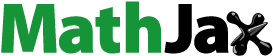
ABSTRACT
Information on Arctic sea-ice motion in summer is critical for operational sea-ice monitoring and prediction and commercial navigation. Affected by the melting of the sea-ice surface, the accuracy and spatial coverage of the sea-ice velocity (SIV) retrieved by brightness temperature (TB) during the melting period are always lower than in winter. Our research indicates that the lack of ice–water discrimination in summer for the Advanced Microwave Scanning Radiometer 2 (AMSR2) 36 GHz TB is the main reason for the poor quality of its SIV results. We propose a new SIV retrieval scheme based on the polarization difference (P) of the 36 GHz TB. The 36-P SIV has a higher spatial coverage than that derived from 18-H, 36-H and 36-V SIVs and a higher maximum cross-correlation coefficient than 36 GH TB-derived SIVs. Compared with buoy and Synthetic Aperture Radar SIVs, the 36-P SIV’s error is close to that of 18-H SIV and significantly lower than that of 36 TB SIV. Therefore, our results indicate that 36-P is more competitive than the 36 GHz TB in SIV retrieval in summer. Further analysis shows that our proposed scheme is compatible with the two current mainstream SIV tracking algorithms, demonstrating its great potential for future summer SIV retrieval.
1. Introduction
Sea-ice motion is an important factor shaping the spatial distribution of sea-ice concentration (SIC) and thickness and ice age (Shokr and Ye Citation2023), and also plays a key role in the total balance of Arctic sea ice (Kimura et al. Citation2013). As an important parameter to describe the changes in Arctic sea ice, the acceleration of sea-ice motion is also an important indicator of the current rapid change in Arctic sea ice (Rampal, Weiss, and Marsan Citation2009; Tschudi, Meier, and Stewart Citation2020; Zhang et al. Citation2022). Reliable sea-ice motion data are therefore critical for climate research and navigation safety (Min et al. Citation2022).
Satellite remote sensing is the most widely used method to obtain the characteristics of sea-ice over the whole Arctic Ocean. In addition to radiometer, and radar, Global Navigation Satellite System-Reflectometry also has great potential in detecting sea ice in polar regions (Yan and Huang Citation2016; Citation2019). Due to the high spatial resolution of Synthetic Aperture Radar (SAR) imagery, and their smaller susceptibility to the effects of clouds and polar nights, sea-ice velocity (SIV) retrievals based on SAR imagery have become a research hotspot in recent decades (Kwok et al. Citation2008; Komarov and Barber Citation2014; Howell et al. Citation2018; Moore et al. Citation2021). However, current SAR ice velocity products have limited coverage in time and space. Long-term climate research still needs a greater accumulation of SAR data.
Passive microwave (PM) imagery has become the main source for studying SIV because of its high temporal and spatial coverage (Ezraty, Girard-Ardhuin, and Piollé Citation2007; Lavergne et al. Citation2010; Tschudi, Meier, and Stewart Citation2020). Three commonly used operational SIV datasets are all largely dependent on PM-derived ice velocity (Ezraty, Girard-Ardhuin, and Piollé Citation2007; Lavergne et al. Citation2010; Tschudi, Meier, and Stewart Citation2020). Although these SIV products have been widely used in climate research (Spreen et al. Citation2020; Krumpen et al. Citation2021), a common outstanding problem with them is that the quality of summer SIV is lower than that during the freezing period (Hwang Citation2013; Sumata et al. Citation2014; Gui et al. Citation2020;Wang et al. Citation2022). This quality varies significantly in different seasons and is also commonly found in other sea-ice parameters (SIC and sea-ice thickness), which has attracted extensive research (Min et al. Citation2023). This difference is usually related to changes in surface properties caused by melting snow on the surface of summer sea ice. Due to the homogeneity of the sea-ice surface resulting from the melting of sea ice in summer, the success rate of summer SIV retrievals based on 36 GHz or 89 GHz brightness temperature (TB), which is the convention for winter SIV retrievals, is significantly reduced, and the uncertainty increases considerably (Ezraty, Girard-Ardhuin, and Piollé Citation2007; Kwok Citation2008). Kwok (Citation2008) pointed out that using the low-frequency band (18 GHz) for summer SIV retrieval can greatly improve its success rate. In order to achieve temporally continuous SIV data, existing operations usually adopt one of two methods to obtain the summer SIV – one is based on the limited SIV obtained from the 36 GHz TB and merged with other SIV products [as adopted by the National Snow and Ice Data Center (NSIDC)]; and the other involves acquiring SIV retrievals based on 18 GHz TB with a lower nominal spatial resolution [as adopted by the Satellite Application Facility on Ocean and Sea Ice (OSI SAF)]. These two methods inevitably sacrifice data quality to obtain complete data coverage. Hence, such summer SIV data is inconsistent with those obtained in other seasons, thereby impacting the reliability of studies based on SIV (e.g. research on sea-ice deformation).
Considering the shortcomings of current PM summer SIV data, this paper proposes a new scheme for retrieving Arctic summer SIV based on the polarization difference of 36 GHz TB to improve the spatial coverage of SIV derived from 36 GHz TB in summer, while retaining the data resolution of the 36 GHz TB. We introduce the data used for SIV retrieval and comparison in section 2. Then, we present the performance of the improved SIV from different aspects, as well as validation results, in section 3. In section 4, we discuss the strengths and weaknesses of our research. Finally, in section 5, we summarize our findings and present our conclusions.
2. Data and methods
2.1. Data
TB and SIC are essential for SIV retrieval. The gridded AMSR2 TB at 18 and 36 GHz used for SIV retrieval from are processed and distributed by the University of Bremen with a spatial resolution of 12.5 km. The daily AMSR2 SIC data from OSI SAF with a 10.0 km resolution (Baordo, Vargas, and Howe Citation2023) are used to filter the open water area because of their best overall superior performance in these areas among different SIC data sets (Ivanova et al. Citation2015).
In addition, a processed TB data set for pure sea ice (SI, SIC = 100%) and open water (OW, SIC = 0%) are employed to improve our tracking method. This round-robin data package (RRDP) that collects the satellite observed TB is widely used in SIC algorithms. The OW data in the RRDP are verified by the ice chart data from the Danish Meteorological Institute and the US National Ice Center. The TB data with 100% SIC are constrained by the convergence of ice cover observed by ENVISAT ASAR. Note that the data with cloudiness higher than 5% are excluded.
Four different sources of SIV data are used to compare with our SIV results. First, we use the in-situ SIV observations derived from the International Arctic Buoy Program (IABP) data as a reference. The positions of buoys are converted into daily SIVs before SIV comparison. Second, sea-ice drift data from the Sentinel-1 SAR with a 10 km resolution from the Copernicus Marine Environment Monitoring Service are employed to validate the PM SIV in summer. Besides, daily SIV products distributed by Institut Français de Recherche pour l'Exploitation de la Mer (Ifremer) derived from 89 GHz TB (Ezraty, Girard-Ardhuin, and Piollé Citation2007); and NSIDC derived from 36 GHz TB (Tschudi, Meier, and Stewart Citation2020) are used for comparison with the improved SIV in this study.
2.2. Improved retrieval scheme for summer SIV
The main reason for the low quality of current SIV data in summer is that the source data they use have poor spatial coverage in summer (Wang et al. Citation2022). To reduce the impact of total sea-ice coverage in different seasons on the effective SIV coverage, here we define the spatial coverage as the area with valid SIV divided by the area with SIC > 15% to measure the quality of SIV data. Comparing the spatial coverage of NSIDC-36 GHz and Ifremer-89 GHz, we find that both spatial coverages of SIV are significantly lower during the melting period than the freezing period ((a)). Kwok (Citation2008) speculated that the low sensitivity to atmospheric moisture of the 18 GHz TB results in the high spatial coverage of SIV in summer derived from them. Still, this has not been discussed in depth. However, we noticed that if the atmospheric moisture affects the SIV retrieval based on the 36 GHz TB, the missing data ((b)) should be mainly located in the marginal ice zone that is closer to the open water, rather than the area covered by multi-year ice with high SIC. Hence, we suspect that there are other reasons affecting the quality of the SIV retrieval in summer.
Figure 1. (a) Daily spatial coverage of SIV derived from different data sources from May to September in 2019. (b) Spatial distribution of missing SIV vector counts of NSIDC-SSM from May to September in 2019, in which the red line represents the 50% SIC contour.

Prominent features often lead to better results when tracking SIV. Unlike winter, which mainly tracks the emissivity characteristics of the sea-ice surface, as the sea ice melts in summer, the water in the sea-ice surface (or the ice–water boundary) becomes an important tracer for pattern matching. Theoretically, the greater the degree of discrimination of the surface feature in the tracking image, the higher the correlation of pattern matching, and thus the higher the success rate of SIV retrievals. However, the actual result is exactly the opposite. Therefore, we speculate that pattern matching (or ice–water discrimination) based on TB in summer may not be as effective as in the sea-ice freezing period.
Here, we first study the TB in different bands based on the RRDP, which is less affected by the atmosphere. In general, as the frequency increases, OW and SI become increasingly indistinguishable in the TB image. At 36 GHz, although the TB of OW in the horizontal channel is lower than that of SI generally, there are still many OW samples confused with SI ((b)). This confusion between SI and OW is more serious at 89 GHz ((c)). It should be noted that this confusion between SI and OW is based on strictly selected RRDP data and may be more common in ice–water mixed grids. However, at 18 GHz, the clusters of SI and OW are significantly separated ((a)). This also explains why the 18 GHz TB can be used to efficiently retrieve the SIV in summer, while the retrievals based on 36 and 89 GHz TB fail.
Figure 2. (a–c) Scatterplots of vertical and horizontal TB at (a) 18 GHz, (b) 36 GHz, and (c) 89 GHz from RRDP. (d–f) Scatterplots of vertical TB and polarization difference at (d) 18 GHz, (e) 36 GHz, and (f) 89 GHz from RRDP.

If we can find an input field that can better distinguish OW and SI based on the TB of 36 or 89 GHz, we can obtain a reliable SIV field during the melting season. The polarization difference of TB (EquationEquation 1(1)
(1) ), which is widely used in SIC retrievals (Cavalieri, Gloersen, and Campbell Citation1984; Spreen, Kaleschke, and Heygster Citation2008), presents great potential for summer SIV retrieval since there is a significant difference in the polarization difference for SI and OW. Further analysis indicates that by taking the polarization difference and vertical TB as coordinates, the clusters of SI and OW become more dispersed in both the 36 and 89 GHz channel ((e,f)). This has little effect on the results of 18 GHz because SI and OW can be distinguished well based on 18 GHz TB ((a)). In this paper, we focus on the feasibility of summer SIV retrieval based on the polarization difference of 36 GHz TB:
(1)
(1) where
and
denote the TB for vertical and horizontal polarization, respectively.
2.3. Sea-ice motion tracking method
Maximum Cross-correlation (MCC) methods are mostly used as pattern matching methods to track sea-ice motion based on PM imagery. An improved method proposed by Lavergne et al. (Citation2010), called the Continuous Maximum Cross-correlation (CMCC) method, is thought to efficiently reduce the quantization effect. In this paper, we employ the CMCC method to track SIV based on PM imagery (Lavergne et al. Citation2010). The flowchart of SIV retrieval is presented in . First, the input gridded fields at the start and end days are both enhanced by a Laplacian filter introduced by Ezraty, Girard-Ardhuin, and Piollé (Citation2007). Then, following Ezraty, Girard-Ardhuin, and Piollé (Citation2007), we perform the autocorrelation test on the starting image and discard templates with maximum correlation higher than 0.6. The templates with SIC lower than 30% are also discarded before retrieval. It is worth noting that the size of the template window is set to 11 × 11 pixels; the time interval is set to one day. Then, according to the CMCC method, we identify the three most likely locations for the end of SIV by comparing the correlation coefficients between the template and the target window. Hereafter, we acquire the location with the highest correlation coefficients based on these candidates using the Nelder–Mead algorithm (Nelder and Mead Citation1965). As a key parameter in post-processing schemes, the minimum correlation threshold (the low band of the maximum cross-correlation coefficient) will obviously affect the quality of the SIV results. In previous studies, the minimum correlation threshold is usually between 0.3 and 0.6, e.g. 0.3 (Lavergne et al. Citation2010), 0.4 (Tschudi, Meier, and Stewart Citation2020) or 0.6 (Ezraty, Girard-Ardhuin, and Piollé Citation2007). Here, considering that the success rate of SIV retrieval during the melting period is often lower than that during the freezing period, the minimum correlation threshold we use is 0.5, and the SIV results with a maximum cross-correlation coefficient lower than 0.5 are discarded. To more directly reflect the quality of the SIV retrieved from different initial-value fields, we do not perform a consistency check with neighboring vectors or winds.
3. Results and analysis
3.1. Spatial coverage of SIV
We use the CMCC tracking method described in Section 2.3 to retrieve the SIV of the entire sea-ice melting season from 1 May to 30 September 2019 based on the 18-H, 36-H and 36-V TB, and the 36-P, respectively. Insufficient spatial coverage is the main disadvantage of SIV retrieval at 36 GHz TB. Therefore, we first perform an intercomparison of the spatial coverage between the four different sets of SIV results. Our results show that the mean spatial coverage in summer (May–September) of the four kinds of SIVs is 81.12%, 74.89%, 68.01% and 81.93%, respectively. Among them, the 36-P SIV has the highest spatial coverage and the 36-V SIV has the lowest spatial coverage as well as the strongest intraseasonal variation ((a)). During June–August, when the lack of SIV is most severe, the spatial coverage of 36-P and 18-H SIV are significantly higher than that of the other two results. We infer that the considerably lower spatial coverage of the 36-V SIV relative to the 36-H SIV might be related to the lower ice–water discrimination of 36-V (shown in (b)). In addition, there is a correlation between the spatial coverage of the four SIVs on a daily basis, and the correlation coefficients between 36-H, 36-V, and 36-P and 18-H SIV are 0.71, 0.57 and 0.78, respectively. The minimums of the daily spatial coverage of the 36-V and 36-H SIV both occurr between the end of July and August, which also implies that there is a close relationship between the low quality of SIV based on the 36 GHz TB and the poor discrimination of ice and water with the 36 GHz TB. In summary, compared with directly using 36 GHz TB for SIV retrieval, using the 36-P can effectively improve the spatial coverage from mid-May to mid-September.
Figure 4. (a) Daily spatial coverage of four sets of SIV results in summer 2019. (b) Proportions of SIV with different quality during retrieval process. The green and red bars represent the SIV that failed the auto-correlation test and the convergence detection of the correlation calculation, respectively. The yellow part represents the SIV with a maximum cross-correlation coefficient lower than 0.5, and the purple part represents the remaining valid SIV results.

We further analyze the influence of the three processes of autocorrelation detection, MCC convergence detection, and MCC lower bound filtering, mentioned in Section 3.2, on the spatial coverage of SIV. 36-P SIV has the highest percentage of valid SIV, close to that of 18-H SIV but higher than that of the 36-H and 36-V SIVs ((b)). Compared with 18-H SIV (91.1%), the advantage of 36-P SIV is that it has more templates that can pass the autocorrelation detection (93.2%), which may be related to its over-smoothness of the ice surface features due to the low real spatial resolution of 18 GHz TB (only half that of the other data). Compared with 36-TB SIV, the advantage of 36-P SIV is that it has a higher maximum cross-correlation coefficient and more than 81% of the SIV data are valid vectors (purple bar in (b)). Autocorrelation is a parameter that measures the significance of ice surface features, and the maximum cross-correlation coefficient also has a great relationship with the significance of sea-ice surface features. Therefore, our results indicate that the higher spatial coverage of 36-P SIV with respect to the other three SIVs is caused by its more significant sea-ice surface characteristics.
Next, we further investigate the spatiotemporal variation of the spatial coverage of the four sets of SIV results on a monthly basis. Overall, the SIVs of 18-H and 36-P have high and similar spatial coverage in summer (). In May and September, low spatial coverage mainly occurs in the marginal ice zones, such as Baffin Bay, the Kara Sea, and Fram Strait. For the SIV of 36-H and 36-V, the decrease in spatial coverage from June to August mainly occurs in the central Arctic and the northern Canadian Arctic Archipelago. However, in the same area, the spatial coverage of 18-H- and 36-P-derived SIV only decreases slightly. The low spatial-coverage values of 36-H and 36-V SIV exhibit a latitudinally increasing feature as the sea ice melts. Considering that the spatial coverage of 36-H and 36-V SIV decreases most rapidly in June, we further analyze the variations in SIC () and find that the standard deviation (SD) of SIC in the Arctic is significantly higher in June than in other summer months. This suggests that this spatial coverage reduction is linked to the surface melting of sea ice.
3.2. Comparison of the maximum cross-correlation coefficients of different SIV fields
In addition to the spatial coverage of the valid SIV, the maximum cross-correlation coefficient obtained by SIV retrievals can also measure the quality of the results. Generally, the higher the maximum cross-correlation coefficient, the higher the reliability of the SIV (Shi et al. Citation2022). In this section, we compare the differences between the four sets of SIVs in terms of their maximum cross-correlation coefficients (). The results show that the overall maximum cross-correlation coefficient of the 36-P SIV is consistent with that of other channels. The spatial correlations of 18-H, 36-V and 36-H with 36-P are 0.61, 0.34 and 0.65, respectively. The maximum cross-correlation coefficient of 36-P SIV is higher than that of 36-V and 36-H SIV ((a)), but lower than the that of 18-H SIV, and this difference is more significant when the maximum cross-correlation coefficient is lower ((b)).
Figure 6. 2-D density plots of the maximum cross-correlation coefficient of SIV (a) 36-V vs 36-P; (b) 18-H vs 36-P, along with the the (c) mean and (d) SD of the daily maximum cross-correlation coefficient in retrievals by 18-H, 36-H, 36-V, and 36-P TBs in summer 2019.

Further research ((c,d)) shows that the 18-H SIV has the highest maximum cross-correlation coefficient (0.87) and the lowest SD of the maximum cross-correlation coefficient (0.09), while the 36-V SIV has the lowest maximum cross-correlation coefficient (0.74) and the highest SD of the maximum cross-correlation coefficient (0.11). Among the three 36 TB-based SIVs, the 36-P SIV has the highest maximum cross-correlation coefficient (0.83) from June to August. Its maximum cross-correlation coefficient is 2% and 8% higher than that of the 36-H and 36-V SIV, respectively; and its SD of the maximum cross-correlation coefficient is 6% and 21% lower than that of the 36-H and 36-V SIV, respectively. In summary, in addition to increasing the spatial coverage of SIV, using 36-P can also improve the quality of SIV results effectively.
3.3. Comparison with buoy SIV data
Although the polarization difference -derived SIV at 36 GHz has higher spatial coverage than from 36 TB, it is still not clear whether using 36-P can improve the accuracy of the SIV. As the most reliable SIV observations at present, buoy SIV data have been widely used in previous verification studies (Lavergne et al. Citation2010; Hwang Citation2013; Lei et al. Citation2020; Wang et al. Citation2022). Here, we use IABP buoy data as a reference to compare the PM-derived SIV obtained from different input fields. A total of 1965 buoy SIV samples are included in the statistical analysis in the summer of 2019. Our results show that, among the four sets of SIV results, 36-P SIV has the highest correlation of ice speed and the smallest SD of the difference in ice speed (). The 18-H SIV has the highest correlation of angle and the smallest SD of the difference in angle. The six statistical measures of 36-P SIV are relatively close to those of 18-H SIV, and are only lower than the 36-H and 36-V SIV in terms of angle and speed difference. This shows that, compared with the 36-H and 36-V SIV, the quality of 36-P SIV has been improved. It is worth noting that the SDs of the difference in sea-ice speed of the four datasets in this paper are higher than the result (1.86 km/d) reported by Wang et al. (Citation2022). We believe there are two reasons for this difference. First, our data have not been post-processed, so the noise of the data is higher than the operational product. Secondly, these two studies used different buoys and had different sample sizes.
Table 1. Statistics of SIV in summer 2019 with respect to buoy observations.
3.4. Comparison with SAR SIV
SAR data are becoming increasingly crucial in SIV research, but because the temporal consistency of SAR SIV data is lower than that of buoys, inconsistency between SAR and buoy SIV data has been reported (Palerme and Müller Citation2021). However, it is undeniable that the amount of SAR SIV data available is larger, and the spatial coverage is significantly improved compared to buoy data. Here, we also use the Sentinel-1 SAR SIV, with higher resolution and accuracy than PM SIV, as a reference to compare the PM-derived SIV obtained from different input fields. In order to reduce the impact of oversampling of SAR on the verification results, we take every third SAR SIV for comparison. Although we have not conducted a consistency check on the PM SIVs, samples with angle deviations greater than 90° from the SAR SIV are excluded. We obtain a total of 145,683 pairs of samples from May to September in 2019, and a total of 53,776 pairs of samples from June to August. The results () show that, in summer, the 18-H SIV has the lowest velocity and angle error, and the velocity error of the 36-P SIV is slightly higher than that of 18-H but significantly lower than that of the other two 36TB SIVs. From June to August, the 18-H SIV still has the lowest velocity and angle error, but the difference between the 18-H and 36-P SIV is significantly reduced. However, the statistical differences in the results between these two SIVs and the other two 36 TB-derived SIVs increase significantly.
Table 2. Statistics of SIV in summer 2019 with respect to SAR.
4. Discussion
The MCC algorithm is the most commonly used SIV tracking algorithm. This study uses a new scheme to improve the SIV results based on the CMCC tracking algorithm. It is not yet known whether the improved results are valid for the MCC algorithm. Therefore, we also use the MCC algorithm to retrieve the SIV in the summer of 2019. The results show that the spatial coverage of SIV obtained by the MCC algorithm is very similar to the results obtained by the CMCC algorithm, especially their seasonal changes (). The summer average spatial coverage of the four kinds of SIVs derived from the MCC method is 73.61%, 65.23%, 59.40% and 71.91%, respectively. This means that the new scheme can be applied to the MCC SIV tracking algorithm, which means that the new scheme is not sensitive to the tracking algorithm.
Figure 7. Monthly SIV (arrows) and spatial coverage of SIV (shading) derived from the MCC method in summer 2019.

This study uses the polarization difference fields for SIV retrieval from May to September. However, that is not to say that the algorithm is impractical in other seasons. (a) shows that the spatial coverage of 36-P SIV can still be close to 80% in early May and late September. However, the spatial coverage of 36-V and 36-H SIV during this period is higher than that of 36-P SIV, offering significant improvement compared to the melting period. The ice velocity quality based on 36-P is better in summer because the ice-water distinction is higher in summer; during the freezing period of sea ice, the water point proportion decreases, and the spatial variation of the ice surface’s characteristics replaces the ice-water discrimination into higher, easily identifiable features, which can explain why the ice velocity inverted using the 36-P scheme can mainly improve the quality of ice velocity during the melting period, rather than the sea-ice freezing period.
We notice that in the newly released daily SIV product by OSI SAF (OSI-455, available from https://osi-saf.eumetsat.int/products/osi-455), the summer SIV inversion only uses 36-V and 36-H TB and does not use the 18 GHz TB. Their higher spatial coverage of summer SIV is related to the post-processing method and data merging. We believe that their research results do not conflict with the results based on our new scheme. On the contrary, if the 36-P SIV is introduced into their system, the error of their SIV product will be further reduced.
This study mainly focuses on improving the quality of PM-derived SIV in summer. We are not committed to generating a new set of SIV data, but our methods can be easily applied to existing operational SIV retrieval systems. At the same time, although this research is based on AMSR2 data, it can be applied to PM sensors aboard the SSMIS system and FY series satellites.
5. Summary and conclusions
Arctic sea-ice motion is critical to the study of polar climate change, and summer sea-ice motion is particularly critical to the navigational safety of commercial ships. Compared with winter SIV data, summer SIV data contain larger errors and more missing values than in other periods. Combined with RRDP data, our research highlights that the lack of ice–water discrimination in summer for 36 GHz TB is the most important reason for the poor quality of its SIV results. Our study found that 36-P can distinguish sea ice and water in summer effectively. Therefore, we conducted SIV retrievals for 36-P using the CMCC tracking algorithm. Results showed that, compared with the SIV obtained by 18-H, 36-V and 36-H TB, the 36-P-derived SIV has higher spatial coverage in summer, at up to 86.91% (it can also reach 86.01% in June–August). Further analysis revealed that using 36-P can efficiently reduce the proportion of divergence in CMCC calculations, thereby improving the success rate of SIV tracking.
Through comparison with buoy and SAR data, although the SD of the SIV difference of 36-P is slightly higher than that of 18-H, it is significantly lower than that of 36-H and 36-V, and this difference is more significant in June–August. In summary, we believe that 36-P has great potential in retrieving SIV in summer, especially in June–August. Compared with using 18 GHz TB to retrieve SIV in summer, the difference in spatial resolution between the SIV retrieved based on 36-P and the SIV retrieved based on 36 GHz TB in other seasons will be significantly reduced. The advantage of this spatial resolution consistency will be more significant when studying sea-ice deformation and other sea-ice motion properties based on SIV.
Acknowledgments
The authors declare that they have no known competing financial interests or personal relationships that could have influenced the work reported in this study. We greatly appreciate the valuable comments from Thomas Lavergne and two anonymous reviewers.
Disclosure statement
No potential conflict of interest was reported by the author(s).
Data availability statement
Daily gridded brightness temperature is available at https://seaice.uni-bremen.de/data. OSI SAF sea ice concentration is available at https://osi-saf.eumetsat.int/products/osi-408-a. RRDP data sets are available at http://esa-cci.nersc.no/?q = round%20robin. IABP buoy data are available at https://iabp.apl.uw.edu/data.html. Sentinel-1 SAR sea ice drift is available at https://data.marine.copernicus.eu/product/SEAICE_GLO_SEAICE_L4_NRT_OBSERVATIONS_011_006/services. Ifremer sea ice drift is available at ftp://ftp.ifremer.fr/ifremer/cersat/products/gridded/psi-drift/. NSIDC sea ice motion is available at https://nsidc.org/data/nsidc-0116/versions/4.
Additional information
Funding
References
- Baordo, F., L. F. Vargas, and E. Howe. 2023. “OSI SAF Product User Manual for Global Sea Ice Concentration Level 2 and Level 3, OSI-410-a, OSI-401-d, OSI-408-a. Version 1, 1/11/2022.” Available online: https://osisaf-hl.met.no/sites/osisaf-hl/files/user_manuals/osisaf_pum_ice-conc_l2-3_v1p0.pdf.
- Cavalieri, D. J., P. Gloersen, and W. J. Campbell. 1984. “Determination of Sea Ice Parameters with the Nimbus 7 SMMR.” Journal of Geophysical Research: Atmospheres 89 (D4): 5355–5369. https://doi.org/10.1029/JD089iD04p05355.
- Ezraty, Robert, Fanny Girard-Ardhuin, and Jean-François Piollé. 2007. Sea Ice Drift in the Central Arctic Estimated From Seawinds/Quikscat Backscatter Maps User’s Manual.
- Gui, Dawei, Ruibo Lei, Xiaoping Pang, Jennifer K. Hutchings, Guangyu Zuo, and Mengxi Zhai. 2020. “Validation of Remote-Sensing Products of Sea-Ice Motion: A Case Study in the Western Arctic Ocean.” Journal of Glaciology 66 (259): 807–821. https://doi.org/10.1017/jog.2020.49.
- Howell, Stephen E.L., Alexander S. Komarov, Mohammed Dabboor, Benoit Montpetit, Michael Brady, Randall K. Scharien, Mallik S. Mahmud, Vishnu Nandan, Torsten Geldsetzer, and John J. Yackel. 2018. “Comparing L- and C-Band Synthetic Aperture Radar Estimates of Sea Ice Motion Over Different Ice Regimes.” Remote Sensing of Environment 204: 380–391. https://doi.org/10.1016/j.rse.2017.10.017.
- Hwang, Byongjun. 2013. “Inter-Comparison of Satellite Sea Ice Motion with Drifting Buoy Data.” International Journal of Remote Sensing 34 (24): 8741–8763. https://doi.org/10.1080/01431161.2013.848309.
- Ivanova, N., L. T. Pedersen, R. T. Tonboe, S. Kern, G. Heygster, T. Lavergne, A. Sørensen, et al. 2015. “Inter-Comparison and Evaluation of Sea Ice Algorithms: Towards Further Identification of Challenges and Optimal Approach Using Passive Microwave Observations.” The Cryosphere 9 (5): 1797–1817. https://doi.org/10.5194/tc-9-1797-2015.
- Kimura, Noriaki, Akira Nishimura, Yohei Tanaka, and Hajime Yamaguchi. 2013. “Influence of Winter Sea-Ice Motion on Summer Ice Cover in the Arctic.” Polar Research 32 (1): 20193. https://doi.org/10.3402/polar.v32i0.20193.
- Komarov, Alexander S., and David G. Barber. 2014. “Sea Ice Motion Tracking from Sequential Dual-Polarization RADARSAT-2 Images.” IEEE Transactions on Geoscience and Remote Sensing 52 (1): 121–136. https://doi.org/10.1109/TGRS.2012.2236845.
- Krumpen, Thomas, Luisa Von Albedyll, Helge F. Goessling, Stefan Hendricks, Bennet Juhls, Gunnar Spreen, Sascha Willmes, et al. 2021. “MOSAiC Drift Expedition from October 2019 to July 2020: Sea Ice Conditions from Space and Comparison with Previous Years.” The Cryosphere, https://doi.org/10.5194/tc-15-3897-2021.
- Kwok, Ron. 2008. “Summer Sea Ice Motion from the 18 GHz Channel of AMSR-E and the Exchange of Sea Ice Between the Pacific and Atlantic Sectors.” Geophysical Research Letters 35 (3), https://doi.org/10.1029/2007GL032692.
- Kwok, Ron, E. C. Hunke, W. Maslowski, D. Menemenlis, and J. Zhang. 2008. “Variability of Sea Ice Simulations Assessed with RGPS Kinematics.” Journal of Geophysical Research: Oceans 113 (11), https://doi.org/10.1029/2008JC004783.
- Lavergne, T., S. Eastwood, Z. Teffah, H. Schyberg, and L. A. Breivik. 2010. “Sea ice Motion from low-Resolution Satellite Sensors: An Alternative Method and its Validation in the Arctic.” Journal of Geophysical Research: Oceans 115 (10), https://doi.org/10.1029/2009JC005958.
- Lei, Ruibo, Dawei Gui, Jennifer K. Hutchings, Petra Heil, and Na Li. 2020. “Annual Cycles of Sea Ice Motion and Deformation Derived from Buoy Measurements in the Western Arctic Ocean Over Two Ice Seasons.” Journal of Geophysical Research: Oceans 125 (6), https://doi.org/10.1029/2019JC015310.
- Lu, Junshen, Georg Heygster, and Gunnar Spreen. 2018. “Atmospheric Correction of Sea Ice Concentration Retrieval for 89 GHz AMSR-E Observations.” IEEE Journal of Selected Topics in Applied Earth Observations and Remote Sensing 11 (5): 1442–1457. https://doi.org/10.1109/JSTARS.2018.2805193.
- Min, Chao, Qinghua Yang, Dake Chen, Yijun Yang, Xiangying Zhou, Qi Shu, and Jiping Liu. 2022. “The Emerging Arctic Shipping Corridors.” Geophysical Research Letters 49 (10), https://doi.org/10.1029/2022GL099157.
- Min, Chao, Qinghua Yang, Hao Luo, Dake Chen, Thomas Krumpen, Nabir Mamnun, Xiaoyu Liu, and Lars Nerger. 2023. “Improving Arctic Sea-Ice Thickness Estimates with the Assimilation of CryoSat-2 Summer Observations.” Ocean-Land-Atmosphere Research 2. https://doi.org/10.34133/olar.0025.
- Moore, G. W. K., S. E. L. Howell, M. Brady, X. Xu, and K. McNeil. 2021. “Anomalous Collapses of Nares Strait Ice Arches Leads to Enhanced Export of Arctic Sea Ice.” Nature Communications 12 (1), https://doi.org/10.1038/s41467-020-20314-w.
- Nelder, J. A., and R. Mead. 1965. “A Simplex Method for Function Minimization.” The Computer Journal 7 (4): 308–313.965. https://doi.org/10.1093/comjnl/7.4.308.
- Palerme, Cyril, and Malte Müller. 2021. “Calibration of Sea Ice Drift Forecasts Using Random Forest Algorithms.” The Cryosphere 15 (8): 3989–4004. https://doi.org/10.5194/tc-15-3989-2021.
- Rampal, P., J. Weiss, and D. Marsan. 2009. “Positive Trend in the Mean Speed and Deformation Rate of Arctic Sea Ice, 1979-2007.” Journal of Geophysical Research: Oceans 114 (5), https://doi.org/10.1029/2008JC005066.
- Shi, Q., J. Su, G. Spreen, and Q. Yang. 2022. “An Improved Sea-Ice Velocity Retrieval Algorithm Based on 89 GHz Brightness Temperature Satellite Data in the Fram Strait.” Earth and Space Science 9 (6), https://doi.org/10.1029/2021EA002170.
- Shokr, Mohammed, and Yufang Ye. 2023. “Why Does Arctic Sea Ice Respond More Evidently Than Antarctic Sea Ice to Climate Change?” Ocean-Land-Atmosphere Research 2. https://doi.org/10.34133/olar.0006.
- Spreen, Gunnar, Laura de Steur, Dmitry Divine, Sebastian Gerland, Edmond Hansen, and Ron Kwok. 2020. “Arctic Sea Ice Volume Export Through Fram Strait from 1992 to 2014.” Journal of Geophysical Research: Oceans 125 (6), https://doi.org/10.1029/2019JC016039.
- Spreen, Gunnar, Lars Kaleschke, and G. Heygster. 2008. “Sea ice Remote Sensing Using AMSR-E 89-GHz Channels.” Journal of Geophysical Research: Oceans 113 (2), https://doi.org/10.1029/2005JC003384.
- Sumata, Hiroshi, Thomas Lavergne, Fanny Girard-Ardhuin, Noriaki Kimura, Mark A. Tschudi, Frank Kauker, Michael Karcher, and Rüdiger Gerdes. 2014. “An Intercomparison of Arctic Ice Drift Products to Deduce Uncertainty Estimates.” Journal of Geophysical Research: Oceans 119 (8): 4887–4921. https://doi.org/10.1002/2013JC009724.
- Tschudi, Mark A., Walter N. Meier, and J. Scott Stewart. 2020. “An Enhancement to Sea Ice Motion and Age Products at the National Snow and Ice Data Center (NSIDC).” The Cryosphere 14 (5): 1519–1536. https://doi.org/10.5194/tc-14-1519-2020.
- Wang, Xue, Runtong Chen, Chao Li, Zhuoqi Chen, Fengming Hui, and Xiao Cheng. 2022. “An Intercomparison of Satellite Derived Arctic Sea Ice Motion Products.” Remote Sensing 14 (5), https://doi.org/10.3390/rs14051261.
- Yan, Qingyun, and Weimin Huang. 2016. “Spaceborne GNSS-R Sea Ice Detection Using Delay-Doppler Maps: First Results from the U.K. TechDemoSat-1 Mission.” IEEE Journal of Selected Topics in Applied Earth Observations and Remote Sensing 9 (10): 4795–4801. https://doi.org/10.1109/JSTARS.2016.2582690.
- Yan, Qingyun, and Weimin Huang. 2019. “Sea Ice Remote Sensing Using GNSS-R: A Review.” Remote Sensing, https://doi.org/10.3390/rs11212565.
- Zhang, Fanyi, Xiaoping Pang, Ruibo Lei, Mengxi Zhai, Xi Zhao, and Qiongqiong Cai. 2022. “Arctic Sea Ice Motion Change and Response to Atmospheric Forcing Between 1979 and 2019.” International Journal of Climatology 42 (3): 1854–1876. https://doi.org/10.1002/joc.7340.