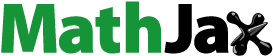
ABSTRACT
Flood models rely on accurate topographic data representing the bare earth ground surface. In many parts of the world, the only topographic data available are the free, satellite-derived global Digital Elevation Models (DEMs). However, these have well-known inaccuracies due to limitations of the sensors used to generate them (such as a failure to fully penetrate vegetation canopies and buildings). We assess five contemporary, 1 arc-second (≈30 m) DEMs -- FABDEM, Copernicus DEM, NASADEM, AW3D30 and SRTM -- using a diverse reference dataset comprised of 65 airborne-LiDAR surveys, selected to represent biophysical variations in flood-prone areas globally. While vertical accuracy is nuanced, contingent on the specific metrics used and the biophysical character of the site being assessed, we found that the recently-released FABDEM consistently ranked first, improving on the second-place Copernicus DEM by reducing large positive errors associated with forests and buildings. Our results suggest that land cover is the main factor explaining vertical errors (especially forests), steep slopes are associated with wider error spreads (although DEMs resampled from higher-resolution products are less sensitive), and variable error dependency on terrain aspect is likely a function of horizontal geolocation errors (especially problematic for AW3D30 and Copernicus DEM).
1. Introduction
Flood impacts are rising globally (UNDRR Citation2022), driven primarily by rapid urbanisation and increasing settlement in floodplains (Ford et al. Citation2019; Tellman et al. Citation2021) and exacerbated by the increasing hydro-meteorological variability associated with climate change (Arnell and Gosling Citation2016). These interconnected changes in flood susceptibility and human exposure mean that past flood events are less reliable indicators of future impacts. Particularly given this non-stationarity in flood drivers, modelling is an important tool in understanding and reducing flood risk, enabling emergency managers and city planners to predict and prepare for plausible future conditions and events.
As well as the hydro-meteorological inputs, an integral requirement for such flood models is topographic data, ideally representing ‘bare earth’ ground elevations (Sampson et al. Citation2015; Sanders Citation2007). The vertical accuracy of these data is critical since the inundation depths and extents simulated by flood models are highly sensitive to even minor vertical errors, especially in low-gradient floodplains (Horritt and Bates Citation2002).
Currently, the optimal source for such topographic data is high-precision Light Detection and Ranging (LiDAR) surveys (Hancock et al. Citation2021) that can penetrate vegetation canopies (to record the ground beneath) and distinguish between different objects (such as vegetation, buildings and ground). When LiDAR surveys are processed to filter out vegetation, buildings and other surface obstructions, they can yield high-resolution ‘bare earth’ Digital Terrain Models (DTMs) at very high accuracies, with vertical Root Mean Square Error (RMSE) typically well below 30 cm (Hodgson and Bresnahan Citation2004). Local and even regional-scale DTMs derived from airborne LiDAR surveys are increasingly available in high-income countries but remain extremely rare in low-income countries (Sampson et al. Citation2016), where the risk to lives and livelihoods is often the greatest (Rentschler, Salhab, and Jafino Citation2022).
Where accurate DTMs are not available, regional-scale flood modelling often relies instead on freely-available global Digital Elevation Models (DEMs). These DEMs are typically characterised by coarse spatial resolutions (1 arc-second at best, roughly 30 m at the equator) and significant vertical errors (Hawker, Neal, and Bates Citation2019). The spaceborne sensors used to generate these global DEMs -- either Synthetic Aperture Radar (SAR) or stereoscopic imaging -- penetrate vegetation canopies to varying degrees (and buildings not at all), describing a topographic surface that generally sits closer to a Digital Surface Model (DSM) than a DTM (Guth et al. Citation2021). In addition, the global DEMs are subject to a range of potential distortions associated with atmospheric conditions at the time of raw data capture, sensor motion/alignment, post-processing routines, and void-filling methods (Rodríguez, Morris, and Belz Citation2006; Takaku et al. Citation2015).
For the purposes of flood modelling, these vertical errors (defined here as deviations from the ‘bare earth’ ground surface) can act as artificial sinks or obstructions that detain or divert simulated flows (Sampson et al. Citation2016), resulting in misleading flood maps and exposure assessments. Significantly, these vertical errors often have a positive bias (i.e. ground elevations are overestimated), spuriously diverting flood waters around thick vegetation and built-up areas (Neal et al. Citation2009). This is especially problematic when estimating the coastal inundation extent associated with a given (absolute) sea level (Gesch Citation2018), and represents the largest source of uncertainty when modelling coastal inundation under climate change (Kulp and Strauss Citation2019). Investigating the impact of this positive vertical bias in one of the most widely-used DEMs (SRTM), Kulp and Strauss (Citation2019) estimated that it would result in global population exposure to coastal flooding being underpredicted by a factor of three, indicating the magnitude of the problem.
There have been sustained calls for an improved DEM (in terms of spatial resolution and vertical accuracy) to support more reliable flood modelling at regional and global scales, particularly in data-scarce environments (Hawker et al. Citation2018; Sampson et al. Citation2016; Schumann Citation2014; Simpson et al. Citation2015). Spaceborne LiDAR holds promise but current mission designs (such as GEDI and ICESat-2) are limited to sparse (spatially discontinuous) sampling -- for instance, GEDI is expected to image no more than 4% of the Earth's surface over its lifetime (Dubayah et al. Citation2020). This level of coverage may be sufficient to inform very coarse DTMs, such as the 5 km-resolution raster produced by Vernimmen, Hooijer, and Pronk (Citation2020) for global lowlands. However, this is not fit-for-purpose at the spatial resolutions necessary for regional-scale flood modelling, variously estimated at 5–50 m (Savage et al. Citation2016; Winsemius et al. Citation2019).
Consequently, regional-scale flood models in many parts of the world will continue to rely on the DEMs currently available. As such, it is important to understand their relative accuracies and particular strengths/weaknesses. Considering only those with a horizontal resolution of 1 arc-second, summarises the main DEMs available, derived from either Synthetic Aperture Radar (SAR) or stereoscopic imagery. Note the differences in spatial coverage (at higher latitudes) and the significant variation in acquisition times, in terms of both the year and duration of data collection.
Table 1. Overview of freely-available, 1 arc-second global DEM products.
Numerous studies have assessed the accuracy of one or more of these DEMs, reflecting their importance across a range of applications beyond flood modelling; including landslide prediction (Ciampalini et al. Citation2016), ecological modelling (Moudrý et al. Citation2018), and wetland carbon dynamics (Laudon et al. Citation2011). Until recently, there was a general consensus that the SRTM DEM was most accurate (Sampson et al. Citation2015; Sanders Citation2007), with AW3D30 sometimes preferred (Courty, Soriano-Monzalvo, and Pedrozo-Acuña Citation2019; Jain et al. Citation2018) and ASTER consistently found to be least accurate (Gesch Citation2018; Hirt, Filmer, and Featherstone Citation2010). For a more detailed overview of past accuracy assessments, readers are referred to Mesa-Mingorance and Ariza-López (Citation2020) and Purinton and Bookhagen (Citation2021).
Since October 2018, four new DEMs have been released to the public, based on either SRTM (NASADEM) or the more recent TanDEM-X mission (TanDEM-X 90, Copernicus DEM and FABDEM). Although there are limited comparative assessments available, these appear to be significant improvements over the older options (Guth and Geoffroy Citation2021; Marsh, Harder, and Pomeroy Citation2023), including for flood modelling applications (Garrote Citation2022). Significantly, the most recent DEM (FABDEM) used machine learning (random forest models) to reduce vertical errors (Hawker et al. Citation2022), with more advanced deep learning techniques based on multi-modal data (Hong et al. Citation2021, Citation2023) showing potential to provide further improvements in the future, as indicated by preliminary studies (Meadows and Wilson Citation2021; Nguyen et al. Citation2022).
Many of the past DEM accuracy assessments have also investigated the factors influencing vertical accuracy, providing insights into DEM suitability (e.g. for different terrains or land cover classes) and vertical error correction. The most significant explanatory factors consistently identified are land cover and slope, with aspect sometimes found to be important too (Kramm and Hoffmeister Citation2021; Mesa-Mingorance and Ariza-López Citation2020). In general, vertical errors are largest for land cover classes representing elevated surfaces at least partially opaque to spaceborne sensors (such as forest canopies and buildings) and for steep slopes (Gdulová, Marešová, and Moudrý Citation2020; Hawker, Neal, and Bates Citation2019).
The purpose of this study is to provide a comprehensive accuracy assessment of contemporary and freely-available DEMs, using a large and diverse collection of study sites representative of the land cover and terrain conditions found in flood-prone areas globally. Our assessment is based on well-established methodologies and accuracy metrics (allowing us to situate our results in the context of past studies); its importance lies instead in assessing recently-released DEMs (especially FABDEM) in flood-prone environments and in using a globally distributed reference dataset to enable more general, robust conclusions. As well as evaluating the overall accuracy of each DEM, we explore how accuracy varies by land cover and terrain (slope and aspect). These outcomes will support flood modellers in selecting the most appropriate DEM for a given region, based on its land cover and terrain, and understanding its limitations (likely error profile).
2. Material and methods
2.1. Reference data
To assess each DEM's vertical accuracy, reference elevation data are needed. These reference data should define the bare ground surface with an accuracy at least three times greater than that of the dataset being assessed (Maune Citation2007). Reported Root Mean Square Error (RMSE) values for the DEMs considered here typically range from 3–15 m (Gesch Citation2018; Uuemaa et al. Citation2020), implying that reference elevation data should have RMSE values below 1 m.
Based on its ability to penetrate vegetation canopies and filter out non-ground returns, airborne LiDAR captures the true ground surface with very high accuracy, reporting vertical RMSE values well within 0.3 m (Gesch Citation2018; Hodgson and Bresnahan Citation2004). For this study, we collated 65 DTMs derived from airborne LiDAR surveys, spread across 18 countries and covering more than 14,000 km. This provided more than 18.2 million grid cells for each DEM, which we believe to be the largest and most diverse reference dataset processed for this purpose. A detailed summary of these DTM datasets is provided in Table S1 (Supplementary Material).
2.2. Site selection
Study sites were selected to collate a representative sample of the typical biophysical conditions found in flood-prone areas globally, constrained by the limited availability of high-resolution ( m) airborne LiDAR DTMs. We used the following factors to stratify our sample: (1) land cover, (2) climate zone, (3) degree of urbanisation, and (4) slope. The specific land cover dataset used is described in Section 2.6.2, climate zones are the present-day Köppen–Geiger zones published by Beck et al. (Citation2018), degree of urbanisation is the 2020 raster in the Global Human Settlement Layer Data Package 2022 (Schiavina, Melchiorri, and Pesaresi Citation2022), and slope ranges were calculated using the MERIT DEM (Yamazaki et al. Citation2017).
As an indicative delineation of flood-prone areas, we merged the GFPLAIN250m global floodplain raster (Nardi et al. Citation2019) with the Low Elevation Coastal Zone (LECZ) raster published by MacManus et al. (Citation2021), representative of fluvial and coastal flood susceptibility respectively. Reference DTMs located directly within these flood-prone areas were preferred wherever possible. However, we also included DTMs in adjacent areas, targeting a representative sample of the four factors listed above, with reference to the distributions estimated for each factor within flood-prone areas globally. These global distributions within flood-prone areas were calculated using Google Earth Engine (Gorelick et al. Citation2017), excluding permanent water bodies from the analysis using the Surface Water Occurrence layer published by Pekel et al. (Citation2016). A threshold of 90% was used to filter out ocean or inland lake cells, while retaining occasionally-flooded plains and riverbanks (determined through visual assessments and a sensitivity analysis).
Effectively, this guided our site selection to minimise coverage in areas highly unlikely to be affected by floods (e.g. steep mountainsides) and to collate a diverse evaluation database representing the typical conditions in flood-prone areas globally. The locations of the selected sites are shown in , along with the resulting areal coverage of the four selection factors (bars) and their corresponding distributions in flood-prone areas globally (dotted lines).
2.3. Global DEMs
Considering all publicly-available DEMs, we limit our evaluation to the 1 arc-second products, given the well known impact of spatial resolution on DEM derivatives (Vaze, Teng, and Spencer Citation2010) and subsequent complications in comparing accuracies at different resolutions. Of the DEMs listed in , only ASTER is excluded (in the interests of brevity and clarity in visual comparisons), given that past studies have so consistently found it to be inferior to other options (Hirt, Filmer, and Featherstone Citation2010; Uuemaa et al. Citation2020).
All considered DEMs are summarised below, grouped by the satellite mission during which their primary raw data were collected (either synthetic aperture radar or panchromatic stereoscopic imagery). Within each group, the different DEM products available represent alternative processing workflows, with more recent options taking advantage of algorithms and/or supplementary data sources not available to previous iterations.
2.3.1. Shuttle Radar Topography Mission (SRTM)
The Shuttle Radar Topography Mission (SRTM) was flown over 11 days in February 2000, collecting C-band synthetic aperture radar (SAR) over land areas between 60N and 56
S, representing around 80% of the total landmass (Farr et al. Citation2007). We use version 3 of the 1 arc-second SRTM DEM, released in 2015.
Released in February 2020, the 1 arc-second NASADEM (version 1) is a complete re-processing of the SRTM radar data, taking advantage of improved SAR methods and newly-available elevation data (especially ICESat altimetry and version 3 of the ASTER DEM) to fill voids (Crippen et al. Citation2016).
2.3.2. Advanced Land Observing Satellite (ALOS)
From 2006–2011, the Panchromatic Remote-sensing Instrument for Stereo Mapping (PRISM) sensor on board the ALOS satellite (Japan Aerospace Exploration Agency) captured stereoscopic imagery with a resolution of 2.5 m (Takaku et al. Citation2016). This was used to produce a very high-resolution (0.15 arc-seconds) commercial DEM, ALOS World 3D (AW3D), later resampled to the freely-available ALOS World 3D 30m (AW3D30) DEM, with a resolution of 1 arc-second (Tadono et al. Citation2016). We use the latest version available at the time of writing for each tile of interest (version 3.2 for one tile and 3.1 elsewhere).
2.3.3. TerraSAR-X add-on for Digital Elevation Measurement (TanDEM-X)
The TanDEM-X mission is a public-private partnership between the German Aerospace Centre (DLR) and Airbus Defence and Space, collecting X-band SAR data over the entire globe between December 2010 and January 2015. This initially resulted in two 0.4 arc-second DEMs - a commercial product (WorldDEM) developed by Airbus Defence and Space and the TanDEM-X DEM, available on request to researchers but not the general public (Rizzoli et al. Citation2017). However, the European Space Agency (ESA) have since released the Copernicus DEM, a resampling of the higher-resolution WorldDEM product which may benefit from the manual corrections applied there, especially the flattening of water bodies (Fahrland et al. Citation2022). Two resolutions are publicly-available: GLO-90 (3 arc-seconds) and GLO-30 (1 arc-second).
We evaluate here the 1 arc-second version (GLO-30, version v2022), which comes in two formats: DGED and DTED. These differ in precision (floating-point and integer, respectively) and longitudinal spatial resolution at high latitudes (above 50). To test the assumption that the higher precision offered by DGED translates into higher vertical accuracy, both formats are included in summary tables, with figures generally showing only the DGED format (for visual clarity).
The most recent addition to this group of publicly-available 1 arc-second DEMs is the Forest And Buildings Removed DEM (FABDEM), for which we assess version 1.2, released in January 2023 (Neal and Hawker Citation2023). This is based on the Copernicus DEM (GLO-30 DGED) but uses random forest models to correct the vertical errors associated with forests and buildings, with a reported halving of absolute errors in test sites (Hawker et al. Citation2022). The limited independent validations currently available report similarly impressive accuracy metrics (Marsh, Harder, and Pomeroy Citation2023), suggesting great potential for future flood modelling applications in data-scarce areas, noting that a licensing fee is required for any commercial applications.
2.4. Pre-processing
To enable direct comparisons, all datasets were pre-processed to achieve a common coordinate reference system and vertical datum. All of the DEMs use WGS84 for their horizontal coordinates, while elevations are provided with reference to either the EGM96 (SRTM, NASADEM, AW3D30) or EGM2008 (Copernicus DEM, FABDEM) geoid model. We selected the EGM2008 geoid for our evaluation, based on its superior accuracy to EGM96 (Pavlis et al. Citation2012). For any DEMs not originally using EGM2008, vertical datum shifts were applied using the dem_geoid function in the NASA Ames Stereo-Pipeline toolbox, version 3.1 (Beyer, Alexandrov, and McMichael Citation2018).
While all DEMs considered here are nominally 1 arc-second in resolution, the Copernicus DEMs switch to wider longitudinal grid spacing above latitudes of 50 (1.5 and 2.0 arc-seconds for DGED and DTED, respectively, with further changes at higher latitudes). To maintain a consistent resolution for our analysis, we resampled the Copernicus DEMs to 1 arc-second (using the bilinear method) for the three sites above 50
latitude.
The higher-resolution reference DTMs were then resampled (average method) to match the grid resolution and alignment of each DEM in turn, using GDAL command-line utilities, version 3.4.2 (GDAL/OGR contributors Citation2022) for the horizontal transformation (to WGS84) and the NASA Ames Stereo-Pipeline for vertical datum shifts (to EGM2008). This approach allowed the direct evaluation of each DEM in its native grid alignment, with the only modifications being either vertical datum shifts (applied to SRTM, NASADEM and AW3D30) or resampling to 1 arc-second resolution (applied to the Copernicus DEMs for the three sites above 50).
Before beginning our analysis, we applied two manual data checks and edits. The first was to manually exclude grid cells for which there were obvious topographical changes during the overall raw data capture period (2000–2015), using multi-temporal Google Earth imagery to identify these (primarily quarries and coastal cliffs), following the approach taken by Hawker, Neal, and Bates (Citation2019). Secondly, where buildings were identified in the reference DTMs (usually large warehouses presumably missed by the LiDAR processing algorithms), these were removed and then filled using Inverse Distance Weighting (IDW) interpolation from surrounding ground cells.
2.5. Error metrics
We define the vertical error in each DEM as its deviation from the bare ground surface described by the reference DTMs. This is evaluated on a cell-by-cell basis for all study sites, using the resampled version of each DTM (matching that particular DEM's grid alignment):
(1)
(1) where the error term (
) for each grid cell i is the difference between the elevation defined for that cell by the DEM (
) and the resampled reference DTM (
).
As well as visualising the statistical distributions of error values using histograms, we summarise them using a range of error metrics recommended in the literature. This enables easier comparisons between DEMs and with past studies, keeping in mind that all single-value metrics are simplifications and can be misleading if used in isolation (Hawker, Neal, and Bates Citation2019). Considering past DEM accuracy studies, the most commonly-used metrics include the Mean Error (ME), Mean Absolute Error (MAE), Standard Deviation (STD), and Root Mean Square Error (RMSE), as defined below.
(2)
(2)
(3)
(3)
(4)
(4)
(5)
(5)
However, these metrics all assume that errors follow a Gaussian (normal) distribution and have no significant outliers, even though this is rarely the case for DEMs (Gesch Citation2018). For alternative error metrics more robust to non-Gaussian distributions and the presence of outliers, we include three recommended by Höhle and Höhle (Citation2009): median error, Normalised Median Absolute Deviation (NMAD), and absolute deviation at the 95% percentile (LE95).
(6)
(6)
(7)
(7) where
denotes the median error value and
is a percentile of the absolute error values. The NMAD describes the spread of a distribution, similarly to STD but without that metric's sensitivity to outliers. In the case that errors do follow a Gaussian distribution (and sufficient samples are available), the NMAD will be identical to the STD (Höhle and Höhle Citation2009).
All metric values are provided in summary tables, with four shown in the figures to highlight different properties of the error distributions: median error (centre), NMAD (spread), RMSE (for comparison with past studies) and LE95 (tail errors).
2.6. Vertical accuracy assessment
Before assessing vertical accuracy, we applied a water mask to filter out DEM cells identified as ocean, as these are not relevant to terrestrial flood modelling and often subject to significant distortions, particularly for SAR-based DEMs (Wessel et al. Citation2018). For all DEMs, we used the Water Body Mask (WBM) rasters provided with the Copernicus DEM (GLO-30 DGED) (Fahrland et al. Citation2022).
2.6.1. Overall vertical accuracy
We assessed the overall accuracy of each DEM by pooling all available error values (across all study sites, with no filters applied), comparing their distributions (using histograms), and calculating the error metrics described in Section 2.5.
2.6.2. Vertical accuracy by land cover
To investigate how vertical error varies with land cover, we label each DEM grid cell with a land cover class using the European Space Agency (ESA) WorldCover 10m 2020 v100 product (Zanaga et al. Citation2021). This was selected for its high spatial resolution, a relatively small Minimum Mapping Unit (MMU) of 100 m (meaning each grid cell is labelled based on its own land cover, rather than evaluated in aggregate with neighbouring grid cells) and an independent validation confirming comparable accuracy with coarser-resolution options (Venter et al. Citation2022).
Of the 11 land cover classes available, two were excluded (‘Snow & ice’ and ‘Moss & lichen’), given negligible coverage within our study sites or flood-prone areas globally. The remaining classes are summarised in , along with the classifications used for the other analysis factors.
Table 2. Categorical classifications used for the primary analysis factors.
To focus on the significance of land cover, we first filtered out grid cells on steep slopes (for which large errors are expected), in an attempt to isolate the influence of land cover from that of slope. A threshold of 15 was applied for this, the same value used by Uuemaa et al. (Citation2020) for this purpose, which excluded 7.7% of our full error dataset.
2.6.3. Vertical accuracy by slope
In addition to land cover, slope is well known to have a significant influence on DEM error (Hawker, Neal, and Bates Citation2019). We derive rasters of slope (and aspect, covered in the following section) from the resampled reference DTMs, using the geodetic formulae of Florinsky (Citation2016) as implemented in the WhiteboxTools application, version 2.1 (Lindsay Citation2016). This avoids the need to reproject the DTMs first (e.g. to UTM), which can significantly alter their characteristics (Guth and Kane Citation2021).
To simplify the presentation of results, we reclassified slope into six unequal classes, allowing more detail for lower slopes (), and assessed the distribution of vertical errors in each class using histograms and error metrics.
2.6.4. Vertical accuracy by aspect
Aspect, the direction that a terrain slope faces, may also be associated with DEM errors, especially on steep slopes (Gdulová, Marešová, and Moudrý Citation2020). Most past studies assessing this have found at least a weak correlation (Szabó, Singh, and Szabó Citation2015; Uuemaa et al. Citation2020) but there is no clear consensus on the pattern expected for each DEM (Z. Liu et al. Citation2020). This may be due to the limited number of sites evaluated in each study and the stronger influence of varying land cover and slope distributions in each aspect direction. Where a clear pattern is observed, this is generally attributed to satellite orbit and sensor orientation, with foreslopes (slopes facing the sensor) expected to be more accurate than backslopes (Shortridge and Messina Citation2011; Toutin Citation2002).
Error dependency on aspect will be most apparent on steep slopes (Gdulová, Marešová, and Moudrý Citation2020), so we filtered out DEM cells with slope < 10, the same threshold used by Szabó, Singh, and Szabó (Citation2015) for this purpose. Reclassifying aspect into eight directions around the compass (), we found that distributions of land cover and slope within each of these aspect classes were quite different. Since land cover and slope are well known to significantly affect DEM errors, we addressed this class imbalance using a stratified sampling approach (with strata being land cover-slope class pairs). For each aspect class, we took repeated random samples, weighted so as to target the same overall distributions found in the full high-slope dataset. This was intended to correct for variations in error due to differing land cover and/or slope distributions in each direction, to see if any clear patterns remained that might be attributed to aspect. Further details are provided in Text S1 (Supplementary Material).
Some authors have suggested that any variation of vertical error with aspect is likely a result of horizontal geolocation errors (Nuth and Kääb Citation2011), rather than differing vegetation or terrain conditions (Z. Liu et al. Citation2020). Where that is the case, the relationship between aspect and vertical error (normalised by the tangent of the slope) would be expected to follow a cosine function, the parameters of which provide the direction and magnitude of the geolocation correction required (Nuth and Kääb Citation2011). Importantly, this relationship may be masked for DEM tiles derived by merging multiple scenes, each of which may have its own geolocation error (Guan et al. Citation2020), which could explain why error dependency on aspect is not always clear.
We assess this separately for each site, using only steep slopes (≥ 10) and excluding ‘Tree cover’ cells (given highly asymmetric error distributions that were found to bias results). For sites with a sufficient sample (at least 2,000 cells, spread across different aspect directions), we fit a cosine function to predict the slope-normalised error as a function of aspect, using the curve_fit function in the scipy Python package (version 1.9.3). Goodness-of-fit is evaluated by assessing each plot visually (see Figure S1, Supplementary Material, for an example) and testing whether the predicted slope-normalised error would enable a superior error correction than simply subtracting the mean slope-normalised error calculated for that site.
3. Results
3.1. Overall vertical accuracy
Distributions of the vertical errors calculated for each DEM (across all study sites) are visualised in , showing an overview (a) and then focusing on the centre (b) and the left (c) and right (d) tails. Above the overview plot (a), our four key error metrics are visualised with reference to the same x-axis, noting that only one of the GLO-30 formats is shown (indistinguishable at this scale). As expected by Höhle and Höhle (Citation2009), these DEM errors do not follow a Gaussian distribution (see Figure S2).
Figure 2. Density histograms of vertical error for each DEM: (a) overview plot, with selected metrics shown at the top and labels indicating the fraction of each distribution visible, (b) centre of the distribution, (c) left tail and (d) right tail.

presents overall error metric values, highlighting in each row the best (blue) and worst (red) performing DEMs for that metric. FABDEM consistently ranked first across all metrics, while AW3D30 was last for all but MAE and NMAD (for which SRTM errors were even higher).
Table 3. Overall error metrics for each DEM, highlighting the best (blue) and worst (red) DEM for each metric.
3.2. Vertical accuracy by land cover
shows histograms (left column) and error metrics (right column) for each DEM within each of the land cover classes considered (rows), limiting the analysis to slopes < 15 (to exclude the impact of very steep slopes on vertical errors). Metric values are given in Table S2.
3.3. Vertical accuracy by slope
visualises DEM errors (using histograms, with error metrics along the top) for each of the eight slope classes considered (a-h), noting that these provide more detail at the low-slope end. Steeper slopes are clearly associated with wider error distributions (a mix of positive and negative error values) and increasingly positive median errors. Metric values are provided in Table S3.
Figure 4. Density histograms and selected error metrics for all DEMs within each slope class (a-h), with labels indicating the sample size available within each slope class (for each DEM).

To assess and compare the sensitivity of each metric to slope (across DEMs), shows the change in error metric values as slope increases. For this analysis, each metric was evaluated over equal-interval slope ranges (2.5) to see more clearly the structural form of these relationships (linear or otherwise).
3.4. Vertical accuracy by aspect
Based on the probabilistic stratified sampling approach outlined in Section 2.6.4, shows the mean error metrics calculated in each aspect direction across all sites, split by hemisphere to highlight the contrasting patterns observed for AW3D30. These results suggest that vertical errors do vary by aspect, although the range of this variation is significantly lower than for land cover or slope.
Figure 6. Mean error metrics by aspect direction, for sites in the northern (left column) and southern (right column) hemispheres.

To explore whether this variation in vertical error by aspect might be due to horizontal geolocation errors, summarises for each DEM the distribution of geolocation corrections estimated (for sites where this was possible), following the approach outlined in Section 2.6.4. Radial histograms (a-e) show the number of corrections estimated in each direction for each DEM (colours indicate correction magnitude) and the strip plot (f) shows distributions of correction magnitudes. (Note that a map showing the spatial distribution of these estimated geolocation corrections is provided in Figure S3.)
4. Discussion
4.1. Overall vertical accuracy
Considering the overall error distributions (), the most striking result is the difference between the DEMs derived from older satellite missions (SRTM, AW3D30, NASADEM) and the more recent products based on the TanDEM-X mission (GLO-30 DGED, GLO-30 DTED and FABDEM). The latter group show much narrower distributions (e.g. the NMAD for GLO-30 DGED is 1.27 m, compared with 3.65 m for SRTM) and are centred more closely on zero error (e.g. median error is 0.21 m for GLO-30 DGED, compared with 1.65 m for SRTM).
As expected, the DGED format (floating-point precision) of GLO-30 seems superior to the DTED format (integer precision), especially in the centre of the distributions (where DGED is slightly narrower), although distribution tails are very similar. In both formats, the GLO-30 DEMs have a relatively high proportion of large positive errors (see (d)), most of which are found in densely-vegetated areas. This is reflected in high LE95 values, underscoring the limitations of short-wavelength X-band radar in penetrating vegetation canopies to record the ground surface (Schlund et al. Citation2019).
While some past studies preferred AW3D30 over SRTM (Jain et al. Citation2018; Uuemaa et al. Citation2020), we find that AW3D30 has the largest errors (judged by median error, RMSE and LE95) of all DEMs considered here, albeit by a small margin. This slight underperformance compared with the other DEMs from older missions (SRTM and NASADEM) seems to be due primarily to a higher fraction of large positive errors. Considering errors m, we find they make up 3.6% of AW3D30 grid cells across our study sites, compared with only 2.0% and 1.5% for SRTM and NASADEM, respectively.
Two of the DEMs assessed here might be considered ‘improved’ versions of another, albeit in different ways: NASADEM is based on a re-processing of the SRTM radar data (Crippen et al. Citation2016), while FABDEM used machine learning to address vertical errors in GLO-30 DGED (Hawker et al. Citation2022). In both cases, the newer DEMs do appear superior to their sources, based on error metrics and a visual comparison of their error distributions. NASADEM shows a more symmetrical distribution centred closer to zero, while FABDEM has significantly reduced the large positive errors found in GLO-30. In both cases however (especially for FABDEM), this is at the expense of increasing the distribution of large negative errors, which will have implications when these DEMs are used for flood modelling. A detailed comparison is shown in Figure S4.
Given the scarcity of open-access LiDAR-derived DTMs, some used as reference data here were also used by Hawker et al. (Citation2022) to train the FABDEM correction model, biasing evaluations of its performance. For a more rigorous assessment, we divide our reference sites into those seen (13) versus unseen (52) during FABDEM training and then evaluate the change in error metric values (from GLO-30 DGED to FABDEM) for each set. As shown in Figure S5, the percentage change in metric values for the unseen sites (b) is comparable -- and in some cases, superior -- to the changes for the sites seen during training (a). This suggests that the FABDEM correction model generalises well to new application areas.
4.2. Vertical accuracy by land cover
Land cover has a significant effect on vertical errors, as evident from the wide variation in error histograms (by land cover class) shown in . This is likely a function of the height of elevated surfaces (e.g. tree canopies and building rooftops), the density of vegetation or built infrastructure, and the differing penetration capability of each satellite sensor. For example, X-band radar (GLO-30) uses a shorter wavelength than C-band radar (SRTM, NASADEM), meaning it penetrates less deeply into vegetation canopies (Schlund et al. Citation2019), resulting in higher vertical errors in thickly-vegetated areas (Wessel et al. Citation2018).
The land cover classes evaluated in this study are ‘Tree cover’, ‘Shrubland’, ‘Grassland’, ‘Cropland’, ‘Built-up’, ‘Bare/sparse vegetation’, ‘Water bodies’, ‘Herbaceous wetland’ and ‘Mangroves’. Of these, ‘Tree cover’ is clearly the most significant driver of DEM error, with the highest error metrics out of all land cover classes, across all DEMs (with the single exception of FABDEM's median error, for which the ‘Mangroves’ class is slightly higher). Errors under ‘Tree cover’ tend to have relatively wide distributions, with a significant positive bias and long right tails.
Next in significance is ‘Mangroves’, another type of tree cover, with the second highest RMSE and LE95 values across all DEMs. Error distributions here highlight the significance of numerical precision. Given that reference ground elevations in this land cover class are clustered closely around zero (being coastal environments), the impact of rounding for the integer-precision DEMs (SRTM, NASADEM, AW3D30, with GLO-30 DTED not shown) is visible as multimodal peaks around each metre value, rather than a continuous distribution (as seen for the floating-point precision DEMs: GLO-30 DGED and FABDEM).
Errors within ‘Built-up’ areas are generally high, although this varies significantly by DEM, with FABDEM least impacted (LE95 2.61 m) and AW3D30 most (LE95 7.42 m). ‘Water bodies’ show similar median error and RMSE values (with even higher LE95 values), although these distributions are much noisier than for other land cover classes. This is likely due to the temporal variation in river/lake water levels (Li et al. Citation2022) and low signal coherence for the SAR-based DEMs (Wendleder et al. Citation2013), potentially exacerbated by the relatively small sample sizes available for this class.
Past studies have found that water bodies were especially problematic for the TanDEM-X DEMs (Kramm and Hoffmeister Citation2021). However, GLO-30 (based on the same raw data) does not appear to suffer from these distortions, with the lowest RMSE and LE95 values of all DEMs. This is likely due to the hydro-enforcement (flattening of water bodies and ensuring elevations along rivers consistently slope downstream) performed for the commercial WorldDEM product, from which GLO-30 is derived (Fahrland et al. Citation2022).
Error metrics for ‘Grassland’ and ‘Cropland’ are similar, with the latter slightly lower across all DEMs, perhaps due to the relative homogeneity of agricultural land, whereas natural grasslands will contain the occasional tree or shrub, biasing DEM elevations higher.
Aside from the multimodal error distribution in ‘Mangroves’ (discussed above), distributions within the other land cover classes are generally unimodal, with the exception of ‘Shrubland’ and ‘Herbaceous wetland’, for which GLO-30 DGED and FABDEM are distinctly bimodal. Hawker, Neal, and Bates (Citation2019) noticed a similar pattern for the 3 arc-second TanDEM-X (same raw data source) within short vegetation zones. This likely reflects the diverse vegetation types grouped within each of the high-level classifications used in global land cover maps.
FABDEM's focus on improving vertical errors due to forests and buildings is clear, showing significant changes in distribution (compared with GLO-30 DGED) within the ‘Tree cover’, ‘Mangroves’ and ‘Built-up’ classes especially. Error distributions in other classes remain similar.
4.3. Vertical accuracy by slope
Steeper slopes are clearly associated with higher vertical errors (), showing wider error distributions and increasingly positive median errors. However, assessing the direct impact of slope is complicated given that ‘Tree cover’ fraction increases consistently with slope, making it hard to disentangle the impact of forest canopies from that of the slope in itself. We speculate that the rightward shift in median error is likely due to increasing forest cover, while the wider error spreads may be directly attributed to slope. This is likely due to geometric distortions in satellite imagery inputs (which make it harder to match stereo images) and/or horizontal offset errors (which have only minor impacts in flat areas but are much more significant on steep slopes), as suggested by Li et al. (Citation2022).
This strong association between slope and vertical errors has been well documented before; more interesting is to compare the rates at which error metrics change with slope (). For most DEMs, we found a fairly linear relationship between slope and error metric until 30–35, after which the rate of change increases (except for median errors, which plateau). AW3D30 differs in that the relationship between slope and error metric continues to be roughly linear even for the steepest slopes evaluated here, as found by Guan et al. (Citation2020). This is especially noteworthy for the LE95 metric, which increases dramatically for other DEMs above slopes of 40
(particularly for FABDEM) but continues on the same linear trend for AW3D30.
Focusing on lower slopes (of most relevance to floodplain modelling), FABDEM shows not only the lowest vertical errors but also the least sensitivity to changes in slope (i.e. the flattest lines in ). Up to 30–35, median errors remain very close to zero and very large errors (as indicated by LE95) increase at a slower rate than for other DEMs (with the caveat that they increase dramatically for slopes above 35
).
4.4. Vertical accuracy by aspect
Looking at the variation in error metric values across the eight aspect direction classes considered (), we found evidence of a small but consistent pattern for each DEM. For large error metrics (RMSE and LE95), SRTM and NASADEM show higher errors on south-facing slopes and lower errors on north-facing slopes, regardless of hemisphere. This is similar to the pattern found by Uuemaa et al. (Citation2020) but differs from most other past studies, which have generally found higher SRTM errors on slopes facing north (Carrera-Hernández Citation2021; Szabó, Singh, and Szabó Citation2015) or north-west (Hawker, Neal, and Bates Citation2019; Shortridge and Messina Citation2011). This divergence may be a function of sample size, with most studies evaluating a relatively small collection of sites (usually in the same geographical region) or reflect biases present in earlier SRTM versions.
Past studies assessing AW3D30 have generally found little to no error variation by aspect (Z. Liu et al. Citation2020; Uuemaa et al. Citation2020), but our results suggest a relatively strong dependency if study sites in each hemisphere are assessed separately. For sites in the northern hemisphere, errors are highest to the east or south-east, while in the southern hemisphere, slopes facing north tend to have the highest errors. We note that this contrasting pattern is not observed for the SAR-based DEMs, and speculate that it may relate to the photogrammetry matching process and solar illumination, perhaps in terms of differing horizontal offset errors by solar incidence angle.
We found relatively low variation in GLO-30 (and, by extension, FABDEM) error with aspect, except that large error metrics (RMSE and LE95) are consistently lowest on north/north-west facing slopes. There is also weak evidence for slightly higher errors on east-facing slopes in the northern hemisphere and west-facing slopes in the southern hemisphere, which would correspond to backslopes during the initial TanDEM-X data collection period (Rizzoli et al. Citation2017). This is seen most clearly for FABDEM, perhaps because errors relating to land cover have been so effectively reduced, leaving terrain-based errors more exposed. While the literature is currently limited on this, we note that our results are consistent with the higher errors on east-facing slopes reported by Marsh, Harder, and Pomeroy (Citation2023) for a northern hemisphere site.
When we assessed the possibility that vertical error dependency on aspect was primarily a function of geolocation errors (), we found clearest evidence of this for AW3D30 and GLO-30. AW3D30 had both the highest number of sites where an offset was evident (27) and the highest mean offset magnitude (5.6 m). Required corrections were predominantly to the south-east or east, except for sites in New Zealand and Melanesia, where a northward shift was indicated (see Figure S3). These make up most of the steep-slope southern hemisphere sites evaluated in this study, so it is unclear whether the differing error profiles seen for AW3D30 in actually relate to hemisphere or a more specific, regional issue. As for GLO-30, the geolocation corrections estimated were more consistent in direction (mostly to the south or south-west) but smaller than for other DEMs (mean magnitude 3.1 m).
While a full investigation of the impact of applying these geolocation corrections to each DEM for each relevant site is beyond the scope of this study, we did so for one site in the Canary Islands (a suitable test site, given mostly steep slopes and minimal tree cover). This resulted in significant improvements to error metrics (see Figure S6), particularly for steep slopes (≥10), where vertical errors showed lower variation (NMAD reduced by 35% for AW3D30 and 41% for GLO-30) and lower large error metrics (LE95 reduced by 13% for AW3D30 and 33% for GLO-30). This preliminary result suggests that geolocation errors may be a significant source of vertical errors for some DEMs, noting that this will vary dependent on the individual scenes merged for each DEM tile.
4.5. Which is more significant -- land cover or slope?
Past studies have consistently focused on land cover and slope as the two main factors explaining vertical DEM errors (Magruder, Neuenschwander, and Klotz Citation2021). However, there is no consensus as to which is most important, which would help DEM users identify the most error-prone areas in a given study site. Some studies found that land cover is the dominant factor (Li et al. Citation2022), while others suggest it is slope (Carrera-Hernández Citation2021; K. Liu et al. Citation2019; Uuemaa et al. Citation2020). This divergence may be due to the limited sites considered in each study, corresponding to small subsets of the full range of land cover and slope combinations possible. The relatively large and diverse reference dataset collated here allows a more comprehensive assessment across these combinations.
Following Li et al. (Citation2022), we begin by evaluating the variation in each error metric with slope, for individual land cover classes. As an example, shows how RMSE varies with slope for six land cover classes (with others excluded given very little variation in slope). This allows a direct comparison of the influence of slope (within each subplot, how much does RMSE rise as slope increases?) versus land cover (for a given slope class, across all subplots, what is the variation in RMSE?). Taking SRTM as an example, we find that RMSE varies by up to 5.4 m by slope (within ‘Bare/sparse vegetation’ land cover) compared with 6.2 m by land cover (between the ‘Tree cover’ and ‘Shrubland’ classes, within the highest slope class).
Figure 8. Comparison of RMSE variation with slope (within each subplot) and land cover (across all subplots, for each slope class), for the six land cover classes for which significant variation in slope was found.

Extending this approach, we assess for each error metric its variation by slope and by land cover class. summarises these results, presenting for each metric (a-d) both the mean of these assessed range values and the maximum range observed, with some clear patterns evident. Firstly, land cover is generally more important than slope, when assessed by median error (all DEMs), LE95 (all DEMs) or RMSE (all but NASADEM). Secondly, GLO-30 is the DEM most impacted by land cover (across all metrics), likely reflecting the limited penetration capacity of its X-band radar. Thirdly, GLO-30, FABDEM and AW3D30 are the least impacted by slope, likely due to their derivation from higher-resolution DEMs which capture elevations on steep slopes more accurately, even when resampled to the same resolution as the other DEMs (Courty, Soriano-Monzalvo, and Pedrozo-Acuña Citation2019).
4.6. Most accurate DEM by land cover and slope
For many DEM users, the main question is which product is ‘most accurate’ for their particular application site. This is a nuanced question, which can be assessed in different ways using the results presented above, but we also try to summarise DEM performance as succinctly as possible, according to the two factors found to influence it most (land cover and slope).
considers all combinations of land cover (x-axis) and slope (y-axis) class for which data were available, indicating the best-performing DEM for each according to our four key metrics. Two versions of this performance grid are presented: (a) including FABDEM, openly available for download but requiring a licensing fee for commercial applications, and (b) excluding FABDEM, so as to show only DEMs free in terms of both access and cost.
Figure 10. Performance summary grids, indicating the best-performing DEM by land cover and slope class (where available) by the four key metrics, considering (a) all DEMs, and (b) excluding FABDEM (which requires a licensing fee for commercial applications).

Based on this comparison, FABDEM is generally the best-performing DEM, especially under ‘Tree cover’ and ‘Mangroves’ (with forests one of their priorities for correction) and in low-slope ‘Built-up’ areas. Interestingly, it is often outperformed by GLO-30 (its source DEM) in steeper slopes (except under ‘Tree cover’, where it is consistently most accurate, across all metrics). This is likely due to the smoothing filters applied during the post-processing of FABDEM, intended to reduce over-corrections and filter out noise.
If FABDEM is not an option (given its licensing fee for commercial applications), GLO-30 is the next best option under all land covers except for ‘Tree cover’, where NASADEM is more accurate across most metrics. While most error metrics are similar for the two GLO-30 formats, DGED (floating-point precision) should generally be preferred, given the quantisation effect of the integer-precision DTED format on derived variables such as slope (Evans and Cox Citation1999).
4.7. Limitations
We have not considered the potential impact of temporal variations amongst the DEMs, reference DTMs and analysis datasets (e.g. land cover) used here, aside from manually identifying and excluding locations where significant topographical changes are likely (quarries and coastal cliffs). However, this temporal variation may be significant, in terms of when each dataset was collected (with potential mismatches in surface conditions) and the duration of data collection (relevant to seasonal variation). As Li et al. (Citation2022) point out, this is likely to be especially significant over the more variable land cover classes, such as wetlands, water bodies and developing urban areas. Furthermore, most of our reference DTMs are relatively recent (e.g. more than half date from 2018 or later), biasing our accuracy assessment towards DEMs developed using more recently-collected data (especially GLO-30 and, by extension, FABDEM).
During the development of each DEM, elevations from other DEMs available at the time are used to fill voids, such that each is a composite product. We have simply evaluated each DEM as is, rather than attempting to account for the provenance of each grid cell or restricting our analysis to the ‘native’ data in each DEM.
Finally, we have not evaluated the potential implications of the raster processing steps required to convert all DEMs and reference DTMs to a common spatial reference system. Vertical datum shifts may be the most significant of these, often relying on relatively coarse regional or global geoid models to estimate the shift to be applied to each grid cell, introducing uncertainty which we have not attempted to quantify.
5. Conclusions
Regional-scale flood models often rely on topographic data from one of the global DEMs, despite known vertical inaccuracies resulting from the limited ability of spaceborne sensors to capture the true ground surface or precisely geolocate imagery. In this study, we assessed the vertical accuracy of five contemporary DEMs using a diverse collection of high-accuracy reference datasets (derived from airborne LiDAR surveys), selected to represent the biophysical variations in flood-prone areas globally. The best-performing DEMs were those derived from the most recent mission (TanDEM-X): the Copernicus DEM GLO-30 DGED (although the short wavelength X-band radar struggled to penetrate tree canopies) and FABDEM (which used random forest models to predict and correct vertical biases associated with forests and buildings in GLO-30 DGED). However, performance varied somewhat depending on the land cover, terrain slope and error metric used, such that the ‘best’ DEM for a given application will depend on the local biophysical conditions and the metric(s) of particular relevance.
We found land cover to be the most significant factor influencing vertical errors, with tree cover especially problematic for all DEMs, although those derived from longer wavelength C-band radar (SRTM and NASADEM) seemed better able to penetrate vegetation canopies. Slope was clearly associated with higher errors too, especially in terms of wider spreads (due to a mix of positive and negative deviations, whereas errors due to land cover tended to have a positive bias). Sensitivity to slope varied by DEM though, with those derived from higher-resolution products (AW3D30, GLO-30 and FABDEM) found to be less sensitive. The influence of terrain aspect on error is less consistent and may be a function of the varying geolocation errors affecting the individual scenes merged to produce a given DEM tile. Preliminary results suggest that AW3D30 and GLO-30 in particular may be subject to systematic (but sub-pixel) offsets that could be corrected in future versions. However, this should be evaluated using a larger collection of steep slope sites, since our reference data are biased towards the lower slopes found in flood-prone areas.
In conclusion, we found FABDEM to be the most accurate DEM overall (especially for forests and low-slope terrain), suggesting that its error correction methodology is effective at reducing large positive errors in particular and that it generalises well to new application sites. Where FABDEM is not an option (given licensing costs for commercial applications), GLO-30 DGED is the clear runner-up under most conditions, with the exception of forests, where NASADEM (re-processed SRTM data) is more accurate. Our results suggest that these newer DEMs should be the preferred inputs to future regional-scale flood models, although further assessments are needed with regards to hydrological derivatives (e.g. stream networks and catchment delineations) and simulated flood hazards (e.g. inundation depths and extents).
Supplemental Material
Download PDF (1.5 MB)Acknowledgments
For their help in acquiring and understanding the airborne-LiDAR DTMs used here as reference data, we are very grateful to Tristan Goulden, Nicholas Rollings, Lara Röttcher, Karim Sadr, Gregoire Vincent, Joe Mulligan, Debora Drucker, Chris Crook, Serene Ho, Rosario Ang and Tanel Hurt. Michael Meadows was supported by a RTP Stipend Scholarship from the Australian Government and a Postgraduate Research Scholarship from Natural Hazards Research Australia (NHRA).
Disclosure statement
No potential conflict of interest was reported by the author(s).
Data availability statement
The global DEMs assessed here are freely available online: SRTM from https://lpdaac.usgs.gov/products/srtmgl1v003, NASADEM from https://lpdaac.usgs.gov/products/nasadem_hgtv001, AW3D30 from https://www.eorc.jaxa.jp/ALOS/en/dataset/aw3d30/aw3d30_e.htm, GLO-30 from https://panda.copernicus.eu/web/cds-catalogue/panda, and FABDEM from https://data.bris.ac.uk/data/dataset/s5hqmjcdj8yo2ibzi9b4ew3sn. We used the Google Earth Engine Catalog to access both the MERIT DEM (https://developers.google.com/earth-engine/datasets/catalog/MERIT_DEM_v1_0_3) and the Surface Water Occurrence layer (https://developers.google.com/earth-engine/datasets/catalog/JRC_GSW1_3_GlobalSurfaceWater). The datasets used to indicate flood-prone areas are both available online: GFPLAIN250m at https://doi.org/10.6084/m9.figshare.6665165.v1 and the Low Elevation Coastal Zone (LECZ) raster at https://doi.org/10.7927/d1x1-d702. In addition to the MERIT DEM (used to derive slope), the other datasets used to evaluate the biophysical conditions in flood-prone areas globally are ESA WorldCover 2020 (https://worldcover2020.esa.int/downloader), present-day Köppen–Geiger zones (https://www.gloh2o.org/koppen) and GHSL Degree of Urbanisation rasters (https://data.jrc.ec.europa.eu/dataset/4606d58a-dc08-463c-86a9-d49ef461c47f). All (65) reference DTMs collated for this study are summarised in Table S1, including online access details wherever available.
Correction Statement
This article has been corrected with minor changes. These changes do not impact the academic content of the article.
References
- Arnell, Nigel W., and Simon N. Gosling. 2016. “The Impacts of Climate Change on River Flood Risk At the Global Scale.” Climatic Change 134 (3): 387–401. https://doi.org/10.1007/s10584-014-1084-5.
- Beck, Hylke E., Niklaus E. Zimmermann, Tim R. McVicar, Noemi Vergopolan, Alexis Berg, and Eric F. Wood. 2018. “Present and Future Köppen–Geiger Climate Classification Maps At 1-km Resolution.” Scientific Data 5 (1): 180214. https://doi.org/10.1038/sdata.2018.214.
- Beyer, Ross A., Oleg Alexandrov, and Scott McMichael. 2018. “The Ames Stereo Pipeline: NASA's Open Source Software for Deriving and Processing Terrain Data.” Earth and Space Science 5 (9): 537–548. https://doi.org/10.1029/2018EA000409.
- Carrera-Hernández, J. J. 2021. “Not All DEMs Are Equal: An Evaluation of Six Globally Available 30 m Resolution DEMs with Geodetic Benchmarks and LiDAR in Mexico.” Remote Sensing of Environment 261:112474. https://doi.org/10.1016/j.rse.2021.112474.
- Ciampalini, Andrea, Federico Raspini, William Frodella, Federica Bardi, Silvia Bianchini, and Sandro Moretti. 2016. “The Effectiveness of High-Resolution LiDAR Data Combined with PSInSAR Data in Landslide Study.” Landslides 13 (2): 399–410. https://doi.org/10.1007/s10346-015-0663-5.
- Courty, Laurent Guillaume, Julio César Soriano-Monzalvo, and Adrián Pedrozo-Acuña. 2019. “Evaluation of Open-Access Global Digital Elevation Models (AW3D30, SRTM, and ASTER) for Flood Modelling Purposes.” Journal of Flood Risk Management 12 (S1): e12550. https://doi.org/10.1111/jfr3.12550.
- Crippen, R., S. Buckley, P. Agram, E. Belz, E. Gurrola, S. Hensley, M. Kobrick. 2016. “NASADEM Global Elevation Model: Methods and Progress.” ISPRS-International Archives of the Photogrammetry, Remote Sensing and Spatial Information Sciences XLI-B4:125–128. https://doi.org/10.5194/isprsarchives-XLI-B4-125-2016.
- Dubayah, Ralph, James Bryan Blair, Scott Goetz, Lola Fatoyinbo, Matthew Hansen, Sean Healey, Michelle Hofton. 2020. “The Global Ecosystem Dynamics Investigation: High-Resolution Laser Ranging of the Earth's Forests and Topography.” Science of Remote Sensing 1:100002. https://doi.org/10.1016/j.srs.2020.100002.
- Evans, I. S., and N. J. Cox. 1999. “Relations Between Land Surface Properties: Altitude, Slope and Curvature.” In Process Modelling and Landform Evolution, edited by S. Hergarten and H. J. Neugebauer, Vol. 78 of Lecture Notes in Earth Sciences, 13–45. Berlin, Germany: Springer.
- Fahrland, Ernest, Hanne Paschko, Philipp Jacob, and Hanjo Kahabka. 2022. Copernicus Digital Elevation Model: Product Handbook. Technical Report AO/1-9422/18/I-LG. Potsdam, Germany: Airbus Defence and Space GmbH.
- Farr, Tom G., Paul A. Rosen, Edward Caro, Robert Crippen, Riley Duren, Scott Hensley, Michael Kobrick. 2007. “The Shuttle Radar Topography Mission.” Reviews of Geophysics 45 (2). https://doi.org/10.1029/2005RG000183.
- Florinsky, Igor V. 2016. Digital Terrain Analysis in Soil Science and Geology. 2nd Edition. London, UK: Elsevier.
- Ford, Alistair, Stuart Barr, Richard Dawson, James Virgo, Michael Batty, and Jim Hall. 2019. “A Multi-Scale Urban Integrated Assessment Framework for Climate Change Studies: A Flooding Application.” Computers, Environment and Urban Systems 75:229–243. https://doi.org/10.1016/j.compenvurbsys.2019.02.005.
- Garrote, Julio. 2022. “Free Global DEMs and Flood Modelling—A Comparison Analysis for the January 2015 Flooding Event in Mocuba City (Mozambique).” Water 14 (2): 176. https://doi.org/10.3390/w14020176.
- GDAL/OGR contributors. 2022. GDAL/OGR Geospatial Data Abstraction Software Library. Technical Report. Open Source Geospatial Foundation.
- Gdulová, Kateřina, Jana Marešová, and Vítězslav Moudrý. 2020. “Accuracy Assessment of the Global TanDEM-X Digital Elevation Model in a Mountain Environment.” Remote Sensing of Environment 241:111724. https://doi.org/10.1016/j.rse.2020.111724.
- Gesch, Dean B.. 2018. “Best Practices for Elevation-Based Assessments of Sea-Level Rise and Coastal Flooding Exposure.” Frontiers in Earth Science 6:230. https://doi.org/10.3389/feart.2018.00230.
- Gorelick, Noel, Matt Hancher, Mike Dixon, Simon Ilyushchenko, David Thau, and Rebecca Moore. 2017. “Google Earth Engine: Planetary-Scale Geospatial Analysis for Everyone.” Remote Sensing of Environment 202:18–27. https://doi.org/10.1016/j.rse.2017.06.031.
- Guan, Liyi, Hongbo Pan, Siyuan Zou, Jun Hu, Xiaoyong Zhu, and Ping Zhou. 2020. “The Impact of Horizontal Errors on the Accuracy of Freely Available Digital Elevation Models (DEMs).” International Journal of Remote Sensing 41 (19): 7383–7399. https://doi.org/10.1080/01431161.2020.1759840.
- Guth, Peter, and Tera Geoffroy. 2021. “LiDAR Point Cloud and ICESat-2 Evaluation of 1 Second Global Digital Elevation Models: Copernicus Wins.” Transactions in GIS 25 (5): 2245–2261. https://doi.org/10.1111/tgis.12825.
- Guth, Peter, and Morgan Kane. 2021. “Slope, Aspect, and Hillshade Algorithms for Non-Square Digital Elevation Models.” Transactions in GIS 25 (5): 2309–2332. https://doi.org/10.1111/tgis.12852.
- Guth, Peter L., Adriaan Van Niekerk, Carlos H. Grohmann, Jan-Peter Muller, Laurence Hawker, Igor V. Florinsky, Dean Gesch. 2021. “Digital Elevation Models: Terminology and Definitions.” Remote Sensing 13 (18): 3581. https://doi.org/10.3390/rs13183581.
- Hancock, Steven, Ciara McGrath, Christopher Lowe, Ian Davenport, and Iain Woodhouse. 2021. “Requirements for a Global Lidar System: Spaceborne Lidar with Wall-to-Wall Coverage.” Royal Society Open Science 8 (12): 211166. https://doi.org/10.1098/rsos.211166.
- Hawker, Laurence, Paul Bates, Jeffrey Neal, and Jonathan Rougier. 2018. “Perspectives on Digital Elevation Model (DEM) Simulation for Flood Modeling in the Absence of a High-Accuracy Open Access Global DEM.” Frontiers in Earth Science 6:233. https://doi.org/10.3389/feart.2018.00233.
- Hawker, Laurence, Jeffrey Neal, and Paul Bates. 2019. “Accuracy Assessment of the TanDEM-X 90 Digital Elevation Model for Selected Floodplain Sites.” Remote Sensing of Environment 232 (111319): 111319. https://doi.org/10.1016/j.rse.2019.111319.
- Hawker, Laurence, Peter Uhe, Luntadila Paulo, Jeison Sosa, James Savage, Christopher Sampson, and Jeffrey Neal. 2022. “A 30 m Global Map of Elevation with Forests and Buildings Removed.” Environmental Research Letters 17 (2): 024016. https://doi.org/10.1088/1748-9326/ac4d4f.
- Hirt, C., M. S. Filmer, and W. E. Featherstone. 2010. “Comparison and Validation of the Recent Freely Available ASTER-GDEM Ver1, SRTM Ver4.1 and GEODATA DEM-9S Ver3 Digital Elevation Models Over Australia.” Australian Journal of Earth Sciences 57 (3): 337–347. https://doi.org/10.1080/08120091003677553.
- Hodgson, Michael E., and Patrick Bresnahan. 2004. “Accuracy of Airborne Lidar-Derived Elevation.” Photogrammetric Engineering & Remote Sensing 70 (3): 331–339. https://doi.org/10.14358/PERS.70.3.331.
- Höhle, Joachim, and Michael Höhle. 2009. “Accuracy Assessment of Digital Elevation Models by Means of Robust Statistical Methods.” ISPRS Journal of Photogrammetry and Remote Sensing 64 (4): 398–406. https://doi.org/10.1016/j.isprsjprs.2009.02.003.
- Hong, Danfeng, Lianru Gao, Naoto Yokoya, Jing Yao, Jocelyn Chanussot, Qian Du, and Bing Zhang. 2021. “More Diverse Means Better: Multimodal Deep Learning Meets Remote-Sensing Imagery Classification.” IEEE Transactions on Geoscience and Remote Sensing 59 (5): 4340–4354. https://doi.org/10.1109/TGRS.2020.3016820.
- Hong, Danfeng, Bing Zhang, Hao Li, Yuxuan Li, Jing Yao, Chenyu Li, Martin Werner, et al. 2023. “Cross-City Matters: A Multimodal Remote Sensing Benchmark Dataset for Cross-City Semantic Segmentation Using High-Resolution Domain Adaptation Networks.” Remote Sensing of Environment 299:113856. https://doi.org/10.1016/j.rse.2023.113856.
- Horritt, M. S., and P. D. Bates. 2002. “Evaluation of 1D and 2D Numerical Models for Predicting River Flood Inundation.” Journal of Hydrology 268 (1–4): 87–99. https://doi.org/10.1016/S0022-1694(02)00121-X.
- Jain, Akshay O., Tejaskumar Thaker, Ashish Chaurasia, Parth Patel, and Anupam Kumar Singh. 2018. “Vertical Accuracy Evaluation of SRTM-GL1, GDEM-V2, AW3D30 and CartoDEM-V3.1 of 30-m Resolution with Dual Frequency GNSS for Lower Tapi Basin India.” Geocarto International 33 (11): 1237–1256. https://doi.org/10.1080/10106049.2017.1343392.
- Kramm, Tanja, and Dirk Hoffmeister. 2021. “Comprehensive Vertical Accuracy Analysis of Freely Available DEMs for Different Landscape Types of the Rur Catchment, Germany.” Geocarto International 1–26. https://doi.org/10.1080/10106049.2021.1984588.
- Kulp, Scott A., and Benjamin H. Strauss. 2019. “New Elevation Data Triple Estimates of Global Vulnerability to Sea-Level Rise and Coastal Flooding.” Nature Communications 10 (1): 4844. https://doi.org/10.1038/s41467-019-12808-z.
- Laudon, Hjalmar, Martin Berggren, Anneli Ågren, Ishi Buffam, Kevin Bishop, Thomas Grabs, Mats Jansson, and Stephan Köhler. 2011. “Patterns and Dynamics of Dissolved Organic Carbon (DOC) in Boreal Streams: The Role of Processes, Connectivity, and Scaling.” Ecosystems 14 (6): 880–893. https://doi.org/10.1007/s10021-011-9452-8.
- Li, Hui, Jiayang Zhao, Bingqi Yan, Linwei Yue, and Lunche Wang. 2022. “Global DEMs Vary From One to Another: An Evaluation of Newly Released Copernicus, NASA and AW3D30 DEM on Selected Terrains of China Using ICESat-2 Altimetry Data.” International Journal of Digital Earth 15 (1): 1149–1168. https://doi.org/10.1080/17538947.2022.2094002.
- Lindsay, J. B.. 2016. “Whitebox GAT: A Case Study in Geomorphometric Analysis.” Computers & Geosciences 95:75–84. https://doi.org/10.1016/j.cageo.2016.07.003.
- Liu, Kai, Chunqiao Song, Linghong Ke, Ling Jiang, Yuanyuan Pan, and Ronghua Ma. 2019. “Global Open-Access DEM Performances in Earth's Most Rugged Region High Mountain Asia: A Multi-Level Assessment.” Geomorphology 338:16–26. https://doi.org/10.1016/j.geomorph.2019.04.012.
- Liu, Zhiwei, Jianjun Zhu, Haiqiang Fu, Cui Zhou, and Tingying Zuo. 2020. “Evaluation of the Vertical Accuracy of Open Global DEMs Over Steep Terrain Regions Using ICESat Data: A Case Study Over Hunan Province, China.” Sensors 20 (17): 4865. https://doi.org/10.3390/s20174865.
- MacManus, Kytt, Deborah Balk, Hasim Engin, Gordon McGranahan, and Rya Inman. 2021. “Estimating Population and Urban Areas At Risk of Coastal Hazards, 1990–2015: How Data Choices Matter.” Earth System Science Data 13 (12): 5747–5801. https://doi.org/10.5194/essd-13-5747-2021.
- Magruder, Lori, Amy Neuenschwander, and Brad Klotz. 2021. “Digital Terrain Model Elevation Corrections Using Space-Based Imagery and ICESat-2 Laser Altimetry.” Remote Sensing of Environment 264:112621. https://doi.org/10.1016/j.rse.2021.112621.
- Marsh, Christopher B., Phillip Harder, and John W. Pomeroy. 2023. “Validation of FABDEM, a Global Bare-Earth Elevation Model, Against UAV-Lidar Derived Elevation in a Complex Forested Mountain Catchment.” Environmental Research Communications 5 (3): 031009. https://doi.org/10.1088/2515-7620/acc56d.
- Maune, David F. ed. 2007. Digital Elevation Model Technologies and Applications: The DEM Users Manual. 2nd ed. Bethesda, Md: American Society for Photogrammetry and Remote Sensing.
- Meadows, Michael, and Matthew Wilson. 2021. “A Comparison of Machine Learning Approaches to Improve Free Topography Data for Flood Modelling.” Remote Sensing 13 (2): 275. https://doi.org/10.3390/rs13020275.
- Mesa-Mingorance, José L., and Francisco J. Ariza-López. 2020. “Accuracy Assessment of Digital Elevation Models (DEMs): A Critical Review of Practices of the Past Three Decades.” Remote Sensing12 (16): 2630. https://doi.org/10.3390/rs12162630.
- Moudrý, Vítězslav, Vincent Lecours, Kateřina Gdulová, Lukáš Gábor, Lucie Moudrá, Jan Kropáček, and Jan Wild. 2018. “On the Use of Global DEMs in Ecological Modelling and the Accuracy of New Bare-Earth DEMs.” Ecological Modelling 383:3–9. https://doi.org/10.1016/j.ecolmodel.2018.05.006.
- Nardi, F., A. Annis, G. Di Baldassarre, E. R. Vivoni, and S. Grimaldi. 2019. “GFPLAIN250m, a Global High-Resolution Dataset of Earth's Floodplains.” Scientific Data 6 (1): 180309. https://doi.org/10.1038/sdata.2018.309.
- Neal, Jeffrey C., Paul D. Bates, Timothy J. Fewtrell, Neil M. Hunter, Matthew D. Wilson, and Matthew S. Horritt. 2009. “Distributed Whole City Water Level Measurements From the Carlisle 2005 Urban Flood Event and Comparison with Hydraulic Model Simulations.” Journal of Hydrology 368 (1–4): 42–55. https://doi.org/10.1016/j.jhydrol.2009.01.026.
- Neal, Jeffrey, and Laurence Hawker. 2023. “FABDEM V1-2.” January. https://doi.org/10.5523/bris.s5hqmjcdj8yo2ibzi9b4ew3sn.
- Nguyen, Ngoc Son, Dong Eon Kim, Yilin Jia, Srivatsan V. Raghavan, and Shie Yui Liong. 2022. “Application of Multi-Channel Convolutional Neural Network to Improve DEM Data in Urban Cities.” Technologies 10 (3): 61. https://doi.org/10.3390/technologies10030061.
- Nuth, C., and A. Kääb. 2011. “Co-Registration and Bias Corrections of Satellite Elevation Data Sets for Quantifying Glacier Thickness Change.” The Cryosphere 5 (1): 271–290. https://doi.org/10.5194/tc-5-271-2011.
- Pavlis, Nikolaos K., Simon A. Holmes, Steve C. Kenyon, and John K. Factor. 2012. “The Development and Evaluation of the Earth Gravitational Model 2008 (EGM2008).” Journal of Geophysical Research: Solid Earth 117 (B4https://doi.org/10.1029/2011JB008916.
- Pekel, Jean-François, Andrew Cottam, Noel Gorelick, and Alan S. Belward. 2016. “High-Resolution Mapping of Global Surface Water and Its Long-Term Changes.” Nature 540 (7633): 418–422. https://doi.org/10.1038/nature20584.
- Purinton, Benjamin, and Bodo Bookhagen. 2021. “Beyond Vertical Point Accuracy: Assessing Inter-Pixel Consistency in 30 m Global DEMs for the Arid Central Andes.” Frontiers in Earth Science 9:901. https://doi.org/10.3389/feart.2021.758606.
- Rentschler, Jun, Melda Salhab, and Bramka Arga Jafino. 2022. “Flood Exposure and Poverty in 188 Countries.” Nature Communications 13 (1): 3527. https://doi.org/10.1038/s41467-022-30727-4.
- Rizzoli, Paola, Michele Martone, Carolina Gonzalez, Christopher Wecklich, Daniela Borla Tridon, Benjamin Bräutigam, Markus Bachmann. 2017. “Generation and Performance Assessment of the Global TanDEM-X Digital Elevation Model.” ISPRS Journal of Photogrammetry and Remote Sensing132:119–139. https://doi.org/10.1016/j.isprsjprs.2017.08.008.
- Rodríguez, Ernesto, Charles S. Morris, and J. Eric Belz. 2006. “A Global Assessment of the SRTM Performance.” Photogrammetric Engineering & Remote Sensing 72 (3): 249–260. https://doi.org/10.14358/PERS.72.3.249.
- Sampson, Christopher C., Andrew M. Smith, Paul D. Bates, Jeffrey C. Neal, Lorenzo Alfieri, and Jim E. Freer. 2015. “A High-Resolution Global Flood Hazard Model.” Water Resources Research 51 (9): 7358–7381. https://doi.org/10.1002/2015WR016954.
- Sampson, Christopher C., Andrew M. Smith, Paul D. Bates, Jeffrey C. Neal, and Mark A. Trigg. 2016. “Perspectives on Open Access High Resolution Digital Elevation Models to Produce Global Flood Hazard Layers.” Frontiers in Earth Science 3:85. https://doi.org/10.3389/feart.2015.00085.
- Sanders, Brett F.. 2007. “Evaluation of On-Line DEMs for Flood Inundation Modeling.” Advances in Water Resources 30 (8): 1831–1843. https://doi.org/10.1016/j.advwatres.2007.02.005.
- Savage, James Thomas Steven, Paul Bates, Jim Freer, Jeffrey Neal, and Giuseppe Aronica. 2016. “When Does Spatial Resolution Become Spurious in Probabilistic Flood Inundation Predictions?.” Hydrological Processes 30 (13): 2014–2032. https://doi.org/10.1002/hyp.10749.
- Schiavina, Marcello, Michele Melchiorri, and Martino Pesaresi. 2022. “GHS-SMOD R2022A -- GHS Settlement Layers, Application of the Degree of Urbanisation Methodology (Stage I) to GHS-POP R2022A and GHS-BUILT-S R2022A, Multitemporal (1975–2030).” June. https://doi.org/10.2905/4606D58A-DC08-463C-86A9-D49EF461C47F.
- Schlund, Michael, Daniel Baron, Paul Magdon, and Stefan Erasmi. 2019. “Canopy Penetration Depth Estimation with TanDEM-X and Its Compensation in Temperate Forests.” ISPRS Journal of Photogrammetry and Remote Sensing 147:232–241. https://doi.org/10.1016/j.isprsjprs.2018.11.021.
- Schumann, Guy J.-P.. 2014. “Fight Floods on a Global Scale.” Nature 507 (7491): 169–169. https://doi.org/10.1038/507169e.
- Shortridge, Ashton, and Joseph Messina. 2011. “Spatial Structure and Landscape Associations of SRTM Error.” Remote Sensing of Environment 115 (6): 1576–1587. https://doi.org/10.1016/j.rse.2011.02.017.
- Simpson, Alanna, Simone Balog, Delwyn Moller, Benjamin Strauss, and Keiko Saito. 2015. “An Urgent Case for Higher Resolution Digital Elevation Models in the World's Poorest and Most Vulnerable Countries.” Frontiers in Earth Science 3:50. https://doi.org/10.3389/feart.2015.00050.
- Szabó, Gergely, Sudhir Kumar Singh, and Szilárd Szabó. 2015. “Slope Angle and Aspect As Influencing Factors on the Accuracy of the SRTM and the ASTER GDEM Databases.” Physics and Chemistry of the Earth, Parts A/B/C 83–84:137–145. https://doi.org/10.1016/j.pce.2015.06.003.
- Tadono, T., H. Nagai, H. Ishida, F. Oda, S. Naito, K. Minakawa, and H. Iwamoto. 2016. “Generation of the 30 M-Mesh Global Digital Surface Model by ALOS PRISM.” In The International Archives of the Photogrammetry, Remote Sensing and Spatial Information Sciences, Vol. XLI-B4, June, 157–162. Copernicus GmbH.
- Takaku, Junichi, Takeo Tadono, Ken Tsutsui, and Mayumi Ichikawa. 2015. “Quality Status of High Resolution Global DSM Generated from ALOS PRISM.” In 2015 IEEE International Geoscience and Remote Sensing Symposium (IGARSS), July, 3854–3857.
- Takaku, Junichi, Takeo Tadono, Ken Tsutsui, and Mayumi Ichikawa. 2016. “Validation of AW3D Global DSM Generated From ALOS PRISM.” ISPRS Annals of the Photogrammetry, Remote Sensing and Spatial Information Sciences III-4:25–31. https://doi.org/10.5194/isprs-annals-III-4-25-2016.
- Tellman, B., J. A. Sullivan, C. Kuhn, A. J. Kettner, C. S. Doyle, G. R. Brakenridge, T. A. Erickson, and D. A. Slayback. 2021. “Satellite Imaging Reveals Increased Proportion of Population Exposed to Floods.” Nature 596 (7870): 80–86. https://doi.org/10.1038/s41586-021-03695-w.
- Toutin, Thierry. 2002. “Impact of Terrain Slope and Aspect on Radargrammetric DEM Accuracy.” ISPRS Journal of Photogrammetry and Remote Sensing 57 (3): 228–240. https://doi.org/10.1016/S0924-2716(02)00123-5.
- UNDRR. 2022. Global Assessment Report on Disaster Risk Reduction 2022: Our World at Risk: Transforming Governance for a Resilient Future. Technical Report. Geneva: United Nations Office for Disaster Risk Reduction (UNDRR).
- Uuemaa, Evelyn, Sander Ahi, Bruno Montibeller, Merle Muru, and Alexander Kmoch. 2020. “Vertical Accuracy of Freely Available Global Digital Elevation Models (ASTER, AW3D30, MERIT, TanDEM-X, SRTM, and NASADEM).” Remote Sensing 12 (21): 3482. https://doi.org/10.3390/rs12213482.
- Vaze, Jai, Jin Teng, and Georgina Spencer. 2010. “Impact of DEM Accuracy and Resolution on Topographic Indices.” Environmental Modelling & Software 25 (10): 1086–1098. https://doi.org/10.1016/j.envsoft.2010.03.014.
- Venter, Zander S., David N. Barton, Tirthankar Chakraborty, Trond Simensen, and Geethen Singh. 2022. “Global 10 m Land Use Land Cover Datasets: A Comparison of Dynamic World, World Cover and Esri Land Cover.” Remote Sensing 14 (16): 4101. https://doi.org/10.3390/rs14164101.
- Vernimmen, Ronald, Aljosja Hooijer, and Maarten Pronk. 2020. “New ICESat-2 Satellite LiDAR Data Allow First Global Lowland DTM Suitable for Accurate Coastal Flood Risk Assessment.” Remote Sensing 12 (17): 2827. https://doi.org/10.3390/rs12172827.
- Wendleder, Anna, Birgit Wessel, Achim Roth, Markus Breunig, Klaus Martin, and Susanne Wagenbrenner. 2013. “TanDEM-X Water Indication Mask: Generation and First Evaluation Results.” IEEE Journal of Selected Topics in Applied Earth Observations and Remote Sensing 6 (1): 171–179. https://doi.org/10.1109/JSTARS.2012.2210999.
- Wessel, Birgit, Martin Huber, Christian Wohlfart, Ursula Marschalk, Detlev Kosmann, and Achim Roth. 2018. “Accuracy Assessment of the Global TanDEM-X Digital Elevation Model with GPS Data.” ISPRS Journal of Photogrammetry and Remote Sensing 139:171–182. https://doi.org/10.1016/j.isprsjprs.2018.02.017.
- Winsemius, Hessel C., Philip J. Ward, Ivan Gayton, Marie-Claire ten Veldhuis, Didrik H. Meijer, and Mark Iliffe. 2019. “Commentary: The Need for a High-Accuracy, Open-Access Global DEM.” Frontiers in Earth Science 7. https://doi.org/10.3389/feart.2019.00033.
- Yamazaki, Dai, Daiki Ikeshima, Ryunosuke Tawatari, Tomohiro Yamaguchi, Fiachra O'Loughlin, Jeffery C. Neal, Christopher C. Sampson, Shinjiro Kanae, and Paul D. Bates. 2017. “A High-Accuracy Map of Global Terrain Elevations.” Geophysical Research Letters 44 (11): 5844–5853. https://doi.org/10.1002/2017GL072874.
- Zanaga, Daniele, Ruben Van De Kerchove, Wanda De Keersmaecker, Niels Souverijns, Carsten Brockmann, Ralf Quast, Jan Wevers, et al. 2021. “ESA WorldCover 10 m 2020 V100.” October. https://doi.org/10.5281/zenodo.5571936.