blocks as the main micro scale (Herold, Couclelis, and Clarke Citation2005; Simwanda and Murayama Citation2017; Vanderhaegen and Canters Citation2017), this study, from the perspective of coordinated development of urban agglomerations, on the basis of mining parcel-scale landscape functions, further explored the landscape function organizations at the town, county and city scales. It effectively deepened our understanding of the landscape functions structure and connections of the GBA. At present, there are obvious scale effects have been proven in accessible green space landscape difference, evolutionary mechanisms of industry landscape, even spatial assessment of ‘production-living-ecological’ landscapes (Chen et al. Citation2022; Gao et al. Citation2022; Zhuang and Ye Citation2023), which puts forward new requirements for the scientific management of multi-scale social economy and ecological landscapes in large regions. Here, we selected Guangzhou and Foshan in the GBA to unfold our urban planning implications based on the results of multi-scale analysis. The ‘Integration of Guangzhou and Foshan’ serves as a crucial example of coordinated regional development within the GBA, aiming to achieve synchronized economic growth and upgrading through cooperation and mutual benefit. Therefore, understanding the cross-scale landscape functional differentiation is undoubtedly important for Guangzhou-foshan to make more reasonable functional collaborative planning. We discovered distinct and unique landscape functions patterns at different scales by conducting NRCA calculations in both Guangzhou and Foshan.
At the city scale, the result shows that Foshan excels in industry and agriculture landscape functions, while Guangzhou focuses primarily on ecology and business landscape functions (-d1, d2). Therefore, the development path of ‘resource sharing and functional complementarity’ can be considered a wise choice for Guangzhou and Foshan. In terms of economic development, enhancing infrastructure establishment such as improving transportation networks (Farahani et al. Citation2013), which could further facilitate the trade and business exchanges between the two cities.
Figure 10. The multi-scale structure characteristics of landscape functions and the design of development paths in Guangzhou and Foshan. The upper left corner of the sub figure is marked with different scales. (a) and (b) represent the primary landscape functions and secondary landscape functions at town, county and city scales, respectively.

At the county scale, distinct areas in Guangzhou and Foshan are emerging, where agriculture, particularly characterized by paddy fields and aquaculture ponds, takes center stage (-c1, c2). Among them, the paddy landscape function dominated in the northeast-southwest direction can be considered as the primary agricultural development mode in Guangzhou and Foshan. The ecological landscape function dominated by forest forms a natural ecological barrier under this mode, which to some extent could promote the formation of modern green agriculture (Koohafkan, Altieri, and Gimenez Citation2012). In addition, the paddy and aquaculture ponds landscape functions dominated in the northwest-southeast direction can be seen as a secondary mixed agricultural development mode in Guangzhou and Foshan. In the future, producing various agriculture produces or foods to meet the diverse nutritional requirement of people could be considered as a feasible path.
At the town scale, Guangzhou and Foshan demonstrates a landscape function structure with an elliptical concentric-circle pattern as the main development feature, supported by a multi-center structure (-b1, b2). On the one hand, the concentric structure exhibits functional differentiation from the center to the periphery. The first concentric ring is dedicated to residential purposes, the second ring focuses on industry-business activities, the third ring comprises paddy-aquaculture, and the fourth ring consists of the forest. Therefore, in the future, while preserving the ecological environment of the fourth ring, efforts could be made to enhance the technological and innovative levels of the industry-business sectors in the second ring, which help to establish modern and environmentally-friendly agriculture in the third ring. On the other hand, the spatial layout encompasses multiple residential and industry-business clusters arranged in a triangular pattern. Many urban areas or metropolitan areas in Europe such as Brussels, Ghent, etc. have adopted polycentric spatial structures in their planning to achieve balanced spatial development (Möck and Küpper Citation2020). Therefore, it is advisable to emphasize balanced development and avoid excessive concentration of resources in the future. Furthermore, each center can leverage its own distinctive advantageous industries to promote competition and cooperation among regions, thereby enhancing the overall development level of the area.
Finally, at the parcel-scale (a), the residential and business landscape functions of Guangzhou and Foshan are concentrated and distributed at the intersection of their administrative boundaries, which undoubtedly becomes a key element facilitating close socioeconomic connections between the two cities. Additionally, in the northern part of Foshan, there is a distribution of aquaculture ponds, paddy, dry land, and adjacent industry landscape functions, surrounded by forest landscape function. Hence, this region has the potential for developing green leisurial functions, and a diversified and processing-intensive agricultural development model can also be considered for the northern part of Foshan in the future. In the northeastern region of Guangzhou, there are large areas of paddy and dry land landscape functions, and it could be considered to forge modern agriculture comprehensive development model on the premise of not changing the shape of the parcel (Ge et al. Citation2019).
Furthermore, as a finely detailed landscape function unit, leveraging the power of big geodata, we have the opportunity to delve deeper into significant attribute information within each specific functional parcel in the future, including vital data such as population, GDP, and NPP (Net Primary Productivity).etc. The aim is to create an integrated ‘spatial scene’ unit that seamlessly combines social, economic, and ecological attribute information (Wang et al. Citation2022a). In this way, different from using LULC data, we could try to construct a novel assessment model that in spatial sustainable development, natural hazard, and resources and environment carrying capability, etc based on previous studies (Gao et al. Citation2022; Liu et al. Citation2020; Meng et al. Citation2023; Zeng et al. Citation2023), and realize spatial intelligent optimization in future regional development at a finer scale (Pan et al. Citation2023; Tang et al. Citation2022).
4.3. Potential factors of landscape functions heterogeneity at different spatial scales
The disparities in landscape functions across various scales are governed by systematic influencing mechanisms. Whether at the scale of parcel, where human activities in a small area are vividly depicted, or at the town scale, artificial factors typically play a pivotal role in contributing to the heterogeneity of landscape functions. A case in point is the government's implementation of land market reform, introducing the dual-track land right and subsequently resulting in variations in urban and rural land prices. This, in turn, has spurred an increase in landscape functions with an industrial focus in suburban areas (Feng and Zhou Citation2005; Wei and Zhao Citation2009). Furthermore, planners take into account a combination of factors such as the city's developmental history, transportation network structure, social population movements, and land rent to systematically categorize the urban landscape functions into business, industry, and residence. This strategic approach aims to promote sustainable urban and community development, incorporating well-established urban spatial development patterns like concentric-circle (Burgess, Park and McKenzie Citation1925), sectors (Hoyt Citation1939), and multiple centers (Harris and Ullman Citation1945). In this study, with the introduction of the ‘14th Five-Year Plan’ for the development of Guangzhou-Foshan urbanization, coupled with improvement in transportation infrastructure, the construction of manufacturing bases and the delineation of development areas, has diversified and perfected landscape function types in the two regions. This acceleration has further facilitated the pace of urban integration. At the town scale, the observed features of concentric circles and multiple centers spatial structure of landscape functions in Guangzhou-Foshan underscore that the government's systematic urban planning layout is the primary influencing factor.
However, at the county and city scales, natural factors predominantly shape the large-scale landscape's functional differentiation. For example, Fan (Citation2015) highlighted the varied advantages in resource endowment based on the geography, landform and climatic environment of different regions, facilitating the delineation of multi-scale functional zones at the national, provincial and county in China. In this study, the ecological landscape functions of Zhaoqing, Jiangmen and Huizhou at the county and city scales stands out, a consequence of the topography surrounding the GBA with mountains on three sides. These areas have a relatively high altitude, sufficient light and humidity, and vigorous vegetation growth, making them well-suited for leisure tourism and eco-tourism cities. On the other hand, Dongguan and Shenzhen primarily exhibit industry and business landscape functions. This is attributed their proximity to the mouth of the Pearl River, featuring deep water ports and an advantageous geographical location. These conditions create favorable circumstances for large-scale cargo transportation and international traffic and trade, hence shaping the predominant landscape functions of industry and commerce in these two cities.
Moving forward, our research aims to delve into specific social, economic, and natural indicators across various scales. Employing analytical tools like the geographical detector (Wang et al. Citation2010), we plan to conduct systematic and quantitative analyzes of multi-scale landscape function differentiations. This approach will deepen our understanding of the relative influence of diverse factors, offering more nuanced insights into urban planning considerations.
4.4. Applicability and limitation of our study framework
The proposed framework was developed based on open big geodata as its primary data source, providing a foundation for robust transferability and generalization to other regions. The key datasets used in this study such as Land Use/Land Cover (LULC), road networks, Point of Interest (POI), and even remote sensing thematic data have achieved unparalleled global coverage (Haklay and Weber Citation2008; Jia et al. Citation2023; Wang et al. Citation2022b; Yang and Huang Citation2021; Zanaga et al. Citation2022). The widespread availability allows other scholars to conduct similar experiments in other regions using our framework. In comparison, the socioeconomic data used in previous studies have varied availability across countries and inconsistent statistical methods and standards persist (Jean et al. Citation2016). This variability poses potential obstacles to the reproducibility of relevant studies. For example, Song et al. (Citation2021) encountered difficulties in mining regional functions at the city scale in China due to the lack of relevant statistical data in the Qinghai-Tibet Plateau, Inner Mongolia, and some regions of Yunnan. In contrast, McCallum et al. (Citation2022) successfully used the big geodata of night lights to map economic growth, the scale of poverty, and environmental conditions, especially in places where conventional survey data are lacking. This further shows that the application of big geodata mining landscape functions in less developed countries and regions has hope and potential.
Moreover, big geodata offers the opportunity to overcome the constraints imposed by administrative boundaries, enabling landscape function mining at non-administrative boundaries. For instance, landform zones, the spatial unit for studying the impact of landform on the shaping and altering process of the Earth's surface (Cheng et al. Citation2011). Detailed information on landform zones within the GBA is provided in Supporting material 1. To illustrate, we present detailed results for Hong Kong and Dongguan within the GBA (). In Hongkong, aside from forest, paddy, and residential landscape function types, business function of distributing in the low-elevation marine alluvial plain accounts for a large proportion of secondary landscape functions. In Dongguan, in addition to aquaculture, paddy, and dry land landscape function types, industry function of distributing in the low altitude erosional denudation low platform plays a substantial role as a secondary landscape function type. These findings are consistent with the urban functional positioning of Hong Kong and Dongguan. Besides, we also found the landform type and natural boundary influence the heterogeneity of landscape functions. Therefore, the use of big geodata to mine landscape functions holds immense potential in serving various fields, which could contribute to more informed decision-making in multiple disciplines, benefiting a wide range of stakeholders.
Figure 11. Mining landscape functions distribution at the landform zone by NRCA. a.-b. represents the primary and secondary landscape functions, respectively of the second-level landform zone in Hongkong. c.-d. represents the primary and secondary landscape functions, respectively, of the second-level landform zone scale in Dongguan. NOD means no data.

This framework presents opportunities for refinement. Firstly, the inherent positional bias of POIs and the insufficient subdivision of street block sizes contribute to a less than optimal analytical details and accuracy, especially for residential and business functions. A potential avenue for improvement involves correcting POI locations and considering further subdivision within street blocks, incorporating landscape pattern features of buildings to provide more detailed insights (Xing and Meng Citation2018; Zhou et al. Citation2020). Secondly, the multi-scale mining of landscape functions in this study is exclusively based on the areal coverage of parcel-scale landscape functions. However, urban functional development direction can be influenced by various factors, such as the proportion of populations engaged in different industries (Wang et al. Citation2012). Contemporary studies are exploring the combination of socioeconomic statistics and big geodata, showcasing promising results in multi-scale landscape function mining (Rosier et al. Citation2022;Srivastava, Vargas-Munoz, and Tuia Citation2019). Therefore, with access to comprehensive socioeconomic data, integrating big geodata and socioeconomic data can also be considered a viable option to enhance the robustness of our framework.
5. Conclusion
To address the issue of previous studies focusing on a single macro or micro scale landscape functions mining, which makes it difficult to understand the complex multi-scale landscape functions structure in large regions. This study proposes a framework for mining multi-scale landscape functions from parcel to urban agglomerations using big geodata. The study area selected is the GBA in China, one of the world's four major bay areas. We combined four types of big geodata, namely LULC, road networks, POI and remote sensing thematic data, to divide the parcel-scale landscape functions with frequency density and spatial overlay methods. Subsequently, The NRCA was then employed to identify the primary and secondary landscape functions of the GBA at town, county, city scales even non-administrative boundary scale. The main conclusions are as follows:
Big geodata are proved to be effective in mining multi-scale landscape functions through showing high consistencies including in-field investigations and comparisons with established policy planning positions.
Multi-scale landscape functions mining using big geodata, which enable us to understand the unique structure characters and development patterns of landscape functions across scales. This approach provides valuable insights for regional coordinated development.
In addition to the traditional administrative unit divisions, big geodata is also adept at uncovering multi-scale landscape functions irrespective of administrative boundaries, such as natural geographical zones.
The framework proposed in this study offers significant potential for rapidly and cost-effectively mining of multi-scale and large-scope landscape functions, thereby supporting urban planners in their planning, design, and scientific research endeavors.
Geolocation information
State Key Laboratory of Resources and Environmental Information System, Institute of Geographic Sciences and Natural Resources Research, Chinese Academy of Sciences (No. 11, Datun Road, Chaoyang District, Beijing, China).
Disclosure statement
No potential conflict of interest was reported by the author(s).
Data availability statement
The data are available from the corresponding author on reasonable request.
Additional information
Funding
References
- Al-Harbi, K. M. A. S. 2001. “Application of the AHP in Project Management.” International Journal of Project Management 19 (1): 19–27. https://doi.org/10.1016/S0263-7863(99)00038-1.
- Angelis, V., A. Angelis-Dimakis, and K. Dimaki. 2016. “Identifying Clusters of Regions in the European South, Based on Their Economic, Social and Environmental Characteristics.” Region 3 (2): 71–102. https://doi.org/10.18335/region.v3i2.81.
- Aoyama, H. 1954. “A Study of Stratified Random Sampling.” Annals of the Institute of Statistical Mathematics 6 (1): 1–36. https://doi.org/10.1007/BF02960514.
- Assessment, M. E. 2005. Ecosystems and Human Well-Being. Washington, DC: Island Press.
- Aumayr, C. M. 2007. “European Region Types in EU-25.” European Journal of Comparative Economics 4 (2): 110–147.
- Bangi, D. E. 2012. “Concepts, Approach and Indicators for Sustainable Regional Development.” Advances in Environmental Biology 6 (3): 967–980.
- Brown, I., and M. Castellazzi. 2014. “Scenario Analysis for Regional Decision-Making on Sustainable Multifunctional Land Uses.” Regional Environmental Change 14 (4): 1357–1371. https://doi.org/10.1007/s10113-013-0579-3.
- Burgess, E., R. Park, and R. McKenzie. 1925. The city: Suggestions forinvestigation of human behavior inthe urban environment. Chicago: University of Chicago Press 1–46.
- Buyantuyev, A., J. Wu, and C. Gries. 2010. “Multiscale Analysis of the Urbanization Pattern of the Phoenix Metropolitan Landscape of USA: Time, Space and Thematic Resolution.” Landscape and Urban Planning 94 (3-4): 206–217. https://doi.org/10.1016/j.landurbplan.2009.10.005.
- Cadenasso, M. L., S. T. Pickett, and K. Schwarz. 2007. “Spatial Heterogeneity in Urban Ecosystems: Reconceptualizing Land Cover and a Framework for Classification.” Frontiers in Ecology and the Environment 5 (2): 80–88. https://doi.org/10.1890/1540-9295(2007)5[80:SHIUER]2.0.CO;2.
- Chen, B., Y. Tu, S. Wu, Y. Song, Y. Jin, C. Webster, X. Bing, and P. Gong. 2022. “Beyond Green Environments: Multi-Scale Difference in Human Exposure to Greenspace in China.” Environment International 166: 107348. https://doi.org/10.1016/j.envint.2022.107348.
- Cheng, W., C. Zhou, B. Li, Y. Shen, and B. Zhang. 2011. “Structure and Contents of Layered Classification System of Digital Geomorphology for China.” Journal of Geographical Sciences 21 (5): 771–790. https://doi.org/10.1007/s11442-011-0879-9.
- De Groot, R. 2006. “Function-analysis and Valuation as a Tool to Assess Land use Conflicts in Planning for Sustainable, Multi-Functional Landscapes.” Landscape and Urban Planning 75 (3-4): 175–186. https://doi.org/10.1016/j.landurbplan.2005.02.016.
- De Groot, R., and L. Hein. 2007. “Concept and Valuation of Landscape Functions at Different Scales.” In Multifunctional Land use: Meeting Future Demands for Landscape Goods and Services, edited by U. Mander, H. Wiggering, and K. Helming, 15–36. Berlin: Springer Verlag. https://doi.org/10.1007/978-3-540-36763-5_2.
- Di Ludovico, D., and F. D’Ascanio. 2019. “European Cross-Scale Spatial Planning and Territorial Frames in the Italian Median Macroregion.” European Planning Studies 27 (7): 1369–1390. https://doi.org/10.1080/09654313.2019.1581729.
- Ding, Y., H. Xu, and C. Wang. 2020. “Research on Urban Functional Area Recognition Integrating OSM Road Network and POI Data.” Geogrpahical Information Science 36: 57–63. https://doi.org/10.3969/j.issn.1672-0504.2020.04.009.
- Du, S., S. Du, B. Liu, and X. Zhang. 2021. “Mapping Large-Scale and Fine-Grained Urban Functional Zones from VHR Images Using a Multi-Scale Semantic Segmentation Network and Object Based Approach.” Remote Sensing of Environment 261: 112480. https://doi.org/10.1016/j.rse.2021.112480.
- Egri, Z., and T. Tánczos. 2015. “Spatial Layers and Spatial Structure in Central and Eastern Europe.” Regional Statistics 5 (2): 34–61. https://doi.org/10.15196/RS05203.
- Fan, J. 2015. “Draft of Major Function Oriented Zoning of China.” Acta Geographica Sinica 70 (2): 186–201. https://doi.org/10.11821/dlxb201502002.
- Fang, C., and D. Yu. 2017. “Urban Agglomeration: An Evolving Concept of an Emerging Phenomenon.” Landscape and Urban Planning 162: 126–136. https://doi.org/10.1016/j.landurbplan.2017.02.014.
- Farahani, R. Z., E. Miandoabchi, W. Y. Szeto, and H. Rashidi. 2013. “A Review of Urban Transportation Network Design Problems.” European Journal of Operational Research 229 (2): 281–302. https://doi.org/10.1016/j.ejor.2013.01.001.
- Feng, J., and Y. Zhou. 2005. “Suburbanization and the Changes of Urban Internal Spatial Structure in Hangzhou, China.” Urban Geography 26 (2): 107–136. https://doi.org/10.2747/0272-3638.26.2.107.
- Fischer, A. P. 2018. “Forest Landscapes as Social-Ecological Systems and Implications for Management.” Landscape and Urban Planning 177: 138–147. https://doi.org/10.1016/j.landurbplan.2018.05.001.
- Foody, G. M. 2002. “Status of Land Cover Classification Accuracy Assessment.” Remote Sensing of Environment 80 (1): 185–201. https://doi.org/10.1016/S0034-4257(01)00295-4.
- Gao, K., X. Yang, Z. Wang, H. Zhang, C. Huang, and X. Zeng. 2022. “Spatial Sustainable Development Assessment Using Fusing Multisource Data from the Perspective of Production-Living-Ecological Space Division: A Case of Greater bay Area, China.” Remote Sensing 14 (12): 2772. https://doi.org/10.3390/rs14122772.
- Ge, D., Z. Wang, S. Tu, H. Long, H. Yan, D. Sun, and W. Qiao. 2019. “Coupling Analysis of Greenhouse-led Farmland Transition and Rural Transformation Development in China’s Traditional Farming Area: A Case of Qingzhou City.” Land Use Policy 86: 113–125. https://doi.org/10.1016/j.landusepol.2019.05.002.
- Gong, P., B. Chen, X. Li, H. Liu, J. Wang, Y. Bai, J. Chen, X. Chen, L. Fang, and S. Feng. 2020. “Mapping Essential Urban Land use Categories in China (EULUC-China): Preliminary Results for 2018.” Science Bulletin 65 (3): 182–187. https://doi.org/10.1016/j.scib.2019.12.007.
- Grabaum, R., and B. C. Meyer. 1998. “Multicriteria Optimization of Landscapes Using GIS-Based Functional Assessments.” Landscape and Urban Planning 43 (1-3): 21–34. https://doi.org/10.1016/S0169-2046(98)00099-1.
- Grippa, T., S. Georganos, S. Zarougui, P. Bognounou, E. Diboulo, Y. Forget, M. Lennert, S. Vanhuysse, N. Mboga, and E. Wolff. 2018. “Mapping Urban Land use at Street Block Level Using Openstreetmap, Remote Sensing Data, and Spatial Metrics.” ISPRS International Journal of Geo-Information 77: 246. https://doi.org/10.3390/ijgi7070246.
- Gu, C., Y. Wang, Y. Shao, and J. Gu. 2015. “Research on Administrative Divisions Based on Functional Areas Analysis: A Case of Shaoxing Metropolitan Area.” Acta Geographica Sinica 70: 1187–1201. https://doi.org/10.11821/dlxb201508001.
- Haklay, M., and P. Weber. 2008. “Openstreetmap: User-Generated Street Maps.” IEEE Pervasive Computing 7 (4): 12–18. https://doi.org/10.1109/MPRV.2008.80.
- Hall, P. G. K., ed. 2006. “Pain.” In The Polycentric Metropolis: Learning from Mega-City Regions in Europe. London: Earthscan.
- Harris, C. D., and E. L. Ullman. 1945. “The Nature of Cities.” The Annals of the American Academy of Political and Social Science 242 (1): 7–17. https://doi.org/10.1177/000271624524200103.
- Heffner, K., and P. Gibas. 2016. “Functional Areas in the Regions and Their Links to Scope sub-Regional Centres Impact.” Studia Regionalia, 27–39. https://doi.org/10.12657/studreg-46-02.
- Hermosilla, T., J. Palomar-Vázquez, Á Balaguer-Beser, J. Balsa-Barreiro, and L. A. Ruiz. 2014. “Using Street Based Metrics to Characterize Urban Typologies.” Computers, Environment and Urban Systems 44: 68–79. https://doi.org/10.1016/j.compenvurbsys.2013.12.002.
- Herold, M., H. Couclelis, and K. C. Clarke. 2005. “The Role of Spatial Metrics in the Analysis and Modeling of Urban Land use Change.” Computers, Environment and Urban Systems 29 (4): 369–399. https://doi.org/10.1016/j.compenvurbsys.2003.12.001.
- Herold, M., X. Liu, and K. C. Clarke. 2003. “Spatial Metrics and Image Texture for Mapping Urban Land use.” Photogrammetric Engineering & Remote Sensing 269 (9): 991–1001. https://doi.org/10.14358/PERS.69.9.991.
- Hoyt, H. 1939. “The Structure and Growth of Residential Neighborhoods in American Cities.” US Government Printing Office.
- Jean, N., M. Burke, M. Xie, W. M. Davis, D. B. Lobell, and S. Ermon. 2016. “Combining Satellite Imagery and Machine Learning to Predict Poverty.” Science 353 (6301): 790–794. https://doi.org/10.1126/science.aaf7894.
- Jia, M., Z. Wang, D. Mao, C. Ren, K. Song, C. Zhao, C. Wang, X. Xiao, and Y. Wang. 2023. “Mapping Global Distribution of Mangrove Forests at 10-m Resolution.” Science Bulletin, https://doi.org/10.1016/j.scib.2023.05.004.
- Koohafkan, P., M. A. Altieri, and E. H. Gimenez. 2012. “Green Agriculture: Foundations for Biodiverse, Resilient and Productive Agricultural Systems.” International Journal of Agricultural Sustainability 10 (1): 61–75. https://doi.org/10.1080/14735903.2011.610206.
- Leemans, R., and R. S. De Groot. 2003. Millennium Ecosystem Assessment: Ecosystems and Human Well-Being: A Framework for Assessment.
- Liu, X., and Y. Long. 2016. “Automated Identification and Characterization of Parcels with OpenStreetMap and Points of Interest.” Environment and Planning B: Planning and Design 43 (2): 341–360. https://doi.org/10.1177/0265813515604767.
- Liu, Y., C. Lu, X. Yang, Z. Wang, and B. Liu. 2020. “Fine-scale Coastal Storm Surge Disaster Vulnerability and Risk Assessment Model: A Case Study of Laizhou Bay, China.” Remote Sensing 12 (8): 1301. https://doi.org/10.3390/rs12081301.
- Ma, Q., J. Wu, C. He, and G. Hu. 2018. “Spatial Scaling of Urban Impervious Surfaces Across Evolving Landscapes: From Cities to Urban Regions.” Landscape and Urban Planning 175: 50–61. https://doi.org/10.1016/j.landurbplan.2018.03.010.
- McCallum, I., C. C. M. Kyba, J. C. L. Bayas, E. Moltchanova, M. Cooper, J. C. Cuaresma, S. Pachauri, et al. 2022. “Estimating Global Economic Well-Being with Unlit Settlements.” Nature Communications 13 (1): 2459. https://doi.org/10.1038/s41467-022-30099-9.
- Meng, D., Y. Liu, Z. Wang, X. Yang, X. Liu, J. Zhang, and K. Gao. 2023. “Decreasing Vulnerability of Storm Surge Disasters in Coastal Cities of China Over the Past 30 Years.” Journal of Marine Science and Engineering 11 (1): 128. https://doi.org/10.3390/jmse11010128.
- Möck, M., and P. Küpper. 2020. “Polycentricity at its Boundaries: Consistent or Ambiguous?” European Planning Studies 28 (4): 830–849. https://doi.org/10.1080/09654313.2019.1666802.
- Niu, N., X. Liu, H. Jin, X. Ye, Y. Liu, X. Li, Y. Chen, and S. Li. 2017. “Integrating Multi-Source big Data to Infer Building Functions.” International Journal of Geographical Information Science 31 (9): 1871–1890. https://doi.org/10.1080/13658816.2017.1325489.
- Novotný, J., S. Chakraborty, and I. Maity. 2022. “Urban Expansion of the 43 Worlds’ Largest Megacities: A Search for Unified Macro-Patterns.” Habitat International 129: 102676. https://doi.org/10.1016/j.habitatint.2022.102676.
- Ovando-Montejo, G. A., P. Kedron, and A. E. Frazier. 2021. “Relationship Between Urban Size and Configuration: Scaling Evidence from a Hierarchical System in Mexico.” Applied Geography 132: 102462. https://doi.org/10.1016/j.apgeog.2021.102462.
- Pan, T., F. Su, F. Yan, V. Lyne, Z. Wang, and L. Xu. 2023. “Optimization of Multi-Objective Multi-Functional Landuse Zoning Using a Vector-Based Genetic Algorithm.” Cities 137: 104256. https://doi.org/10.1016/j.cities.2023.104256.
- Pei, T., S. Sobolevsky, C. Ratti, S. L. Shaw, T. Li, and C. Zhou. 2014. “A new Insight Into Land use Classification Based on Aggregated Mobile Phone Data.” International Journal of Geographical Information Science 28 (9): 1988–2007. https://doi.org/10.1080/13658816.2014.913794.
- Ren, C., Z. Wang, Y. Zhang, B. Zhang, L. Chen, Y. Xi, X. Xiao, et al. 2019. “Rapid Expansion of Coastal Aquaculture Ponds in China from Landsat Observations During 1984–2016.” International Journal of Applied Earth Observation and Geoinformation 82: 101902. https://doi.org/10.1016/j.jag.2019.101902.
- Rosenblatt, M. 1956. “Remarks on Some Nonparametric Estimates of a Density Function.” The Annals of Mathematical Statistics, 832–837. https://doi.org/10.1214/aoms/1177728190.
- Rosier, J. F., H. Taubenböck, P. H. Verburg, and J. van Vliet. 2022. “Fusing Earth Observation and Socioeconomic Data to Increase the Transferability of Large-Scale Urban Land use Classification.” Remote Sensing of Environment 278: 113076. https://doi.org/10.1016/j.rse.2022.113076.
- Sharma, S., P. K. Joshi, and C. Fürst. 2022. “Exploring Multiscale Influence of Urban Growth on Landscape Patterns of two Emerging Urban Centers in the Western Himalaya.” Land 11 (12): 2281. https://doi.org/10.3390/land11122281.
- Simwanda, M., and Y. Murayama. 2017. “Integrating Geospatial Techniques for Urban Land use Classification in the Developing sub-Saharan African City of Lusaka, Zambia.” ISPRS International Journal of Geo-Information 6 (4): 102. https://doi.org/10.3390/ijgi6040102.
- Song, X., Q. Feng, F. Xia, X. Li, and J. Scheffran. 2021. “Impacts of Changing Urban Land-use Structure on Sustainable City Growth in China: A Population-Density Dynamics Perspective.” Habitat International 107: 102296. https://doi.org/10.1016/j.habitatint.2020.102296.
- Sowińska-Świerkosz, B., and D. Soszyński. 2022. “Spatial Indicators as a Tool to Support the Decision-Making Process in Relation to Different Goals of Rural Planning.” Land Use Policy 119: 106180. https://doi.org/10.1016/j.landusepol.2022.106180.
- Srivastava, S., J. E. Vargas-Munoz, and D. Tuia. 2019. “Understanding Urban Landuse from the Above and Ground Perspectives: A Deep Learning, Multimodal Solution.” Remote Sensing of Environment 228: 129–143. https://doi.org/10.1016/j.rse.2019.04.014.
- Sun, X., J. Wu, H. Tang, and P. Yang. 2022. “An Urban Hierarchy-Based Approach Integrating Ecosystem Services Into Multiscale Sustainable Land use Planning: The Case of China.” Resources, Conservation and Recycling 178: 106097. https://doi.org/10.1016/j.resconrec.2021.106097.
- Tang, Y., M. Wang, Q. Liu, Z. Hu, J. Zhang, T. Shi, G. Wu, and F. Su. 2022. “Ecological Carrying Capacity and Sustainability Assessment for Coastal Zones: A Novel Framework Based on Spatial Scene and Three-Dimensional Ecological Footprint Model.” Ecological Modelling 466: 109881. https://doi.org/10.1016/j.ecolmodel.2022.109881.
- Vanderhaegen, S., and F. Canters. 2017. “Mapping Urban Form and Function at City Block Level Using Spatial Metrics.” Landscape and Urban Planning 167: 399–409. https://doi.org/10.1016/j.landurbplan.2017.05.023.
- Van de Voorde, T., W. Jacquet, and F. Canters. 2011. “Mapping Form and Function in Urban Areas: An Approach Based on Urban Metrics and Continuous Impervious Surface Data.” Landscape and Urban Planning 102 (3): 143–155. https://doi.org/10.1016/j.landurbplan.2011.03.017.
- Vasishth, A. 2008. “A Scale-Hierarchic Ecosystem Approach to Integrative Ecological Planning.” Progress in Planning 70 (3): 99–132. https://doi.org/10.1016/j.progress.2008.05.001.
- Vu, T. T., N. V. A. Vu, H. P. Phung, and L. D. Nguyen. 2021. “Enhanced Urban Functional Land use map with Free and Open-Source Data.” International Journal of Digital Earth 14 (11): 1744–1757. https://doi.org/10.1080/17538947.2021.1970262.
- Wang, Z., K. Gao, X. Yang, F. Su, C. Huang, T. Shi, F. Yan, et al. 2022a. “Land use/Land Cover Classification Development from a Geographical Perspective.” Geographical Research 41: 2946–2962. https://doi.org/10.11821/dlyj020220076.
- Wang, J., X. Li, G. Christakos, Y. Liao, T. Zhang, X. Gu, and X. Zheng. 2010. “Geographical Detectors-Based Health Risk Assessment and its Application in the Neural Tube Defects Study of the Heshun Region, China.” International Journal of Geographical Information Science 24 (1): 107–127. https://doi.org/10.1080/13658810802443457.
- Wang, J., X. Liu, L. Peng, H. Chen, L. Driskell, and X. Zheng. 2012. “Cities Evolution Tree and Applications to Predicting Urban Growth.” Population and Environment 33 (2-3): 186–201. https://doi.org/10.1007/s11111-011-0142-4.
- Wang, Z., X. Yang, and C. Zhou. 2021. “Geographic Knowledge Graph for Remote Sensing big Data.” Journal OfGeoraphical Information Science 23 (1): 16–28. https://doi.org/10.12082/dqxxkx.2021.200632.
- Wang, Z., J. Zhang, X. Yang, C. Huang, F. Su, X. Liu, Y. Liu, and Y. Zhang. 2022b. “Global Mapping of the Landside Clustering of Aquaculture Ponds from Dense Time-Series 10 m Sentinel-2 Images on Google Earth Engine.” International Journal of Applied Earth Observation and Geoinformation 115: 103100. https://doi.org/10.1016/j.jag.2022.103100.
- Wei, Y., and M. Zhao. 2009. “Urban Spill Over vs. Local Urban Sprawl: Entangling Land-use Regulations in the Urban Growth of China’s Megacities.” Land use Policy 26 (4): 1031–1045. https://doi.org/10.1016/j.landusepol.2008.12.005.
- Werner, J., S. Herrmann, and A. Lovett. 2017. “Clusters of Rural European Regions: An Approach to Show the Multi-Dimensional Character of Core-Peripheral Patterns.” Core-Periphery Patterns Across the European Union, 177–197. https://doi.org/10.1108/978-1-78714-495-820171006.
- Willemen, L., P. H. Verburg, L. Hein, and M. E. van Mensvoort. 2008. “Spatial Characterization of Landscape Functions.” Landscape and Urban Planning 88 (1): 34–43. https://doi.org/10.1016/j.landurbplan.2008.08.004.
- Wu, J., and O. L. Loucks. 1995. “From Balance of Nature to Hierarchical Patch Dynamics: A Paradigm Shift in Ecology.” The Quarterly Review of Biology 70 (4): 439–466. https://doi.org/10.1086/419172.
- Xie, G., C. Lu, L. Zhen, S. Cao, Y. Zhang, and Y. Leng. 2009. “Objective, Progress and Methodology of Spatial Function Zoning.” Geographical Research 28 (3): 561–570. https://doi.org/10.1016/S1874-8651(10)60080-4.
- Xing, H., and Y. Meng. 2018. “Integrating Landscape Metrics and Socioeconomic Features for Urban Functional Region Classification.” Computers, Environment and Urban Systems 72: 134–145. https://doi.org/10.1016/j.compenvurbsys.2018.06.005.
- Yan, X., C. Song, T. Pei, X. Wang, M. Wu, T. Liu, H. Shu, and J. Chen. 2022. “Revealing Spatiotemporal Matching Patterns Between Traffic Flux and Road Resources Using big Geodata-A Case Study of Beijing.” Cities 127: 103754. https://doi.org/10.1016/j.cities.2022.103754.
- Yang, J., and X. Huang. 2021. “The 30 m Annual Land Cover Dataset and its Dynamics in China from 1990 to 2019.” Earth System Science Data 13 (8): 3907–3925. https://doi.org/10.5194/essd-13-3907-2021.
- Yang, C., Q. Li, Z. Hu, J. Chen, T. Shi, K. Ding, and G. Wu. 2019. “Spatiotemporal Evolution of Urban Agglomerations in Four Major bay Areas of US, China and Japan from 1987 to 2017: Evidence from Remote Sensing Images.” Science of the Total Environment 671: 232–247. https://doi.org/10.1016/j.scitotenv.2019.03.154.
- Yao, Y., X. Li, X. Liu, P. Liu, Z. Liang, J. Zhang, and K. Mai. 2017. “Sensing Spatial Distribution of Urban Land use by Integrating Points-of-Interest and Google Word2Vec Model.” International Journal of Geographical Information Science 31 (4): 825–848. https://doi.org/10.1080/13658816.2016.1244608.
- Yu, R., J. Cai, and P. Leung. 2009. “The Normalized Revealed Comparative Advantage Index.” The Annals of Regional Science 43 (1): 267–282. https://doi.org/10.1007/s00168-008-0213-3.
- Zanaga, D., R. Van De Kerchove, D. Daems, W. De Keersmaecker, C. Brockmann, G. Kirches, J. Wevers, et al. 2022. ESA WorldCover 10 m 2021 v200.
- Zeng, X., X. Yang, S. Zhong, Z. Wang, Y. Ding, D. Meng, and K. Gao. 2023. “Comprehensive Evaluation of Resource and Environmental Carrying Capacity at a National Scale: A Case Study of Southeast Asia.” Sustainability 15 (7): 5791. https://doi.org/10.3390/su15075791.
- Zhang, X., S. Du, and Q. Wang. 2017. “Hierarchical Semantic Cognition for Urban Functional Zones with VHR Satellite Images and POI Data.” ISPRS Journal of Photogrammetry and Remote Sensing 132: 170–184. https://doi.org/10.1016/j.isprsjprs.2017.09.007.
- Zhao, S. 2009. “Rethinking the Meaning of Intensive Land use: Based on Analyzing Intensive Land use Patterns in Hongkong.” China Land Sci 23: 73–77.
- Zhou, Y.. 2023. “The Historical Evolution and Future Prospects of the Guangdong-Hong Kong-Macao Greater Bay Area.” In Studies on China's Special Economic Zones 5, 81–92. Singapore: Springer Nature Singapore.
- Zhou, W., D. Ming, X. Lv, K. Zhou, H. Bao, and Z. Hong. 2020. “SO–CNN Based Urban Functional Zone Fine Division with VHR Remote Sensing Image.” Remote Sensing of Environment 236: 111458. https://doi.org/10.1016/j.rse.2019.111458.
- Zhuang, L., and C. Ye. 2023. “More Sprawl Than Agglomeration: The Multi-Scale Spatial Patterns and Industrial Characteristics of Varied Development Zones in China.” Cities 140: 104406. https://doi.org/10.1016/j.cities.2023.104406.