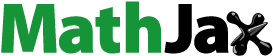
ABSTRACT
Increased tourism forms subdivide the tourism market. Therefore, distinguishing spatiotemporal behaviors of different types of tourists is of great significance for tourism marketing and planning. In this study, we constructed a Spatial–Temporal-Preference model based on spatial clustering, seasonal-trend decomposition and statistical analysis, using multi-source data. It provides a novel frame for comparing tourist behavioral characteristics from a new perspective. Meanwhile, providing a method to rapidly identify the Tourism Infrastructure Supply and Demand Pattern (TISDP) fusing nighttime light and social media data. Then the framework was applied to analyze the differences between sightseeing tourists (STs) and cycling tourists (CTs) in Yunnan Province, China. The results showed clear spatial differences in location popularity and flow data with STs favoring northwestern Yunnan, and CTs favoring eastern Yunnan. Tourist behavior varied also seasonally and tourists preferred visiting minority areas or areas with better infrastructure. The tourism industry in the study area developed rapidly after 2013, although still with an unbalanced infrastructure supply and demand. The CTs loss areas were greater in northeastern and northwestern Yunnan and south of Kunming. The proposed methods help to identify differences in tourism activities and has important implications to tourism infrastructure planning and sustainable development.
1. Introduction
Tourism is one of the most important components of the global economy (Inkson and Minnaert Citation2022). Development of the economy and tourism industry has led to the emergence of multiple methods of travel and different reasons for tourism, such as sports and leisure tourism (Bouchet and Sobry Citation2019), gourmet tourism (Ellis et al. Citation2018). Therefore, to ensure the sustainable development of tourism, it is important to understand the behavior patterns of different tourists. Following technological advances, researchers are employing new types of geographic data to analyze the spatiotemporal characteristics of tourist behavior including self-driving tourism (Li and Wang Citation2020), sightseeing tourism (He et al. Citation2018), and cycling tourism (Mou et al. Citation2022), highlighting the important role of geographic datasets in tourism research.
For example, online social sharing platforms widely used in tourism with users recording and sharing their trips, photos, and geographic coordinates. Thus, social media has become an emerging source of large amounts of geographic data characterized by high accuracy and a fast update speed, known as ‘digital footprints’ (Girardin et al. Citation2008). The spatiotemporal characteristics of tourists can then be effectively mined through the spatiotemporal information contained in digital footprints (Qin et al. Citation2022; Salas-Olmedo et al. Citation2018). Moreover, recently nighttime light (NTL) remote sensing data which are strongly correlated with the intensity of human activities (Wu et al. Citation2022; Zeng et al. Citation2021), the level of economic development (Gibson, Olivia, and Boe-Gibson Citation2020), and the degree of infrastructure development (Yu et al. Citation2018), are widely used as basic data to monitor urban development (Zhu et al. Citation2023), economic activity and human activity. The digital travel footprint is a concentrated expression of human travel behavior. The behavioral characteristics of tourists and travel experiences depend on tourism infrastructure (Mandić, Mrnjavac, and Kordić Citation2018), which predominantly includes transportation, accommodation, food and drink, shopping, entertainment, scenic infrastructure and other potential infrastructure. Potential infrastructure refers to various service locations that meet the potential needs of tourists, such as gas stations, rural toilets, etc. However, as tourism infrastructure is typically unevenly distributed, local governments are increasingly focusing on improving tourism infrastructure in an attempt to ensure national competitiveness and further develop the tourism industry (Petrova et al. Citation2018). The premise of improving infrastructure is to identify areas with insufficient infrastructure and prevent excess facility resources. This highlights the need to identify the tourism infrastructure supply–demand pattern (TISDP). These infrastructures are the major components in point of interest (POI) data. POI data can reflect the spatial differences in infrastructure development by describing the aggregation of data in a virtual geographic space. NTL data reflect the level of urban and rural development by describing the brightness of lights in different locations, thus a significant spatial correlation exists between POI density and NTL brightness (Ye et al. Citation2019). Many studies have demonstrated the correlation between NTL and road infrastructure, hotel facilities, tourism POI, etc. (Chang et al. Citation2022; Das et al. Citation2021). However, NTL can reflect the regional nature of infrastructure services better than POI (Zhang and Wei Citation2022). Specifically, the stable annual average NTL radiance, which can indicate infrastructure from street lights and exterior building lights, can indirectly reflect the level of infrastructure availability (Pandey, Brelsford, and Seto Citation2022). Thus, NTL remote sensing data can be combined with the characteristics of human tourism activities to analyze the TISDP and the development of tourism can be analyzed through changes in supply and demand. These data provide an effective data source for tourism activity mapping.
However, it is difficult to identify tourist behavior only by analyzing the characteristics of a single type of tourists. That is, the behaviors of different types of tourists can vary in the same area according to differences in tourism resources and scenic attraction locations. Nevertheless, few studies have compared the spatiotemporal behavior of different types of tourists. Sports and leisure tourism is currently one of the most popular forms of travel, with cycling tourism the most popular form of sports and leisure tourism globally (Han et al. Citation2020; Han, Meng, and Kim Citation2017). Sightseeing is the most common form of tourism in the world. Traditional sightseeing tourism and cycling tourism, which represent different travel modes and experiences, have both received extensive attention in tourism management.
Therefore, the main contributions and innovations of this paper are as follows: Unlike most previous research that primarily analyzed the behavior of a type of tourists, we propose a novel framework for the comparative analysis of tourist behavior starting from a new perspective. And identify the TISDP fusing social media digital footprints and NTL data. Using the framework, it is helpful to explore the differences in behavioral characteristics between the two types of tourists clearly and identify TISDP rapidly, including spatiotemporal behavior, preferences, and infrastructure needs. In this study, the framework was applied the to explore the differences in behavioral characteristics between sightseeing tourists (STs) and cycling tourists (CTs) in Yunnan Province, China. First, we explore spatiotemporal behavior differences between the two types of tourists using social media digital footprint data, with STs data derived from Flickr website and CTs data derived from 2bulu website. Then, NTL and ethnic minority distribution data for the study area are combined to analyze tourist preferences. Finally, profit and loss calculations are used to combine social media digital footprints and NTL to analyze infrastructure development in scenic spots and identify the TISDP. The method presented in this study provides a reference for comparing tourist behaviors in other regions and has substantial implications for tourism marketing, human behavior observation, and sustainable development.
2. Literature review
2.1. Research on differences in tourism behavior
Differences in tourism demand causes tourists to express different behavioral characteristics such as destination choice and spatiotemporal behavioral patterns (Edwards and Griffin Citation2013). Exploring the differences in tourism behavior can therefore provide a decision-making basis for sustainable tourism development. Previous research on differences in tourism behavior has generally focused on differences in regions, races, times, and cultures. For example, scholars studied the differences in tourism behaviors and attitudes of tourists from different cultural backgrounds. Differences in the interests, motivations, and behaviors of people from different cultural backgrounds were compared using questionnaires and online comments of tourists in scenic spots (Kang and Moscardo Citation2006; Kim and Lee Citation2000; Kirilenko, Stepchenkova, and Hernandez Citation2019). Choe, Kim, and Joun (Citation2019) studied the seasonal differences in tourism and their impact on stay duration, consumption, and tourists’ response to destination advertising. Moreover, Ibănescu, Stoleriu, and Gheorghiu (Citation2018) and Meng and Uysal (Citation2008) analyzed the differences in travel participation and travel expenditures between genders. These differences are embodied in the choice of destination preference, travel route, and time.
However, despite the continued development of tourism, few studies have discussed the differences in the behavioral patterns of different types of tourists, such as ordinary STs and CTs. Thus, an in-depth comparison of the behavioral characteristics of different tourists can help guide subdivided tourism planning and sustainable tourism development.
2.2. Mining the spatiotemporal behavior patterns of tourists
To distinguish differences in tourist behavior, we must first understand the spatiotemporal behavior patterns of tourists. The tourism experience involves three domains: time, space, and activity (Huang et al. Citation2020). Spatiotemporal behavioral patterns are the behavioral characteristics of tourists and are composed of time, space, and tourist activities during travel. Spatial tourism behavior reflects tourist activities in different locations, whereas temporal behavior reflects tourist activities at different times (Mou et al. Citation2022). The underlying reasons for each behavioral pattern can be used to inform tourism management.
In the field of spatiotemporal tourism pattern mining, digital footprint data derived from social media is often used in combination with other types of spatiotemporal pattern mining, because of their obvious advantages in quantity and quality (Korpilo, Virtanen, and Lehvävirta Citation2017). Tourism scholars have implemented the following practices based on the multi-source data: Ridwana and Himayah (Citation2020) distinguished tourism-related clusters from normal clusters by exploring different Twitter metadata along with OpenStreetMap and NTL data, then used a remote sensing solution to recommend the minimum level pf essential facilities at tourist sites. Xue and Zhang (Xue and Zhang Citation2020) studied the impact of distance on tourist behavior by combining Weibo digital footprints, user profiles and POI data sets, then analyzed the differences in spatial and consumption patterns among long-distance tourists, short-distance tourists and local tourists. However, studies that only analyze the spatial distribution of tourist behavior, lack analysis of time and scenery preferences. Conversely, Dong et al. (Citation2023) used a multi-source data footprint to classify five modes of ecotourism and identify the spatiotemporal behavior patterns and entertainment preferences of each mode through clustering and a classification tree. This research considered both time and space factors such as the tour time, route length, and scenic spot visits, but didn't consider the reasons for different characteristics and motivations of the five tourism modes.
The combination of digital footprint data and other research methods provides a methodology for tourism research that can be used for multiple purposes, such as spatial, hotspot, and preference analyses. Thus, the use of multi-source data allows for more comprehensive mining of spatiotemporal behavioral patterns.
However, different regions have different tourism patterns and scenic spot characteristics, leading to different travel preferences among tourists; therefore, it is important to also consider the characteristics of the study area and objectives of the study.
2.3. Application of NTL remote sensing data in tourism research
NTL remote sensing data can provide continuous and relatively accurate global nighttime brightness data, which can be used to identify the human economic activity, infrastructure levels, and their spatial characteristics (Zhang et al. Citation2015). Therefore, it is widely used for monitoring urbanization and human behavior, including urban built-up areas (Chang et al. Citation2022), urban sprawl (Kabanda Citation2022), disaster resilience (Liu et al. Citation2023; Liu et al. Citation2023), and human activity (Song et al. Citation2022).
Many scholars have combined NTL data with digital footprints to mine spatiotemporal information related to tourism. For example, the coupling analysis of NTL and digital footprints analyzes the spatial correlation (Wang et al. Citation2019) and seasonal variation of NTL images and their correlation with tourism activities (Krikigianni, Tsiakos, and Chalkias Citation2019).
Recently, NTL data have become a new data source for quantitative research on the night economy. Moreover, Zeng, Liu, and Du (Citation2022) studied the distribution of service facilities using NTL data, analyzed the relationship between activity hotspots and infrastructure supply and demand, and provided a new perspective for studying the relationship between human activities and infrastructure (Zeng, Liu, and Du Citation2022). However, the application of NTL data to tourism research is predominantly limited to correlations; thus, quantitative analyses are lacking.
Many researchers consider infrastructure factors when studying tourism activities (Liu, Huang, and Yang Citation2020; Ridwana and Himayah Citation2020). Future tourism development depends on the improvement and modernization of infrastructure (Jovanoviä and Ivana Citation2016) as a lack of tourist facilities can affect tourist satisfaction and travel choices (Kinash et al. Citation2019). However, research on tourism infrastructure typically focuses on single factors such as transportation, scenic spots, and hotels, or a combination of a few factors, with little research covering all aspects of tourism and public service facilities (Liu, Huang, and Yang Citation2020).
In summary, there is a lack of research on differences in tourist behavior in different forms of tourism. Spatiotemporal behavior pattern mining of tourists can extract their behavioral characteristics and preferences, which is the basis for distinguishing differences in tourist behavior. Meanwhile, the construction of tourism infrastructure is important for improving the tourist attractions of the region and the travel experience of tourists. NTL data have been proven to be effective and convenient in the field of human and economic activities. They can be used not only to analyze the spatiotemporal development characteristics of the tourism industry, but also to identify the relationship between supply and demand. Therefore, in this study, we perform spatial clustering, a seasonal-trend decomposition procedure based on Loess (STL), and Spearman correlation analysis of digital footprints data to analyze the differences in spatiotemporal behavior patterns between CTs and STs. We also identify TISDP by using profit and loss value calculations of NTL data.
3. Materials and methodology
3.1. Study area
Yunnan Province is located in the southwest of the People's Republic of China () and is a well-known tourist paradise which with abundant landform types, climate types, ethnic minorities and tourism resources, making it one of the top tourist destinations for culture, heritage, and nature (Lin and Wen Citation2018). Yunnan Province has a total area of 394,100 km2 and encompasses all topographic and climatic types, except for oceans and deserts. Its rich landscapes and favorable climates attract many tourists. Complex landforms provide high-quality tourism resources for cycling. In 2022, the province received 840 million tourists and achieved a total tourism income of 944.90 billion yuan, an increase of 27.3% and 21.2%, respectively. Thus, tourism has become a trillion-yuan industry in Yunnan Province.
3.2. Data collection and processing
The data sources, collection and processing procedures employed in this study were as follows:
Flickr (https://www.flickr.com). Flickr collects various photographs from different regions of the world sets detailed attribute information, which enables it to provide rich image data and related descriptions. Research shows that Flickr data includes about 17% of Chinese users and users from other countries (Lee and Barton Citation2011). Therefore, the obtained data provide comprehensive information on both domestic and foreign tourists but are broadly more representative of STs.
Using the Flickr python API filckrapi (https://www.flickr.com/services/api/) and searching by keywords and time, this study obtained 177,944 geotagged images metadata for Yunnan Province from 2004 to 2022. We then stored the photo ID (Photo_id), user ID (Nsid), shooting time (Taken_time), longitude (Lon), latitude (Lat), url, and other attributes in csv. file. Flickr data accrue some noise data and errors during the crawling process that require cleaning. The cleaning rules and principles are as follows. (1) Delete coordinate points that are not in the study area. Points within the study area were filtered and exported using ArcGIS location-based selection and study area boundaries. Because this study investigates the spatial and temporal characteristics of tourists in the study area. (2) Delete duplicate data using python; that is, records with exactly the same Photo_id and Nsid. This is because the exact same data create data redundancy, which affects the data balance. (3) Delete records of the same traveler in the same geographic location using python; that is, records with equal Nsid, Taken_time, lon, and lat. This is because multiple records of a traveler in the same location only show repeated behaviors of the traveler visiting the destination. After processing, 28,067 records were obtained; example data are presented in .
(2) 2bulu Outdoor Travel website (https://www.2bulu.com/). This platform provides users with a medium to share travel trajectories. Users can download cycling tracks and photographs with geographical coordinates for free (Mou et al. Citation2022).
Table 1. Flickr sightseeing tourist example data.
Image-marked points form Cycling records (1,848) were obtained from 2bulu by python, which reflect the landscape preferences of the cyclists and included TrackId, UserId, Time, longitude (Lon), and latitude (Lat) as shown in . The 2bulu data is obtained by python as shapefiles but some noise data were included. Thus, the following process was performed. (1) Merge all shapefiles through ArcGIS. (2) Delete coordinate points that are not in the study area. Points within the study area were filtered and exported as csv. file using ArcGIS location-based selection and study area boundaries. (3) Delete records of the same traveler in the same geographic location using python; that is, records with the same UserId, Time, Lon, and Lat. After removing the noise data, we obtained 44,435 labeled points with images.
(3) NTL Remote Sensing Imagery Project: Annual Defense Meteorological Satellite Program (DMSP) NTL and Visible Infrared Imaging Radiometer Suite (VIIRS) NTL data sets were used in this study after consistency processing (Zhao et al. Citation2020). These data are suitable for research in mid-low latitudes such as Southeast Asia, the United States, and China.
Table 2. Cycling tourist example data.
To address the issue of the inconsistency and incomparability between DMSP and VIIRS NTL data, we used the approach of Zhao et al. (Citation2020), who integrated the following VIIRS and DMSP data processing procedures. (1) Resample annual VIIRS data using a kernel density method to achieve the same spatial resolution as DMSP data. (2) Aggregate VIIRS data using a logarithmic transformation. (3) Convert VIIRS data using the sigmoid function model. (4) Apply the quantitative relationship identified by the 2012 and 2013 NTL observations with better fitting performance to the processed VIIRS data in subsequent years. (5) Round the fitting results and correct simulated values larger than 63 to generate the simulated DMSP data (2014–2018). Finally, we obtained the time-extended NTL dataset (1992–2018), which represents annual average data with a spatial resolution of 30 arc-seconds.
3.3. Methods
3.3.1. Spatiotemporal behavior pattern mining: spatial cluster analysis and STL
Tourists document and share photographs during their travels. The location of these scenes is not necessarily conventional, but may include some characteristic buildings, streets, areas where food is concentrated, and areas where internet celebrities check in. Therefore, we defined scenic spots as popular areas that are attractive to tourists and embody tourist behavior. The spatial behavior of tourist travel mainly involves moving between scenic spots; Thus, it is necessary to extract popular areas, namely attractions, to identify the spatial behavior patterns of tourists moving between attractions. In this study, scenic spots were extracted from data points using the HDBSCAN clustering algorithm. HDBSCAN is a simple, efficient, and robust density-based hierarchical clustering method (McInnes, Healy, and Astels Citation2017) that construct a mutual reach graph by calculating the reachable distance between neighboring points and core points, and finally introduces hierarchical clustering and cluster tree compression to obtain the final clustered clusters. The steps of the HDBSCAN algorithm (Campello, Moulavi, and Sander Citation2013) are as follows:
Perform spatial transformation based on density: Use the mutual reachability distance to represent the distance between two sample points:
(1)
(1) where
indicates the Euclidean distance between p and q. The core distance is defined as:
(2)
(2) where
indicates the distance between the current sample point and its kth nearest neighbor sample point.
Build a Mutual Reachability Graph whose vertex is data point and edge weight is reachability distance between any two points. Then build a minimum spanning tree by splitting the graph according to the edge weights between the data point.
Build a clustering hierarchy: Arrange the edges in the tree in increasing order according to the reachable distance. Then, merge the two subgraphs connected to each edge in turn to generate the hierarchical clustering structure.
Compress the clustering tree: HDBSCAN extracts distinct clusters by running the single-link clustering algorithm on the pruned minimum spanning tree. These clusters correspond to regions of different density in the original data.
Extract the clusters: The algorithms assign data points to a cluster or label them as noise points.
Choosing a suitable travel date is an expression of the temporal characteristics of tourists. The decomposition of seasonal time series first proposed by (Cleveland and Tiao Citation1976), is per formed to decompose the time series into several components. Here, STL was used as the time series decomposition method, which is widely used because it can handle any type of seasonality and is robustness to outliers. STL decomposes a series into three components: Seasonality, Trends, and Residuals (Cleveland et al. Citation1990), to obtain the tourist travel trends and repeated short-term cycles in the time series, then analyze seasonal changes in tourism behavior.
3.3.2. Ethnic characteristics and infrastructure preferences: spatial correlation
The Spearman correlation coefficient (Zar Citation2005) is a nonparametric statistical method commonly used to measure the monotonic correlation between two variables. Additionally, it is insensitive to data errors and extreme values, which can reduce the influence of outliers. In this study, fishing nets were used to connect the data and extract density values, and the Spearman correlation coefficient was calculated to identify the correlation between tourist footprints, ethnic minority areas, and infrastructure.
3.3.3. TISDP for tourists and scenic spots: calculation of profit and loss
The NTL reflects the service level of lighting facilities and infrastructure around a scenic spot; a higher service level can provide travelers with a better experience. The profit and loss value can reflect the supply and demand relationship between the two factors (Liu, Huang, and Yang Citation2020). To identify whether the infrastructure around scenic spots meet the needs of tourists, this study used profit and loss values to reflect the TISDP, identify areas where supply and demand are insufficient, and analyze spatiotemporal patterns. In this study, the profit and loss values of the two were defined as
(3)
(3) where C represents the profit and loss value of the NTL infrastructure and traveler demand,
represents the value after reclassification of NTL data, and
represents the value of traveler demand after reclassification. A representative year was selected to analyze the temporal and spatial changes in NTL infrastructure around the scenic spot according to the following procedure.
Connect the travel footprint and NTL data to fishing nets of the same scale, and calculate the sum of the values in the grid.
Unify the reclassification results of NTL values in different years by classifying the year with the largest NTL value,
, according to the Jenks natural breaks algorithm (Jenks Citation1967). Other years were graded according to the same breakpoint and were reclassified (
indicates the maximum NTL value for each year).
Normalize values to
and reclassify them. Unify the reclassification results of the Flickr sightseeing and cycling footprint points using the Jenks natural breaks algorithm. Reclassify the two sets of point data based on the same breakpoint. The normalization formula is as follows:
(4)
(4) where N represents the normalized result,
represents the sum of travelers in the grid,
represents the maximum NTL value,
represents the maximum value of the sum of travelers in a single grid, and the value range of N is [0,
].
Calculate the profit and loss values of the two sets of data and analyze the profit and loss distribution and temporal and spatial changes of nighttime infrastructure.
4. Results
4.1. Spatiotemporal characteristics: spatial clustering and STL
shows the point distribution of the tourist footprints of the flicker STs number and CTs number. The popularity distributions differed between the two types of tourists. The destinations recorded by STs were widely distributed in northwest Yunnan Province, Kunming, and Honghe, whereas CTs destinations were concentrated in Kunming, Qujing, and Yuxi. Visits to Zhaotong were also popular. These differences are reflective of destination choice.
Furthermore, STs and CTs were clustered using HDBSCAN to construct an Origin-Destination (OD) flow space network, as shown in , where the colored lines represent the number of Origin-Destination routes. A linear density analysis was performed, as shown in . The main tourism spatial pattern of Yunnan Province had a triangular structure, with Kunming, Dali, and Lijiang as the main cores. Tourist movements then extended from the core to the surrounding areas. However, STs flowed to Diqing, Yuxi, Honghe, and Xishuangbanna, whereas CTs tended to flow to the surrounding cities, with Kunming as the center, illustrating the main difference in the flow of tourists between the two groups in Yunnan Province. According to the clustering results, the most popular scenic spots for STs were Dali Erhai Lake Scenic Area, Dali Ancient City, Yuenyang Hani Terrace, Tien Lake Scenic Area, Kunming Wuhua District Central Business District, Lijiang Ancient City, Green Lake Park, and the Stone Forest Scenic Area along the Jinsha River. In addition, the Yulong Snow Mountain and Meili Snow Mountain Scenic Areas had many STs, which shows the potential for snow mountain tourism. The main flow directions among the scenic spots were Kunming Green Lake Park-Erhai Lake Scenic Area, ShangriLa Dukezong Ancient City-Kunming Cuihu Park, and Stone Forest Scenic Area-Kunming Green Lake Park. Among these, the Erhai Lake Scenic Area and Green Lake Park were the most popular tourist routes. Kunming was the main transportation hub connecting various cities in Yunnan Province. The short-distance between the Kunming Railway Station and Green Lake may be the reason for the high number of visits to Green Lake. The Erhai Lake's beautiful scenery and unique humanistic customs make it a location for many popular film and television dramas (Marafa, Chan, and Li Citation2022), such as ‘The Demi-Gods and Semi-Devils’, ‘Breakup Buddies’, and ‘Rattan’. Meanwhile, in the past 2 years, Dali has continued to rise in popularity because of its leisurely lifestyle, and literary and artistic atmosphere. This may explain why Erhai Lake has become the most attractive scenic spot in the Yunnan Province.
The differences between the most popular scenic spots for CTs and STs were reflected in the Fuxian Lake Scenic Area, mountains around Qujing, West View Route, and Simao District of Pu’er City. The most popular route between scenic spots was the route along the Meili Snow Mountain – Tien Lake Scenic Area-Fuxian Lake. More obvious differences were observed on the routes to the mountains and lakes around Kunming. These differences are mainly because CTs prefer challenging routes with long paths, complex terrain, and natural landscapes such as mountains and water.
This study counted the number of tourists visiting each month, sorted them by time, and created an STL of the time series of the two types of tourists. presents the STL results of the time series for the two types of tourists. The horizontal axis represents the month from the initial time of data acquisition. (1) In terms of tourist trends, there were differences in the numbers of flicker STs and CTs. According to Flicker Tourists, the tourism trend in Yunnan first increased and then peaked in 2013. Restrictions on domestic users to access Flicker websites and the violent terrorist incident at the Kunming train station in 2014 may be the reasons for the subsequent decline in the number of tourists. Flickr is related to foreign tourist visits, and the number of tourists was very small after 2020. The number of CTs gradually increased and stabilized after mid-2020. The main reason for this was that the new corona virus epidemic restricted travel both at home and abroad, which has affected the tourism industry. (2) Both have seasonality but there are differences. The STs mainly traveled to Yunnan in April, August, and October and reached its peak in autumn. CTs were mainly concentrated in March, April, and May. Spring was the most popular travel time, followed by summer and early autumn.
4.2. Ethnic characteristics and infrastructure preferences: spatial correlation analysis of Spearman correlation coefficient
The variety of ethnic minorities in Yunnan Province has generated abundant ethnic tourism in scenic spots and surrounding human settlements (Walsh and Swain Citation2004). Zhao et al. (Citation2021) obtained a distribution of 25 ethnic minorities in Yunnan Province through the geographical name data and the census statistics of Yunnan Province, and recorded them with the point method. In this study, ethnic minorities were merged to create fishing nets and connected to distribution points to obtain the regional distribution density of ethnic minorities in Yunnan Province as shown in , where the distribution of ethnic minority areas number were displayed.
To explore the attractiveness of ethnic minority areas to tourists, we calculated the tourist-ethnic minority area correlation index as follows:
(5)
(5) where P is the traveler-minority area correlation index,
is the grid of minority areas,
is the grid with tourist footprints, N is the number of tourist footprints in minority areas, and
is the total number of tourist footprints.
The calculation results are listed in . Most footprints recorded by tourists were distributed in ethnic minority areas, indicating that these areas were tourist-biased destinations. For example, among the popular scenic spots, the Stone Forest Scenic Area, Erhai Lake Scenic Area, and Lijiang are mainly home to the Yi, Bai, and Naxi ethnic minority groups. The tourist-ethnic minority area correlation index was lower for CTs than for STs, which may be because CTs travel routes are typically farther from residential areas.
Table 3. Result of the traveler-minority area correlation index.
Moreover, we calculated the Spearman correlation coefficient between the number of minority areas and the number of tourist footprint in Yunnan Province, which reflect the ethnic characteristics of the landscape and environment and the attractiveness of the area, respectively. The correlation was close to zero for both types of tourists, indicating no monotonic correlation between the concentration of ethnic minority characteristics areas and their attractiveness to tourists.
In recent years, the tourism industry has developed rapidly and the infrastructure of scenic spots has gradually improved. NTL remote sensing data can reflect the level of human activities, economic development and the level of infrastructure. To explore tourist preferences for infrastructure, we extracted the NTL data density fishnet matrix and tourist density fishnet matrix for Yunnan Province in 2018 and calculated the Spearman correlation coefficient between the two. The distribution of NTL data in 2018 is shown in .
The correlation coefficients between tourist matrices and the NTL data matrix are shown in , indicating a weak positive correlation between the two variables. However, p < 0.001 indicates that the correlation coefficient was statistically significant. Thus, areas with higher NTL value had a greater impact on the number of tourists. This is because higher NTL value indicate more complete scenic spots and related regional infrastructure, which can provide better tourism services to tourists. However, the famous scenic spots in Yunnan Province are predominantly natural landscapes, which have less infrastructure than urban scenic spots, which may explain the weak correlation. This indicates an imbalance between the infrastructure of some attractions and the supply and demand of travelers. Furthermore, nighttime tourism is not prominent in Yunnan Province because it is highly dependent on light.
Table 4. Results of Spearman correlation coefficient for two types of tourists.
4.3. Tourism infrastructure supply and demand pattern
To accurately identify scenic spots with a low level of infrastructure and analyze the TISDP more accurately, we created a hexagonal fishing net with an almost circular shape that has a richer spatial topology relationship with a unit area of 10 km2. Flickr STs data, CTs data, and NTL 2004, 2011, and 2018 were connected to the fishing nets. Tourism and NTL data were reclassified in the grid, divided into six categories, and assigned values from zero to five. The profit and loss values were then calculated according to formula (3), with a value range of [−5, 5], where [−5, −1] indicated that the supply of lighting services at night was insufficient (a loss area); 0 indicated that the supply and demand relationship was balanced; and [1,5] indicated that the supply of infrastructure at night was sufficient (a profit area). Representative spatial distribution of the TISDP in Yunnan Province for 2004, 2011, 2018, are shown in .
Figure 8. Supply and demand pattern of tourists and infrastructure in scenic spots in Yunnan Province, China.

shows the analysis of the spatial pattern and volution of the relationship between scenic infrastructure and tourist supply and demand from 2004 to 2018. This showed that the major loss areas for ordinary STs were Diqing, Lijiang, and the northern part of Dali in Yunnan Province, in western Nujiang, in Xishuangbanna, and in southern Honghe. A few areas were distributed far from the centers of other cities. The scenic spots in northwestern Yunnan are mainly natural landscapes, such as plateau snow-capped mountains, wetlands, and canyons. In recent years, many tourists have been attracted to the beautiful scenery, but the complex landforms, low population density, and lack of shopping, accommodation, entertainment, and other infrastructure have led to an insufficient NTL supply. The loss area for CTs includes the same areas for STs as well as areas in the northern part of Yuxi, around the Fuxian Lake Scenic Area, the western mountainous area of Qujing, and the Jiaozi Mountain Scenic Area in northern Kunming. Spatial differences exist in the relationship between the two types of tourists and infrastructure supply and demand, which are related to different preferences of ordinary STs and CTs in some scenic spots analyzed in . While attracted to scenery, CTs are more inclined to desire challenging mountain and water terrain that improve the cycling experience. These results also indirectly reflect the correlation between CTs and NTL.
According to the spatial pattern of Yunnan tourism and the spatial distribution of profit and loss values, tourism development in Yunnan Province follows a multi-core collaborative development model. Around the scenic spots, the deficit areas of the two types of travelers and night service infrastructure decreased over time, whereas the profit area expanded and gradually spread to include loss areas until the profit area covered all major scenic spots in 2018. The profit area around scenic spots increased slightly from 2004 to 2011 but increased rapidly from 2011 to 2018. This is in line with the ‘Opinions on Building a Strong Tourism Province’ promoted by Yunnan Province in 2013, which aimed to vigorously develop the tourism industry. However, some areas of loss remained, including the Meili Snow Mountain, Napa Lake, Blue Moon Scenic Area, and Erhai Lake scenic areas. Taking the scenic spots in southwestern Shangri-La as an example, as shown in , no significant improvement in the TISDP occurred from 2004 to 2011, and loss areas still dominated. From 2011 to 2018, the areas surrounding scenic spots developed rapidly toward profit areas; however, the area as a whole exhibited a loss because of a lack of infrastructure at night in the snowy mountain scenic area, which is attributed to the unsuitable terrain for most tourists to travel at night. In the case of insufficient infrastructure, facilities tend to be closed at night, resulting in a lack of light. However, tourists with night travel needs cannot obtain better services.
Figure 9. Part of the scenic spot in the southwest of Shangri-La in (a) 2004, (b) 2011, and (c) 2018.

Identifying the loss and profit area based on the profit and loss values can help decision makers further analyze the types of tourist facilities that are lacking in the area. For example, CTs may rely more on catering and road lights, whereas STs may rely more on accommodation and entertainment. Combined with the characteristics of scenic spots and the above analysis can enable more effective regional planning.
5. Discussion
The Increase in different types of tourists require more sophisticated tourism management and planning of tourism infrastructure. Driven by this demand, we proposed a new data-driven framework for mining differences in tourist behavior from a novel perspective. This framework accurately reveals the temporal and spatial behavioral differences and the TISDP.
The purpose was to explore the differences in spatiotemporal characteristics and preferences of STs and CTs, and to identify TISDP. The study found obvious differences between two types of tourists in the choice of destinations and the spatial flow between scenic spots. The overall tourism characteristics of destinations and destination flows in Yunnan are consistent with those found by Liu et al. (Citation2021). However, the differences are reflected in the following. (1) Although both types of tourists have similar preferences for popular scenic spots, the concentration of popular scenic spots is more obvious for STs and includes fewer visits to non-traditional scenic spots, whereas CTs choose a greater variety of scenic spots. (2) Different scenic spot preferences lead to different flow directions. STs show the flow direction from Kunming (Green Lake, Tien Lake, Stone Forest), Dali (Erhai Lake, ancient city), and Lijiang (ancient city, Jade Dragon Snow Mountain). In addition to the flow to popular scenic spots, CTs showed that they liked the route from Kunming to Shangri-La, the scenery of Fuxian Lake, and the mountains of the city near Kunming.
The reason for these differences may be that CTs search for the traditional characteristics of territories based on tangible and intangible elements to achieve a unique tourist experience that differs significantly from that of mass-tourism experiences (Gazzola et al. Citation2018). Moreover, mountain biking and cycling around the lake are more enjoyable and challenging and enable more exercise and experience of natural scenery. However, STs are more satisfied with scenic spots with better infrastructure (Blazeska, Strezovski, and Klimoska Citation2018), which improves their overall experience. Social media and the internet may also effect the choice of popular destinations for STs (Lin and Huang Citation2006). Transportation conditions are another important factor determining the attractiveness of destinations and the scale of tourist flow (Martín and Fernández Citation2022). Furthermore, factors such as the tourists themselves, the environment and the infrastructure lead to differences in visitor behavior. All of these factors will continue to exist in the future. Therefore, identifying these spatial characteristics can provide guidance for tourist travel route planning and the sales of tourism products. Such research can also be used to advertise the landscape to STs as well as unique or challenging routes to CTs, enabling the development of personalized tourism and the sustainable development of cycling tourism.
The choice of travel dates also differs between the two types of tourists, with both exhibiting seasonality (a relatively stable cycle of fluctuation). STs tend to arrive in Yunnan in late spring and particularly autumn, whereas CTs generally travel in spring, as well as late autumn and early winter. Climate and weather conditions have considerable impact on cycling travel behavior, especially rain (Gebhart and Noland Citation2014; Meng et al. Citation2016). These findings are consistent with the results of Fang and Yin (Citation2015), indicating that spring, autumn and winter are suitable tourism seasons and that climate has an impact on the suitability of tourism in Yunnan. Yunnan is warm, dry, and rain free during the spring. However, rainfall is concentrated and heavy in summer, with the rainy season lasts from May to October. Flood is also common during the rainy season of summer and autumn. Thus, the number of CTs is lower in the summer and early fall. This demonstrates the influence of weather on CTs. Autumn is cool, and the temperature in winter is relatively low when it rains. The temperatures in spring and autumn are suitable, and there is less rain; therefore, these seasons are suitable for tourism. Tourists in autumn not only experience various scenic spots but also enjoy unique attractions, such as snowy mountain landscapes and Siberia Black-headed Gull in Tien Lake. Therefore, identifying temporal tourist behavior patterns can help tourism management departments regulate tourism resources, transportation resources and tourism flow.
According to the temporal trend in the number of tourists, an initial increase in visits was followed by a decrease, which may be attributed to domestic network access restrictions and violent terrorist incidents in 2013, as well as the COVID-19 pandemic in 2020 (Luo et al. Citation2021). CTs have been increasing in number annually, but the trend has stabilized over the past 2 years and has also been influenced by the novel coronavirus epidemic (Abbas et al. Citation2021).
Another major feature of Yunnan is its diverse ethnic minority landscape (Yang Citation2011). Both types of tourists like to visit minority areas, but the correlation shows that the numbers of tourists does not have a significant correlation with the concentration of ethnic characteristics. There are two possible reasons for this finding. (1) The widespread distribution of ethnic minority areas and unbalanced development of ethnic minorities in Yunnan (Jianlei et al. Citation2019). (2) Most tourists do not typically visit or experience ordinary ethnic landscapes but instead visit the popular scenic spots of ethnic minorities, and visiting scattered ethnic minorities is less attractive. Comparing the two types of tourists, less CTs visit minority areas because cycling routes are typically far from residential areas. This indicates that the characteristics of ethnic minorities areas still require further development. Understanding this situation can guide the governments to promote minority economies through tourism. That is, by increasing the attractiveness of ethnic minority landscapes and promoting the traditional culture of ethnic minorities. This is important for sustainable regional development and poverty reduction (Tu and Zhang Citation2020).
Good infrastructure in scenic spots can also increase tourist attractiveness to a region (Lee, Chen, and Huang Citation2014). This is evidenced by the correlation between tourists and NTL data. STs prefer scenic spots with better infrastructure, reflected by NTL data such as street lights, night service areas, and restaurants and commercial areas open during the night.
Knowing this feature, it is meaningful to improve tourism infrastructure to attract tourists. Although infrastructure alone is insufficient for improving tourism competitiveness, it is crucial for attracting visitors to tourism destinations and meeting their needs once they arrive (Cao et al. Citation2022). The NTL data show that Yunnan's tourism infrastructure has continuously improved in recent years, yet infrastructure loss areas still remain. This indicates an imbalance between the supply and demand of tourists and infrastructure in these areas. Fewer loss areas exist for STs than for CTs, with CTs loss areas in Qujing, northern Kunming, and Fuxian Lake. As many tourist routes of CTs are in economically underdeveloped areas, such as mountainous areas, this also confirms the spatial characteristics of CTs. Thus, from the perspective of infrastructure, local governments should improve infrastructure based on the identified loss areas. However, the type of infrastructure to improve, e.g. roads, streetlights, restaurants, or commercial areas, should be further analyzed according to tourist needs. Such research can ensure the more sustainable development of tourism infrastructure with fewer negative impacts on the ecological environment and human settlements (Hall Citation2019).
6. Conclusions
In this study, we present a novel research framework from the perspective of differences in the spatiotemporal behavioral characteristics of different types of tourists, specifically STs and CTs, that combines digital footprints, NTL, and ethnic minority data. A fast and accurate method based on NTL data is applied to TISDP identification. By exploring the behavioral differences between STs and CTs, this research reveals the degree of infrastructure improvement required to promote tourism in Yunnan Province, China. The results highlight clear spatial differences between the two types of tourists, which are reflected in hotspots and tourism flows. Temporal differences are reflected by their travel period, that is, autumn for STs and spring for CTs. And research found ethnic minority areas and well-established infrastructure are more attractive to both types of tourists, especially to STs. From a tourism infrastructure perspective, Yunnan tourism exhibits multi-core collaborative development. According to NTL data of scenic spot, tourism in Yunnan Province has developed rapidly in recent years. However, NTL loss areas indicate that the infrastructure of some popular scenic spots and those dominated by natural landscapes remains incomplete, resulting in an imbalance between that the TISDP and tourists, which can be further planned and improved.
By distinguishing behavioral differences and infrastructure information between different types of tourists, this research provides key information for management. The research program further distinguishes the behavioral patterns of tourists in the subdivided tourism market and expands the research direction. Simultaneously, to avoid excess infrastructure and tourism bubbles (Hiernaux-Nicolas Citation2015), it is important to improve tourism infrastructure by identifying loss areas.
However, it was difficult to remove the small amount of CTs data from the flicker data, which may have affected the characteristics of the STs. In the future, attempts can be made to remove cycling data and other noise data by using textual descriptions or image recognition. Moreover, this study was conducted on a large time and space of the study are large temporal and spatial with scenic spots as the identifying unit and the overall trends and seasonality analyzed over time. The resulting tourism characteristics are important for guiding tourism planning. However, on a smaller scale, such as identifying the characteristics of tourists within a particular scenic area or during a certain period, is more difficult to obtain. Finally, we didn't perform a quantitative analysis of the factors influencing the spatiotemporal behavior and TISDP characteristics of tourists, such as the quantitative impact of traffic on tourist behavior and the causes of specific losses in the TISDP analysis. The above limitations will be directions for future research.
Acknowledgements
We would like to express our great appreciation to the editors and all anonymous reviewers for constructive comments that helped improve the manuscript.Meanwhile, thanks to editage (https://www.editage.cn/) for its professional support.
Disclosure statement
No potential conflict of interest was reported by the author(s).
Data availability statement
Tourist data is obtained for free from 2bulu website (https://www.2bulu.com/) and Flickr (https://www.flickr.com). Nighttime light data is provided by the Earth Observation Group (EOG) (https://eogdata.mines.edu/download_dnb_composites.html, accessed on 28 May 2020). And the data that support the findings of this study are available from the corresponding author upon reasonable request.
Additional information
Funding
References
- Abbas, J., R. Mubeen, P. T. Iorember, S. Raza, and G. Mamirkulova. 2021. “Exploring the Impact of COVID-19 on Tourism: Transformational Potential and Implications for a Sustainable Recovery of the Travel and Leisure Industry.” Current Research in Behavioral Sciences 2: 100033. https://doi.org/10.1016/j.crbeha.2021.100033.
- Blazeska, D., Z. Strezovski, and A. M. Klimoska. 2018. “The Influence of Tourist Infrastructure on the Tourist Satisfaction in Ohrid.” UTMS Journal of Economics 9 (1): 85–93.
- Bouchet, P., and C. Sobry. 2019. “Sports Tourism: Contemporary Issues and New Trends on a Global Market.” In The Global Sport Economy, edited by M. Desbordes, P. Aymar, and C. Hautbois, 295–317. London: Routledge.
- Campello, R. J., D. Moulavi, and J. Sander. 2013. “Density-Based Clustering Based on Hierarchical Density Estimates.” In Advances in Knowledge Discovery and Data Mining: 17th Pacific-Asia Conference, PAKDD 2013, Gold Coast, Australia, April 14–17, 2013, Proceedings, Part II 17, edited by J.P. Mining, V.S. Tseng, L. Cao, H. Motoda, and G. Xu, 160–172. Australia: Springer.
- Cao, Q., M. N. I. Sarker, D. Zhang, J. Sun, T. Xiong, and J. Ding. 2022. “Tourism Competitiveness Evaluation: Evidence from Mountain Tourism in China.” Frontiers in Psychology 13: 809314. https://doi.org/10.3389/fpsyg.2022.809314.
- Chang, P., X. Pang, X. He, Y. Zhu, and C. Zhou. 2022a. “Exploring the Spatial Relationship between Nighttime Light and Tourism Economy: Evidence from 31 Provinces in China.” Sustainability 14 (12): 7350. https://doi.org/10.3390/su14127350.
- Chang, D., Q. Wang, J. Xie, J. Yang, and W. Xu. 2022b. “Research on the Extraction Method of Urban Built-Up Areas with an Improved Night Light Index.” IEEE Geoscience and Remote Sensing Letters 19: 1–5. https://doi.org/10.1109/LGRS.2022.3179846.
- Choe, Y., H. Kim, and H.-J. Joun. 2019. “Differences in Tourist Behaviors across the Seasons: The Case of Northern Indiana.” Sustainability 11 (16): 4351. https://doi.org/10.3390/su11164351.
- Cleveland, R. B., W. S. Cleveland, J. E. McRae, and I. Terpenning. 1990. “STL: A Seasonal-Trend Decomposition.” Journal of Official Statistics 6 (1): 3–73.
- Cleveland, W. P., and G. C. Tiao. 1976. “Decomposition of Seasonal Time Series: A Model for the Census X-11 Program.” Journal of the American Statistical Association 71 (355): 581–587. https://doi.org/10.1080/01621459.1976.10481532.
- Das, S., S. Ahmed, S. Haque, and S. Ismail. 2021. Assessing Correlation between Night-Time Light and Road Infrastructure: An Empirical Study. Center for Spatial Studies, UC Santa Barbara, Los Angeles, the United States of America.
- Dong, W., Q. Kang, G. Wang, B. Zhang, and P. Liu. 2023. “Spatiotemporal Behavior Pattern Differentiation and Preference Identification of Tourists from the Perspective of Ecotourism Destination Based on the Tourism Digital Footprint Data.” PLoS One 18 (4): e0285192. https://doi.org/10.1371/journal.pone.0285192.
- Edwards, D., and T. Griffin. 2013. “Understanding Tourists’ Spatial Behaviour: GPS Tracking as an Aid to Sustainable Destination Management.” Journal of Sustainable Tourism 21 (4): 580–595. https://doi.org/10.1080/09669582.2013.776063.
- Ellis, A., E. Park, S. Kim, and I. Yeoman. 2018. “What is Food Tourism?” Tourism Management 68: 250–263. https://doi.org/10.1016/j.tourman.2018.03.025.
- Fang, Y., and J. Yin. 2015. “National Assessment of Climate Resources for Tourism Seasonality in China Using the Tourism Climate Index.” Atmosphere 6 (2): 183–194. https://doi.org/10.3390/atmos6020183.
- Gazzola, P., E. Pavione, D. Grechi, and P. Ossola. 2018. “Cycle Tourism as a Driver for the Sustainable Development of Little-Known or Remote Territories: The Experience of the Apennine Regions of Northern Italy.” Sustainability 10 (6): 1863. https://doi.org/10.3390/su10061863.
- Gebhart, K., and R. B. Noland. 2014. “The Impact of Weather Conditions on Bikeshare Trips in Washington, DC.” Transportation 41 (6): 1205–1225. https://doi.org/10.1007/s11116-014-9540-7.
- Gibson, J., S. Olivia, and G. Boe-Gibson. 2020. “Night Lights in Economics: Sources and Uses.” Journal of Economic Surveys 34 (5): 955–980. https://doi.org/10.1111/joes.12387.
- Girardin, F., F. Calabrese, F. Dal Fiore, C. Ratti, and J. Blat. 2008. “Digital Footprinting: Uncovering Tourists with User-Generated Content.” IEEE Pervasive Computing 7 (4): 36–43. https://doi.org/10.1109/MPRV.2008.71.
- Hall, C. M. 2019. “Constructing Sustainable Tourism Development: The 2030 Agenda and the Managerial Ecology of Sustainable Tourism.” Journal of Sustainable Tourism 27 (7): 1044–1060. https://doi.org/10.1080/09669582.2018.1560456.
- Han, H., L. H. Lho, A. Al-Ansi, and J. Yu. 2020. “Cycling Tourism: A Perspective Article.” Tourism Review 75 (1): 162–164. https://doi.org/10.1108/TR-06-2019-0268.
- Han, H., B. Meng, and W. Kim. 2017. “Bike-Traveling as a Growing Phenomenon: Role of Attributes, Value, Satisfaction, Desire, and Gender in Developing Loyalty.” Tourism Management 59: 91–103. https://doi.org/10.1016/j.tourman.2016.07.013.
- Han, J., Q. Ming, C. Xie, P. Shi, X. Wu, and K. Yin. 2019. “Analysis and Implications of Travel Experience and Perception in Border Ethnic Areas Based on Online Text Mining—A Case Study of Xishuangbanna in Yunnan Province.” In Vol. 1325 of Journal of Physics: Conference Series. J. Phys: Conf. Ser., 012053. Hang Zhou, China: IOP Publishing.
- He, X., Z. Li, Q. Li, Z. Chen, and Y. She. 2018. “On the Spatio-Temporal Characteristics of Tourists in Scenic Areas Based on Digital Footprint—A Case Study of Tourists in Zhangjiajie.” Journal of Natural Science of Hunan Normal University 41 (1): 11–17.
- Hiernaux-Nicolas, D. 2015. “Tourism and Strategic Competitiveness: Infrastructure Development in Mexico City.” In The Infrastructure of Play. edited by D. R. Judd, 202–212. New York: Routledge.
- Huang, X., M. Li, J. Zhang, L. Zhang, H. Zhang, and S. Yan. 2020. “Tourists’ Spatial-Temporal Behavior Patterns in Theme Parks: A Case Study of Ocean Park Hong Kong.” Journal of Destination Marketing & Management 15: 100411. https://doi.org/10.1016/j.jdmm.2020.100411.
- Ibănescu, B.-C., O. M. Stoleriu, and A. Gheorghiu. 2018. “Gender Differences in Tourism Behaviour in the European Union.” Eastern Journal of European Studies 9 (1): 23–43.
- Inkson, C., and L. Minnaert. 2022. Tourism Management: An Introduction. Sage: Thousand Oaks.
- Jenks, G. F. 1967. “The Data Model Concept in Statistical Mapping.” International Yearbook of Cartography 7: 186–190.
- Jovanoviä, S., and I. Ivana. 2016. “Infrastructure as Important Determinant of Tourism Development in the Countries of Southeast Europe.” Ecoforum Journal 5 (1): 288–294.
- Kabanda, T. 2022. “Using Land Cover, Population, and Night Light Data to Assess Urban Expansion in Kimberley, South Africa.” South African Geographical Journal 104 (4): 539–552. https://doi.org/10.1080/03736245.2022.2028667.
- Kang, M., and G. Moscardo. 2006. “Exploring Cross-Cultural Differences in Attitudes towards Responsible Tourist Behaviour: A Comparison of Korean, British and Australian Tourists.” Asia Pacific Journal of Tourism Research 11 (4): 303–320. https://doi.org/10.1080/10941660600931143.
- Kim, C., and S. Lee. 2000. “Understanding the cultural differences in tourist motivation between Anglo-American and Japanese tourists.” Journal of Travel & Tourism Marketing, 9 (1-2): 153–170.
- Kinash, I., L. Arkhypova, A. Polyanska, O. Dzoba, U. Andrusiv, and I. I. Iuras. 2019. “Economic Evaluation of Tourism Infrastructure Development in Ukraine.” In Vol. 477 of IOP Conference Series: Materials Science and Engineering. J. Phys: Conf. Ser., 012020. Hang Zhou, China: IOP Publishing.
- Kirilenko, A. P., S. O. Stepchenkova, and J. M. Hernandez. 2019. “Comparative Clustering of Destination Attractions for Different Origin Markets with Network and Spatial Analyses of Online Reviews.” Tourism Management 72: 400–410. https://doi.org/10.1016/j.tourman.2019.01.001.
- Korpilo, S., T. Virtanen, and S. Lehvävirta. 2017. “Smartphone GPS Tracking—Inexpensive and Efficient Data Collection on Recreational Movement.” Landscape and Urban Planning 157: 608–617. https://doi.org/10.1016/j.landurbplan.2016.08.005.
- Krikigianni, E., C. Tsiakos, and C. Chalkias. 2019. “Estimating the Relationship between Touristic Activities and Night Light Emissions.” European Journal of Remote Sensing 52 (sup1): 233–246. https://doi.org/10.1080/22797254.2019.1582305.
- Lee, C. K., and D. Barton. 2011. “Constructing Glocal Identities through Multilingual Writing Practices on Flickr.com®.” International Multilingual Research Journal 5 (1): 39–59. https://doi.org/10.1080/19313152.2011.541331.
- Lee, C. F., P. T. Chen, and H. I. Huang. 2014. “Attributes of Destination Attractiveness in Taiwanese Bicycle Tourism: The Perspective of Active Experienced Bicycle Tourists.” International Journal of Hospitality & Tourism Administration 15 (3): 275–297. https://doi.org/10.1080/15256480.2014.925726.
- Li, W., and K. Wang. 2020. “The Spatial and Temporal Characteristics and Development of Rural Self-Driving Tour in Henan Province Based on Digital Footprint.” Journal of Yunnan Agricultural University (Social Science 14 (3): 79–85. https://doi.org/10.3969/j.issn.1004-390X(s).201910005.
- Lin, Y., and J. Huang. 2006. “Internet Blogs as a Tourism Marketing Medium: A Case Study.” Journal of Business Research 59 (10–11): 1201–1205. https://doi.org/10.1016/j.jbusres.2005.11.005.
- Lin, Q., and J. J. Wen. 2018. “Tea Tourism and Its Impacts on Ethnic Marriage and Labor Division.” Journal of China Tourism Research 14 (4): 461–483. https://doi.org/10.1080/19388160.2018.1511490.
- Liu, D., A. Huang, and F. Yang. 2020. “Research on the Spatial Distribution Characteristics and Supply–Demand Balance of Tourism Public Facilities Based on Multi-Source Data—A Case Study of Xiamen City in Fujian Province.” Resource Development and Market 36 (10): 1178–1184.
- Liu, Y., W. Liu, Y. Lin, X. Zhang, J. Zhou, B. Wei, G. Nie, and L. Gross. 2023a. “Urban Waterlogging Resilience Assessment and Postdisaster Recovery Monitoring Using NPP-VIIRS Nighttime Light Data: A Case Study of the ‘July 20, 2021’ Heavy Rainstorm in Zhengzhou City, China.” International Journal of Disaster Risk Reduction 90: 103649. https://doi.org/10.1016/j.ijdrr.2023.103649.
- Liu, Y., W. Liu, X. Zhang, Y. Lin, G. Zheng, Z. Zhao, H. Cheng, et al. 2023b. “Nighttime Light Perspective in Urban Resilience Assessment and Spatiotemporal Impact of COVID-19 from January to June 2022 in Mainland China.” Urban Climate, 50: 101591. https://doi.org/10.1016/j.uclim.2023.101591.
- Liu, T., Y. Zhang, H. Zhang, and X. Yang. 2021. “A Methodological Workflow for Deriving the Association of Tourist Destinations Based on Online Travel Reviews: A Case Study of Yunnan Province, China.” Sustainability 13 (9): 4720. https://doi.org/10.3390/su13094720.
- Luo, Y., Y. Li, G. Wang, and Q. Ye. 2021. “Agent-Based Modeling and Simulation of Tourism Market Recovery Strategy after COVID-19 in Yunnan, China.” Sustainability 13 (21): 11750. https://doi.org/10.3390/su132111750.
- Mandić, A., Ž Mrnjavac, and L. Kordić. 2018. “Tourism Infrastructure, Recreational Facilities and Tourism Development.” Tourism and Hospitality Management 24 (1): 41–62. https://doi.org/10.20867/thm.24.1.12.
- Marafa, L. M., C.-S. Chan, and K. Li. 2022. “Market Potential and Obstacles for Film-Induced Tourism Development in Yunnan Province in China.” Journal of China Tourism Research 18 (2): 245–267. https://doi.org/10.1080/19388160.2020.1819498.
- Martín, J. M. M., and J. A. S. Fernández. 2022. “The Effects of Technological Improvements in the Train Network on Tourism Sustainability. An Approach Focused on Seasonality.” Sustainable Technology and Entrepreneurship 1 (1): 100005. https://doi.org/10.1016/j.stae.2022.100005.
- McInnes, L., J. Healy, and S. Astels. 2017. “hdbscan: Hierarchical Density Based Clustering.” The Journal of Open Source Software 2 (11): 205. https://doi.org/10.21105/joss.00205.
- Meng, F., and M. Uysal. 2008. “Effects of Gender Differences on Perceptions of Destination Attributes, Motivations, and Travel Values: An Examination of a Nature-Based Resort Destination.” Journal of Sustainable Tourism 16 (4): 445–466. https://doi.org/10.1080/09669580802154231.
- Meng, M., J. Zhang, Y. D. Wong, and P. H. Au. 2016. “Effect of Weather Conditions and Weather Forecast on Cycling Travel Behavior in Singapore.” International Journal of Sustainable Transportation 10 (9): 773–780. https://doi.org/10.1080/15568318.2016.1149646.
- Mou, N., Z. Liu, Y. Zheng, T. Makkonen, T. Yang, and L. Zhang. 2022. “Cycling in Tibet: An Analysis of Tourists’ Spatiotemporal Behavior and Infrastructure.” Tourism Management 88: 104418. https://doi.org/10.1016/j.tourman.2021.104418.
- Pandey, B., C. Brelsford, and K. C. Seto. 2022. “Infrastructure Inequality Is a Characteristic of Urbanization.” Proceedings of the National Academy of Sciences 119 (15): e2119890119. https://doi.org/10.1073/pnas.2119890119.
- Petrova, M., N. Dekhtyar, O. Klok, and O. Loseva. 2018. “Regional Tourism Infrastructure Development in the State Strategies.” Problems and Perspectives in Management 16 (4): 259–274. https://doi.org/10.21511/ppm.16(4).2018.22.
- Qin, X., X. Li, W. Chen, H. Tan, L. Luo, and X. Xu. 2022. “Tourists’ Digital Footprint: The Spatial Patterns and Development Models of Rural Tourism Flows Network in Guilin, China.” Asia Pacific Journal of Tourism Research 27 (12): 1336–1354. https://doi.org/10.1080/10941665.2023.2166420.
- Ridwana, R., and S. Himayah. 2020. “Utilization of Remote Sensing Technology and Geographic Information Systems for Tourism Development.” International Journal of Applied Sciences in Tourism and Events 4 (2): 158–169. https://doi.org/10.31940/ijaste.v4i2.2042.
- Salas-Olmedo, M. H., B. Moya-Gómez, J. C. García-Palomares, and J. Gutiérrez. 2018. “Tourists’ Digital Footprint in Cities: Comparing Big Data Sources.” Tourism Management 66: 13–25. https://doi.org/10.1016/j.tourman.2017.11.001.
- Song, L., J. Wang, Y. Zhang, F. Zhao, S. Zhu, L. Jiang, Q. Du, X. Zhao, and Y. Li. 2022. “Sliding Window Detection and Analysis Method of Night-Time Light Remote Sensing Time Series—A Case Study of the Torch Festival in Yunnan Province, China.” Remote Sensing 14 (20): 5267. https://doi.org/10.3390/rs14205267.
- Tu, J., and D. Zhang. 2020. “Does Tourism Promote Economic Growth in Chinese Ethnic Minority Areas? A Nonlinear Perspective.” Journal of Destination Marketing & Management 18: 100473. https://doi.org/10.1016/j.jdmm.2020.100473.
- Walsh, E. R., and M. B. Swain. 2004. “Creating Modernity by Touring Paradise: Domestic Ethnic Tourism in Yunnan, China.” Tourism Recreation Research 29 (2): 59–68. https://doi.org/10.1080/02508281.2004.11081444.
- Wang, Y., Z. Wang, Z. Deng, P. Cheng, and Y. Li. 2019. “Spatial Coupling Analysis between POI, Luminous Remote Sensing and Weibo Sign-in Data: A Case Study of Beijing.” Remote Sensing Information 34 (06): 18–26.
- Wu, J., Y. Tu, Z. Chen, and B. Yu. 2022. “Analyzing the Spatially Heterogeneous Relationships between Nighttime Light Intensity and Human Activities across Chongqing, China.” Remote Sensing 14 (22): 5695. https://doi.org/10.3390/rs14225695.
- Xue, L., and Y. Zhang. 2020. “The Effect of Distance on Tourist Behavior: A Study Based on Social Media Data.” Annals of Tourism Research 82: 102916. https://doi.org/10.1016/j.annals.2020.102916.
- Yang, L. 2011. “Ethnic Tourism and Cultural Representation.” Annals of Tourism Research 38 (2): 561–585. https://doi.org/10.1016/j.annals.2010.10.009.
- Ye, T., N. Zhao, X. Yang, Z. Ouyang, X. Liu, Q. Chen, K. Hu, et al. 2019. “Improved Population Mapping for China Using Remotely Sensed and Points-of-Interest Data within a Random Forests Model.” Science of the Total Environment 658: 936–946. https://doi.org/10.1016/j.scitotenv.2018.12.276.
- Yu, B., M. Tang, Q. Wu, C. Yang, S. Deng, K. Shi, C. Peng, J. Wu, and Z. Chen. 2018. “Urban Built-Up Area Extraction from Log-Transformed NPP-VIIRS Nighttime Light Composite Data.” IEEE Geoscience and Remote Sensing Letters 15 (8): 1279–1283. https://doi.org/10.1109/LGRS.2018.2830797.
- Zar, J. H. 2005. “Spearman Rank Correlation.” Encyclopedia of Biostatistics 7. https://doi.org/10.1002/0470011815.b2a15150.
- Zeng, L., T. Liu, and P. Du. 2022. “Research Method of Temporal and Spatial Distribution Pattern of Night-Time Economy Based on Multi-Source Data.” Journal of Geo-Information Science 24 (01): 38–49. https://doi.org/10.12082/dqxxkx.2022.210212.
- Zeng, W., Y. Zhong, D. Li, and J. Deng. 2021. “Classification of Recreation Opportunity Spectrum Using Night Lights for Evidence of Humans and POI Data for Social Setting.” Sustainability 13 (14): 7782. https://doi.org/10.3390/su13147782.
- Zhang, Q., N. Levin, C. Chalkias, and H. Letu. 2015. “Nighttime Light Remote Sensing: Monitoring Human Societies from Outer Space.” In Remote Sensing Handbook, edited by P.S. Thenkabail, 289–310. Boca Raton: CRC Press.
- Zhang, S., and H. Wei. 2022. “Identification of Urban Agglomeration Spatial Range Based on Social and Remote-Sensing Data—For Evaluating Development Level of Urban Agglomeration.” ISPRS International Journal of Geo-Information 11 (8): 456. https://doi.org/10.3390/ijgi11080456.
- Zhao, F., L. Song, Z. Peng, J. Yang, G. Luan, C. Chu, J. Ding, S. Feng, Y. Jing, and Z. Xie. 2021. “Night-Time Light Remote Sensing Mapping: Construction and Analysis of Ethnic Minority Development Index.” Remote Sensing 13 (11): 2129. https://doi.org/10.3390/rs13112129.
- Zhao, M., Y. Zhou, X. Li, C. Zhou, W. Cheng, M. Li, and K. Huang. 2020. “Building a Series of Consistent Night-Time Light Data (1992–2018) in Southeast Asia by Integrating DMSP-OLS and NPP-VIIRS.” IEEE Transactions on Geoscience and Remote Sensing 58 (3): 1843–1856. https://doi.org/10.1109/TGRS.2019.2949797.
- Zhu, J., Z. Lang, S. Wang, M. Zhu, J. Na, and J. Zheng. 2023. “Using Dual Spatial Clustering Models for Urban Fringe Areas Extraction Based on Night-Time Light Data: Comparison of NPP/VIIRS, Luojia 1-01, and NASA’s Black Marble.” ISPRS International Journal of Geo-Information 12 (10): 408. https://doi.org/10.3390/ijgi12100408.