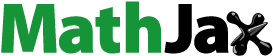
ABSTRACT
The intensified thermal environment in suburban areas is raising wide concerns for human society and public health due to rapid urbanization. Although the satellite-derived surface urban heat island intensity (SUHII) is a commonly used indicator, it still needs to be determined the SUHII between urban and suburban areas due to the challenges in delineating their boundaries with changes. Thus, a comprehensive analysis of the spatial explanatory variables (SEVs) and SUHII among urban and suburban areas is highly needed. Here, using the long-term satellite observations, we analyzed the spatiotemporal patterns of SUHII in different temporal intervals (i.e. seasonal and diurnal) and the contribution of SEVs in urban and suburban areas. Our results indicate that SUHII shows predominantly increasing trend from 2012–2021 in cities of China. Despite the trends of SEVs (i.e. increasing/decreasing) being relatively consistent in both urban and suburban, the latter shows a higher proportion regarding the trends in various SEVs. Besides, the partial least squares regression (PLSR) model shows that the major contributors to SUHII in urban areas are landscape shape index (LSI), patch density (PD), and the digital elevation model (DEM), while in suburban areas, those critical SEVs are LSI, normalized difference built-up index (NDBI), and DEM. These findings can facilitate the sustainable design of urban planning in a nature-based solution.
1. Introduction
China’s rapid urbanization process has caused the urban heat island (UHI) effect by altering the urban land surface (Guo et al. Citation2020; Xu et al. Citation2022; Li, Liu, and Yu Citation2014). The UHI is widely seen in the urban ecosystem, where the temperature in urban areas is hotter than nearby suburban areas (Chatterjee and Majumdar Citation2022; Amir Siddique et al. Citation2021; Moazzam, Doh, and Lee Citation2022). As a commonly used indicator to characterize the UHI, the surface UHI intensity (SUHII) derived from the satellite observations of land surface temperature (LST) data, has been widely used in diverse applications (Moazzam, Doh, and Lee Citation2022; Yang, Huang, and Tang Citation2019; Gage and Cooper Citation2017; Shi et al. Citation2023). The intensified UHI effect has raised wide concerns on human beings, such as heatwave and energy consumption, and water-atmosphere interactions, particularly under future development goals with sustainable cities (Chatterjee and Majumdar Citation2022; Moazzam, Doh, and Lee Citation2022; Amir Siddique et al. Citation2021; Macintyre et al. Citation2021; Hsu et al. Citation2021; Mu et al. Citation2022).
The spatiotemporal pattern of SUHII is closely related to diverse direct and indirect factors such as socioeconomic level, urban landscape, and urban form (Li et al. Citation2020; Ramírez-Aguilar and Souza Citation2019; Adelia et al. Citation2019; He et al. Citation2023). Large amounts of socio-economic activities (e.g. population density, building usage patterns, traffic flow and industrial activity) during urbanization can increase the heat capacity of the city, leading to the change of the UHI (Guo et al. Citation2020; Liu et al. Citation2022; Liang et al. Citation2020). In addition, the landscape pattern indexes could also be utilized to analyze the distribution and spatial organization characteristics of various landscape types within cities, facilitating studies relating to the formation and evolution of UHI. However, different landscape indexes can yield varied impacts on SUHII due to their distinct spatial patterns of land use and land cover (Shao et al. Citation2023; Guo et al. Citation2020; Liu et al. Citation2021). Moreover, the natural environment plays a significant role in influencing UHI through various mechanisms. For instance, topographic features such as mountains and rivers can alter the climate and temperature distribution within cities, thereby impacting the intensity of UHI (Liu et al. Citation2023; Hu, Dai, and Guldmann Citation2020). Besides, a series building environment (e.g. building height, building density and building materials) can impact SUHII by obstructing wind flow and absorbing and storing solar energy (Shao et al. Citation2023; Hu, Dai, and Guldmann Citation2020; Yin et al. Citation2018; Zhou et al. Citation2022). However, existing studies mainly focused on examining the impact factors of SUHII only from the perspective of urban areas. Meanwhile, there is a lack of long-term investigation into the multidimensional drivers of SUHII.
Suburban areas, as sensitive zones of urbanization, have a gap in studies of the long-term spatial explanatory variables (SEVs)’ impacts on SUHII due to the difficulty of identifying their boundaries and their distinct differences from urban area (Moazzam, Doh, and Lee Citation2022; Ming et al. Citation2023; Zhang, Li, and Han Citation2020). The suburban areas are seen as a ‘bridge’ connecting urban and rural areas. As a transition area between urban and rural areas, suburban areas undergo complex and dynamic demographic, landscape, and socioeconomic processes (Tian and Qian Citation2021; Liu, Shi, and Wu Citation2022). This dynamic process has significant implications for SUHII formation and diffusion. Different from urban or rural areas, suburban areas are associated with a high degree of mixed land uses, making it difficult to define their extent (Tian and Qian Citation2021; Koch et al. Citation2019; Peng et al. Citation2018). Although existing studies about SUHII have delineated simple buffer zones for suburban areas (Zhou et al. Citation2014; Yang, Huang, and Tang Citation2019), such a manner may underestimate or overestimate the SUHII for large cities and small cities (Yao et al. Citation2018; Liu et al. Citation2023), due to the heterogeneity of China's urban scale and development. Although several studies that focus on identifying suburban areas have been carried out (Tian and Qian Citation2021; Koch et al. Citation2019; Peng et al. Citation2018), they are limited to individual cities or provinces and lack accurate identification of suburban areas over a long temporal span. Examining SUHII based on suburban and urban extent not only excludes the influence of natural factors on SUHII in rural areas but also avoids the misestimation of SUHII in small and medium-sized cities caused by the extent of consistent buffer zones in the rural areas, allowing us to better understand the contribution of urbanization to temperature (Yao et al. Citation2018; Zhou et al. Citation2014; Yao et al. Citation2019; Li et al. Citation2020).
The novelties of this study include (1) calculating SUHII based on the identification of long time-series suburban regions, and (2) comparing the impacts of long time-series urban and suburban SEVs on SUHII. The general objective for this study is to quantitatively assess SUHII by creating a comprehensive long-term dataset specifically focused on suburban areas. Meanwhile, it aims to analyze and compare the similarities and disparities regarding the impacts of urban and suburban SEVs on SUHII. Based on this, we explored the impacts of long-term (2012–2021) multi-dimensional urban and suburban SEVs on daytime and nighttime SUHII. We first calculated SUHII based on long time-series urban and suburban boundaries. Then, we obtained multidimensional SEVs within the urban and suburban boundaries and explored the effect of multidimensional SEVs on SUHII in urban and suburban areas. The findings will enhance our understanding of the correlation between SEVs and SUHII, providing valuable insights to inform and guide evidence-based practices in urban planning and design.
2. Study area and data source
2.1. Study area
In this study, we selected prefecture-level cities in China as our basic research unit. We excluded cities with missing data, primarily due to the unavailability of MODIS LST and other ancillary data (e.g. statistics). In total, 201 prefecture-level cities in China were finally selected, mainly distributed in central and eastern China, where the urbanization levels and the consequent UHI are distinct (Liu et al. Citation2023; Li et al. Citation2020; Gong, Li, and Zhang Citation2019). Besides, we divided these cities into four groups (i.e. 15 megacities, 102 large cities, 55 medium-sized cities, and 29 small cities) for analysis in our study (, Table S7), according to standards from the State Council.
2.2. Data source
We adopted LST data and the China suburban extent datasets as our primary datasets for SUHII analysis and other ancillary datasets (e.g. population, statistics, nighttime light, and land use/cover). Here, we derived the LST data in 2012–2021 from the Moderate Resolution Imaging Spectroradiometer (MODIS) 8-day composite product (MOD11A2 product version 006, Terra), around 10:30 am and 10:30 pm (Yao et al. Citation2019; Si et al. Citation2022; Wan, Hook, and Hulley Citation2015). This data has good performance in characterizing LST due to its agree with in situ measured LSTs well within ±1 K in the range from 263 to 322 K (Wan et al. Citation2002). The China suburban extent data were derived in our previous study using nighttime light data (Liu, Shi, and Wu Citation2022), which contains urban and suburban boundaries over the past decade. In addition, we used other ancillary datasets to analyze the relationships between the SEVs and SUHII. We derived population density (Pop) and per capita road density (Road) data from the LandScan (Dobson et al. Citation2000) and statistic yearbook, respectively, to reflect the socioeconomic activities in cities. The nighttime data from satellites was also included in our study. Meanwhile, we calculated three landscape indexes, including patch density (PD), landscape shape index (LSI), and Splitting Index (SPLIT), respectively, using the land use/cover data (Friedl and Sulla-Menashe Citation2022). These indexes were widely used for the SUHII analysis (Guo et al. Citation2020). In addition, we used the digital elevation model (DEM) and green coverage (Green) (including forests, shrublands, Savannas, and grasslands) to characterize the nature environments, which were derived from the Shuttle Radar Topography Mission (STRM) DEM (Reuter, Nelson, and Jarvis Citation2007) and land use/cover data, respectively. Finally, we extracted building height (Height) and normalized difference built-up index (NDBI) to indicate the built-up environment, using the Chinese building height (CNBH) (Wu et al. Citation2023) and the MODIS Nadir Bidirectional Reflectance Distribution Function Adjusted Reflectance (NBAR) product (MCD43A4 V6) (Schaaf and Wang Citation2021), respectively. We calculated these variables for urban and suburban areas separately. Details about these variables and their information can be referred to in Table S1.
3. Methods
We developed the analysis framework below () to explore urban and suburban SEV’s impacts on the SUHII. First, we calculated SUHII from MODIS data using derived urban and suburban boundaries from NTL satellite observations ((a)). Second, we derived multiple SEVs from different aspects, such as the socio-economic activities, landscape indexes, natural and built-up environments ((b)). Finally, we explored urban and suburban SEV’s impacts on the daytime and nighttime SUHII, across different temporal scales (e.g. annual, seasonal, and diurnal) ((c)).
Figure 2. The proposed overall framework in our study, including the calculation of SUHII using urban and suburban boundaries (a), the derived SEVs from multiple aspects (b), and the exploration of the SEV’s impacts on SUHII (c).

3.1. The calculation of SUHII using urban and suburban boundaries
We calculated the SUHII using the temperature difference between urban and suburban areas based on LST data (see EquationEq. 1(1)
(1) ). We removed unreliable and cloud effects pixels of LST data and derived the complete monthly LST of daytime and nighttime through the 8-day LST data monthly compositions, during the whole year and specific seasons (i.e. summer (June to August) and winter (December to February)). We processed the LST data from 2012 to 2021, using the Google Earth Engine (GEE) platform. It is worthy to note that cities with substantial missing pixels mainly located in western region, which have been excluded in our study. Additionally, we only selected cities with both urban and suburban pixels when calculating the SUHII.
(1)
(1) We also derived urban and suburban boundaries from NTL datasets, spanning from 2012-2021. Satellite-derived NTL data have been widely used to characterize diverse socio-economic activities, such as population, electricity consumption, and building density (Li et al. Citation2020; Shi et al. Citation2014; Zhao et al. Citation2020; Mu et al. Citation2022; Li and Zhou Citation2017). Here, we used the NTL data to identify urban and suburban areas (Liu, Shi, and Wu Citation2022; Tian and Qian Citation2021). First, we derived rough urban and suburban boundaries from the NTL data utilizing the K-means approach ((a)). Then, we implemented a temporal consistency approach to ensure the rationality of identified urban and suburban pixels regarding their changes in consecutive years (e.g. from non-urbanized to urbanized pixels monotonously) (Li, Gong, and Liang Citation2015; Li and Gong Citation2016) ((b)). Finally, we optimized derived urban and suburban boundaries using three rules, including filling the ‘hole’, excluding independently emerged suburban areas, and removing suburban areas smaller than 2km2 ((c)). Our derived urban and suburban boundaries contained more details, in particularly, the identified suburban areas are consistent with suburban population, vegetation, and landscape characteristics (Fig. S1) (Liu, Shi, and Wu Citation2022). Details about the urban and suburban boundary can be referred to in Liu et al. (Citation2022). The derived urban and suburban boundaries can used to calculate the SUHII and analyze the urban and suburban socio-economic and environmental applications (Liu et al. Citation2023).
3.2. The derived SEVs from multiple aspects
We selected ten SEVs (i.e. socio-economic activities, landscape indexes, natural and built-up environments) to comprehensively explore the relationship between SEVs and SUHII (). These variables are frequently used in analyzing the drivers of SUHII as well as being characterized long time series, dynamics, and rich spatial information (Guo et al. Citation2020; Ming et al. Citation2023; Shao et al. Citation2023). And we averaged the different variables within the city units without changing the resolution of the original data. Socio-economic factors have a significant impact on urban climate since they directly drive urban space expansion physically (Guo et al. Citation2020). Here, we selected three socio-economic indicators, i.e. Pop, mean nighttime light (Light), and Road, to represent different impacts from socio-economic factors. Meanwhile, SUHII is also related to land cover, as well as its spatial configuration and landscape characteristics (Gage and Cooper Citation2017; Chatterjee and Majumdar Citation2022). Here, we selected PD, LSI, and SPLIT for a quantitative evaluation across cities, using the FRAGSTATS 4.3 software. Besides, natural conditions (e.g. terrain and vegetation) also contribute to the spatial distribution of LST. For instance, green space is important in mitigating the urban heat island effect through evaporation and shade provision (Liu et al. Citation2023; Guo et al. Citation2020). Thus, we selected the DEM and Green as two explanatory variables. In addition, we also included two indicators of Height and NDBI to characterize the built-up environments, both of them closely related to human activities and anthropogenic heat flux (Li et al. Citation2020; Hu, Dai, and Guldmann Citation2020; Yuan et al. Citation2023; Li et al. Citation2020).
Table 1. Description and calculation process of spatial explanatory variables in this study.
3.3. The exploration of the SEVs’ effects on SUHII
We explored the spatiotemporal trends of SUHII and SEVs for 201 cities from 2012 to 2021, using the Mann-Kendall (MK) test and Sen's slope approach (Mann Citation1945). The MK test and Sen's slope approaches were considered reliable for trend detection, which has been widely used to analyze climate and environmental variables (Blöschl et al. Citation2019; Yang, Huang, and Tang Citation2019; Siddiqui et al. Citation2021). Here, we determined the significance according to the MK test (with a threshold of 0.1), while the temporal increasing / decreasing trend was estimated using Sen’s slope approach.
Then, we explored the relationship between urban and suburban SEVs and SUHII using the partial least squares regression (PLSR) model. The PLSR model is robust and advantageous regarding its capacity to analyze both X- and Y-variables with noisy and collinear observations, or incomplete multivariate in standardized data matrices (Yao et al. Citation2022; Zhang, Li, and Han Citation2020). It performs iterative regression to estimate the principal components that represent both the X and Y variations, and the algorithm terminates when the regression equations reach satisfactory accuracy through the cross-validation. Specifically, we established the linear regression model to connect the SUHII (Dependent variable Y) and the spatial explanatory variables (Independent variable X) using the PLSR model (see EquationEq. 2(2)
(2) ).
(2)
(2) where P is the number of X; t = 1, 2, … , n is the size of observation (sample) for X and Y; βp is the regression coefficient of Xtp, indicating the relative contribution of Xtp to Y.
In addition, we measured the variable importance in the projection (VIP) of urban and suburban SEVs using PLSR model. In general, the greater VIP value is, the more significant the variable is in explaining the model, i.e. the VIP greater than one indicates that the X is significant in explaining Y (Yang et al. Citation2022; Yao et al. Citation2022). The formula for calculating VIP is as follows (see EquationEq. 3(3)
(3) ).
(3)
(3) where P is the number of independent variables; m is the number of extracted components; k is dependent variable; th is the hth component of the independent variables; R2(yk, th) is the square of the correlation between yk and th;
is the weight of the contribution of the independent variable Xj to the construction of the th component.
4. Results and discussion
4.1. Spatiotemporal trends in SUHII
The increasing trends of SUHII (i.e. annual and seasonal) were widely observed in Chinese cities due to the rapid urbanization (Liu et al. Citation2023; Liang et al. Citation2020) (, Table S8), similar to the findings of Yao et al. (Citation2019) and Yang, Huang, and Tang (Citation2019). The increase/decrease trend of SUHII was more significant at nighttime than daytime. Annual and summer SUHII was dominated by increasing trends ((a, b)), i.e. more than 50% of cities exhibit increasing trends in the nighttime, and such a trend is more evident than that in the daytime ((c)). However, the winter SUHII showed predominantly decreasing trends both in the daytime (5.97%) and nighttime (18.41%) (). The fact of urban areas experience higher temperatures at night is likely due to the absorption and storage of solar radiation by built-up surfaces and buildings, which is notably different compared to suburban areas, where built-up surfaces and buildings are sparsely distributed. This leads to an increase in the nighttime SUHII in many cities (Zhang, Murray, and Turner Ii Citation2017; Zhou et al. Citation2014). Besides, from the seasonal aspect, the SUHII was more significant in summer, whereas it is relatively consistent in winter ((a,b)), consistent with findings in Yang et al. (Citation2019). The cities with a significant increasing trend of SUHII in summer were approximately 50%, while the proportions of cities without noticeable changes of SUHII in winter were 90.05% and 79.10% in daytime and nighttime, respectively ((c)). Increasing human activities, including the use of air conditioning and traffic flow, along with relatively high solar radiation intensity and atmospheric stability, are attributable to the increase of summer SUHII (Zhou et al. Citation2014).
4.2. Spatiotemporal trends in SEVs of urban and suburban areas
In general, the SEV’s spatiotemporal trends (e.g. increasing/decreasing) were relatively consistent in urban and suburban regions, with a more pronounced trend observed in suburban areas (). In both urban and suburban areas, variables of Road, PD, LSI, and SPLIT exhibited a significant increasing trend, while Pop, Light, Height showed an opposite pattern ((a, b)). Specifically, the percentage of cities with a increase in Road, PD, LSI, and SPLIT in urban was 89.05%, 56.72%, 99% and 82.08%, respectively. A similar result was also observed in suburban areas regarding these variables ((c)). The growing economy and improved living standards are driving an increasing demand for accessibility and ecological considerations, resulting in higher per capita road area (e.g. Road) and landscape indexes (e.g. PD, LSI, and SPLIT) (Guo et al. Citation2020; Liang et al. Citation2020; Liu et al. Citation2021). The suburban areas exhibited greater increasing/decreasing trends in various SEVs compared to urban areas ((a, b)). The proportions of variables in suburban areas such as Road, PD, LSI, and SPLIT were higher than in urban areas, with increments of 4.48%, 23.38%, 1%, and 14.93%, respectively. In addition, variables of Pop, Light, and Height in suburban areas exhibited a decrease of 10.95%, 25.88%, and 8.45%, respectively, compared to that in urban areas ((c)). This phenomenon may be attributable to the comprehensive impacts of urban sprawl, suburban land use transformation, and land development, as well as environmental protection and land-use management (Foelske et al. Citation2019). For some specific indicators, their urban and suburban areas showed opposite trends. For instance, DEM in urban areas exhibited a prominent decreasing trend (50.75%). In contrast, it showed an increasing trend (63.19%) in suburban areas ((c)). Green in urban areas was relatively consistent. In comparison, suburban areas exhibited a substantial increasing trend of 83.09% ((c)). The urban areas were characterized by relatively low elevation, with extensive built-up areas for residential, commercial, and infrastructure purposes. However, the suburban areas were located at the city's periphery, with relatively lower population density, ample land resources, and distinct green coverage (Liu, Shi, and Wu Citation2022; Tian and Qian Citation2021). It was worth noting that NDBI didn’t show any significant temporal variations in urban or suburban areas ().
4.3. The VIP values of the urban and suburban spatial explanatory variables
Across different SUHII scenarios, those SEVs with relatively high (i.e. above one) VIP values were distinctly different. This finding is also demonstrated by Shao et al.(Citation2023) regarding the importance of the contribution of individual characteristics to the annual mean SUHII. Still, this characteristic remained relatively consistent across different levels of urbanization (). SEVs showed significant variability regarding their explanatory power for LST across different conditions (e.g. urban and suburban at daytime and nighttime) ((a)). PD was contributable to explain the SUHII pattern at nighttime (i.e. both in urban and suburban). In contrast, LSI showed a close relationship to the SUHII pattern during daytime ((a)). Besides, it was worth noting that those SEVs were different between daytime and nighttime, even in the same category (e.g. urban and suburban). Specifically, LSI, PD, NDBI, and PD were the key indexes that significantly influenced the variation in LST from urban daytime to suburban nighttime, with VIP values of 1.36, 2.00, 1.75, and 1.51 ((a), Table S2). Also, SEVs in different sizes cities exhibited similar patterns in terms of their explanatory ability to LST ((b)). Among them, natural environment SEVs (VIP value: 1.08) in urban daytime and landscape SEVs (VIP value: 1.26) in urban nighttime had the strongest explanatory power for LST, while landscape SEVs (VIP value: 1.13) in suburban daytime and natural environment SEVs (VIP value: 1.14) in suburban nighttime also played a significant role ((b), Table S3-6).
4.4. The effects of urban and suburban spatial explanatory variables on seasonal SUHII
The SEV’s contribution to the SUHII varies significantly under different conditions (). We used the standardized partial regression coefficients to explain the correlation of SEVs on SUHII and its importance (Zhang, Li, and Han Citation2020) (, Table S2). The PLSR model is generally significant (p-value is infinitely close to 0). When keeping other variables constant, during the daytime, urban and suburban models accounted for 14.8% to 21.3% and 15.9% to 37.3% of the variation in SUHII respectively (Table S2). However, at nighttime, urban and suburban models accounted for 2.4% to 32.9% and 5.9% to 11.9% of the variation in SUHII, respectively (Table S2). The diurnal and nocturnal SUHII varied in their trends and intensities due to changed SEVs. During the daytime, several variables, including Pop, Light, LSI, and Height, showed positive correlations with the SUHII, whereas Road, NDBI, and DEM variables showed negative correlations. Among these variables, LSI had the strongest positive correlation with SUHII, while NDBI had the strongest negative correlation. During the nighttime, SUHII increased with variations in Road, LSI, and DEM, whereas it decreased with variations in PD, SPLIT, Green, and Height. DEM showed the strongest positive correlation with SUHII, while PD showed the strongest negative correlation. Urban and suburban SEV’s impacts on the SUHII were varying (). In urban areas, there were significant positive correlations between daytime and nighttime SUHII and Pop, Light, and LSI. Conversely, we observed significant negative correlations between SUHII, PD, SPLIT, and NDBI. Among these variables, LSI showed the strongest positive correlation with SUHII, while PD had the strongest negative correlation. Only LSI showed a predominantly positive and significant correlation with daytime and nighttime SUHII in suburban areas.
4.5. Impacts of SEVs on seasonal SUHII in different city size
There was a distinct difference regarding the impacts of SEVs on SUHII, both in terms of intensity and trend (). Notably, we found that the intensity of these SEVs’ impacts weakened as city size decreased. Specifically, the average intensity of SEVs’ impacts on SUHII ranged from 0.15 in megacities to 0.09 in small cities (, Table S3-6). This observation can also be seen in the model's explanation of variations in SUHII, for example, in megacities ((a)), during the daytime and nighttime, urban and suburban models accounted for 40.7% to 61.1% and 20.1% to 57.9% of the variation in SUHII respectively (Table S3). Whereas in small cities ((d)), during the daytime and nighttime, urban and suburban models only accounted for 16.9% to 35.3% and 0% to 27.6% of the variation in SUHII (Table S6). There were varying mechanisms by which urban and suburban SEVs affect the SUHII at different scales of cities. Specifically, within urban areas, Pop and PD played a key role in driving SUHII, and their impacts were primarily positive (except for the SUHII in nighttime and megacities), while PD was predominantly negatively correlated. In suburban areas, Pop and Height had the highest contribution to SUHII. In megacities, large cities, and medium-sized cities, the effects of Pop and Height on SUHII were mainly negatively correlated, whereas in small cities, they were predominantly positively correlated. In addition, we also observed distinct differences during daytime and nighttime in explaining the SUHII at different city sizes. Specifically, Pop and Height had the strongest influence on daytime SUHII, from megacities to small cities. Pop exhibited a positive correlation, while Height showed a negative correlation (except for small cities). However, during nighttime, DEM and PD emerged as key factors impacting SUHII. DEM demonstrated a positive correlation, while PD demonstrated a negative correlation. These findings highlight the complex and varied nature of SUHII dynamics across different urban contexts. There were still some consistent patterns in the relationship between SEVs and SUHII across different city sizes. For example, the correlation coefficient matrices of large cities and medium-sized cities also exhibited a similar distribution pattern, although medium-sized cities generally displayed weaker correlations compared to large cities. Moreover, the correlation coefficient values at nighttime in small cities were relatively low.
5. Conclusions
In this study, we explored the urban and suburban SEV’s effects on SUHII in daytime and nighttime. First, we calculated SUHII based on long time-series urban and suburban boundaries and LST data. Then, we derived SEVs from four dimensions (socio-economic, landscape indexes, nature, and building environments) within urban and suburban boundaries. Finally, we explored the urban and suburban SEV’s effects on SUHII using the PLSR model. Our main findings are as follows: (1) Suburban areas showed a higher intensity of increasing/decreasing trends in various SEVs than urban areas. (2) Although SEVs showed variations in explaining LST under different conditions, this variability remained consistent across different levels of urbanization. (3) The SEV’s strongest positive/negative correlations on SUHII were found to be for LSI/NDBI (daytime), DEM/PD (nighttime), and LSI/PD (urban areas). (4) At different city sizes, although SEV’s impacts on SUHII showed significant heterogeneity, the SEV’s impacts on SUHII showed a generally consistent direction and intensity in large cities and medium-sized cities. This study provides a fresh perspective to help us understand the effect of urban development on UHI.
Based on our findings, policies aim to mitigating the UHI are recommended for future urban planning. The temperature both in urban and suburban region should be considered, rather than focusing on urban areas only (Tian and Qian Citation2021). Also, the natural landscape (e.g. green spaces and their distributions) should be considered to maximize the cooling effects in urban and suburban regions (Liu et al. Citation2023). Besides, polices should be made according to different city sizes (e.g. megacities and small cities), with considerations of future climate change and urbanization (Yuan et al. Citation2023).
Several limitations should be addressed in the future. The expanding urban and suburban areas mainly represented the temporal trends of DEM and Height due to the need for national-scale long-term time series data. Also, only four dimensions (socio-economic activities, landscape indexes, natural and built-up environments) comprising ten SEVs were selected for this study, potentially overlooking other variables in different dimensions that may impact the SUHII. Finally, our study ignored the SUHII effects by SEVs in different climatic contexts because the commonly used climate variables (e.g. ERA5) are associated with relatively coarse spatial resolutions (e.g. > 25km) (Muñoz-Sabater et al. Citation2021). In the future, we will explore more dimensional (such as climatic factors) SEV's effects on SUHII and their intrinsic influence mechanisms in a comprehensive and detailed manner by integrating different climatic context.
Supplemental Material
Download MS Word (3.2 MB)Acknowledgments
The authors would like to thank two anonymous reviewers for their constructive comments and suggestions, which greatly improved the quality of the manuscript.
Disclosure statement
No potential conflict of interest was reported by the author(s).
Data availability
The China Suburban Extent Dataset are available in National Earth System Science Data Center at http://nnu.geodata.cn/data/datadetails.html?dataguid=232582871367490&docid=1. The Moderate Resolution Imaging Spectroradiometer (MODIS) 8-day composite products (MOD11A2 product version 006, Terra) were collected at https://lpdaac.usgs.gov/products/mod11a2v006/.
Additional information
Funding
References
- Adelia, A. S., C. Yuan, L. Liu, and R. Q. Shan. 2019. “Effects of Urban Morphology on Anthropogenic Heat Dispersion in Tropical High-Density Residential Areas.” Energy and Buildings 186: 368–383. https://doi.org/10.1016/j.enbuild.2019.01.026.
- Amir Siddique, Muhammad, Yu Wang, Ninghan Xu, Nadeem Ullah, and Peng Zeng. 2021. “The Spatiotemporal Implications of Urbanization for Urban Heat Islands in Beijing: A Predictive Approach Based on CA–Markov Modeling (2004–2050).” Remote Sensing 13 (22): 4697. https://doi.org/10.3390/rs13224697.
- Blöschl, Günter, Julia Hall, Alberto Viglione, Rui AP Perdigão, Juraj Parajka, Bruno Merz, David Lun, Berit Arheimer, Giuseppe T Aronica, and Ardian Bilibashi. 2019. “Changing Climate Both Increases and Decreases European River Floods.” Nature 573 (7772): 108–111. https://doi.org/10.1038/s41586-019-1495-6.
- Chatterjee, Uday, and Sushobhan Majumdar. 2022. “Impact of Land Use Change and Rapid Urbanization on Urban Heat Island in Kolkata City: A Remote Sensing Based Perspective.” Journal of Urban Management 11 (1): 59–71. https://doi.org/10.1016/j.jum.2021.09.002.
- Dobson, Jerome E, Edward A Bright, Phillip R Coleman, Richard C Durfee, and Brian A Worley. 2000. “LandScan: A Global Population Database for Estimating Populations at Risk.” Photogrammetric Engineering and Remote Sensing 66 (7): 849–857.
- Foelske, Lorraine, Carena J Van Riper, William Stewart, Amy Ando, Paul Gobster, and Len Hunt. 2019. “Assessing Preferences for Growth on the Rural-Urban Fringe Using a Stated Choice Analysis.” Landscape and Urban Planning 189: 396–407. https://doi.org/10.1016/j.landurbplan.2019.05.016.
- Friedl, M., and D. Sulla-Menashe. 2022. “MODIS/Terra + Aqua Land Cover Type Yearly L3 Global 500m SIN Grid V061.” In, edited by NASA EOSDIS Land Processes Distributed Active Archive Center.
- Gage, Edward A, and David J Cooper. 2017. “Relationships Between Landscape Pattern Metrics, Vertical Structure and Surface Urban Heat Island Formation in a Colorado Suburb.” Urban Ecosystems 20: 1229–1238. https://doi.org/10.1007/s11252-017-0675-0.
- Geng, Xiaolei, Dou Zhang, Chengwei Li, Yuan Yuan, Zhaowu Yu, and Xiangrong Wang. 2023. “Impacts of Climatic Zones on Urban Heat Island: Spatiotemporal Variations, Trends, and Drivers in China from 2001–2020.” Sustainable Cities and Society 89: 104303. https://doi.org/10.1016/j.scs.2022.104303.
- Gong, Peng, Xuecao Li, and Wei Zhang. 2019. “40-Year (1978–2017) Human Settlement Changes in China Reflected by Impervious Surfaces from Satellite Remote Sensing.” Science Bulletin 64 (11): 756–763. https://doi.org/10.1016/j.scib.2019.04.024.
- Guo, Andong, Jun Yang, Xiangming Xiao, Jianhong Xia, Cui Jin, and Xueming Li. 2020. “Influences of Urban Spatial Form on Urban Heat Island Effects at the Community Level in China.” Sustainable Cities and Society 53: 101972. https://doi.org/10.1016/j.scs.2019.101972.
- He, Bao-Jie, Wei Wang, Ayyoob Sharifi, and Xiao Liu. 2023. “Progress, Knowledge Gap and Future Directions of Urban Heat Mitigation and Adaptation Research Through a Bibliometric Review of History and Evolution.” Energy and Buildings: 112976.
- Hsu, Angel, Glenn Sheriff, Tirthankar Chakraborty, and Diego Manya. 2021. “Disproportionate Exposure to Urban Heat Island Intensity Across Major US Cities.” Nature Communications 12 (1): 2721. https://doi.org/10.1038/s41467-021-22799-5.
- Hu, Yunfeng, Zhaoxin Dai, and Jean-Michel Guldmann. 2020. “Modeling the Impact of 2D/3D Urban Indicators on the Urban Heat Island Over Different Seasons: A Boosted Regression Tree Approach.” Journal of Environmental Management 266: 110424.
- Koch, Jennifer, Monica A Dorning, Derek B Van Berkel, Scott M Beck, Georgina M Sanchez, Ashwin Shashidharan, Lindsey S Smart, Qiang Zhang, Jordan W Smith, and Ross K Meentemeyer. 2019. “Modeling Landowner Interactions and Development Patterns at the Urban Fringe.” Landscape and Urban Planning 182: 101–113. https://doi.org/10.1016/j.landurbplan.2018.09.023.
- Li, Xuecao, and Peng Gong. 2016. “An “Exclusion-Inclusion” Framework for Extracting Human Settlements in Rapidly Developing Regions of China from Landsat Images.” Remote Sensing of Environment 186: 286–296. https://doi.org/10.1016/j.rse.2016.08.029.
- Li, Xuecao, Peng Gong, and Lu Liang. 2015. “A 30-Year (1984–2013) Record of Annual Urban Dynamics of Beijing City Derived from Landsat Data.” Remote Sensing of Environment 166: 78–90. https://doi.org/10.1016/j.rse.2015.06.007.
- Li, Xuecao, Peng Gong, Yuyu Zhou, Jie Wang, Yuqi Bai, Bin Chen, Tengyun Hu, Yixiong Xiao, Bing Xu, and Jun Yang. 2020. “Mapping Global Urban Boundaries from the Global Artificial Impervious Area (GAIA) Data.” Environmental Research Letters 15 (9): 094044. https://doi.org/10.1088/1748-9326/ab9be3.
- Li, Xuecao, Xiaoping Liu, and Le Yu. 2014. “A Systematic Sensitivity Analysis of Constrained Cellular Automata Model for Urban Growth Simulation Based on Different Transition Rules.” International Journal of Geographical Information Science 28 (7): 1317–1335. https://doi.org/10.1080/13658816.2014.883079.
- Li, Yunfei, Sebastian Schubert, Jürgen P Kropp, and Diego Rybski. 2020. “On the Influence of Density and Morphology on the Urban Heat Island Intensity.” Nature Communications 11 (1): 2647. https://doi.org/10.1038/s41467-020-16461-9.
- Li, Xuecao, and Yuyu Zhou. 2017. “Urban Mapping Using DMSP/OLS Stable Night-Time Light: A Review.” International Journal of Remote Sensing 38 (21): 6030–6046. https://doi.org/10.1080/01431161.2016.1274451.
- Li, Xuecao, Yuyu Zhou, Peng Gong, Karen C Seto, and Nicholas Clinton. 2020. “Developing a Method to Estimate Building Height from Sentinel-1 Data.” Remote Sensing of Environment 240: 111705. https://doi.org/10.1016/j.rse.2020.111705.
- Li, Xuecao, Yuyu Zhou, Min Zhao, and Xia Zhao. 2020. “A Harmonized Global Nighttime Light Dataset 1992–2018.” Scientific Data 7 (1): 168. https://doi.org/10.1038/s41597-020-0510-y.
- Liang, Ze, Shuyao Wu, Yueyao Wang, Feili Wei, Jiao Huang, Jiashu Shen, and Shuangcheng Li. 2020. “The Relationship Between Urban Form and Heat Island Intensity Along the Urban Development Gradients.” Science of the Total Environment 708: 135011. https://doi.org/10.1016/j.scitotenv.2019.135011.
- Liu, Huimin, Bo Huang, Qingming Zhan, Sihang Gao, Rongrong Li, and Zhiyu Fan. 2021. “The Influence of Urban Form on Surface Urban Heat Island and its Planning Implications: Evidence from 1288 Urban Clusters in China.” Sustainable Cities and Society 71: 102987. https://doi.org/10.1016/j.scs.2021.102987.
- Liu, Xue, Yujia Ming, Yong Liu, Wenze Yue, and Guifeng Han. 2022. “Influences of Landform and Urban Form Factors on Urban Heat Island: Comparative Case Study Between Chengdu and Chongqing.” Science of the Total Environment 820: 153395. https://doi.org/10.1016/j.scitotenv.2022.153395.
- Liu, Shirao, Kaifang Shi, and Yizhen Wu. 2022. “Identifying and Evaluating Suburbs in China from 2012 to 2020 Based on SNPP–VIIRS Nighttime Light Remotely Sensed Data.” International Journal of Applied Earth Observation and Geoinformation 114: 103041. https://doi.org/10.1016/j.jag.2022.103041.
- Liu, Shirao, Kaifang Shi, Yizhen Wu, and Yuanzheng Cui. 2023. “Suburban Greening and Suburbanization Changing Surface Urban Heat Island Intensity in China.” Building and Environment 228: 109906. https://doi.org/10.1016/j.buildenv.2022.109906.
- Macintyre, Helen L, Clare Heaviside, Xiaoming Cai, and Revati Phalkey. 2021. “The Winter Urban Heat Island: Impacts on Cold-Related Mortality in a Highly Urbanized European Region for Present and Future Climate.” Environment International 154: 106530. https://doi.org/10.1016/j.envint.2021.106530.
- Mann, Henry B. 1945. “Nonparametric Tests Against Trend.” Econometrica: Journal of the Econometric Society, 245–259.
- Ming, Yujia, Yong Liu, Jianping Gu, Jinzhu Wang, and Xue Liu. 2023. “Nonlinear Effects of Urban and Industrial Forms on Surface Urban Heat Island: Evidence from 162 Chinese Prefecture-Level Cities.” Sustainable Cities and Society 89: 104350. https://doi.org/10.1016/j.scs.2022.104350.
- Moazzam, Muhammad Farhan Ul, Yang Hoi Doh, and Byung Gul Lee. 2022. “Impact of Urbanization on Land Surface Temperature and Surface Urban Heat Island Using Optical Remote Sensing Data: A Case Study of Jeju Island, Republic of Korea.” Building and Environment 222: 109368. https://doi.org/10.1016/j.buildenv.2022.109368.
- Mu, Haowei, Xuecao Li, Haijiao Ma, Xiaoping Du, Jianxi Huang, Wei Su, Zhen Yu, Chen Xu, Hualiang Liu, and Dongqin Yin. 2022. “Evaluation of the Policy-Driven Ecological Network in the Three-North Shelterbelt Region of China.” Landscape and Urban Planning 218: 104305. https://doi.org/10.1016/j.landurbplan.2021.104305.
- Mu, Haowei, Xuecao Li, Yanan Wen, Jianxi Huang, Peijun Du, Wei Su, Shuangxi Miao, and Mengqing Geng. 2022. “A Global Record of Annual Terrestrial Human Footprint Dataset from 2000 to 2018.” Scientific Data 9 (1): 176. https://doi.org/10.1038/s41597-022-01284-8.
- Muñoz-Sabater, Joaquín, Emanuel Dutra, Anna Agustí-Panareda, Clément Albergel, Gabriele Arduini, Gianpaolo Balsamo, Souhail Boussetta, Margarita Choulga, Shaun Harrigan, and Hans Hersbach. 2021. “ERA5-Land: A State-of-the-art Global Reanalysis Dataset for Land Applications.” Earth System Science Data 13 (9): 4349–4383. https://doi.org/10.5194/essd-13-4349-2021.
- Peng, Jian, Yanxu Liu, Jing Ma, and Shiquan Zhao. 2018. “A new Approach for Urban-Rural Fringe Identification: Integrating Impervious Surface Area and Spatial Continuous Wavelet Transform.” Landscape and Urban Planning 175: 72–79. https://doi.org/10.1016/j.landurbplan.2018.03.008.
- Ramírez-Aguilar, Edwin Alejandro, and Léa Cristina Lucas Souza. 2019. “Urban Form and Population Density: Influences on Urban Heat Island Intensities in Bogotá, Colombia.” Urban Climate 29: 100497. https://doi.org/10.1016/j.uclim.2019.100497.
- Reuter, Hannes Isaak, Andy Nelson, and Andrew Jarvis. 2007. “An Evaluation of Void-Filling Interpolation Methods for SRTM Data.” International Journal of Geographical Information Science 21 (9): 983–1008. https://doi.org/10.1080/13658810601169899.
- Schaaf, C., and Z. Wang. 2021. “MODIS/Terra + Aqua BRDF/Albedo Nadir BRDF Adjusted Ref Daily L3 Global - 500m V061.” In, edited by NASA EOSDIS Land Processes Distributed Active Archive Center.
- Shao, Ledi, Weilin Liao, Peilin Li, Ming Luo, Xuehui Xiong, and Xiaoping Liu. 2023. “Drivers of Global Surface Urban Heat Islands: Surface Property, Climate Background, and 2D/3D Urban Morphologies.” Building and Environment, 110581.
- Shi, Zitong, Xuecao Li, Tengyun Hu, Bo Yuan, Peiyi Yin, and Dabang Jiang. 2023. “Modeling the Intensity of Surface Urban Heat Island Based on the Impervious Surface Area.” Urban Climate 49: 101529. https://doi.org/10.1016/j.uclim.2023.101529.
- Shi, Kaifang, Bailang Yu, Yixiu Huang, Yingjie Hu, Bing Yin, Zuoqi Chen, Liujia Chen, and Jianping Wu. 2014. “Evaluating the Ability of NPP-VIIRS Nighttime Light Data to Estimate the Gross Domestic Product and the Electric Power Consumption of China at Multiple Scales: A Comparison with DMSP-OLS Data.” Remote Sensing 6 (2): 1705–1724. https://doi.org/10.3390/rs6021705.
- Si, Menglin, Zhao-Liang Li, Françoise Nerry, Bo-Hui Tang, Pei Leng, Hua Wu, Xia Zhang, and Guofei Shang. 2022. “Spatiotemporal Pattern and Long-Term Trend of Global Surface Urban Heat Islands Characterized by Dynamic Urban-Extent Method and MODIS Data.” ISPRS Journal of Photogrammetry and Remote Sensing 183: 321–335. https://doi.org/10.1016/j.isprsjprs.2021.11.017.
- Siddiqui, Asfa, Gautami Kushwaha, Bhaskar Nikam, S. K. Srivastav, Ankita Shelar, and Pramod Kumar. 2021. “Analysing the day/Night Seasonal and Annual Changes and Trends in Land Surface Temperature and Surface Urban Heat Island Intensity (SUHII) for Indian Cities.” Sustainable Cities and Society 75: 103374. https://doi.org/10.1016/j.scs.2021.103374.
- Tian, Yasi, and Jing Qian. 2021. “Suburban Identification Based on Multi-Source Data and Landscape Analysis of its Construction Land: A Case Study of Jiangsu Province, China.” Habitat International 118: 102459. https://doi.org/10.1016/j.habitatint.2021.102459.
- Wan, Zhengming, Simon Hook, and Glynn Hulley. 2015. “MOD11A2 MODIS/Terra Land Surface Temperature.” Emissivity Daily L3 Global 1km SIN Grid 6.
- Wan, Zhengming, Yulin Zhang, Qincheng Zhang, and Zhao-liang Li. 2002. “Validation of the Land-Surface Temperature Products Retrieved from Terra Moderate Resolution Imaging Spectroradiometer Data.” Remote Sensing of Environment 83 (1-2): 163–180. https://doi.org/10.1016/S0034-4257(02)00093-7.
- Wang, Litao, Shixin Wang, Yi Zhou, Wenliang Liu, Yanfang Hou, Jinfeng Zhu, and Futao Wang. 2018. “Mapping Population Density in China Between 1990 and 2010 Using Remote Sensing.” Remote Sensing of Environment 210: 269–281. https://doi.org/10.1016/j.rse.2018.03.007.
- Wu, Wan-Ben, Jun Ma, Ellen Banzhaf, Michael E Meadows, Zhao-Wu Yu, Feng-Xiang Guo, Dhritiraj Sengupta, Xing-Xing Cai, and Bin Zhao. 2023. “A First Chinese Building Height Estimate at 10 m Resolution (CNBH-10 m) Using Multi-Source Earth Observations and Machine Learning.” Remote Sensing of Environment 291: 113578. https://doi.org/10.1016/j.rse.2023.113578.
- Xu, Gang, Muchen Zheng, Yaxing Wang, and Jing Li. 2022. “The Urbanization of Population and Land in China: Temporal Trends, Regional Disparities, Size Effect and Comparisons of Measurements.” China Land Science 36 (05): 80–90.
- Yang, Qiquan, Xin Huang, and Qiuhong Tang. 2019. “The Footprint of Urban Heat Island Effect in 302 Chinese Cities: Temporal Trends and Associated Factors.” Science of the Total Environment 655: 652–662. https://doi.org/10.1016/j.scitotenv.2018.11.171.
- Yang, Qi, Shuheng Li, Jiahao Li, Jianfeng Du, and Jiachuan Wang. 2022. “Response of Phenology Phases of Four Deciduous Trees to Climate Change in Xi'an.” Acta Ecologica Sinica 42 (04): 1462–1473. https://doi.org/10.5846/stxb202011212992.
- Yao, Lei, Shuo Sun, Chaoxue Song, Yixu Wang, and Ying Xu. 2022. “Recognizing Surface Urban Heat ‘Island’ Effect and its Urbanization Association in Terms of Intensity, Footprint, and Capacity: A Case Study with Multi-Dimensional Analysis in Northern China.” Journal of Cleaner Production 372: 133720. https://doi.org/10.1016/j.jclepro.2022.133720.
- Yao, Rui, Lunche Wang, Xin Huang, Wei Gong, and Xiangao Xia. 2019. “Greening in Rural Areas Increases the Surface Urban Heat Island Intensity.” Geophysical Research Letters 46 (4): 2204–2212. https://doi.org/10.1029/2018GL081816.
- Yao, Rui, Lunche Wang, Xin Huang, Ying Niu, Yisen Chen, and Zigeng Niu. 2018. “The Influence of Different Data and Method on Estimating the Surface Urban Heat Island Intensity.” Ecological Indicators 89: 45–55. https://doi.org/10.1016/j.ecolind.2018.01.044.
- Yin, Chaohui, Man Yuan, Youpeng Lu, Yaping Huang, and Yanfang Liu. 2018. “Effects of Urban Form on the Urban Heat Island Effect Based on Spatial Regression Model.” Science of the Total Environment 634: 696–704. https://doi.org/10.1016/j.scitotenv.2018.03.350.
- Yuan, Bo, Xuecao Li, Liang Zhou, Tiecheng Bai, Tengyun Hu, Jianxi Huang, Dongjie Liu, Yangchun Li, and Jincheng Guo. 2023. “Global Distinct Variations of Surface Urban Heat Islands in Inter- and Intra-Cities Revealed by Local Climate Zones and Seamless Daily Land Surface Temperature Data.” ISPRS Journal of Photogrammetry and Remote Sensing 204: 1–14. https://doi.org/10.1016/j.isprsjprs.2023.08.012.
- Zhang, Hao, Tian-Tian Li, and Jie-Jie Han. 2020. “Quantifying the Relationship Between Land use Features and Intra-Surface Urban Heat Island Effect: Study on Downtown Shanghai.” Applied Geography 125: 102305. https://doi.org/10.1016/j.apgeog.2020.102305.
- Zhang, Yujia, Alan T Murray, and B. L. Turner Ii. 2017. “Optimizing Green Space Locations to Reduce Daytime and Nighttime Urban Heat Island Effects in Phoenix, Arizona.” Landscape and Urban Planning 165: 162–171. https://doi.org/10.1016/j.landurbplan.2017.04.009.
- Zhao, Min, Yuyu Zhou, Xuecao Li, Weiming Cheng, Chenghu Zhou, Ting Ma, Manchun Li, and Kun Huang. 2020. “Mapping Urban Dynamics (1992–2018) in Southeast Asia Using Consistent Nighttime Light Data from DMSP and VIIRS.” Remote Sensing of Environment 248: 111980. https://doi.org/10.1016/j.rse.2020.111980.
- Zhou, Decheng, Shuqing Zhao, Shuguang Liu, Liangxia Zhang, and Chao Zhu. 2014. “Surface Urban Heat Island in China's 32 Major Cities: Spatial Patterns and Drivers.” Remote Sensing of Environment 152: 51–61. https://doi.org/10.1016/j.rse.2014.05.017.
- Zhou, Yi, Haile Zhao, Sicheng Mao, Guoliang Zhang, Yulin Jin, Yuchao Luo, Wei Huo, Zhihua Pan, Pingli An, and Fei Lun. 2022. “Exploring Surface Urban Heat Island (SUHI) Intensity and its Implications Based on Urban 3D Neighborhood Metrics: An Investigation of 57 Chinese Cities.” Science of the Total Environment 847: 157662. https://doi.org/10.1016/j.scitotenv.2022.157662.