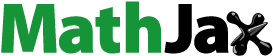
ABSTRACT
Evaluating the inequity of healthcare accessibility across demographic groups in the post-COVID era is of critical importance for an aging society like Japan – it helps to achieve better social equity via distributing healthcare resources in health planning and policy making. Our study contributes to the first post-covid evaluation of multi-modal healthcare accessibility in Tokyo, Japan, the most populated metropolis in the world. A further novelty goes to the multi-dimensional examination of the inequity of healthcare accessibility (i.e. hospitals) by public transit, driving and walking – the horizontal inequity across urban space and the vertical inequity across three demographic groups (the young, adult and elderly) through network analysis, spatial accessibility analysis and inequity indexing. We find that low healthcare access areas mainly appear in the peri-urban space as well as regions less covered by public transit. Compared to the adult group, the elderly group experiences significant inequity of healthcare access particularly in the peri-urban areas where driving is the dominant transport mode to access healthcare facilities. We provide timely evidence to the Japanese government and health authorities to have a holistic and latest understanding of multi-modal healthcare access across different demographic groups in the post-COVID era.
1. Introduction
The outbreak of COVID-19 has caused global concerns in many countries’ national public health systems and disproportional impacts on different age groups (Chiriboga et al. Citation2020). Clinic services and medical resources were prioritised for COVID-19 patients, exacerbating the inequity of healthcare access that had been observed in many countries before the COVID-19 outbreak (Krouse Citation2020). Meanwhile, the economic downturn and unemployment induced by COVID-19 has shifted labour forces, accelerating reverse migration from urban to rural space where living costs are lower (Dandekar and Ghai Citation2020). Such a changing population landscape may further widen the inequity of healthcare access over living space and across populations with various demographic and socioeconomic backgrounds. Among them, the elderly are the most vulnerable group, not only in exposure to viral transmission but also in the face of the onsite burden of diseases, vaccination, and health outcomes (Daoust Citation2020). Thus, it is critical to evaluate the inequity of healthcare access across different demographic groups, particularly the elderly, in the period towards the later stage of the COVID-19 pandemic. As advocated by the World Health Organization (Citation2020) and the United Nations (Citation2020), addressing healthcare inequity is urgently needed by each government. This is particularly the case for a super-aging society like Japan – a nation with the highest proportion of elderly in the world by 2022 (The World Bank Citation2022).
Although the Japanese government has put tremendous efforts into controlling COVID-19 transmission, the national healthcare system has encountered increasing challenges along the multi-wave pandemic timeline (Ghaznavi et al. Citation2022; Makiyama et al. Citation2021). As the primary task for policy making and implementation in the post-COVID era, evaluating healthcare access in Japan needs to take into account the demand of different age groups and the utility of multiple transport modes, given most Japanese cities are connected by public transit (Tanimoto and Hanibuchi Citation2021). There were a few limited studies evaluating the inequity of healthcare access in the pre-COVID-19 Japan (Ito et al. Citation2017; Shakya et al. Citation2018; Shinjo and Aramaki Citation2012; Watanabe and Hashimoto Citation2012), although they were largely survey-based or policy-oriented or conducted at a coarse level (e.g. town, city or prefecture). A recent work examined the accessibility of primary healthcare facilities in Fukuoka City, Japan but did not distinguish the differences in healthcare access by various transport modes and/or across age groups (Du and Zhao Citation2022). What is lacking in the literature is a timely and fine-level evaluation of healthcare access in the post-COVID era, considering both transport modes and demographic groups – the primary objective that this study aims to achieve.
Drawing on the latest 2022 census data at the finest level (chome in Japanese or census blocks), road network and medical data in 2022, the novelty of this study is to provide a grained-level post-covid valuation of the inequity of multi-modal healthcare accessibility by public transit, driving and walking in two dimensions (Jayaraj and Subramanian Citation2006) – the horizontal inequity across urban space and the vertical inequity across three demographic groups (the young, adult and elderly) in Tokyo Metropolis, the most populous and aging metropolitan region in the world. Tokyo Metropolis positions in the Kanto region in the middle of Japan. It is a long, narrow metropolitan region, running about 90 kilometres east to west and 25 km north to south, with around 2,200 square kilometres and 14 million populations in 2022 (Statistics Bureau of Japan Citation2022a). The central Tokyo Metropolis consists of 23 special wards along the coast of Tokyo Bay while its inland part, the Tama region, () consists of 26 cities, 3 towns, and 1 village (Tokyo Metropolitan Government Citation2022). The literature has widely employed.
Figure 1. Geographic context of Tokyo Metropolis. (1) Location of Tokyo Metropolis in Japan; (2) Administrative areas of Tokyo Metropolis, including 23 special wards in the east towards the coast of Tokyo Bay and 26 cities in the Tama region which were further divided as South Tama, West Tama and North Tama according to ‘three Tama’ (san-Tama) classification by Tokyo Metropolitan Government; (3) Population density of Tokyo Metropolis at the census block (chome) level; and (4) the number of hospitals at the census block level.
Sources: Ministry of Land, Infrastructure, Transport and Tourism (Citation2022) and Statistics Bureau of Japan (2022).

The literature has widely employed geographic information system (GIS)-based framework and approaches to address real-world problems (e.g. Black et al. Citation2004; Kim, Byon, and Yeo Citation2018; Yang, Goerge, and Mullner Citation2006). Following these studies, we constructed a GIS-based analytical framework to tackle the below three research questions centred around horizonal and vertical equity of healthcare access: (1) How does multi-modal healthcare access vary across space? (2) What are the inequities of healthcare access across demographic groups? and (3) Where are the areas that most need to enhance services for healthcare access, in particular for the elderly? Through the network analysis, spatial accessibility analysis and inequity indexing, our key findings contribute spatially explicit and timely evidence to policymakers and planning authorities for better health initiatives in Japan. Our analytical framework can be further applied to cope with future public health emergencies in the post-COVID era.
2. Background
2.1. Measures of healthcare accessibility
Accessibility, a term frequently used in transportation research and related planning, describes the ease or difficulty of reaching a destination from a specific location using various transportation modes (Politis et al. Citation2021; Vulevic Citation2016). In the context of public facilities, including healthcare services, accessibility is often defined by the spatial interaction between those seeking services and the providers of these services (Xu et al. Citation2020). Various methods are employed to assess the spatial accessibility of public facilities. These include calculating provider-to-population ratios, measuring the distance to the nearest provider, assessing the average distance to multiple providers, and using gravitational models to evaluate the influence of providers (Wang, Wang, and Liu Citation2021). However, these methods have limitations, such as a sole focus on distance, neglecting the size of supply and demand points, or failing to establish an effective search radius. The two-step floating catchment area (2SFCA) method, a more recent innovation, has evolved into a range of models (Chen and Jia Citation2019; Wang Citation2012). Unlike previous methods, the 2SFCA approach considers the scale of service provision and demand, as well as the travel time between service locations and those in need (Luo and Qi Citation2009). Variations of the 2SFCA method, including enhanced-2SFCA, kernel-2SFCA, Gaussian-2SFCA, and hierarchical-2SFCA, have been developed (Dai Citation2010; Dai and Wang Citation2011; Luo and Qi Citation2009; Luo and Whippo Citation2012; McGrail and Humphreys Citation2014; Tao et al. Citation2014). These adaptations, as Wang (Citation2012) notes, modify the classic 2SFCA model by introducing different search radii, distance-decay functions, and supply-demand scales. Building on the approach taken by (Langford, Higgs, and Fry Citation2016), we employ the enhanced-2SFCA method as formulated by Luo and Qi (Citation2009) incorporating multiple transport modes to construct a multi-modal enhanced-2SFCA method. This method is particularly apt for extensive study areas like Tokyo Metropolis, thanks to its simplicity in implementation, incorporation of multiple distance decay weights, and suitability for handling large data volumes.
2.2. Equity of accessibility
Equity is commonly understood as providing individuals or groups with equal rights and opportunities, as explained by Litman (Citation1999). It incorporates principles of fairness and justice (Carleton and Porter Citation2018). A vast array of research methods have been used to explore various aspects of equity, including overall population equity or equity among different demographic groups (Delbosc and Currie Citation2011), and at diverse spatial levels (Sharaby and Shiftan Citation2012). Equity measurement can be categorised into two main types: horizontal and vertical equities (Camporeale et al. Citation2019). In healthcare access, horizontal equity refers to the equitable access of individuals who use different transportation modes or live in various regions, while vertical equity focuses on the access disparities among populations with varying socioeconomic and demographic characteristics (Langford, Higgs, and Fry Citation2016; Wang et al., Citation2021). Specifically, vertical equity addresses the needs of groups disadvantaged due to mobility limitations, such as the elderly, children, disabled individuals, students, and low-income groups (Ramjerdi Citation1983). Current methodologies for analyzing accessibility (in)equity include using the Gini, Theil, Atkinson, Kolm, and KP indices (Gluschenko Citation2015; Yin et al. Citation2018), regression methods (Liu, Qin, and Xu Citation2019), or graphical representations like the Lorenz curve (Zhang, Zhang, and Zhou Citation2021). This study employs the KP index devised by Gluschenko (Citation2015) to assess accessibility (in)equity. The KP index is particularly advantageous in depicting healthcare accessibility distributions among social groups, as it allows for negative values in the input variable (healthcare accessibility index) and accommodates adjustments in parameters to reflect the non-linear impacts of uneven distribution in different geographic contexts (Wang et al. Citation2022).
2.3. Healthcare accessibility in the Japanese context
Despite considerable efforts by the Japanese government to manage the spread of COVID-19, the country's healthcare system has faced a series of escalating challenges throughout the various stages of the pandemic, as noted by Ghaznavi et al. (Citation2022) and Makiyama et al. (Citation2021). In the context of policy development and implementation post-COVID-19, it becomes crucial to assess healthcare accessibility in Japan with an emphasis on the diverse needs of different age groups and the extensive use of various transportation modes, a point highlighted by Tanimoto and Hanibuchi (Citation2021) considering the extensive public transit network in Japanese cities. Previous research on the disparities in healthcare access in Japan before the pandemic, such as studies by Watanabe and Hashimoto (Citation2012), Ito et al. (Citation2017), Shakya et al. (Citation2018), and Shinjo and Aramaki (Citation2012), tended to be limited in scope, often focusing on surveys, policy analyses or were conducted at broader geographical scales like towns, cities, or prefectures. Moreover, a recent study by Du and Zhao (Citation2022) investigated the accessibility of primary healthcare in Fukuoka City, yet it lacked an examination of how healthcare access varies with different transportation methods and across age groups. This indicates a gap in current research: a detailed, up-to-date analysis of healthcare accessibility in Japan post-COVID-19, which accounts for both transportation methods and demographic diversity, a gap this study intends to fill.
3. Data and method
3.1. Data
Demographic data, including population and age groups, was retrieved from the Statistics Bureau of Japan (Citation2022b) at the level of chome (census blocks) – the smallest statistical unit of the Japanese census. Medical data and transportation network data were obtained from the Ministry of Land, Infrastructure, Transport and Tourism (Citation2022). Medical data provides the X, Y coordinates of three types of medical institutes, including hospitals, regular clinics and dental clinics in Tokyo Metropolis. We included hospitals in our analysis because they have facilities (e.g. surgery rooms and beds) for inpatient services which are critical for COVID-19 treatments but excluded regular and dental clinics that only provide outpatient services to general patients. In addition, the number of hospitals (639 in the whole Tokyo Metropolis) is more manageable and computationally efficient in the network analysis. The attributes of hospitals (e.g. the number of physicians and beds) were used in the calculation of healthcare accessibility to reflect the medical capacity. The original data on transport networks has 19 classifications, including public transit (e.g. railway and bus routes) and roads/trails at different levels. They were reclassified into three major types: (1) public transit including railway and bus routes; (2) drivable roads including motorways, roads at the primary, secondary, and tertiary levels, trunk roads, and residential streets; and (3) walkable/cyclable roads including crossings, cycleway, bridleways, pedestrian paths, footway, tracks, and steps. The attributes of such transport data including connections, turns and driving directions were employed to set up the network database that enabled the calculation of travel routes more realistic and accurate.
3.2. Method
A large body of literature has discussed methods used for measuring healthcare accessibility. The classic two-step floating catchment area (2SFCA) method was widely used (Luo and Wang Citation2003), together its variations including Gaussian-2SFCA, kernel-2SFCA, and fuzzy-2SFCA (Dai Citation2010; Dai and Wang Citation2011; Luo and Qi Citation2009; Luo and Whippo Citation2012; McGrail and Humphreys Citation2014; Tao et al. Citation2014) which were largely based on the concept of enhanced 2SFCA by adopting different search radius and supply-demand scales (Wang Citation2012). In this study, we utilised the 2SFCA method enhanced by Luo and Qi (Citation2009) in our study given it is easy to implement with the consideration of multiple distance decay weights and it is more suitable for vast study areas (e.g. Tokyo Metropolis) and large data volume. Furthermore, our analysis utilised the number of people in each age group (e.g. age 0–18, 18–65 and +65), as defined in the Statistics Bureau of Japan as the assumed number of people by age group using each type of public transit, rather than the actual utilisation data by age group – which is the hypothesised measure adopted in this paper although such data is not available in Tokyo. The enhanced 2SFCA method is operated in two steps:
First, we selected six thresholds of travel distances by different transport modes – 5 and 10 km by public transit, 5 and 10 km by driving, and 1 and 2 km by walking – to define the catchment of a hospital based on the below rationale. The threshold of 5 and 10 km by public transit and driving is corresponding to the 20 and 40 min travel time which have been widely adopted in the current literature (Apparicio et al. Citation2017; Higgs Citation2004; Simoes and Almeida Citation2014) while 1 and 2 km by walking are considered as thresholds within which people find walking most tolerable (Mwaliko et al. Citation2014; Ursulica, Citation2016). Within each catchment, we computed three travel zones with equal distance breaks (Luo and Qi Citation2009) (e.g. 0–3.67 km, 3.67–6.67 km, 6.67–10 km, respectively, in the 10 km scenario). Then we searched all population locations (
) within the travel zone of
from a hospital
to compute the weighted supply-to-demand ratio (
) as follows:
(1)
(1) where
denotes the population of the census unit
that falls within the catchment
(
);
denotes the service capability of a hospital
(e.g. the number of beds and physicians);
denotes the travel distance between
and
;
is the
th travel distance zone within the catchment.
denotes the distance weight for the
th travel distance zone, reflecting the distance decay effect of the access to a hospital
.
Second, we searched all hospitals which were within a certain travel distance zone from each population location
, and summarised the supply-to-demand ratios,
at all population locations as follows:
(2)
(2) where
denotes the healthcare accessibility index of population at a population location
using a particular transport mode
;
denotes the supply-to-demand ratio at a hospital
within the area catered at a population location
(
);
denotes the distance between
and
. We applied the same distance weights derived from Step 1 to different travel distance zones in order to account for distance decay. Tokyo Metropolis has totally 5,610 origins (census blocks as population locations) and 639 destinations (hospitals), generating a network matrix that contains 3,584,790 origin-destination pairs. This matrix was used in the network analysis which was conducted using arcpy.na package in Python 3.10; the analytical results were mapped in ArcGIS Pro 2.8.
Next, we utilised the inequity index developed by Gluschenko (Citation2011), termed as the KP inequity index, to evaluate the inequity of healthcare accessibility in two dimensions – the horizontal inequity (i.e. the inequity across urban space) and the vertical inequity (i.e. the inequity across demographic groups) (Jayaraj and Subramanian Citation2006) in order to have a comprehensive understanding of the inequity of healthcare accessibility. The KP inequity index has the advantage to characterise distributions of input variables (e.g. healthcare accessibility in our case) in a large study area, and it can also reflect the non-linearity of marginal effect caused by the calculation of enhanced 2SFCA (Gluschenko Citation2011) by adjusting the parameter in Equation (3). The lower value of
corresponds to a higher inequity index value for a given unequal distribution. For the vector of healthcare accessibility measures, the KP inequity index can be expressed as (Gluschenko Citation2011):
(1)
(1) where
is the mean of healthcare accessibility measures in each census block (chome) and
denotes a parameter reflecting the non-linearity of marginal effect in the distribution of inequity measures.
The current literature has no consensus on the selection of , and typically generates results for a range of
values for comparison purposes (Estoque et al. Citation2020). Each value of
is consistent with a given constant elasticity
. We used the below approach of choosing the value of
that minimises the sum of squared differences between the individual elasticities (Tao et al. Citation2014). Through the computational experiment, we eventually employed three inequity aversions – the low level of
(0.25), the moderate level of
(0.50), and the high level of
(0.75) – representing various elasticities in the calculation of the KP inequity index:
(2)
(2) where
is the certain constant elasticity;
is the total number of census blocks in a certain region;
is the healthcare accessibility measure of a given census block
.
4. Results
4.1. Spatial patterns of multi-modal healthcare accessibility across demographic groups
The spatial patterns of multi-modal healthcare accessibility by public transit, driving and walking across three demographic groups (young, adult and elderly) are revealed in . By public transit ((1–6)), the pattern of healthcare accessibility in the 23-special-ward region is in a circular form – higher accessibility levels in the city centre and lower levels towards the regional fringe. In the Tama region, higher accessibility appears along the public transit network while the majority of West Tama has low accessibility levels (dark green in (1–6)). By driving (((7–12)), the pattern of healthcare accessibility in the 23-special-ward region is also in a circular form, similar to the pattern by public transit; while the accessibility level in West Tama by driving is apparently lower than that by public transit, possibly due to the mountainous landscape where it is more difficult to travel by driving compared to by public transit. By walking ((13–18)), there are as a wide range of small local clusters with high accessibility levels which are distributed dispersedly across the whole region. The 23-special-ward region presents more local clusters with higher accessibility levels compared to North and South Tama; while West Tama has the lowest accessibility level given the limited number of hospitals and the mountainous topography where it is difficult to travel by walking. Regardless of travel thresholds, the consistency of accessibility between by public transit and by driving is that the elderly group has relatively a higher accessibility level in the 23-special-ward region but a lower accessibility level in South and North Tama compared to the adult group (e.g. (3) versus (2); (9) versus (8)). Collectively, the overall accessibility ((19–21)) combines the accessibility measures of three transport modes (i.e. public transit with 10 km as the threshold, driving with 10 km as the threshold and walking with 2 km as the threshold), reflecting the holistic accessibility subject to the flexible options of transport modes. Compared to the young and adult group, the elderly group is observed to have a higher level of accessibility in the 23-special-ward region (mean = 397.5; statistical details provided in Supplementary Table S1) while a lower level of accessibility in North and South Tama (371.3 and 336.3, respectively). The areas with the lowest accessibility appear in the border of the 23-special-ward region and the Tama region (including Nerima Ward, Chofu City, and Komae City; statistical details provided in Supplementary Table S2), in the south of South Tama (e.g. Machida City and the south of Hachioji City) and the majority of West Tama. It is possible due to the low supply-to-demand ratio in these areas where the number of hospitals and their capacity of services ((4)) are limited but the population density are relatively high ((3)).
4.2. Horizontal and vertical inequity of healthcare accessibility
The KP inequity index () indicates that, regardless of transport modes, the elderly group experiences more inequity in healthcare access compared to adults in the majority of administrative areas (23 special wards, 26 cities and 3 towns) but less inequity in healthcare access compared to the young group with the age less than 18 – who may need accompaniment and supervision from their parents to go to hospitals. When it breaks down to each transport mode, in particular for the elderly group, the most significant inequity of healthcare accessibility by public transit appears in the Minato Ward, followed by the Shinjuku, Taito and Chiyoda Ward within the 23-special-ward region as well as in Ome City, followed by Akiruno City and Hachioji City in the Tama region. Such inequity in healthcare access by driving appears most obviously in the Meguro, Shinagawa and Shibuya Ward within the 23-special-ward region as well as Hachioji City, Ome City and Fuchu City in the Tama region. Such inequity in healthcare access by walking appears most obviously in the Suginami, Bunkyo and Taito Ward within the 23-special-ward region as well as Kunitachi City and Tachikawa City in the Tama region. For the adult group, regardless of transport modes, the discrepancies of inequity in healthcare access across wards and cities are relatively minor compared to the elderly group.
Figure 3. KP inequity index. (1) Overall KP inequity of healthcare for 52 administrative areas in Tokyo Metropolis (23 wards, 26 cities, and 3 towns) across three demographic groups (young, adult and elderly); (2) Separated KP inequity index of healthcare accessibility by public transit, driving and walking and across three demographic groups.
Note: Hinohara Village was not visualised here due to no access to healthcare facilities. Statistical details are provided in the Supplementary Table S3–5.

4.3. Areas that need to improve services for healthcare access
We further delineated the areas with the lowest level of accessibility (the bottom quintile) and the primary transport mode linking to the easiest healthcare accessibility in each census block (chome) ( (1)) for the elderly group (Supplementary Figure S1 and S2 for the young and adult group). For the elderly living in the areas with the lowest level of accessibility (red outlined in (1)) with the primary transport mode as public transit and walking, they may rely on railway and buses, or walk to healthcare facilities if their physical conditions allow. However, for the elderly living in the areas with the lowest level of accessibility with the primary transport mode as driving, they may encounter more problems in healthcare access if they do not have vehicles or cannot drive due to physical constraints. Along this rationale, the areas with the lowest level of accessibility concurrently with driving as the primary transport mode (red areas in (2)) would be the areas that are most needed to enhance services for healthcare access, for example, the provision of home pick-up or mobile clinics for home delivered medical services. South Tama ((3)) has the largest percentage of low-access areas (42.1%) and areas that need to enhance services for healthcare access (59.0%). Among the 53 administrative areas ((4)), the largest percentage of low-access areas appears in Hinohara Village (100%), followed by Machida City (98.1%), Komae City (95.1%), and Edogawa Ward (66.7%) and Ota Ward (47.5%) in the 23-special-ward region. Meanwhile, the largest percentage of the areas that need to enhance services for healthcare access (e.g. the provision of home pick-up or mobile clinics) appears in Koganei City, Mizuho Town and Hinode Town in the Tama region, together with the Toshima Ward and Kita Ward in the 23-special-ward region.
Figure 4. Areas with low accessibility and with the need to improve medical services for the elderly group. (1) Primary transport mode that provides the easiest healthcare accessibility at the census block (chome) level; areas with the lowest accessibility (the bottom quintile in ) are outlined in red; (2) Areas that need to enhance home pick-up services within the lowest accessibility region; (3) Percentages of low-access areas over the total region and percentages of low-access areas that need to improve services over the whole low-access region, classified by four broad administrative region (i.e. the 23-special-ward region, and South, North and West Tama); (4) Same measures as (3) but classified by administrative area (i.e. 23 wards, 26 cities, 3 towns and 1 village). Additional panel figures for the young and adult group are provided in Supplementary Figure S1 and S2.

5. Discussion
5.1. Key findings
Our study tackles an urgent and timely issue of increasingly inequitable healthcare access in the post-COVID era through a grained-level evaluation of the inequity of multi-modal healthcare accessibility by public transit, driving and walking in two dimensions – the horizontal inequity across urban space and the vertical inequity across three demographic groups (the young, adult and elderly) in Tokyo Metropolis, Japan. To our best knowledge, our study makes the first attempt to evaluate multi-modal healthcare access across different age groups in the Japanese COVID-19 context, on the basis of the latest census data to capture the current demographic landscape after the COVID-19 outbreak. Our key findings provide new insights into the current literature. More specifically, we find that the areas with low healthcare access appear on the border between the 23-special-ward region and the Tama region, in South Tama and the majority of West Tama where new hospitals and/or enhanced service capacities are urgently needed. The evaluation of horizontal inequity shows that the inequity in healthcare access exists in both the central area of the 23-special-ward region and the border between the 23-special-ward region and the Tama region; while the evaluation of vertical inequity shows that the elderly group, regardless of transport modes, experiences more inequity in healthcare access compared to adults in the majority of 53 administrative areas (23 wards, 26 cities, and 3 towns and 1 village). The GIS-based analytical framework for measuring multi-modal healthcare accessibility and examining its bi-dimensional inequity across age groups and different transport modes can be applied to other geographic contexts and in response to future public health crises.
5.2. Contribution to the literature
We have incorporated empirical and analytical enhancements in the fields of accessibility evaluation, public health and aging study. First, our network analysis took into account turns, driving directions, connections, and multiple transport modes, generating more realistic measures of network distances between hospitals and homes compared to the existing work (Du and Zhao Citation2022; Ito et al. Citation2017; Shakya et al. Citation2018; Shinjo and Aramaki Citation2012; Watanabe and Hashimoto Citation2012). In addition, the enhanced 2SFCA method considered the distance-decay effect on the supply-demand ratio, producing more accurate measures of healthcare accessibility. Second, the inequity of healthcare access was examined comprehensively in two dimensions – the horizontal inequity across space and the vertical inequity across demographic groups, which have been rarely unveiled in current literature. Third, our grained-level evaluation was conducted at the census block as the smallest census unit, covering the large urban agglomeration of Tokyo Metropolis – the most populous metropolitan region in the world (Tokyo Metropolitan Government Citation2022). Fourth, we delineated low-access locales most needed to enhance healthcare services for the elderly, which can be further integrated into broad aging research and enrich aging studies from a geographic perspective.
5.3. Policy implication
Far-reaching practices for policy implications can be drawn from our findings in the post-COVID era. Our timely findings provide spatially explicit evidence to policymakers and health authorities to have the latest understanding of healthcare access across different demographic groups. Low healthcare access areas are mainly located in the peri-urban space across the border of the 23-special-ward region and the Tama region, and the southern part of South Tama, where it is critically needed for augmented healthcare access and resources. In particular for elderly populations who have experienced significant inequity in healthcare access, those living in the peri-urban region without sufficient coverage of public transit are the most vulnerable group (Kotani Citation2020). Such aging groups may experience declines in physical and cognitive function, rendering them difficult to drive or walk to healthcare facilities (Muramatsu and Akiyama Citation2011); they may not afford to have vehicles or retain driving licenses (Ichikawa, Inada, and Nakahara Citation2020). In this regard, we delineated such low-access areas for elderly populations where medical services need to be enhanced, for example, the provision of home pick-up, home delivered treatments, and mobile clinics. Governments and health authorities at various levels need to make joint efforts to conquer the geographic barrier that exists in healthcare access across administrative borders. Last but importantly, our results suggest that both horizontal and vertical equity need to be considered in the designation of post-pandemic recovery initiatives, particularly in the continuous redistribution of medical resources/services through place-based health planning.
5.4. Limitations
The interpretation of our findings is subject to several limitations that can be further addressed in future studies. First, the enhanced 2SFCA method involved the distance-decay effect based on the equal travel distance breaks. Future studies could conduct surveys to investigate more realistic distance breaks that people would accept to walk or drive to healthcare facilities. Second, the thresholds of travel distances used in the enhanced 2SFCA method are somehow arbitrary, following the experience from the literature (Apparicio et al. Citation2017; Mwaliko et al. Citation2014; Simoes and Almeida Citation2014; Ursulica, Citation2016). Future studies could use travel time as a threshold and consider the uncertainty in travel time (e.g. the time spent for transfer or delay of heavy traffic) as well as the frequency of services in the network analysis to generate more accurate and realistic measures. Third, our analysis utilised the number of people in each age group as the assumed number of people using each type of public transit, rather than the actual utilisation data – which is the hypothesised measure adopted in this study although such data is not publicly available in Tokyo. We call for future studies to use the data with the capacity to capture the actual usage of transport modes for more accurate and realistic measures of multi-modal accessibility. Fourth, our analysis only included hospitals which have the capacity and capability to provide inpatient treatments (Ministry of Land, Infrastructure, Transport and Tourism Citation2022). Supplementary to hospitals as the tertiary or secondary medical facilities in the healthcare hierarchy, it is worthy to further examine the healthcare access to general clinics and dental clinics as the primary medical facilities given they are parts of the national healthcare system.
6. Conclusion
In summary, our study contributes a grain-level evaluation of the inequity of multi-modal healthcare access across demographic groups in the post-COVID era and finds out that the areas with low healthcare access appear in the border between the 23-special-ward region and the Tama region, in South Tama and the majority of West Tama where new hospitals and/or enhanced service capacities are urgently needed. It also delineates low-access locales that most need to enhance healthcare services for the elderly – the horizontal inequity shows that the inequity in healthcare access exists in both the central area of the 23-special-ward region and the border between the 23-special-ward region and the Tama region; while the evaluation of vertical inequity shows that the elderly group, regardless of transport modes, experiences more inequity in healthcare access compared to adults in the majority of 53 administrative areas. More specifically, it offers a practical framework to examine healthcare access corresponding to the shifting demographic landscape alongside the economic downturn and regional migration induced by COVID-19 – which can be readily be applied to other regions and to cope with future public health crises. Delineating locales where elderly populations are most needed to the enhancement of medical services and healthcare equity provides important information for the smart deployment of finite medical resources in a super-aging society. These new insights provide evidence to Japan to better prepare for the aging challenges, guide public health policy, and direct healthcare services in the COVID era and beyond.
Authors’ contributions
Siqin Wang: Conceptualization; Methodology; Software; Validation; Formal analysis; Investigation; Writing – Original Draft; Visualization; Project administration.
Yukio Sadahiro: Investigation; Validation; Data Curation; Writing – Review & Editing; Funding acquisition; Supervision.
Consent for publication
All authors consent for publication.
Ethics approval and consent to participate
This study did not receive nor require ethics approval, as it does not involve human & animal participants.
Supplemental Material
Download MS Word (927 KB)Acknowledgements
The measures of healthcare accessibility are publicly accessible via the project public repository https://figshare.com/articles/dataset/Healthcare_access_Tokyp_Japan/20222007
Disclosure statement
No potential conflict of interest was reported by the author(s).
Data availability statement
Health accessibility data generated by this study are publicly accessible via the project public repository: https://figshare.com/articles/dataset/Healthcare_access_Tokyp_Japan/20222007.
Additional information
Funding
References
- Apparicio, P., J. Gelb, A. S. Dubé, S. Kingham, L. Gauvin, and É. Robitaille. 2017. “The Approaches to Measuring the Potential Spatial Access to Urban Health Services Revisited: Distance Types and Aggregation-error Issues.” International Journal of Health Geographics 16 (1): 1–24. https://doi.org/10.1186/s12942-017-0105-9.
- Black, M., S. Ebener, P. N. Aguilar, M. Vidaurre, and Z. El Morjani. 2004. Using GIS to Measure Physical Accessibility to Health Care, 3–4. Geneva, Switzerland: World Health Organization.
- Camporeale, R., L. Caggiani, and M. Ottomanelli. 2019. “Modeling horizontal and vertical equity in the public transport design problem: A case study.” Transportation Research Part A: Policy and Practice 125: 184–206.
- Carleton, P. R., and J. D. Porter. 2018. “A comparative analysis of the challenges in measuring transit equity: definitions, interpretations, and limitations.” Journal of transport geography 72: 64–75.
- Chen, X., and P. Jia. 2019. “A Comparative Analysis of Accessibility Measures by the Two-step Floating Catchment Area (2SFCA) Method.” International Journal of Geographical Information Science 33 (9): 1739–1758. https://doi.org/10.1080/13658816.2019.1591415.
- Chiriboga, D., J. Garay, P. Buss, R. S. Madrigal, and L. C. Rispel. 2020. “Health Inequity During the COVID-19 Pandemic: A cry for Ethical Global Leadership.” The Lancet 395 (10238): 1690–1691. https://doi.org/10.1016/S0140-6736(20)31145-4.
- Dai, D. 2010. “Black Residential Segregation, Disparities in Spatial Access to Health Care Facilities, and Late-stage Breast Cancer Diagnosis in Metropolitan Detroit.” Health & Place 16 (5): 1038–1052. https://doi.org/10.1016/j.healthplace.2010.06.012.
- Dai, D., and F. Wang. 2011. “Geographic Disparities in Accessibility to Food Stores in Southwest Mississippi.” Environment and Planning B: Planning and Design 38:659–677. https://doi.org/10.1068/b36149.
- Dandekar, A., and R. Ghai. 2020. “Migration and Reverse Migration in the age of COVID-19.” Economic and Political Weekly 55 (19): 28–31.
- Daoust, J. F. 2020. “Elderly People and Responses to COVID-19 in 27 Countries.” PLoS One 15 (7): e0235590. https://doi.org/10.1371/journal.pone.0235590.
- Delbosc, A., and G. Currie. 2011. “The spatial context of transport disadvantage, social exclusion and well-being.” Journal of Transport Geography 19 (6): 1130–1137.
- Du, M., and S. Zhao. 2022. “An Equity Evaluation on Accessibility of Primary Healthcare Facilities by Using V2SFCA Method: Taking Fukuoka City, Japan, as a Case Study.” Land 11 (5): 640. https://doi.org/10.3390/land11050640.
- Estoque, R. C., M. Ooba, X. T. Seposo, T. Togawa, Y. Hijioka, K. Takahashi, and S. Nakamura. 2020. “Heat Health Risk Assessment in Philippine Cities Using Remotely Sensed Data and Social-ecological Indicators.” Nature Communications 11 (1): 1–12. https://doi.org/10.1038/s41467-020-15218-8.
- Ghaznavi, C., A. Eguchi, Y. Tanoue, D. Yoneoka, T. Kawashima, M. Suzuki, M. Hashizume, and S. Nomura. 2022. “Pre-and Post-COVID-19 All-cause Mortality of Japanese Citizens versus Foreign Residents Living in Japan, 2015–2021.” SSM-Population Health 18:101114. https://doi.org/10.1016/j.ssmph.2022.101114.
- Gluschenko, K. P. 2011. “Studies on Income Inequality among Russian Regions.” Regional Research of Russia 1 (4): 319–330. https://doi.org/10.1134/S2079970511040034.
- Gluschenko, K. P. 2015. “On Estimation of Inter-Regional Inequality.” Spatial Economics Prostranstvennaya Ekonomika 4 (44): 39–58. https://doi.org/10.14530/se.2015.4.039-058.
- Higgs, G. 2004. “A Literature Review of the use of GIS-based Measures of Access to Health Care Services.” Health Services and Outcomes Research Methodology 5 (2): 119–139. https://doi.org/10.1007/s10742-005-4304-7.
- Ichikawa, M., H. Inada, and S. Nakahara. 2020. “Increased Traffic Injuries among Older Unprotected Road Users Following the Introduction of an Age-based Cognitive Test to the Driver’s License Renewal Procedure in Japan.” Accident Analysis & Prevention 136:105440. https://doi.org/10.1016/j.aap.2020.105440.
- Ito, J., S. Edirippulige, T. Aono, and N. R. Armfield. 2017. “The Use of Telemedicine for Delivering Healthcare in Japan: Systematic Review of Literature Published in Japanese and English Languages.” Journal of Telemedicine and Telecare 23 (10): 828–834. https://doi.org/10.1177/1357633X17732801.
- Jayaraj, D., and S. Subramanian. 2006. “Horizontal and Vertical Inequality: Some Interconnections and Indicators.” Social Indicators Research 75 (1): 123–139. https://doi.org/10.1007/s11205-004-4649-2.
- Kim, Y., Y. J. Byon, and H. Yeo. 2018. “Enhancing Healthcare Accessibility Measurements Using GIS: A Case Study in Seoul, Korea.” PLoS One 13 (2): e0193013.
- Kotani, K. 2020. “Transportation Issues in Rural Healthcare.” Journal of Preventive Medicine and Public Health 53 (2): 149–150. https://doi.org/10.3961/jpmph.20.038.
- Krouse, H. J. 2020. “COVID-19 and the Widening Gap in Health Inequity.” Otolaryngology–Head and Neck Surgery 163 (1): 65–66. https://doi.org/10.1177/0194599820926463.
- Langford, M., G. Higgs, and R. Fry. 2016. “Multi-modal Two-step Floating Catchment Area Analysis of Primary Health Care Accessibility.” Health & Place 38:70–81. https://doi.org/10.1016/j.healthplace.2015.11.007.
- Litman, T. 1999. Traffic calming: benefits, costs and equity impacts, p 7. Victoria, BC, Canada: Victoria Transport Policy Institute.
- Liu, S., Y. Qin, and Y. Xu. 2019. “Inequality and Influencing Factors of Spatial Accessibility of Medical Facilities in Rural Areas of China: A Case Study of Henan Province.” International Journal of Environmental Research and Public Health 16 (10): 1833. https://doi.org/10.3390/ijerph16101833.
- Luo, W., and Y. Qi. 2009. “An Enhanced Two-step Floating Catchment Area (E2SFCA) Method for Measuring Spatial Accessibility to Primary Care Physicians.” Health & Place 15 (4): 1100–1107. https://doi.org/10.1016/j.healthplace.2009.06.002.
- Luo, W., and F. Wang. 2003. “Measures of Spatial Accessibility to Health Care in a GIS Environment: Synthesis and a Case Study in the Chicago Region.” Environment and Planning B: Planning and Design 30 (6): 865–884. https://doi.org/10.1068/b29120.
- Luo, W., and T. Whippo. 2012. “Variable Catchment Sizes for the Two-step Floating Catchment Area (2SFCA) Method.” Health & Place 18 (4): 789–795. https://doi.org/10.1016/j.healthplace.2012.04.002.
- Makiyama, K., T. Kawashima, S. Nomura, A. Eguchi, D. Yoneoka, Y. Tanoue, Y. Kawamura, et al. 2021. “Trends in Healthcare Access in Japan During the First Wave of the COVID-19 Pandemic, Up to June 2020.” International Journal of Environmental Research and Public Health 18 (6): 3271. https://doi.org/10.3390/ijerph18063271.
- McGrail, M. R., and J. S. Humphreys. 2014. “Measuring Spatial Accessibility to Primary Health Care Services: Utilising Dynamic Catchment Sizes.” Applied Geography 54:182–188. https://doi.org/10.1016/j.apgeog.2014.08.005.
- Ministry of Land, Infrastructure, Transport and Tourism. 2022. National Land Numerical Information Download Service. https://nlftp.mlit.go.jp/ksj/index.html.
- Muramatsu, N., and H. Akiyama. 2011. “Japan: Super-aging Society Preparing for the Future.” The Gerontologist 51 (4): 425–432. https://doi.org/10.1093/geront/gnr067.
- Mwaliko, E., R. Downing, W. O’Meara, D. Chelagat, A. Obala, T. Downing, C. Simiyu, et al. 2014. “‘Not too Far to Walk’: The Influence of Distance on Place of Delivery in a Western Kenya Health Demographic Surveillance System.” BMC Health Services Research 14 (1): 1–9. https://doi.org/10.1186/1472-6963-14-212.
- Politis, I., G. Georgiadis, E. Papadopoulos, I. Fyrogenis, A. Nikolaidou, Kopsacheilis A., and Verani E . 2021. “COVID-19 lockdown measures and travel behavior: The case of Thessaloniki.” Transportation Research Interdisciplinary Perspectives 10: 100345.
- Ramjerdi, F. 1983. “Equity measures and their performance in transportation.” Transportation Research Record 1983 (1): 67–74.
- Shakya, P., M. Tanaka, A. Shibanuma, and M. Jimba. 2018. “Nepalese Migrants in Japan: What is Holding Them Back in Getting Access to Healthcare?” PLoS One 13 (9): e0203645. https://doi.org/10.1371/journal.pone.0203645.
- Sharaby, N., and Y. Shiftan. 2012. “The impact of fare integration on travel behavior and transit ridership.” Transport Policy 21: 63–70.
- Shinjo, D., and T. Aramaki. 2012. “Geographic Distribution of Healthcare Resources, Healthcare Service Provision, and Patient Flow in Japan: A Cross Sectional Study.” Social Science & Medicine 75 (11): 1954–1963. https://doi.org/10.1016/j.socscimed.2012.07.032.
- Simoes, P. P., and R. M. V. Almeida. 2014. “Maternal Mortality and Accessibility to Health Services by Means of Transit-network Estimated Traveled Distances.” Maternal and Child Health Journal 18 (6): 1506–1511. https://doi.org/10.1007/s10995-013-1391-x.
- Statistics Bureau of Japan. 2022a. Preliminary Counts of Population of Japan. https://www.stat.go.jp/english.
- Statistics Bureau of Japan. 2022b. Census. https://www.e-stat.go.jp/stat-search/files?page=1&layout=datalist&toukei=00200521&tstat=000001136464&cycle=0&tclass1=000001136472&tclass2=000001159886&tclass3val=0.
- Tanimoto, R., and T. Hanibuchi. 2021. “Associations between the Sense of Accessibility, Accessibility to Specific Destinations, and Personal Factors: A Cross-sectional Study in Sendai, Japan.” Transportation Research Interdisciplinary Perspectives 12:100491. https://doi.org/10.1016/j.trip.2021.100491.
- Tao, Z., Y. Cheng, T. Dai, and M. W. Rosenberg. 2014. “Spatial Optimization of Residential Care Facility Locations in Beijing, China: Maximum Equity in Accessibility.” International Journal of Health Geographics 13 (1): 1–11. https://doi.org/10.1186/1476-072X-13-1.
- Tokyo Metropolitan Government. 2022. About Our City. https://www.metro.tokyo.lg.jp/english/.
- United Nations. 2020. Sustainable Development Goals. https://www.un.org/sustainabledevelopment/health/.
- Ursulica, T. E. 2016. “The Relationship between Health Care Needs and Accessibility to Health Care Services in Botosani County-Romania.” Procedia Environmental Sciences 32:300–310. https://doi.org/10.1016/j.proenv.2016.03.035.
- Vulevic, A. 2016. “Accessibility Concepts and Indicators in Transportation Strategic Planning Issues: Theoretical Framework and Literature Review.” Logistics, Supply Chain, Sustainability and Global Challenges 7 (1): 58–67.
- Wang, F. 2012. “Measurement, Optimization, and Impact of Health Care Accessibility: A Methodological Review.” Annals of the Association of American Geographers 102 (5): 1104–1112. https://doi.org/10.1080/00045608.2012.657146.
- Wang, S., Y. Liu, and J. Corcoran. 2021. “Equity of public transport costs before and after a fare policy reform: An empirical evaluation using smartcard data.” Transportation Research Part A: Policy and Practice 144: 104–118.
- Wang, S., M. Wang, and Y. Liu. 2021. “Access to Urban Parks: Comparing Spatial Accessibility Measures Using Three GIS-based Approaches.” Computers, Environment and Urban Systems 90:101713. https://doi.org/10.1016/j.compenvurbsys.2021.101713.
- Wang, S., M. Zhang, X. Huang, T. Hu, Q. C. Sun, J. Corcoran, and Y. Liu. 2022. “Urban–Rural Disparity of Social Vulnerability to Natural Hazards in Australia.” Scientific Reports 12 (1): 13665. https://doi.org/10.1038/s41598-022-17878-6.
- Watanabe, R., and H. Hashimoto. 2012. “Horizontal Inequity in Healthcare Access under the Universal Coverage in Japan; 1986–2007.” Social Science & Medicine 75 (8): 1372–1378. https://doi.org/10.1016/j.socscimed.2012.06.006.
- The World Bank. 2022. Population Ages 65 and Above (% of Total Population) – Japan. https://data.worldbank.org/indicator/SP.POP.65UP.TO.ZS?locations=JP.
- World Health Organization. 2020. Extent, Scope and Impacts of COVID-19 on Health Inequities: The Evidence. https://www.who.int/docs/default-source/documents/social-determinants-of-health/overview—covid-19-impacts-(nicole-valentine).pdf.
- Xu, Y., L. E. Olmos, S. Abbar, and M. C. González. 2020. “Deconstructing Laws of Accessibility and Facility Distribution in Cities.” Science Advances 6 (37): eabb4112. https://doi.org/10.1126/sciadv.abb4112.
- Yang, D. H., R. Goerge, and R. Mullner. 2006. “Comparing GIS-based Methods of Measuring Spatial Accessibility to Health Services.” Journal of Medical Systems 30 (1): 23–32. https://doi.org/10.1007/s10916-006-7400-5.
- Yin, C., Q. He, Y. Liu, W. Chen, and Y. Gao. 2018. “Inequality of Public Health and its Role in Spatial Accessibility to Medical Facilities in China.” Applied Geography 92:50–62. https://doi.org/10.1016/j.apgeog.2018.01.011.
- Zhang, D., G. Zhang, and C. Zhou. 2021. “Differences in Accessibility of Public Health Facilities in Hierarchical Municipalities and the Spatial Pattern Characteristics of their Services in Doumen District, China.” Land 10 (11): 1249. https://doi.org/10.3390/land10111249.