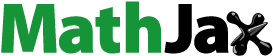
ABSTRACT
Target 14 of the Sustainable Development Goals (SDG 14) depicts a better future regarding the capacity of coastal environments to cope with several developmental challenges. However, gaps in the data and indicators restrict the effective implementation of this global plan. In this context, under the umbrella of the Digital Belt and Road (DBAR) Program, the DBAR_COAST working group (WG) aims to solve the problems of coastal sustainable development along the Maritime Silk Road (MSR). This paper demonstrates the recent efforts of the DBAR_COAST WG to develop indicators based on Earth observation (EO) data (i.e. the natural coastal protection index (NCPI), the local proportion of habitats protected index (LPHPI), and the coastal eco-erosion index (CEEI)), which complement the existing indicators related to SDGs 14.2, 14.5 and 14.7. These indicators all satisfy indicative, robust, scientifically sound and accessible data principles. Based on them, both the coastal protection (NCPI = 0.97) and protected area coverage (LPHPI = 0.38) in the MSR still had much room for improvement in 2020, and pressure from aquaculture expansion (CEEI = 0.11) showed significant regionally spatial heterogeneity. These indicators serve to provide a guideline for indicator-based assessment in monitoring the progress toward achieving SDG 14.
1. Introduction
The coastal zone is a unique and complex geographical unit in which marine and terrestrial ecosystems dynamically interact, and it provides unique ecological and socioeconomic functions (FAO Citation2020; Wang, Meng, and Long Citation2022). In recent years, coastal zones have faced severe threats from increasing populations, resource exploitation and climate change (Hossain, Gain, and Rogers Citation2020; Tenali and McManus Citation2022). In particular, habitat destruction has been the most severe, with the loss of one-third to one-half of the world's fragile coastal and marine habitats during the twenty-first century (Duarte et al. Citation2020). There is a clear tendency to use data to inform the decision-making for solving this global crisis (Gain, Ashik-Ur-Rahman, and Vafeidis Citation2019). Various global initiatives have made this call, among which the 2030 Agenda for Sustainable Development represents an unprecedented opportunity for achieving improvements in the coastal environment (UN Citation2015).
Sustainable Development Goal 14 (SDG 14), which is intended to ‘conserve and sustainably use the oceans, seas and marine resources for sustainable development,’ explicitly addresses coastal economic activities and ecosystems in its three main targets (14.2, 14.5, and 14.7). SDG 14.2 is aimed at sustainably managing and protecting marine and coastal ecosystems. SDG 14.5 encourages countries to take concrete actions to protect and manage their marine ecosystems by setting a target of effectively conserving 10% of marine and coastal areas through the establishment of protected areas or other conservation measures by 2020 (Adams and Judd Citation2016). SDG 14.7 specifically addresses the importance of sustainable fisheries and promotes the elimination of illegal, unreported, and unregulated (IUU) fishing practices (Liu Citation2022). These targets offer a guiding image, including a ‘healthy environment’ (preamble) and ‘healthy and productive oceans’ (target 14.2), but lack operational utility (Von Schuckmann et al. Citation2020). In addition, when reviewing the available databases, particularly their statistics, the evaluation indicators acquired were considered either excessively broad, vague or entirely unrelated to the targets (Warchold et al. Citation2022). Specifically, SDG 14.2.1 faces limitations in scale and interpretation, as it fails to reflect the conservation status of locations and certain coastal habitats (Diz, Morgera, and Wilson Citation2019). SDG 14.5.1 requires an understanding of what habitats and/or species require attention, which is closely linked to the determination of where (within national marine jurisdiction) to allocate 10% of conservation areas (Neumann, Ott, and Kenchington Citation2017). SDG 14.7.1 assesses the sustainable development of aquaculture from an economic perspective while ignoring the possible ecological impacts of aquaculture.
Earth Observation (EO) data offer increasing possibilities for incorporating environmental indicators at various global scales (Dizdaroglu Citation2017; Ruan et al. Citation2022; Wang et al. Citation2023; Zuo et al. Citation2023). A growing number of studies have focused on identifying EO-based indicators for SDG 14. Brander et al. (Citation2015) improved an SDG 14.5 indicator aimed at identifying spatial changes in biodiversity significance (benefits), which can help to inform the choice of protected areas to ensure that adequate attention is given to those areas with the highest biodiversity benefits and those facing the most severe threats. Sarelli et al. (Citation2018) presented a novel automatic methodology to calculate SDG 14.1.1 based on Copernicus Marine Environment Monitoring Service models of phosphate-nitrates-silica-chlorophyll and water transparency. Reimer et al. (Citation2023) presented a marine spatial planning index that can be used to analyze the spatial and temporal distributions of ocean uses to achieve SDG 14.2 and SDG 14.7. Studies have mostly focused on marine indicators, but lacked studies related to explanations of coastal habitat statuses, functions and related human activities.
The Digital Belt and Road Program (DBAR) was initiated at 2016, which uses Big Earth Data as its core, to promote the application of digital technologies, in the process of achieving the SDGs of the Belt and Road Initiative through the sharing of data, technology and knowledge, thus providing scientific and decision-making support and serving and promoting the implementation of the UN 2030 Agenda (Guo and Xiao Citation2016; Guo Citation2018; Guo et al. Citation2021). The program established 9 international scientific working groups (WG), which have carried out a great deal of work on the sustainable development challenges in their respective fields. Among them, the DBAR-COAST WG focuses on improving the ability of coastal and marine environmental monitoring, protection and management, and developing new scientific data, technologies and knowledge to support applications for sustainable human and economic development in coastal regions, thus supporting and promoting the assessment and monitoring of SDGs 6, 11 14 and 15 (Zhang et al. Citation2017).
Here, three indicators were developed to complement existing tools for measuring the progress toward SDG 14 on coastal habitat statuses and human activities by DBAR_COAST WG: the natural coastal protection index (NCPI) is used to measure the amount of nature-based protection provided by marine and coastal habitats; the local proportion of habitats protected index (LPHPI) is used to measure the extent of marine and coastal habitats within protected areas; and the coastal eco-erosion index (CEEI) is used to measure the degree of stress caused by human activities on regional ecosystems. Together these indicators keep countries informed regarding habitat protection and aquaculture pressure. These indicators exhibit substantial improvements over the other indicators in three key aspects: 1) These indicators are spatially explicit and applicable at multiple (national and local) scales; 2) These indicators are flexible and can be easily updated when new habitat data with greater resolution become available; and 3) These indicators all reflect the needs outlined in SDG 14 based on measurable quantities following the findable, accessible, interoperable and reusable (FAIR) principles. These indicators and their construction process reveal the efforts made by countries along the MSR in terms of coastal habitat conservation as well as in the management of coastal human activities. These findings could lead to guidelines for indicator-based assessments of progress made toward achieving SDG 14.
2. Materials and methods
2.1. Data collection and preprocessing
The data in this study included 10 coastal variables as follows: (1) the geospatial data produced by the DBAR_COAST WG (coastline, mangrove and aquaculture pond); (2) the geospatial data of the focal habitats (cold coral, coral reef, seagrass, salt marsh and kelp) as derived from the Ocean Data Viewer (https://data.unep-wcmc.org/); and (3) the data of global protected areas and other effective area-based conservation measures from the UNEP-WCMC and IUCN and exclusive economic zone (EEZ) data from the Flanders Marine Institute. The details for these data are listed in .
Table 1. Details and sources of data in this study.
The datasets produced by the DBAR_COAST WG accurately capture the spatial patterns of multiple aspects of coastal zones along the MSR () and play an essential role in coastal conservation and management, as described in Text S1 (Li et al. Citation2023; Guo, Liao, and Shen Citation2021; Luo et al. Citation2022). A series of preprocessing steps were conducted to address the differences in format of the data. The data from the most recent year were chosen as a proxy for temporally missing data. To balance data accuracy and computational efficiency, the polygon-type and line-type data were projected and converted into raster data with a 30-meter spatial resolution.
2.2. Study area
The study area covers the union of nations and their respective EEZs along the twenty-first Century Maritime Silk Road (MSR), which can make sure that coastlines do not clip the extent of coastal habitats such as mangroves and salt marshes. The MSR is a sea passage for international trade and cultural exchanges (Blanchard and Flint Citation2017), which passes through the coastal zones of many countries, and these countries account for approximately 60% of the world’s population and approximately 30% of the world’s GDP (CITI Citation2015). The coastline length along the MSR is approximately 3.48 × 105 km in length and contains a variety of coastal and marine habitats covering a range from natural to highly altered environments. Among them, the percentage of biotic coastlines, which provide nature-based protection from coastal hazards, such as storms and surges, coastal flooding, and erosion increased from 11.4% in 1990–14.5% in 2020 (Yang et al. Citation2023). The countries along the MSR and their coastline lengths are shown in Table S2.
Exclusive economic zones (EEZs) are ocean areas within 200 nautical miles of coastal area, which cover nearly all areas of the continental shelf and provide a vast majority of the food, natural resources, recreation, livelihoods and other benefits to humankind (Claudet, Loiseau, and Pebayle Citation2021). EEZs are widely used in marine and coastal assessments because they can capture most of the coastal issues of concern to the public, policy makers and resource managers (Halpern Citation2020; Kumagai et al. Citation2022).
2.3. Improved indicators based on earth observation (EO) data
In accordance with the guiding principles outlined in Clause 74 of the 2023 Agenda for Sustainable Development, EO-based indicators should be firmly grounded in robust evidence and derived from country-led assessments and data (UN Citation2015). They are designed to reflect the unique national circumstances, capacities, needs, and priorities of each country. Furthermore, they are crafted with a commitment to adaptability, given the dynamic nature of emerging issues and the continual development of new approaches in the field. On this basis, improved EO-based indicators are defined in this study as ‘a simple and understandable tool for describing, measuring and monitoring complex coastal phenomena.’
Effective coastal indicators can transition from being global to being local at different time scales, and they can be utilized to disseminate coastal knowledge and establish a sustainable coastal observation system for overall scientific assessment and management (Cochran et al. Citation2020). provides the proposed improved EO-based indicators as SDG 14 indicators and illustrates how each indicator relates to the current SDG 14 targets and indicators.
Figure 2. EO-based indicators and how they relate to SDG 14 targets and indicators. Symbols are courtesy of The Global Goals (https://www.globalgoals.org/resources/).

2.3.1. Natural coastal protection index (NCPI) supporting SDG 14.2
The NCPI is aimed at assessing the amount of nature-based protection provided by the marine and coastal habitats that serve as natural buffers against incoming waves. This indicator was modified from the coastal protection goal of the Ocean Health Index (OHI). The goal status score for coastal protection in the OHI is obtained as the geometric mean of the protection provided by mangroves, coral reefs, sea grasses, kelp, salt marshes and coastal sea ice (Halpern et al. Citation2012; Halpern Citation2020). It uses the total area and condition of key habitats within each EEZ for indicator construction without regard to their precise location relative to coastal areas. To improve this problem, the Euclidean distance is used to capture the spatial distribution of habitats relative to coastal zones in the NCPI.
The habitats identified as providing protection for coastal areas in this study include mangroves, warm- and cold-water coral reefs, seagrasses, kelp forests, sandy coastlines and salt marshes (Wang Citation2020). Moreover, human activities are considered to interfere with nature-based protection. First, the habitat layers were converted into a 1 km2 distance grid in EEZs via Euclidean distance. The grid values represent the distance from the habitat. Then, the ratio of the total number of habitat pixels in the grid from 1990 to 2020 was calculated as the health condition of the habitat using formula (2). Finally, the NCPI was calculated through formula (1) using weighted overlay. The weights are shown in .
(1)
(1)
(2)
(2) where
represents the status of coastal protection,
is the rank weight of the
habitat’s protective ability (),
is the number of habitats,
is the Euclidean distance from the kth habitat,
is the health condition of the kth habitat,
is the total number of kth habitat pixels in year t, and
is the total number of kth habitat pixels in the reference year. In this study, 1990 was set as the reference year.
Table 2. Natural Coastal protectiveness ranks . The scores (
) range from –4 to 4, with 4 being the most protective and −2 being the human activity impacts (Sharp et al. Citation2014).
The NCPI was calculated using six habitats and various coastline data () at 1 km grid scales. They were obtained as described in section 2.1. A value of lower than 1 indicates a reduction in protection compared to the reference year, a value of 1 indicates that there has been no change in protection, and a value of larger than 1 indicates an enhancement in protection.
2.3.2. Local proportion of habitats protected index (LPHPI) supporting SDG 14.5
Based on Kumagai et al. (Citation2022), the goal of the LPHPI is to monitor the extent of marine and coastal habitats in protected areas and in other effective area-based conservation measures. Previous efforts to measure the validity of protected areas, such as the protection equity metric (Chauvenet et al. Citation2017) and the mean protection gap metric (Jantke et al. Citation2019), are valuable tools and have led to progress in identifying indicators for total protected area. The LPHPI complements the types and locations of habitats, which are closely linked with determining where (within national marine jurisdiction) to allocate 10% of the conservation areas.
In this study, five marine and coastal habitats (mangroves, salt marshes, coral reefs, seagrasses and kelp) that are widely distributed in the MSR were considered in the context of the proposed 10% targets presented in SDG 14.5. Habitats were chosen based on the scientific community's assessment of the availability of high-quality spatially explicit global data. First, the protected or conserved areas (PCAs) were cleaned and filtered using the method proposed by the Protected Planet Initiative for protected and conserved areas. Then, the PCAs and habitat layers were projected and converted into 1 km2 grids. In addition, all the habitat layers intersected with the PCA layer, which produced the layers that inhabited PCAs. Finally, the LPHPI was calculated as formula (3) with all habitat layers and habitats within PCA layers through zonal statistics.
(3)
(3) where
is the local proportion of the habitat protection index,
is the total number of kth habitat pixels within the PCAs,
is the total number of kth habitat pixels within the jurisdiction, and n is the number of habitats. The index ranges from 0 (no coverage of PCAs) to 1 (100% coverage of PCAs).
2.3.3. Coastal eco-erosion index (CEEI) supporting SDGs 14.2 and 14.7
Based on landscape spatial adjacency theory (Lin et al. Citation2017), the CEEI is aimed at assessing the degree of stress caused by human activities on regional ecosystems through the use of land use data. It reflects the ecological process of horizontal transformations in the natural landscape into seminatural or semiartificial ecotones. Therefore, the CEEI represents the spatial occupation of the natural landscape by the artificial landscape and the landscape adjacency effect. The CEEI calculation accounts for the area of the artificial landscape, the length of the common edge, and the area and number of adjacent patches between the artificial landscape and the natural landscape.
First, land use layers were intersected with the 1 km2 grid through spatial join. The area of artificial landscape within each grid was counted. Then, all the natural landscape layers were intersected with the artificial landscape layers. The intersected layers were spatially counted to obtain the spatially adjacent length and proportion of the number of spatially adjacent patches between the natural landscape and artificial landscape. Finally, the length and proportion of the number of natural landscapes were spatially accounted for. The CEEI is calculated by a raster overlay based on formula (4) and the above parameters.
(4)
(4)
(5)
(5) where
is an indicator at the landscape level for the degree of eco-erosion of artificial landscape to natural landscape;
is the ratio of artificial landscape area to total landscape area;
is the number of natural landscape types;
is the spatial adjacent length between natural landscape and artificial landscape; and
is the spatial adjacent length between
natural landscape and artificial landscape in the total patch perimeter of
natural landscape.
is the proportion of the number of spatially adjacent patches between the
natural landscape and artificial landscape in the total number of patches of the
natural landscape;
is the length of the common edges of patch
;
is the perimeter of patch
;
is the total number of patches of the
natural landscape; and
is the number of spatially adjacent patches between the artificial landscape and the
natural landscape.
In this study, the CEEI value was divided into five grades (0–0.2 extremely low, 0.2–0.5 low, 0.5–0.8 medium, 0.8–1.0 high and >1.0 extremely high). The larger that the CEEI value is, the less space is left for the erosion and expansion of artificial landscapes, and the greater the level of regional ecological stress caused by aquaculture expansion.
2.4. Pearson correlation coefficient
The Pearson correlation coefficient is used to measure the linear dependence between two random variables, which is, in turn, used to reflect the linear correlation between two normal continuous variables (Xu and Deng Citation2017). It was used to measure the contribution of different types of habitat to LPHPI in this study. It is calculated using formula (6), which follows:
(6)
(6) where
is the mean of
,
is the mean of
, and
is the Pearson correlation coefficient of
and
.
3. Results
3.1. Nature-based protection provided by coastal habitats along the MSR based on the NCPI
depicts the NCPI map within the EEZs along the MSR in 2020, as per the calculations derived from formula (1) through the use of a weighted overlay. This figure illustrates the natural coastal protection, as compared to the reference year of 1990 (NCPI = 1.0), that is provided by coastal and marine habitats, which ranges in the illustration from yellow (low value) to dark blue (high value). The mean NCPI score in 2020 of the coastal zones within the EEZ boundaries along the MSR was 0.97 (), indicating that the overall protection capacity provided by the natural coastal habitats has declined. This provides an important benchmark and indicates that there is substantial room for improvement in the level of coastal ecosystem protection along the MSR. Sixty percent of the coastal zones along the MSR exhibited an index score of lower than 1, which indicated that the protection provided by natural coastal ecosystems was decreasing. The 10% of the coastal zones had an index score of over 1.1, indicating an increase in the protection provided by coastal and marine ecosystems in these regions of at least 10%.
Figure 3. Map of Natural Coastal Protection Index (NCPI) in Exclusive Economic Zones (EEZs) along the MSR. The index value lower than 1 indicates the reduction of protection compared to the reference of 1990. The index value of 1 indicates the no change of the protection. The index value greater than 1 indicates the enhance of protection.

Table 3. Mean scores of Natural Coastal Protection Index (NCPI) in different geographic regions.
The NCPI scores varied greatly by region, ranging from 0.46–1.58, with many regions of Southeast Asia and South Africa scoring poorly and parts of Europe, West Asia and various tropical island and uninhabited regions scoring highly (). Among them, Pakistan had the lowest score (0.57), and Iraq had the highest score (1.50). The NCPI for most developed countries, such as France and Spain, were clustered around 1, while some developing countries exhibited higher NCPI values. This is because some developing countries have gone through period of destruction followed by a period of governance. Distance weighting using Euclidean distance clearly showed the level of protection provided by natural ecosystems, thus leading to the implementation of protective measures. Compared to the national scale of the OHI and SDG 14.2.1, the NCPI reveals spatial differences within each country or region’s coastal zones. A higher spatial resolution and better accuracy compensate for the loss of information and the inability to capture fine features in national-scale assessments.
3.2. Status of coastal habitats in the PCAs along the MSR based on the LPHPI
depicts the LPHPI map along the MSR in 2020, according to the calculations generated by formula (3). The figure illustrates the status of coastal habitats in the PCAs, and these illustrations range from yellow (low value) to dark blue (high value). The mean index score for the coastal zones within EEZ boundaries along the MSR was 0.38 (), indicating substantial room for improvement in the area of habitats covered by PCA. More than 32% of EEZs had an index value of less than 0.1, indicating that these habitats have less than 10% PCA coverage, which was insufficient for meeting the 10% protection target in SDG 14.5. Among them, 10 EEZs had a value of 0, and none of these habitats were covered by PCAs. In comparison, 4 EEZs had a value of 1, which indicated that all of these habitats are within PCAs.
Figure 5. The Local Proportion of Habitat Protected Index (LPHPI) of MSR. It illustrates the extent of marine and coastal habitats covered by PCAs in a EEZ compared to the habitat extent, ranging from yellow (low value) to dark blue (high value). The index ranges from 0 (no coverage of PCAs) to 1 (100% coverage of PCAs).

Table 4. Mean scores of Local Proportion of Habitat Protected Index (LPHPI) in different geographic regions.
The LPHPI varied greatly across locations. Many of the top LPHPI values were in Europe, various tropical island and uninhabited regions (such as Romania, Bulgaria and Glorioso Islands), while the low LPHPI values were located in South Asia (such as Bangladesh and India) (). Of all habitats, the highest level of PCA coverage was identified for salt marshes, with a mean score of 0.72, and the lowest level was for kelp, with a score of 0.23 (). The habitat that contributes most to the LPHPI was coral reefs, with a correlation coefficient of 0.85, which accounts for the variation in the spatial distribution of the LPHPI (). Compared to SDG 14.5.1 (coverage of protected areas in relation to marine areas), the LPHPI reveals the degree of protection for each certain habitat and clearly shows which habitats in each country require greater care.
Figure 6. Scores of Habitat Protected Index (LPHPI) within Exclusive Economic Zones (EEZs) along the MSR.

Figure 7. The Local Proportion of Habitat Protected Index (LPHPI) of (a) Seagrass (b) Coral Reef (c) Salt Marshes (d) Kelp and (e) Mangrove in MSR.

Table 5. The mean PCA coverage of each type of habitat and the pearson correlation coefficient (r) between habitats and LPHPI.
3.3. Ecological impact analysis of aquaculture expansion based on CEEI
Explaining the impact of the interactions between human activities and ecosystems is vital. The CEEI was used as the improved indicator for SDG 14.7 to reveal the ecological impacts of aquaculture expansion. Due to the limitation of the spatial extent of the aquaculture pond data, Vietnam was selected as the test area for CEEI in this study. Vietnam exhibits the largest area of coastal aquaculture ponds in Southeast Asia (9363 km2), with an expansion pattern of outlaying and edge expansion (Luo et al. Citation2022). In particular, since the enactment of the Shrimp Aquaculture Export Promotion Program and the Land and Fisheries Law in 1987, which encouraged the transformation of land use to that of aquaculture ponds, the expansion of small-scale and extended aquaculture ponds with a series of ecological and environmental problems has been greatly facilitated (Disperati and Virdis Citation2015).
The average CEEI of aquaculture expansion in Vietnam was 0.11, which was an extremely low grade that indicates that aquaculture expansion puts little pressure on the regional ecology throughout the entire coastal zone. As shown in , this expansion showed significant spatial heterogeneity, with high values mostly concentrated in the south and northeast where there were larger areas of aquaculture ponds, such as the province of Bac Lieu (1.02) and the towns of Vinh Chau (1.03), Tran De (1.04) and Gia Rai (1.16). In addition, to reveal the scale extensibility of the index and fully utilize the advantages of high spatial resolution, we applied the index at the provincial and municipal scales by using formula (4). The CEEI ranges were 0.01–1.02 and 0–1.16 at the provincial and municipal scales, respectively, which indicated that the larger scales have a smaller CEEI value. This is due to the relatively large proportion of aquaculture ponds at smaller scales. A great number of towns with high CEEI were found in the provinces with median and even those with low CEEI. Obviously, a finer-scale assessment provides more details regarding ecological stress for sustainable management. In particular, water was the landscape most affected by aquaculture pond expansion at both the provincial and municipal scales, while forest was the landscape least affected at the provincial scale, and shrubland was the landscape least affected at the municipal scale ().
Figure 8. The spatial distribution of Coastal Eco-Erosion Index (CEEI) in Vietnam coastal zones with (a) provincial and (b) municipal scales.

Table 6. The spatial adjacent length between natural landscape and aquaculture pond in Vietnam coast at the provincial and municipal scale.
4. Discussion
4.1. Testing the validity of the improved indicators
As mentioned by Falck and Spangenberg (Citation2014), the indicators should be indicative, reproducible, transparent, scientifically sound, relevant, robust, sensitive, independent, quantifiable and limited in number. However, it is difficult to simultaneously achieve all of these criteria at the maximum level. Indicative, robust, scientifically sound and accessible data principles were used to test the validity of the improvement indicators used for monitoring the progress made toward SDG 14 (Stokes and Seto Citation2019). Furthermore, the indicators in this study are candidates for SDG 14, as the final decision on which indicator to use in a given situation depends on the context and the users.
Among the indicators, the NCPI is a quantification index for monitoring the use of ‘ecosystem-based approaches’ for achieving SDG 14.2.1. The LPHPI is used to quantify the ‘coverage of protected areas’ for SDG 14.5.1 in terms of specific habitats. The CEEI quantifies ‘sustainable fishers’ in terms of their ecological impacts on aquaculture expansion. All of these indicators reflect the needs of SDG 14 based on measurable/observable quantities. In terms of data accessibility, the data used in this study all followed the FAIR guiding principles. All of the datasets included in the indicators are listed in , and all of the steps involved in indicator construction can be accessed on GitHub (https://github.com/zuo-maker/DBAR_COAST).
As these indicators are based on standard methodologies and previous research, their scientific validity is recognizable, and their repeatability is ensured within the discipline’s customary limits. Specifically, the NCPI represents the spatial upscaling of indicators related to the coastal protection of the OHI and accomplishes spatial expansion by modifying the area to a weighted distance. Developed in 2012 and updated annually, the coastal protection of OHI is highly recognized and robust. In addition, the clean water and biodiversity models of OHI are widely accepted for SDG14 assessments (Rickels et al. Citation2016; UN Citation2022). LPHPI was constructed on the basis provided by Kumagai et al. (Citation2022), which offered a clear picture of the PCA coverage of each habitat. It uses a standardized and reproducible workflow and updates annually in accordance with the FAIR data management principle. CEEI is constructed based on the theory of spatial adjacency and interaction (Hersperger Citation2006), which is widely used in urban expansion studies and can effectively reflect the extent to which different regions and natural landscape types are stressed by the expansion of artificial landscapes.
Figure 9. (a) Regression showing the NCPI and coastal protection of OHI. (b) Regression showing the local proportion of habitat protected index (LPHPI) and the habitat protection indexes of Kumagai et al. (Citation2022). The bars on either side represent the distribution of values for the relevant indicator.

For robustness and accuracy, the NCPI and LPHPI were tested through country-level statistics. The NCPI and LPHPI within EEZs for each country were averaged, and these scores were compared to the scores obtained from the coastal protection of OHI and habitat protection indexes of Kumagai et al. (Citation2022). As shows, both NCPI and LPHPI have a positive linear relationship with the test data. Pixel-level NCPI data explains 58% of the country-level coastal protection of OHI, and pixel-level LPHPI data explains 82% of the habitat protection indexes of Kumagai et al. (Citation2022). At the same time, the NCPI and LPHPI are flexible, and any coastal habitat, such as tidal mudflats (Wu et al. Citation2022; Chen et al. Citation2023), can be added given the availability of high-quality data.
Figure 10. In-situ pictures of the regional indicators validity test. (a) the Silvo fisheries in the Saying Demak district, Indonesia; (b) the seagrass sample area in the Banggai district, Central Sulawesi, Indonesia; (c) the protection belt was broken by the wave in Indonesia; (d) the coast of Sayung sub district in central JAVA, Indonesia. They are provided by Dewayany Sutrisno.

4.2. Validation of the improved indicators at regional scales
Due to the pixel characteristics of the indicators, they can be used for a wide range of scale variations. Therefore, it is applicable at the global and as well as regional levels. These indicators reflect detailed information with which policymakers, the scientific community and stakeholders can better understand the state of conservation of marine and coastal habitats at the local level (). In recent years, the coastal zones in Indonesia, such as Central Java, have been affected by the compounding effects of coastal subsidence and sea level rise, and there is a strong need for ecosystem-based natural protection (Nurhidayah et al. Citation2022). Thus, Indonesia was selected to validate the scalability and usefulness of the indicator at the regional scale in this study.
Indonesia contains 17,054 islands (12,000 inhabitants) and 108,000 kilometers of coastline (Komunikasi Citation2018). Its coastal zones cover a diverse range of habitats, frequently represent fragile ecosystems and serve as habitats for a variety of both marine biota and humans. However, approximately 65% or more of Indonesia's population lives adjacent to or within a coastal zone, thus complicating resource management (Statistik Citation2020). These zones are increasingly threatened by environmental degradation (resource exploitation/overexploitation) and are undergoing unacceptably rapid environmental changes as a result of population growth, urbanization, tourism, and other various and often competing resource use tendencies (Sutrisno et al. Citation2021). Therefore, the indicators used in this study enable the monitoring and assessment of coastal habitats. The results showed that the NCPI of Indonesia was 1.01, which indicated that the habitat conditions in Indonesia in 2020 have remained largely unchanged compared to those in 1990, indicating a relatively successful conservation effect. As and show, the southeastern region of Indonesia, which is known for its abundance of coral reefs and seagrasses, has higher NCPI values (Hernawan et al. Citation2021). The LPHPI is 0.32, which shows that although Indonesia has established a series of national parks, nature reserves and marine reserves (such as Komodo National Park, Sebuku-Sembakung Marine National Park and the Pulau Pahawang Marine Protected Area), covering important ecosystems in coastal areas, there is still room for improvement in the habitat coverage of PCAs. Therefore, Indonesia needs to continue to strengthen its laws, regulations and enforcement of coastal habitat protection, promote public awareness, and cooperate with the international community to jointly address global marine environmental challenges.
4.3. Limitations and prospects of EO-based indicators
While our results show how the selected indicators can potentially contribute to SDG 14 targets, they also highlight their limitations and prospects. To obtain indicator data, the coastal variables used are the result of data collection and collaboration across multiple entities to ensure the most current and widest coverage. However, efforts toward data collection continue to face long-term challenges, including limited geographic scope and low-frequency time lags (Ru et al. Citation2023). In data production, due to the lack of sufficient preexisting global products, samples are still obtained by visual interpretation to ensure reliability, which makes establishing automated processes for data production difficult. In addition, a large amount of habitat data, which are relatively important for the MSR, were used in our indicator construction. There is a lack of corresponding long time-series high-resolution datasets for these habitats. Some habitat data are captured in the form of point vectors, which are excluded from the indicator calculation due to the lack of available polygon vectors. Moreover, there are still many habitats, such as seagrass beds and kelp, that cannot be captured because remote sensing observations generally perform poorly in penetrating water bodies and capturing underwater characteristics.
Regarding the construction method of indicators, although efforts were made to ensure that all of the indicators captured all of the relevant information, they cannot be truly comprehensive, as a full systematic review collects both unavailable and inaccurate data (Newell et al. Citation2019). Most importantly, the selection of coastal and marine habitats included in the NCPI and LPHPI is sensitive. Although the habitat data used in this study were peer reviewed, there is still an inherent knowledge gap between the actual context and the available data. Aside from the mangrove data, the other habitat data possess low temporal resolution and lack a consistent indexed time series. For the LPHPI, an important factor remaining to be considered is that spatially consistent habitat ranges within PCAs do not necessarily mean that specific habitats are protected.
In the future, the development of a dynamic and objective macro-monitoring system for sustainable coastal development can be used to support the implementation of the SDGs (). In terms of data, the spatial and temporal resolution, extraction accuracy, and automation of the existing data should all be improved, and other coastal variables should be expanded to enrich the variety and quantity of coastal data. Furthermore, on the basis of open access data, a measurement standard is needed for regulating the development of Earth Observation Data for sustainable development assessments. In addition, the three (economic, social and environmental) dimensions of the SDGs are generally quantified by techniques and methods that are independently developed and largely lacking in natural integration. Therefore, combining multidisciplinary cross-cutting knowledge to measure the trade-offs and synergies between different objectives in coastal zones and constructing and applying more refined indicators and assessment tools are necessary for better monitoring of the sustainable development process.
Figure 11. Dynamic and objective macro-monitoring system for the coastal sustainable development. Symbols are courtesy of The Global Goals (https://www.globalgoals.org/resources/).

5. Conclusion
In this study, three indicators were developed to complement the existing tools used to measure the progress achieved toward SDG 14: the NCPI captures the amount of nature-based protection provided by marine and coastal habitats; the LPHPI measures the extent of marine and coastal habitats within protected areas; and the CEEI reflects the degree of stress caused by human activities on regional ecosystems. These indicators exhibit substantial improvement over other indicators in three key aspects: (1) They are spatially explicit and applicable at multiple (national and local) scales. (2) They are flexible and can be easily updated when new habitat data with greater resolution become available. (3) They all reflect the needs of SDG 14 based on measurable quantities following the findable, accessible, interoperable and reusable (FAIR) principles. Based on the selected indicators, coastal protection (NCPI = 0.97) and PCA coverage (LPHPI = 0.38) in the MSR still had much room for improvement in 2020, and the pressure exerted by aquaculture expansion (CEEI = 0.11) showed significant regional spatial heterogeneity. The multiscale case of the MSR used in this study fully supports the usability and scientific validity of these indicators and provides guidance on indicator-based assessment for monitoring the progress made toward achieving SDG 14.
Supplemental Material
Download MS Word (22.5 KB)Acknowledgments
The authors were grateful to the data support from Big Data and Cloud Service Platform (CASEarth).
Disclosure statement
No potential conflict of interest was reported by the author(s).
Data availability statement
The data that support the findings are openly available in Gitub at https://github.com/zuo-maker/DBAR_COAST.
Additional information
Funding
References
- Adams, B., and K. Judd. 2016. “2030 Agenda and the SDGs: Indicator Framework, Monitoring and Reporting.” Agenda (durban, South Africa) 10 (18).
- Blanchard, J. M. F., and C. Flint. 2017. “The Geopolitics of China’s Maritime Silk Road Initiative.” Geopolitics 22 (2): 223–245.
- Brander, L., C. Baulcomb, J. A. C. van der Lelij, F. Eppink, A. McVittie, L. Nijsten, and P. van Beukering. 2015. “The Benefits to People of Expanding Marine Protected Areas.” Institute for Environmental Studies, VU University Amsterdam, Report, 15-05.
- Chauvenet, A. L., C. D. Kuempel, J. McGowan, M. Beger, and H. P. Possingham. 2017. “Methods for Calculating Protection Equality for Conservation Planning.” PLoS one 12 (2): e0171591.
- Chen, C., B. Tian, W. Wu, Y. Duan, Y. Zhou, and C. Zhang. 2023. “UAV Photogrammetry in Intertidal Mudflats: Accuracy, Efficiency, and Potential for Integration with Satellite Imagery.” Remote Sensing 15 (7): 1814.
- CITI. 2015. Industrial Cooperation Between Countries Along the Belt and Road. Beijing, China: China International Trade Institute.
- Claudet, J., C. Loiseau, and A. Pebayle. 2021. “Critical Gaps in the Protection of the Second Largest Exclusive Economic Zone in the World.” Marine Policy 124: 104379.
- Cochran, F., J. Daniel, L. Jackson, and A. Neale. 2020. “Earth Observation-Based Ecosystem Services Indicators for National and Subnational Reporting of the Sustainable Development Goals.” Remote Sensing of Environment 244: 111796.
- Disperati, L., and S. G. P. Virdis. 2015. “Assessment of Land-use and Land-Cover Changes from 1965 to 2014 in Tam Giang-Cau Hai Lagoon, Central Vietnam.” Applied Geography 58: 48–64.
- Diz, D., E. Morgera, and M. Wilson. 2019. “Marine Policy Special Issue: Sdg Synergies for Sustainable Fisheries and Poverty Alleviation.” Marine Policy 110: 102860.
- Dizdaroglu, D. 2017. “The Role of Indicator-Based Sustainability Assessment in Policy and the Decision-Making Process: A Review and Outlook.” Sustainability 9 (6): 1018.
- Duarte, C. M., S. Agusti, E. Barbier, G. L. Britten, J. C. Castilla, J. P. Gattuso, R. W. Fulweiler, et al. 2020. “Rebuilding Marine Life.” Nature 580 (7801): 39–51.
- Falck, W. E., and J. H. Spangenberg. 2014. “Selection of Social Demand-Based Indicators: Eo-Based Indicators for Mining.” Journal of Cleaner Production 84: 193–203.
- FAO. 2020. The State of World Fisheries and Aquaculture 2020. Sustainability in action. Rome. https://doi.org/10.4060/ca9229en.
- Freiwald, A., A. Rogers, J. Hall-Spencer, J. M. Guinotte, A. J. Davies, C. Yesson, C. S. Martin, and L. V. Weatherdon. 2018. “Global Distribution of Cold-Water Corals (version 5.0).” Fifth Update to the Dataset in Freiwald et al. (2004) by UNEP-WCMC, in Collaboration with Andre Freiwald and John Guinotte. Cambridge (UK): UNEP-WCMC.
- Gain, A. K., M. Ashik-Ur-Rahman, and A. Vafeidis. 2019. “Exploring Human-Nature Interaction on the Coastal Floodplain in the Ganges-Brahmaputra Delta Through the Lens of Ostrom’s Social-Ecological Systems Framework.” Environmental Research Communications 1(5): 051003.
- Guo, H. 2018. “Steps to the Digital Silk Road.” Nature 554 (7690): 25–27.
- Guo, H., F. Chen, Z. Sun, J. Liu, and D. Liang. 2021a. “Big Earth Data: A Practice of Sustainability Science to Achieve the Sustainable Development Goals.” Sci. Bull 66 (11): 1.
- Guo, Y., J. Liao, and G. Shen. 2021b. “Mapping Large-Scale Mangroves Along the Maritime Silk Road from 1990 to 2015 Using a Novel Deep Learning Model and Landsat Data.” Remote Sensing 13 (2): 245.
- Guo, H., and H. Xiao. 2016. “Earth Observation for the Belt-Road and Digital Belt-Road Initiative.” Bulletin of Chinese Academy of Sciences 31 (5): 535–541.
- Halpern, B. S. 2020. “Building on a Decade of the Ocean Health Index.” One Earth 2 (1): 30–33.
- Halpern, B. S., C. Longo, D. Hardy, K. L. McLeod, J. F. Samhouri, S. K. Katona, K. Kleisner, et al. 2012. “An Index to Assess the Health and Benefits of the Global Ocean.” Nature 488 (7413): 615–620.
- Hernawan, U. E., S. Rahmawati, R. Ambo-Rappe, N. D. Sjafrie, H. Hadiyanto, D. S. Yusup, A. H. Nugraha, et al. 2021. “The First Nation-Wide Assessment Identifies Valuable Blue–Carbon Seagrass Habitat in Indonesia is in Moderate Condition.” Science of The Total Environment 782: 146818.
- Hersperger, A. M. 2006. “Spatial Adjacencies and Interactions: Neighborhood Mosaics for Landscape Ecological Planning.” Landscape and Urban Planning 77 (3): 227–239.
- Hossain, M. S., A. K. Gain, and K. G. Rogers. 2020. “Sustainable Coastal Social-Ecological Systems: How do we Define “Coastal”?” International Journal of Sustainable Development & World Ecology 27 (7): 577–582.
- Jantke, K., C. D. Kuempel, J. McGowan, A. L. Chauvenet, and H. P. Possingham. 2019. “Metrics for Evaluating Representation Target Achievement in Protected Area Networks.” Diversity and Distributions 25 (2): 170–175.
- Jayathilake, D. R. M., and M. J. Costello. 2020. “A Modelled Global Distribution of the Kelp Biome.” Biological Conservation 252: 108815.
- Komunikasi, B. 2018. “Menko Maritim Luncurkan Data Rujukan Wilayah Kelautan Indonesia.” Kementrian Koordinator Bidang Kemaritiman dan Investasi 10.
- Kumagai, J. A., F. Favoretto, S. Pruckner, A. D. Rogers, L. V. Weatherdon, O. Aburto-Oropeza, and A. Niamir. 2022. “Habitat Protection Indexes - new Monitoring Measures for the Conservation of Coastal and Marine Habitats.” Scientific Data 9 (1): 203.
- Li, K., L. Zhang, B. Chen, J. Zuo, F. Yang, and L. Li. 2023. “Analysis of China’s Coastline Changes During 1990–2020.” Remote Sensing 15 (4): 981.
- Liao, J. 2022. Global Spatial Distribution of 30m Mangroves 2000-2020 (GMF30_2000-2020). Beijing: International Research Center of Big Data for Sustainable Development Goals. doi:10.12237/casearth.62ff4caa819aec75a535cbe8.
- Lin, M. X., T. Lin, Q. Y. Qiu, C. G. Sun, F. L. Deng, and G. Q. Zhang. 2017. “Comparition of Ecological Security Stress Effects of Artificial Landscapes on Natural Landscapes in Different Rapid Urban Sprawl Areas.” Ying Yong Sheng tai xue bao = The Journal of Applied Ecology 28 (4): 1326–1336.
- Liu, S. 2022. “Sustainable Fishery and Renewable Energy in Perspective of Sustainable Development Goals (SDGs): Re-Visiting SDG Indicators 7.2.1 and 14.7.1. 1 and 14.7. 1.” European Journal of Sustainable Development 11 (1): 101–101.
- Liu, L., and X. Zhang. 2021. Global 30m Land Cover Classification Product V1.0 in 2020. Beijing.: International Research Center of Big Data for Sustainable Development Goals.
- Luo, J. 2023. A Dataset on the Distribution Range of 30mLandsat TM/OLI Nearshore Aquaculture Areas Along the Maritime Silk Road and its Changes from 1990-2015. Beijing.: International Research Center of Big Data for Sustainable Development Goals.
- Luo, J., Z. Sun, L. Lu, Z. Xiong, L. Cui, and Z. Mao. 2022. “Rapid Expansion of Coastal Aquaculture Ponds in Southeast Asia: Patterns, Drivers and Impacts.” Journal of Environmental Management 315: 115100.
- Neumann, B., K. Ott, and R. Kenchington. 2017. “Strong Sustainability in Coastal Areas: A Conceptual Interpretation of SDG 14.” Sustainability Science 12: 1019–1035.
- Newell, S. L., N. Nagabhatla, N. C. Doubleday, and A. Bloecker. 2019. “The Potential for Locally Managed Marine Area (LMMAs) as a Participatory Strategy for Coastal and Marine Ecosystems-the Global Commons.” OIDA International Journal of Sustainable Development 12 (04): 47–62.
- Nurhidayah, L., P. Davies, S. Alam, N. Saintilan, and A. Triyanti. 2022. “Responding to sea Level Rise: Challenges and Opportunities to Govern Coastal Adaptation Strategies in Indonesia.” Maritime Studies 21 (3): 339–352.
- Reimer, J. M., R. Devillers, R. Zuercher, P. Groulx, N. C. Ban, and J. Claudet. 2023. “The Marine Spatial Planning Index: A Tool to Guide and Assess Marine Spatial Planning.” npj Ocean Sustainability 2 (1): 15.
- Rickels, W., J. Dovern, J. Hoffmann, M. F. Quaas, J. O. Schmidt, and M. Visbeck. 2016. “Indicators for Monitoring Sustainable Development Goals: An Application to Oceanic Development in the European Union.” Earth's Future 4 (5): 252–267.
- Ru, Y., B. Blankespoor, U. Wood-Sichra, T. S. Thomas, L. You, and E. Kalvelagen. 2023. “Estimating Local Agricultural Gross Domestic Product (AgGDP) Across the World.” Earth System Science Data 15 (3): 1357–1387.
- Ruan, L., M. Yan, L. Zhang, X. Fan, and H. Yang. 2022. “Spatial-temporal NDVI Pattern of Global Mangroves: A Growing Trend During 2000–2018.” Science of The Total Environment 844: 157075.
- Sarelli, A., D. Sykas, M. Miltiadou, D. Bliziotis, Y. Spastra, and M. Ieronymaki. 2018. “A Novel Automated Methodology That Estimates the United Nations (UN) Sustainable Development Goal (SDG) 14.1. 1.: Index of Coastal Eutrophication Using the Copernicus Marine Environment Monitoring Service (CMEMS).” In Sixth International Conference on Remote Sensing and Geoinformation of the Environment (RSCy2018) (Vol. 10773, p. 1077302). SPIE.
- Sharp, R., H. T. Tallis, T. Ricketts, A. D. Guerry, S. A. Wood, R. Chaplin-Kramer, and A. L. Vogl. 2014. InVEST User’s Guide. The Natural Capital Project. Collaborative Publication by the Natural Capital Project, Stanford University, University of Minnesota, the Nature Conservancy, and World Wildlife Fund Stanford University: Stanford, CA, USA.
- Statistik, B. P. 2020. “Statistik Sumber Daya Laut Dan Pesisir Perubahan Iklim di Wilayah Pesisir.” BPS RI. Jakarta.
- Stokes, E. C., and K. C. Seto. 2019. “Characterizing Urban Infrastructural Transitions for the Sustainable Development Goals Using Multi-Temporal Land, Population, and Nighttime Light Data.” Remote Sensing of Environment 234: 111430.
- Sutrisno, D., M. Darmawan, A. Rahadiati, M. Helmi, A. Yusmur, M. Hashim, P. T. Y. Shih, R. Qin, and L. Zhang. 2021. “Spatial-planning-based Ecosystem Adaptation (SPBEA): A Concept and Modeling of Prone Shoreline Retreat Areas.” ISPRS International Journal of Geo-Information 10 (3): 176.
- Tenali, S., and P. McManus. 2022. “Climate Change Acknowledgment to Promote Sustainable Development: A Critical Discourse Analysis of Local Action Plans in Coastal Florida.” Sustainable Development 30 (5): 1072–1085.
- UNEP-WCMC and IUCN. 2021. “Protected Planet: The World Database on Other Effective Area-Based Conservation Measures (WDOECM] [On-line], May 2021,” Cambridge, UK: UNEP-WCMC and IUCN.
- UNEP-WCMC and IUCN. 2021. “Protected Planet: The World Database on Protected Areas (WDPA)/The Global Database on Protected Areas Management Effectiveness (GD-PAME)] [On-line], May 2021,” Cambridge, UK: UNEP-WCMC and IUCN.
- UNEP-WCMC, Short FT. 2020. Global Distribution of Seagrasses (Version 7.0). Seventh Update to the Data Layer Used in Green and Short (2003). Cambridge, UK: UNEP-WCMC.
- UNEP-WCMC, WorldFish Centre, WRI, TNC. 2018. “Global Distribution of Warm-Water Coral Reefs, Compiled from Multiple Sources Including the Millennium Coral Reef Mapping Project.” Version 4.0. Includes Contributions from IMaRS-USF and IRD (2005), IMaRS-USF (2005) and Spalding et al. (2001). Cambridge (UK): UNEP-WCMC.
- United Nations. 2015. Transforming our World: The 2030 Agenda for Sustainable Development. New York: United Nations, Department of Economic and Social Affairs.
- United Nations. Department of Economic and Social Affairs. 2022. The Sustainable Development Goals: Report 2022. New York: UN.
- Von Schuckmann, K., E. Holland, P. Haugan, and P. Thomson. 2020. “Ocean Science, Data, and Services for the UN 2030 Sustainable Development Goals.” Marine Policy 121: 104154.
- Wang, H. 2020. “Mapping 21st Century Maritime Silk Road and its Impact to Marine Ecosystems.” Master’ s thesis, Duke University.
- Wang, X., X. Meng, and Y. Long. 2022. “Projecting 1 km-Grid Population Distributions from 2020 to 2100 Globally Under Shared Socioeconomic Pathways.” Scientific Data 9 (1): 563.
- Wang, Z., S. Nie, X. Xi, C. Wang, J. Lao, and Z. Yang. 2023. “A Methodological Framework for Specular Return Removal from Photon-Counting LiDAR Data.” International Journal of Applied Earth Observation and Geoinformation 122: 103387.
- Warchold, A., P. Pradhan, P. Thapa, M. P. I. F. Putra, and J. P. Kropp. 2022. “Building a Unified Sustainable Development Goal Database: Why Does Sustainable Development Goal Data Selection Matter?” Sustainable Development 30 (5): 1278–1293.
- Wu, W., C. Zhi, Y. Gao, C. Chen, Z. Chen, H. Su, W. Lu, and B. Tian. 2022. “Increasing Fragmentation and Squeezing of Coastal Wetlands: Status, Drivers, and Sustainable Protection from the Perspective of Remote Sensing.” Science of the Total Environment 811: 152339.
- Xu, H., and Y. Deng. 2017. “Dependent Evidence Combination Based on Shearman Coefficient and Pearson Coefficient.” Ieee Access 6: 11634–11640.
- Yang, F., L. Zhang, B. Chen, K. Li, J. Liao, R. Mahmood, M. E. Hasan, M. A. Mamun, S. A. Raza, and D. Sutrisno. 2023. “Long-Term Change of Coastline Length Along Selected Coastal Countries of Eurasia and African Continents.” Remote Sensing 15 (9): 2344.
- Zhang, L. 2023. 30m Landsat TM/OLI Shoreline Variation Dataset Along the the Maritime Silk Road from 1990 to 2020. Beijing.: International Research Center of Big Data for Sustainable Development Goals.
- Zhang, L., H. Lin, S. Dewayany, M. K. Myint, S. H. Mohamad, M. L. Zin, H. Zhang, and L. Zhu. 2017. “Earth Observation of Resources and Environment in Coastal Zone Along the Maritime Silk Road.” Bulletin of Chinese Academy of Sciences 32 (Z1): 26–33.
- Zuo, J., L. Zhang, B. Chen, J. Liao, M. Hashim, D. Sutrisno, M. E. Hasan, R. Mahmood, and D. A. Sani. 2023. “Assessment of Coastal Sustainable Development Along the Maritime Silk Road Using an Integrated Natural-Economic-Social (NES) Ecosystem.” Heliyon 9 (6): e17440.