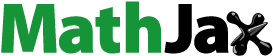
ABSTRACT
The China-Pakistan Transportation Corridor (CPTC) has long faced great challenges in natural disasters and sustainable development. This study develops a localised indicator evaluation system for the CPTC sustainable development. It contains 27 Tier I and 8 Tier II indicators, covering 11 sustainable development goals. Based on this evaluation system, a single-goal, multidimensional, and comprehensive evaluation of the CPTC sustainable development level is conducted for 2015, 2017 and 2020. The results show that, in terms of single goals, Xinjiang has the highest sustainable development level. Khyber Pakhtunkhwa has the poorest level of sustainable development. The Islamabad Capital Territory is better developed for all goals, especially in SDG5, SDG8 and SDG9. Besides these three goals, SDG6, and SDG11 are more prominent in Punjab, whereas SDG2 and SDG3 performed worst. From a multidimensional evaluation, the CPTC sustainable development level has been steadily increasing. However, the social and environmental dimensions of Khyber Pakhtunkhwa have experienced a ‘regression’. The comprehensive evaluation results show that the level of sustainable development in all provinces of the CPTC increase, except for Khyber Pakhtunkhwa. This study identifies CPTC sustainability issues and proposes targeted recommendations for sustainable development.
1. Introduction
Since the twenty-first century, with rapid industrialisation and the expansion of the scope of human activities(Yang et al. Citation2022; Yu and Luo Citation2019), the economy has developed rapidly, which has led to an imbalance between society, economy and environment. To address this issue, in September 2015, the United Nations released ‘Transforming Our World: the 2030 Agenda for Sustainable Development’ based on the Millennium Development Plan. The agenda proposed 17 Sustainable Development Goals (SDGs) with 169 targets and 231 indicators (Ashizawa, Sudo, and Yamamoto Citation2022). The UN Global Indicators Framework for Sustainable Development (GIFSD) offers a framework for evaluating sustainable development (Diaz-Sarachaga, Jato-Espino, and Castro-Fresno Citation2018; United Nations Citation2015). Many countries monitor changes in national SDGs through voluntary national review reports submitted to the UN Political Forum on Sustainable Development (Allen et al. Citation2019). However, issues such as inadequate evaluation systems and lack of data on evaluation indicators, seriously affect evaluations across different regions (Guo et al. Citation2021, Citation2022).
The China-Pakistan Transportation Corridor (CPTC), situated at the confluence of the Himalayas, Karakorum, and the Hindu Kush Mountains in the high Asian region, faces significant challenges in green development. These challenges stem from the region's underdeveloped economy, complex climate (Shelton and Dixon Citation2023), and frequent natural disasters (Ali et al. Citation2019; Chang et al. Citation2021). With the further implementation of the Belt and Road Initiative (BRI) in recent years, what changes have occurred in the CPTC regarding the economy, environment, agriculture, urbanisation (Jiang et al. Citation2022), disaster prevention, and mitigation? How well does the CPTC implement SDGs? Will the 17 SDGs be achieved as expected? These issues are urgent concerns worldwide.
To address the challenges of regional evaluation, the current focus of SDGs evaluation is transitioning from top-level theoretical research to specific quantitative research that presents a variety of evaluation methods. Several studies have started from the single goal of SDGs (Boeren Citation2019; Campbell et al. Citation2018; Ferguson and Roofe Citation2020; Sadoff, Borgomeo, and Uhlenbrook Citation2020) and evaluated them from different dimensions and spatial scales. However, since the achievement of SDGs depends on natural and social systems characterised by complexity, diversity, dynamics, and interconnection (Wu et al. Citation2022), the adoption of an approach based on single-goal evaluation cuts off their holistic and systematic nature, lacks consideration of the relationships among indicators, and fails to form a comprehensive understanding of regional sustainable development level. Comprehensive evaluation is currently a hot topic in the evaluation of sustainable development. Allen et al. (Citation2020) combined GIFSD to form a new dashboard evaluation method for comprehensively evaluating the progress of 144 indicators corresponding to 86 development sub-goals in Australia. McArthur and Rasmussen (Citation2019) proposed a normalisation method to diagnose the inherent diversity of indicators and evaluate the human impact of failing to meet the SDGs.
However, there are some significant problems with the current evaluation of sustainable development. First, special regions were not evaluated. Most of the current SDGs have been assessed at both national and global levels, and there is a lack of evaluation studies for extreme environmental and transboundary regions such as the CPTC. Because these areas span different countries, it is more difficult to evaluate sustainability using uniform indicators. Second, there is a lack of research that aims to capture the spatial and temporal dynamics of the SDG indicators (Giupponi and Gain Citation2017). Sustainable development evaluations considering different spatial (Kussul et al. Citation2020) and temporal dimensions can provide a more comprehensive understanding of the sustainable development process in the study area and better decision support for local development.
To address the aforementioned issues and further understand the sustainable development process of the CPTC since the initiation of the SDGs, this study uses data from 2015 (the start year of SDG implementation), 2020 (the current status with ready data support and the end year for some indicators such as target 3.6: By 2020, halve the number of global deaths and injuries from road traffic accidents), and 2017 (the middle year between 2015 and 2020 to gain a better grasp on SDG implementation trends). The CPTC is selected as the research area. We apply statistical and spatial data to formulate a localised sustainable development indicator system for the CPTC. Based on this evaluation system, a single-goal, multidimensional and comprehensive evaluations of the CPTC sustainable development level are conducted in these specified years. First of all, we combined multiple data sources, including big earth data, to standardise the inconsistent scale of data into unified sustainable development indicators. This addressed the CPTC issue of 8 Tier II (no data). On the other hand, we discovered the lagging development in the central part of the CPTC and imbalanced social, economic and environmental development issues. Consequently, a serial of targeted sustainable development suggestions for CPTC were proposed.
2. Materials and methods
2.1. Study area
The study focuses on administrative and geographical units along the China-Pakistan Transportation Corridor (CPTC)'s Karakorum Highway (KKH). The study area encompasses a buffer zone extending 100 km on either side of the KKH, covering a vast area of 281,340,000 km2. The geographical coordinates range from 40°46′13.75″N to 32°59′20.79″N, and from 71°14′9.81″E to 78°36′37.1″E (). The highway straddles China and Pakistan, starting from Kashgar City in the Xinjiang Uyghur Autonomous Region of China in the north, passing through the Khunjerab boarder port at the China-Pakistan border crossing, and ending in Takot in Pakistan in the south. The total length of the highway is 1224 km, accounting for one-third of the total length of highways in China and two-thirds in Pakistan. This transportation corridor offers great convenience for transportation and trade between China and Pakistan as well as with neighbouring countries such as Iran and Afghanistan.
Figure 1. Overview of the study area.
Note: XJ, Xinjiang Uyghur Autonomous Region; FCT, Islamabad Capital Territory; NWFP, Khyber Pakhtunkhwa; AK, Azad Kashmir; P, Punjab; NA, Gilgit-Baltistan; FATA, Federally Administered Tribal Area.

CPTC is one of the most treacherous and undulating areas in the world. Crossing three major extreme high mountain ranges, namely the Karakorum Mountains, the Hindu Kush Mountains and the western end of the Himalayas, the altitude is generally higher than 4000 m, and it is characterised by alpine climate. Precipitation and temperature are greatly influenced by latitude, with an annual average temperature of 16°C. Predominantly, this area is arid or semi-arid, with an average annual precipitation that less than 250 mm. Winter in this climate zone is long and cold, often windy and snowy. Summer is cool but short, the terrain is precarious, and prone to geological disasters such as landslides, mudslides and glacial lake outbursts.
2.2. Data source and pre-processing
2.2.1. Data source
The data used in this study were divided into two main parts: (1) statistical data with spatial attributes, and (2) remote sensing monitoring data, including land cover, night-time lights, and other geodata. These data sources are listed in and , respectively.
Table 1. Geospatial and statistics data used for the study.
Table 2. Remote sensing data sources used in the study.
2.2.2. Data pre-processing
2.2.2.1. Human activity intensity of the VANUI value construction
Humans are the main factors in sustainable development and the most subjective and dynamic factor in the pursuit of the SDGs. NPP/VIIRS nighttime light data, Normalised Difference Vegetation Index (NDVI), Moderate Normal Different Water Index (MNDWI), and Normalised Difference Built-Up Index (NDBI) data were used to obtain the nighttime light adjustment index Variable of Anthropogenic Nighttime Urban Intensity (VANUI), which was used to characterise the intensity of human activities (Chu et al. Citation2020).
VANUI was derived from adjusting the DN value of the nighttime light data (Zhang, Schaaf, and Seto Citation2013). This adjustment was performed by applying the indices such as NDVI, MNDWI, and NDBI collectively to establish an adjustment coefficient for DN:. It served the purpose of mitigating the impact of vegetation, water bodies, and other factors on the NPP/VIIRS nighttime light data. The human-activity intensity adjustment index VANUI was obtained as follows:
(1)
(1) where
denotes Human activity intensity,
denotes the normalised nighttime light data,
,
,
denote the maximum
value and minimum
value in the nighttime light data .
2.2.2.2. Geographic data spatialisation
Compared with statistical indicators, geospatial indicators can provide a full coverage and more detailed insights at specific geographical locations. Therefore, this study used land cover, nighttime light, and other geospatial data to spatially grid indicators such as population, GDP, road density, and water system density along the CPTC (). There were three main steps for obtaining geospatial gridded data: extracting and calculating land cover data, analysing vector data changes, and clipping existed geospatial data. Specifically, extracting and calculating land cover data involved acquiring the required land cover based on specific classifications, and reclassifying them to form corresponding geospatial indicator data. Vector data change calculation involved setting up establishing a grid based on acquired vector data (e.g. roads and water systems), and performing analyses to obtain corresponding geospatial indicator data. Clipping existed geospatial data was the last step of the data set ready for evaluation.
2.2.2.3. Xinjiang and Pakistan statistical data spatialisation
The statistical data for each province of Pakistan in 2015, 2017 and 2020 were screened from the World Data Bank. The statistics of Xinjiang were obtained from the China Xinjiang Statistical Yearbook. All the selected screened statistics were localised against the SDG indicator framework and assigned to each provincial administrative unit in the study area to spatially represent the regional statistics.
2.3. Methods
Quantitative research, approached from various evaluative perspectives and directions, can push a comprehensive understanding of the CPTC sustainable development level. The methodology of this study involved two main steps: section 2.3.1 Indicators system construction, including (1) formation of localised sustainable development indicators based on the characteristics of the CPTC. (2) Harmonisation all indicators so that they can be aggregated to form sustainable development indicators system; section 2.3.2 Sustainable development evaluation, including (1) The benchmarking of selected indicators against SDGs for single goal evaluation of sustainable development. (2) Evaluation conducted across social, economic, and environmental dimensions, based on established goals. And finally, (3) a comprehensive evaluation carried out by considering the scores and weights of each indicator. The specific methodology is illustrated in .
2.3.1. Indicators system construction
2.3.1.1. Indicators localisation
Localisation emphasises the need for foreign elements to be adapted to the new environments in order to meet specific local requirements (Kramer and MacKinnon Citation1993). The process of localising sustainable development indicators (Patole Citation2018) requires the screening and improvement of indicators based on the natural terrain, population characteristics, and socioeconomic factors. This leads to the indicators that are unique to the local context. The CPTC, which spans China and Pakistan, is marked by significant variations in terrain, a small population size, and frequent natural disasters. Consequently, the localisation of indicators is essential to establish a sustainable development indicator system for CPTC.
This study aims to construct a localised sustainable development evaluation system for CPTC. The selection of indicators adhered to the principles of objectivity, accessibility, and rationality, in accordance with the UN SDGs evaluation requirements. Specific localisation methods included the following aspects: (1) The CPTC is characterised by large terrain undulations, uneven population distribution, and difficulties in acquiring statistical data, so spatialised indicators (such as land restoration and degradation) that can more easily reflect the actual state of sustainable development are mainly selected; (2) The CPTC is sparsely inhabited and concentrated, with an aggregated distribution of various land-use types, so remote sensing data (such as land-use data) are more capable of highlight the sustainable development level of the CPTC; (3) The CPTC is prone to natural disasters due to its topography and climate, so localised indicators for disasters are also formed; (4) The CPTC has undergone significant changes in recent years as a result of the BRI, so more resource environment and socio-economic indicators are selected to analyse the their changes.
2.3.1.2. Indicators harmonisation
Due to the diverse nature and origin of localised indicators, it is necessary to harmonise and aggregate them for better assessing sustainable development. This study mainly completed the harmonisation and aggregation of indicators through the following three steps. Firstly, we improved most indicators by converting them into dimensionless data such as percentages or ratios. Secondly, we had standardised all indicators (0–100) to make them dimensionless and facilitate their comparison. Specific standardisation methods were as in Equations (2) and (3), and the positive and negative directions of the specific indicators were shown in the properties of . Lastly, we spatialised all indicator values, including geospatial and statistical data, by gridding them and resampling them into uniform grid data with a spatial resolution of 100 m, making it easier to aggregate and calculate.
(2)
(2)
(3)
(3) where
denotes the score of each indicator,
denotes the raw data of the indicator,
and
are the maximum and minimum values of the relative levels in the data obtained for the indicator, respectively. Specific scores for each sustainability indicator were obtained by normalisation.
Table 3. Sustainable development indicator system for CPTC.
2.3.2. Sustainable development evaluation
Accurate and quantitative evaluations of sustainable development can serve as a foundational reference for future regional development decisions. A singular approach to such assessment does not yield a holistic understanding of CPTC (Hutton and Chase Citation2016). This research examined the sustainable development of CPTC across three key dimensions: single goal evaluation, socio-economic-environmental evaluation, and comprehensive evaluation. The objective is to provide a comprehensive insight into CPTC's sustainable development trajectory, while also pinpointing areas requiring improvement and identifying potential shortcomings.
2.3.2.1. Single goal evaluation
The scores of each SDG were further calculated based on the standardised score of each indicator obtained in the previous calculation. Considering that each indicator is equally important for its corresponding goal, an equal-weighted average was calculated for each indicator, which was expressed as the ratio of the sum of the scores of each indicator to the number of indicators:
(4)
(4) where
denotes the score of
-th goal,
denotes the score of each indicator corresponding to indicator
,
denotes the number of indicators corresponding to the goal.
2.3.2.2. Socio-economic-environmental multidimensional evaluation
The equal-weighted average method was used to calculate the development of the Socio-economic-environmental multidimensional. Because the principle that equal importance should be given to the economic, social, and environmental dimensions for each sustainable development goal. The overall performance of each province was calculated across these dimensions as follows:
(5)
(5) where
denote the dimensions of economy, society, and environment respectively,
denotes the goal score corresponding to goal
,
is the number of goals contributing to the dimension.
2.3.2.3. Comprehensive evaluation
The entropy method was used to determine the weight of each indicator at the comprehensive sustainable development level. Currently, a large number of sustainable development evaluations used principal component analysis (Dos Santos et al. Citation2019; Kaymaz, Birinci, and Kızılkan Citation2022) to determine weights. However, this method is highly subjective, whereas the entropy method is a more objective method for determining weights through the degree of variability of statistical data. The information carried by the entropy value was used to calculate the weight of each sustainable development indicator, which was then used for comprehensive sustainable development evaluation.
Assuming that there are indicators for an objective in the sustainable development system of the study area, the information entropy value
for the
indicator is calculated as:
(6)
(6) where m denotes the total number of levels for the
-th indicator,
is the proportion of the j-th indicator's
-th level to the total number of observations. The information entropy value reflects the degree of uncertainty or randomness in the distribution of the indicator levels. Higher entropy values indicate greater uncertainty, whereas lower value indicates greater predictability. The information utility values
is:
(7)
(7) the larger the information utility value, the more important the indicator, and the greater the influence on the evaluation results. Thus, the weight of the
-th indicator can be obtained using the following equation:
(8)
(8) The standardised indicator data were multiplied and summed with the weights calculated using the entropy method to obtain the comprehensive score of sustainable development indicators for the CPTC.
(9)
(9) where
is the final calculated composite sustainability score;
is the score for the
-th indicator,
is the calculated weight for each objective;
is the total number of indicators.
3. Results
3.1. Sustainable development indicators system
We modified and screened the UN SDG Indicators framework to form a CPTC localised indicator system (). The indicators selection criteria mainly include rationality (whether the selected indicators are suitable for CPTC region), objectivity (whether the acquired data can objectively reflect the corresponding indicators), and accessibility (whether the selected indicators can be obtained through statistical data and geospatial data).
The system consists of three levels: (1) Indicator level, consisting of a total of 35 indicators, with 27 Tier I indicators (with available methodology and data) and 8 Tier II indicators (with available methodology but no data); (2) Goal level, including SDG1, SDG2, SDG3, SDG4, SDG5, SDG6, SDG8, SDG9, SDG11, and SDG15; (3) System level, which evaluates the sustainable development of CPTC from social, economic and environmental dimensions. In , ‘A’ demonstrates that the calculation method for the United Nations sustainable development indicators is directly applied to obtain the results; ‘B’ denotes a new calculation method suitable for CPTC, based on the United Nations sustainable development indicator calculation method, with refinements and adjustments to the indicators. Specifically, we converted indicators with dimensions into ratios or percentages without dimensions to allow for a cross-regional comparison of indicators.
3.2. Sustainable development evaluation
3.2.1. Single goal evaluation
To visualise the sustainable development level of each province in the CPTC, we use a traffic light dashboard proposed by Sachs et al. (Citation2022). The seven provinces are ranked 1–7 in terms of their SDG scores, and are organised into four gradients: the first gradient is for the provinces ranked 1–2, indicating the highest sustainable development level of CPTC, denoted by ‘’; The second gradient is provinces ranked 3–4, indicating a higher sustainable development level, denoted by ‘
’; provinces ranked 5–6, indicating an average sustainable development level, are indicated by ‘
’; and province ranked 7, indicating a poorest sustainable development level, is indicated by ‘
’. ‘
’ indicate that no data are available for this corresponding SDG. A final visualisation map of each sustainable development goal situation for each province is derived ().
The dashboard and single goal scores reveal that Xinjiang Uygur Autonomous Region of the CPTC is outstanding in sustainable development. The region has high, rapid progress in all of the SDGs, with seven ranking in the top positions. Khyber Pakhtunkhwa Province has more room for improvement, especially in SDG1 (No Poverty), SDG8 (Economic Development), and SDG11 (Sustainable Cities). It can also be deduced that Khyber Pakhtunkhwa province is economically backward and has a slow urbanisation process in CPTC. As the capital of Pakistan, Islamabad Capital Territory has superior and accelerated progress towards various sustainable development goals compared to other provinces, especially SDG5 (Gender Equality), SDG8 (Economic Growth), and SDG9 (Sustainable Industrialisation) are in the first rank; The data deficit in Azad Kashmir has been substantially reduced, placing it in the middle of the pack overall, with balanced development across all objectives; In FATA, the SDG2 (Zero Hunger) and SDG16 (Strong Institutions for Peace and Justice) are developing at a faster pace compared to the SDGs in other areas, while all others are developing steadily; In Gilgit-Baltistan, SDG16 and SDG5 are developing at a higher level compared to the SDGs in other areas, while SDG4 is developing poorly, and education needs further improvement; In Punjab, SDG5 (Gender Equality), SDG6 (Clean Water), SDG8 (Economic Development), SDG9 (Sustainable Industrialisation) and SDG11 (Sustainable Cities) are in the first rank and perform well compared to the SDGs in other areas, while in SDG2 (Zero Hunger) and SDG3 (Healthy Life) have the poorest performance. It also shows that although the province has a high level of economic development, there are many poor people and a large gap between the rich and the poor. Gilgit-Baltistan and FATA stand in the middle of sustainable development.
3.2.2. Socio-economic-environmental multidimensional evaluation
Owing to the numerous development goals, it is not easy to compare the shortcomings of the development of each region’s development using only quantitative research on each development goal. At the same time, it is difficult to form a comprehensive understanding of CPTC sustainable development. Economic, social, environmental analyses can qualitatively describe the development status of each CPTC dimension. A comprehensive sustainable development evaluation of CPTC is conducted to understand its overall development. The sustainability scores calculated for each dimension in 2015, 2017 and 2020 are listed in , the spatial distribution of the sustainability level score for the socio-economic-environmental dimensions of the CPTC is shown in .
Table 4. socio-economic-environmental dimension sustainability score.
On the economic front, the overall level of economic sustainable development has increased from 2015 to 2020. However, the rate of development in 2017–2020 was slower than 2015–2017 due to the impact of the COVID-19 epidemic (Nundy et al. Citation2021), especially in the capital regions of Azad Kashmir and Islamabad. On a social level, the overall level of the region is increasing, especially in the northern and southern regions; however, but the rate of development has slowed significantly. Individual provinces, such as Khyber Pakhtunkhwa, have experienced a severe regression in terms of social sustainable development. This may be related to the impact of COVID-19 (Fenner and Cernev Citation2021; Nundy et al. Citation2021; Wang and Huang Citation2021), the frequency of natural disasters (Rahman et al. Citation2021; Waqas et al. Citation2021) and land degradation (Ahmad et al. Citation2021; Zhao et al. Citation2023) in the province. The most rapid development among the three dimensions was in the environmental dimension, which improved significantly in all provinces except Khyber Pakhtunkhwa.
Overall, the social, economic and environmental dimensions of sustainable development in the CPTC have been steadily increasing from 2015 to 2020 (), while only Khyber Pakhtunkhwa province is showing ‘regression’, which has negative environmental and social growth. The reason may be related to economic development that brings social imbalances and environmental damage (e.g. natural disasters and land degradation) and COVID-19. The Xinjiang Uyghur Autonomous Region has developed rapidly in the past five years with balanced and rapid development in all three dimensions, ranking first in terms of economic, environmental and social aspects. It has become the most prominent region for sustainable development in the CPTC. Punjab has performed well in economic and environmental aspects but has also experienced negative social development. Therefore, Punjab should pay more attention to social issues such as gender equality, health care, and education in its subsequent development to ensure a balanced approach to the 2030 Sustainable Development Goals; Islamabad Capital Territory has experienced rapid economic and environmental development in the past five years, and has achieved a balanced development in all three aspects of social, economic, and environmental development; FATA’s sustainable development level is low in 2015, but it has developed rapidly in recent years and is now basically on par with the sustainable development levels of Pakistani provinces; Gilgit-Baltistan is the lowest economic level in the district in 2015, but after five years of development, it has caught up with Khyber Pakhtunkhwa; Azad Kashmir has improved in social, economic and environmental dimensions, with significant progress being made in social sustainability as the fastest-growing aspect.
3.2.3. Comprehensive evaluation
The overall sustainable development of the CPTC is ‘high on both ends and low in the middle’. The northern Xinjiang Uyghur Autonomous Region has the highest level of sustainable development, while the southern of Punjab Province has a relatively high level of sustainable development. In contrast, the central part of the CPTC, including the Gilgit-Baltistan and Azad Kashmir, are located in areas with high levels of environmental development but a low level of economic sustainability, resulting in low levels of overall sustainable development. Subsequently, the overall sustainability scores for each province of CPTC were calculated, as shown in .
From 2015 to 2017, the overall of sustainable development level of the entire CPTC, except for the Khyber Pakhtunkhwa, has been increasing. It also fully reflects the advantages of the BRI, driving the sustainable development of the whole CPTC (Cao Citation2019; Guo et al. Citation2018). The Xinjiang Uyghur Autonomous Region has consistently ranked first among the entire CPTC and has the fastest improvement rate of sustainable development. However, compared with 2015–2017, the development rate has slowed down, which is associated with the COVID-19 in 2019 (Health Citation2020; Nundy et al. Citation2021; Wang and Huang Citation2021). In addition, Islamabad Capital Territory is experiencing rapid sustainable development. Other provinces have shown a slow increase in sustainability levels. The overall development level of the Khyber Pakhtunkhwa province is declining, and this decline is more serious. Although the economic sustainability score is increasing, the overall level is declining because of the significant decline in the social and environmental scores. This warns us that economic development should be accompanied by social and environmental factors to achieve sustainable development.
4. Discussion
4.1. Performance of VANUI
Land use data is closely related to the intensity of human activities, as indicated by the VANUI. The modified nighttime light data can effectively capture and characterise the intensity of human activities. We find that there is a strong correlation between VANUI and different types of land use, with buildings exhibiting the highest correlation, followed by farmland, water/grassland, forest/bushland, and snow/ice, which is consistent with Zhang, Schaaf, and Seto (Citation2013). These results show that the land use image can be used as an effective reference to verify the accuracy of VANUI ().
4.2. Sustainable development evaluation
(1) | Evaluation method |
The selection of a suitable localised indicator framework is a prerequisite for sustainable development evaluation. The Sustainable Development Solution Network (SDSN) proposed by Lynch and Sachs (Citation2021.) provides a practical method and basis for assessing various national levels around the world of sustainable development. In this study, we developed a CPTC regional-level localised sustainable development evaluation system based on SDSN. In this assessment system, the selection of the number of indicators and the localisation of indicators are grounded in and integrated with the region. We spatialise all evaluation indicators (including geographical and statistical indicators), to generate spatial data with consistent grids. This approach allows for a comprehensive, multidimensional grid-based evaluation using gridded spatial information that can cover and reflect actual geospatial differences across the entire region, providing a more detailed representation of the level of sustainable development. For example, (b) better reflects the detailed population distribution in CPTC compared with (a). It is clear from (b) that the population in the N.W.F.P. province is mainly concentrated in the southern region, which cannot be captured by the administrative division population distribution data. In addition, we reference the evaluation method of Sachs et al., but our method not only includes single-goal and comprehensive sustainable development evaluation, but also evaluates the sustainable development goals in three dimensions of social, economic, and environmental aspects, comprehensively grasping the level of sustainable development of CPTC from different perspectives. Huan et al. (Citation2021) developed a composite SDG index to assess the comprehensive performance of achieving SDGs. However, their evaluation results were also only presented at the regional level for each country and did not include a detailed grid-based evaluation. In addition, Shen et al. (Citation2020) and Vanham et al. (Citation2018) had conducted assessments of water resource sustainability based on individual targets of SDG6. Although these assessments provided more specific insights, they tended to fragment the overall concept of sustainable development. In contrast, our study evaluates the sustainability of the research area from multiple dimensions, providing a more comprehensive understanding of its shortcomings in terms of sustainable development.
Figure 8. Comparison of population distribution between (a) administrative division data and (b) gridded data.

Additionally, the evaluation data (including geospatial data and statistical data) we used are all open-sourced data from various official websites, which enhances the reproducibility and the extensibility of our research. And we change sustainable development indicators into ratio form, standardisation, gridding, aggregate all sustainable development indicators together, which also provides a solution for the aggregation of indicators with different sources and natures. Moreover, this study presents a reference case for sustainable development evaluation in regions that lack data and span multiple administrative units.
(2) | Evaluation result |
By the single goal, multidimensional and comprehensive evaluations of CPTC, we find that the sustainable development level in CPTC hugely has improved from 2015 to 2020. However, the development rate has significantly slowed, and the spatial level of sustainable development shows a pattern of ‘high at both ends and low in the middle’. The sustainable development level in terms of society, economy and environment has been improved in all areas of CPTC except for Khyber Pakhtunkhwa, which is consistent with the findings of other relevant studies in the region. In economic development, according to WEO statistics (Outlook Citation2023), the national per capita income of Pakistan was INR 29,176.78 in 2015 and INR 34,586.67 in 2020, which also showed that the Pakistani economy has been growing steadily for the five years. However, Ashraf (Citation2023) suggests that the economic growth rate has declined, which is consistent our findings on the economic sustainability. In terms of environmental sustainability, Ashraf further proves the increase in CO2 emissions in the CPTC, but this does not contradict the increased level of environmental sustainability in our study, because a single CO2 emission can’t be used as a criterion for good or bad environmental sustainability. Demena and Afesorgbor (Citation2020) also prove that foreign direct investment, such as BRI, enhances environmental sustainability, which confirms the results of our study on environmental aspects. In terms of social justice, Pakistan has seen an increase in women's employment over the past several years due to higher education levels (Abbass et al. Citation2022) and a deeper concept of gender equality (Majid and Siegmann Citation2021), which reflects a significant improvement in the social aspects of Pakistan (Kanwal, Chong, and Pitafi Citation2018), which is consistent with our conclusion that CPTC's level of social sustainability has increased.
4.3. Analysis of driving factors
The change in the spatial and temporal pattern of the sustainable development level of the CPTC as a major transportation corridor of the China-Pakistan Economic Corridor has been influenced by various factors such as policy implementation, geographical conditions, climate, and social environment.
BRI has promoted for sustainable development. From 2015 to 2020, the level of sustainable development of the CPTC increased steadily, which is inextricably linked to the BRI (Cao Citation2019). The Chinese government's BRI and the UN SDGs have the same roots and are mutually reinforced. The BRI is centred on corridor construction, which has led to the development of transportation, energy, and infrastructure in the CPTC (Ali Citation2018).It directly driven the development of SDG7, SDG8, and SDG9, providing more employment opportunities and economic development, and indirectly driven the development of SDG2, SDG4, and SDG6 within the region.
The sustainable development rate has slowed due to the COVID-19 pandemic. Our study found a slowdown in 2017–2020 compared with 2015–2017, which is related to the global outbreak of COVID-19 at the end of 2019 (Nundy et al. Citation2021). The pandemic increased the number of deaths and had a significant direct impact on SDG3, SDG4, and SDG16, as well as on SDG2, SDG7, and SDG8, posing an indirect threat. This has led to a slowdown in the CPTC sustainable development.
Geographic conditions affect the spatial distribution of sustainable development. The CPTC crosses the Karakorum Mountains, the Hindu Kush and the western end of the Himalayas, with large topographical fluctuations, showing a topographic feature of ‘high in the middle and low at the ends’. Consequently, the social and economic aspects of sustainable development within the CPTC also show a pattern of ‘high at both ends and low in the middle’, with Gilgit-Baltistan and Azad Kashmir in the middle having a low level of sustainable development, while Xinjiang in the north and Islamabad in the south have a high level of sustainable development in comparison.
The reasons for the ‘regression’ of sustainable development in Khyber Pakhtunkhwa. From 2015 to 2020, the Khyber Pakhtunkhwa Province is adversely affected by accelerated urbanisation and overgrazing, leading to land degradation, loss of agricultural land, and a significant decrease in grassland and arable land (Ahmad et al. Citation2021) (). These factors have contributed to the deterioration of the ecosystem. In addition, the region is vulnerable to natural disasters such as floods (Waqas et al. Citation2021) ((a)), cyclones, and droughts (Rahman et al. Citation2021) due to its topography and other geographic factors. These natural disasters have further hindered sustainable development in the region (Hamidi, Zeng, and Khan Citation2020). The frequency of natural disasters in the CPTC is shown in (b), based on data from EM-DAT. Furthermore, the lack of educational resources (Khan Citation2021), social security issues (Din et al. Citation2022), and low levels of healthcare in Khyber Pakhtunkhwa (Israr et al. Citation2020) have negatively impacted the social aspects of development in the province.
Figure 9. Natural disasters in CPTC (a is Flood hazard of Khyber Pakhtunkhwa province in 2016 (PDMA, 2016) Image source: https://floodlist.com/asia/pakistan-least-46-killed-floods-khyber-pakhtunkhwa; b is Number of Natural Disasters in the CPTC 2015-2020).

4.4. Development suggestions
Targeted remediation of shortcomings of sustainable development. The central region of the CPTC needs targeted interventions, especially SDG4 (Quality Education) and SDG8 (Decent Work and Economic Growth), which are shortcomings of the sustainable development goals of the region. Targeted enhancement of SDG4 and SDG8 sustainable development levels to narrow the gap between them and the respective sustainable development goals. Simultaneously, the coordinated development of each region should be achieved, bridging the gap between the central northern and southern regions in terms of development goals. However, these interventions should not be performed at the expense of environmental goals. There is a pressing need to balance economic development with social equity, security, and ecological quality to achieve smooth and comprehensive sustainable development of society, economy, and environment.
Optimising the strategies for economic development. CPTC has witnessed significant land degradation and a marked expansion in cultivated land, leading to poor environmental indicators. For instance, Khyber Pakhtunkhwa witnessed an increase in sustainable economic development from 2015 to 2020, but a decline in environmental sustainability. Therefore, we recommend undertaking an industrial transformation and promotion of green and sustainable industries. Reducing the development of heavy industry and agriculture, combining the characteristics of its own environmental and geographical advantages, developing tourism and high-tech industries, and enhancing the economy while improving the level of sustainable development from environmental and social aspects.
Improve the ability to prevent and mitigate disasters. The CPTC is very vulnerable to natural disasters (), such as floods, landslides, and mudslides due to its undulating terrain and complex topography, which are important factors that limit its development. The disaster prevention and mitigation capacity of the region must be improved to enhance sustainable development.
4.5. Future work direction
In this study, we use spatialised and statistical indicators to construct the CPTC's sustainable development indicator system, and conduct single-goal evaluation, multidimensional evaluation, and comprehensive evaluation of the CPTC are carried out for the SDGs proposed by the United Nations. Follow-up research can be improved and developed in the following aspects: (1) Due to the special geographical location of the study area, data acquisition is relatively limited. In future research, we can combine various data, such as field surveys, to strengthen the collection and integration of big earth data, enrich and expand the number of evaluation indicators, and evaluate them in more detail. (2) SDGs are not completely independent from each other, and there are synergies or trade-offs among them. Actions taken for one goal may enhance (McCollum et al. Citation2018) or offset exist between another goal, resulting in an imbalance in the SDGs (Mulligan et al. Citation2020). For example, using coal to improve energy access (SDG7) accelerates climate change (SDG13) and damages human health through air pollution (SDG3). In future research, synergy and trade-off analyse among indicators can be strengthened by considering the interrelationships among the SDGs in an integrated manner to contribute more deeply to the overall evaluation. (3) Finally, all the 17 SDGs are accessed from a more comprehensive perspective. That means more related indicators at the goal and indicator levels can be involved to support a comprehensive and localised assessment of sustainable development in this region in the future.
5. Conclusion
Oriented to the gap of sustainable development evaluation in high Asian area, this study develops a localised indicator evaluation system for the sustainable development of CPTC. Based on this evaluation system, a single-goal, multidimensional and comprehensive evaluation of the CPTC sustainable development level in 2015, 2017 and 2020 is conducted. The sustainable development level of the CPTC greatly improve from 2015 to 2020, and the development rate from 2015 to 2017 is higher than that from 2017 to 2020. This reflects the advantages of the BRI, which has benefited the entire CPTC. From a spatial perspective, the sustainable development levels of the CPTC show a pattern of ‘high at the ends and low in the middle’, with Xinjiang in the north and Islamabad in the south having higher sustainable development levels, while Gilgit-Baltistan and Azad Kashmir in the centre having lower sustainable development levels and Khyber Pakhtunkhwa province typifies a ‘regression’ in sustainable development levels. Despite the economic scores improved considerably, the social and environmental scores decrease significantly, leading to lower overall scores. This is a warning to the government to intervene in economic development and focus on the social and environmental aspects to achieve sustainable development. Targeted interventions are needed in the South to address these gaps, especially in socioeconomic-related SDGs. This study not only fills in the gaps of 8 Tier II (no data) in CPTC, but also identifies existing sustainability issues and offers targeted recommendations for sustainable development.
Disclosure statement
No potential conflict of interest was reported by the author(s).
Data availability statement
Publicly available datasets were analysed in this study, specific data sources can be found in and .
Additional information
Funding
References
- Abbass, K., M. Asif, A. A. K. Niazi, T. F. Qazi, A. Basit, and F. A. Al-Muwaffaq Ahmed. 2022. “Understanding the Interaction Among Enablers of Quality Enhancement of Higher Business Education in Pakistan.” PLoS One 17 (5): e0267919. https://doi.org/10.1371/journal.pone.0267919.
- Ahmad, S., M. Israr, M. Amin, M. Hashim, N. Ahmad, and R. Ahmad. 2021. “Two-decade Spatio-Temporal Land Use and Cover Changes in District Shangla of Khyber Pakhtunkhwa, Pakistan.” Sarhad Journal of Agriculture 37 (4): 1230–1237.
- Ali, M. 2018. “The China-Pakistan Economic Corridor: Tapping Potential to Achieve the 2030 Agenda in Pakistan.” China Quarterly of International Strategic Studies 4 (02): 301–325. https://doi.org/10.1142/S2377740018500094.
- Ali, S., P. Biermanns, R. Haider, and K. Reicherter. 2019. “Landslide Susceptibility Mapping by Using a Geographic Information System (GIS) Along the China–Pakistan Economic Corridor (Karakoram Highway), Pakistan.” Natural Hazards and Earth System Sciences 19 (5): 999–1022. https://doi.org/10.5194/nhess-19-999-2019.
- Allen, C., G. Metternicht, T. Wiedmann, and M. Pedercini. 2019. “Greater Gains for Australia by Tackling all SDGs but the Last Steps Will be the Most Challenging.” Nature Sustainability 2 (11): 1041–1050. https://doi.org/10.1038/s41893-019-0409-9.
- Allen, C., M. Reid, J. Thwaites, R. Glover, and T. Kestin. 2020. “Assessing National Progress and Priorities for the Sustainable Development Goals (SDGs): Experience from Australia.” Sustainability Science 15 (2): 521–538. https://doi.org/10.1007/s11625-019-00711-x.
- Ashizawa, T., N. Sudo, and H. Yamamoto. 2022. How Do Floods Affect the Economy? An Empirical Analysis using Japanese Flood Data. Bank of Japan. https://www.boj.or.jp/en/research/wps_rev/wps_2022/data/wp22e06.pdf.
- Ashraf, J. 2023. “Does Political Risk Undermine Environment and Economic Development in Pakistan? Empirical Evidence from China–Pakistan Economic Corridor.” Economic Change and Restructuring 56 (1): 581–608. https://doi.org/10.1007/s10644-022-09434-z.
- Boeren, E. 2019. “Understanding Sustainable Development Goal (SDG) 4 on “Quality Education” from Micro, Meso and Macro Perspectives.” International Review of Education 65 (2): 277–294. https://doi.org/10.1007/s11159-019-09772-7.
- Campbell, B. M., J. Hansen, J. Rioux, C. M. Stirling, and S. Twomlow. 2018. “Urgent Action to Combat Climate Change and Its Impacts (SDG 13): Transforming Agriculture and Food Systems.” Current Opinion in Environmental Sustainability 34:13–20. https://doi.org/10.1016/j.cosust.2018.06.005.
- Cao, J. 2019. “China’s Belt and Road Initiative 2.0: Delivering Global Public Goods for Sustainable Development.” China Quarterly of International Strategic Studies 5 (02): 233–248. https://doi.org/10.1142/S2377740019500155.
- Chang, M., P. Cui, X. Dou, and F. Su. 2021. “Quantitative Risk Assessment of Landslides Over the China-Pakistan Economic Corridor.” International Journal of Disaster Risk Reduction 63:102441. https://doi.org/10.1016/j.ijdrr.2021.102441.
- Chu, L., F. Oloo, M. Sudmanns, D. Tiede, D. Hölbling, T. Blaschke, and I. Teleoaca. 2020. “Monitoring Long-Term Shoreline Dynamics and Human Activities in the Hangzhou Bay, China, Combining Daytime and Nighttime EO Data.” Big Earth Data 4 (3): 242–264. https://doi.org/10.1080/20964471.2020.1740491.
- Demena, B. A., and S. K. Afesorgbor. 2020. “The Effect of FDI on Environmental Emissions: Evidence from a Meta-Analysis.” Energy Policy 138:111192. https://doi.org/10.1016/j.enpol.2019.111192.
- Diaz-Sarachaga, J. M., D. Jato-Espino, and D. Castro-Fresno. 2018. “Is the Sustainable Development Goals (SDG) Index an Adequate Framework to Measure the Progress of the 2030 Agenda?” Sustainable Development 26 (6): 663–671. https://doi.org/10.1002/sd.1735.
- Din, J. U., D. Sanaullah, M. Hassan, N. Ihsan, A. Amin, I. Ali, A. Khan, and A. Mumtaz. 2022. “Socio-Economic Implications of Terrorism on Khyber Pakhtunkhwa: A Case Study of ANP Era (2008-2013).” Webology 19 (3): 3095–3111.
- Dos Santos, P. H., S. M. Neves, D. O. Sant’Anna, C. H. De Oliveira, and H. D. Carvalho. 2019. “The Analytic Hierarchy Process Supporting Decision Making for Sustainable Development: An Overview of Applications.” Journal of Cleaner Production 212:119–138. https://doi.org/10.1016/j.jclepro.2018.11.270.
- EM-DAT. 2020. Public emdat Custom Request. https://public.emdat.be/data.
- Fenner, R., and T. Cernev. 2021. “The Implications of the Covid-19 Pandemic for Delivering the Sustainable Development Goals.” Futures 128:102726. https://doi.org/10.1016/j.futures.2021.102726.
- Ferguson, T., and C. G. Roofe. 2020. “SDG 4 in Higher Education: Challenges and Opportunities.” International Journal of Sustainability in Higher Education 21 (5): 959–975. https://doi.org/10.1108/IJSHE-12-2019-0353.
- Giupponi, C., and A. K. Gain. 2017. “Integrated Spatial Assessment of the Water, Energy and Food Dimensions of the Sustainable Development Goals.” Regional Environmental Change 17 (7): 1881–1893. https://doi.org/10.1007/s10113-016-0998-z.
- Guo, H., F. Chen, Z. Sun, J. Liu, and D. Liang. 2021. “Big Earth Data: A Practice of Sustainability Science to Achieve the Sustainable Development Goals.” Science Bulletin 66 (11): 1050–1053. https://doi.org/10.1016/j.scib.2021.01.012.
- Guo, H., D. Liang, Z. Sun, F. Chen, X. Wang, J. Li, L. Zhu, et al. 2022. “Measuring and Evaluating SDG Indicators with Big Earth Data.” Science Bulletin 67 (17): 1792–1801. https://doi.org/10.1016/j.scib.2022.07.015.
- Guo, H., J. Liu, Y. Qiu, M. Menenti, F. Chen, P. F. Uhlir, L. Zhang, et al. 2018. “The Digital Belt and Road Program in Support of Regional Sustainability.” International Journal of Digital Earth 11 (7): 657–669. https://doi.org/10.1080/17538947.2018.1471790.
- Hamidi, A. R., Z. Zeng, and M. A. Khan. 2020. “Household Vulnerability to Floods and Cyclones in Khyber Pakhtunkhwa, Pakistan.” International Journal of Disaster Risk Reduction 46:101496. https://doi.org/10.1016/j.ijdrr.2020.101496.
- Health, T. L. P. 2020. “Will the COVID-19 Pandemic Threaten the SDGs?” The Lancet Public Health 5 (9): e460. https://doi.org/10.1016/S2468-2667(20)30189-4
- Huan, Y., T. Liang, H. Li, and C. Zhang. 2021. “A Systematic Method for Assessing Progress of Achieving Sustainable Development Goals: A Case Study of 15 Countries.” Science of The Total Environment 752:141875. https://doi.org/10.1016/j.scitotenv.2020.141875.
- Hutton, G., and C. Chase. 2016. “The Knowledge Base for Achieving the Sustainable Development Goal Targets on Water Supply, Sanitation and Hygiene.” International Journal of Environmental Research and Public Health 13 (6): 536. https://doi.org/10.3390/ijerph13060536.
- Israr, M., H. Ross, S. Ahmad, N. Ahmad, and U. Pervaiz. 2020. “Measuring Multidimensional Poverty among Farm Households in Rural Pakistan Towards Sustainable Development Goals.” Sarhad Journal of Agriculture 36 (1): 130–142.
- Jiang, H., H. Guo, Z. Sun, Q. Xing, H. Zhang, Y. Ma, and S. Li. 2022. “Projections of Urban Built-up Area Expansion and Urbanization Sustainability in China's Cities Through 2030.” Journal of Cleaner Production 367:133086. https://doi.org/10.1016/j.jclepro.2022.133086.
- Kanwal, S., R. Chong, and H. Pitafi. 2018. “China–Pakistan Economic Corridor Projects Development in Pakistan: Local Citizens Benefits Perspective.” Journal of Public Affairs 19: e1888. https://doi.org/10.1002/pa.1888.
- Kaymaz, ÇK, S. Birinci, and Y. Kızılkan. 2022. “Sustainable Development Goals Assessment of Erzurum Province with SWOT-AHP Analysis.” Environment, Development and Sustainability 24 (3): 2986–3012. https://doi.org/10.1007/s10668-021-01584-w.
- Khan, A. 2021. “Factor Influencing the Implementation of the Sustainable Development Goal Quality Education in Khyber Pakhtunkhwa, Pakistan.” European Journal of Agricultural and Rural Education 2 (4): 1–4.
- Kramer, B., and A. MacKinnon. 1993. “Localization: Theory and Experiment.” Reports on Progress in Physics 56 (12): 1469. https://doi.org/10.1088/0034-4885/56/12/001.
- Kummu, M., M. Taka, and J. H. A. Guillaume. 2020. Data from: Gridded global datasets for Gross Domestic Product and Human Development Index over 1990-2015 [Dataset]. Dryad. https://doi.org/10.5061/dryad.dk1j0.
- Kussul, N., M. Lavreniuk, A. Kolotii, S. Skakun, O. Rakoid, and L. Shumilo. 2020. “A Workflow for Sustainable Development Goals Indicators Assessment Based on High-Resolution Satellite Data.” International Journal of Digital Earth 13 (2): 309–321. https://doi.org/10.1080/17538947.2019.1610807.
- Lynch, A., and J. Sachs. 2021. https://www.everlastconsultancy.nl/wp-content/uploads/2021/12/UnitedStatesSustainableDevelopmentReport2021.pdf. Sustainable DevelopmentSustainable Development.
- Majid, H., and K. A. Siegmann. 2021. “The Effects of Growth on Women’s Employment in Pakistan.” Feminist Economics 27 (4): 29–61. https://doi.org/10.1080/13545701.2021.1942512.
- McArthur, J. W., and K. Rasmussen. 2019. “Classifying Sustainable Development Goal Trajectories: A Country-Level Methodology for Identifying Which Issues and People are Getting Left Behind.” World Development 123:104608. https://doi.org/10.1016/j.worlddev.2019.06.031.
- McCollum, D. L., L. G. Echeverri, S. Busch, S. Pachauri, S. Parkinson, J. Rogelj, V. Krey, J. C. Minx, M. Nilsson, and A.-S. Stevance. 2018. “Connecting the Sustainable Development Goals by Their Energy Inter-Linkages.” Environmental Research Letters 13 (3): 033006. https://doi.org/10.1088/1748-9326/aaafe3.
- Mulligan, M., A. van Soesbergen, D. G. Hole, T. M. Brooks, S. Burke, and J. Hutton. 2020. “Mapping Nature's Contribution to SDG 6 and Implications for Other SDGs at Policy Relevant Scales.” Remote Sensing of Environment 239:111671. https://doi.org/10.1016/j.rse.2020.111671.
- NBS (National Bureau of Statistics). 2020. China Statistical Yearbook. http://www.stats.gov.cn/.
- NOAA (National Oceanic and Atmospheric Administration). 2020. Visible Infrared Imaging Radiometer Suite (VIIRS). https://ngdc.noaa.gov/eog/availability.html.
- Nundy, S., A. Ghosh, A. Mesloub, G. A. Albaqawy, and M. M. Alnaim. 2021. “Impact of COVID-19 Pandemic on Socio-Economic, Energy-Environment and Transport Sector Globally and Sustainable Development Goal (SDG).” Journal of Cleaner Production 312:127705. https://doi.org/10.1016/j.jclepro.2021.127705.
- OSM (OpenStreetMap). 2023. Pakistan-latest.osm. https://www.openstreetmap.org.
- Outlook, W. E. 2023. World Economic Outlook Database. Retrieved April 17, 2023. https://www.imf.org/.
- Patole, M. 2018. “Localization of SDGs Through Disaggregation of KPIs.” Economies 6 (1): 15. https://doi.org/10.3390/economies6010015.
- Rahman, G., A.-u. Rahman, S. Ullah, M. Dawood, M. F. U. Moazzam, and B. G. Lee. 2021. “Spatio-temporal Characteristics of Meteorological Drought in Khyber Pakhtunkhwa, Pakistan.” PLoS One 16 (4): e0249718.
- Sachs, J. D., C. Kroll, G. Lafortune, G. Fuller, and F. Woelm. 2022. Sustainable Development Report 2022. New York: Cambridge University Press. https://s3.amazonaws.com/sustainabledevelopment.report/2022/2022-sustainable-development-report.pdf.
- Sadoff, C. W., E. Borgomeo, and S. Uhlenbrook. 2020. “Rethinking Water for SDG 6.” Nature Sustainability 3 (5): 346–347. https://doi.org/10.1038/s41893-020-0530-9.
- SATP (South Asia Terrorism Portal). 2023. Number of Terrorism Related Incidents. https://www.satp.org/datasheet-terrorist-attack/fatalities/pakistan.
- Shelton, S., and R. D. Dixon. 2023. “Long-term Seasonal Drought Trends in the China-Pakistan Economic Corridor.” Climate 11 (2): 45. https://doi.org/10.3390/cli11020045.
- Shen, M., D. Hongtao, C. Zhigang, X. Kun, Q. Tianci, M. Jinge, L. Dong, S. Kaishan, H. Chunlin, and S. Xiaoyu. 2020. “Sentinel-3 OLCI Observations of Water Clarity in Large Lakes in Eastern China: Implications for SDG 6.3. 2 Evaluation.” Remote Sensing of Environment 247:111950. https://doi.org/10.1016/j.rse.2020.111950.
- United Nations. 2015. Transforming Our World: The 2030 Agenda for Sustainable Development. New York. https://sustainabledevelopment.un.org/content/documents/21252030%20Agenda%20for%20Sustainable%20Development%20web.pdf?ref = truth11.com.
- USGS (United States Geological Survey). 2020. Landsat OLI. https://earthexplorer.usgs.gov/.
- Vanham, D., A. Y. Hoekstra, Y. Wada, F. Bouraoui, A. de Roo, M. M. Mekonnen, W. J. van de Bund, et al. 2018. “Physical Water Scarcity Metrics for Monitoring Progress Towards SDG Target 6.4: An Evaluation of Indicator 6.4. 2 “Level of Water Stress.” Science of The Total Environment 613-614: 218–232. https://doi.org/10.1016/j.scitotenv.2017.09.056.
- Wang, Q., and R. Huang. 2021. “The Impact of COVID-19 Pandemic on Sustainable Development Goals–A Survey.” Environmental Research 202:111637. https://doi.org/10.1016/j.envres.2021.111637.
- Waqas, H., L. Lu, A. Tariq, Q. Li, M. F. Baqa, J. Xing, and A. Sajjad. 2021. “Flash Flood Susceptibility Assessment and Zonation Using an Integrating Analytic Hierarchy Process and Frequency Ratio Model for the Chitral District, Khyber Pakhtunkhwa, Pakistan.” Water 13 (12): 1650. https://doi.org/10.3390/w13121650.
- The World Bank. 2023. IBRD. “GDP (current US$)”. https://data.worldbank.org.cn/indicator/NY.GDP.MKTP.CD.
- WorldPop. 2020. Constrained Individual Countries 2020 (100 m Resolution). https://hub.worldpop.org/geodata/listing?id = 78.
- Wu, X., B. Fu, S. Wang, S. Song, Y. Li, Z. Xu, Y. Wei, and J. Liu. 2022. “Decoupling of SDGs Followed by Re-coupling as Sustainable Development Progresses.” Nature Sustainability 5 (5): 452–459. https://doi.org/10.1038/s41893-022-00868-x.
- Yang, C., H. Liu, Q. Li, X. Wang, W. Ma, C. Liu, X. Fang, Y. Tang, T. Shi, and Q. Wang. 2022. “Human Expansion Into Asian Highlands in the 21st Century and Its Effects.” Nature Communications 13 (1): 4955. https://doi.org/10.1038/s41467-022-32648-8.
- Yu, S.-l., and Y. Luo. 2019. “Application of Light Remote Sensing Data in Monitoring the Relationship Between the Expansion of Human Construction and Its Influencing Factors.” Hubei Agricultural Sciences 58 (16): 133.
- Zhang, X., L. Liu, T. Zhao, Y. Gao, X. Chen, and J. Mi. 2022. “GISD30: Global 30 m Impervious-Surface Dynamic Dataset from 1985 to 2020 Using Time-Series Landsat Imagery on the Google Earth Engine Platform.” Earth System Science Data 14 (4): 1831–1856. https://doi.org/10.5194/essd-14-1831-2022.
- Zhang, Q., C. Schaaf, and K. C. Seto. 2013. “The Vegetation Adjusted NTL Urban Index: A New Approach to Reduce Saturation and Increase Variation in Nighttime Luminosity.” Remote Sensing of Environment 129:32–41. https://doi.org/10.1016/j.rse.2012.10.022.
- Zhao, Y., X. Zhao, D. Fan, and Y. Qiu. 2023. “A Comprehensive Method for Refining Essential SDGs Variables for Land Degradation Monitoring Based on the DPSIR Framework.” International Journal of Digital Earth 16 (1): 741–761. https://doi.org/10.1080/17538947.2023.2182375.