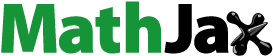
ABSTRACT
OpenStreetMap (OSM) has been playing increasingly important roles in location-based services, urban planning, tourism, and disaster management. In this paper, we examine a fundamental issue: can OSM reliably provide its services in the long run? To address this sustainable issue, we propose (1) inner cycle of career stages (i.e. activity levels) for monitoring the sustainable status of OSM and (2) critical mass theory for identifying driving factors that make OSM sustain. These new tools are used to track individual trajectories of editing behavior in 2005–2021. Our results suggest that OSM has been self-sustaining up to now and does not show a sign of decline yet. This is supported by the ever-decreasing turnover time and stabilized turnover rate. Together with the finding that most contributors transitioned to a higher career stage within a month, we identify an accelerated socialization process within OSM. We find further that it is the critical mass of people rather than content that makes OSM sustain. This contrasts with the common wisdom: recruiting more people with small contributions is far more important to the long-term growth of OSM than relying on a group of core members with large contributions. Implications and recommendations are critically discussed.
1. Introduction
Volunteered geographic information (VGI), in particular OpenStreetMap (OSM), has been a valuable source since its conception (Goodchild Citation2007). After almost 20 years of contribution by different communities, OSM has become an emerging open geospatial dataset with global coverage, sufficient quality, rich semantics, and near real-time updates (Haklay et al. Citation2014; Heipke Citation2010; Zhang et al. Citation2021). During its evolution, communities of armchair mappers, data imports, and corporate editors have emerged and formed their ways of contribution (Sarkar and Anderson Citation2022). To avoid misbehaviors such as malicious editing and vandalism (Li, Anderson, and Niu Citation2021), guidelines were introduced by the OSM Foundation at different stages (Anderson, Sarkar, and Palen Citation2019). However, this could be a double-edged sword. Halfaker et al. (Citation2013) for instance show that Wikipedia has experienced an irreversible decline (since 2007) after a continuous increasing of editors. This was mainly caused by the inappropriate use of quality control and automated tools; the tools and mechanisms were intended for quality control in the face of inflow of massive contributors, but eventually calcified changes contributed by newer editors and made them harder to socialize into the system. This should be highly vigilant for the OSM community as it attracts increasingly more users and is the basis of many applications to date. In this paper, we aim to examine the existence of any sign of such a decline, and more importantly, if and how the OSM project is self-sustaining in the long run. We claim that this is an fundamental issue after the data quality of OSM.
The research on OSM started from the general interest in the quality and usefulness of the data (Haklay et al. Citation2014; Mohammadi and Sedaghat Citation2019; Neis and Zielstra Citation2014; Wang et al. Citation2013; Zacharopoulou, Skopeliti, and Nakos Citation2021). Indeed, the nature of public participation results in uneven quality of OSM data. Data quality approaches generally fall into two categories (Honarparvar, Saeedi, and Liang Citation2021): (i) extrinsic assessment referring to external or ground-true data and (ii) intrinsic assessment without reference to third-party data. In the extrinsic approach, OSM data are compared to official data (Borkowska and Pokonieczny Citation2022; Zhang et al. Citation2022; Zhang and Malczewski Citation2018; Zhou et al. Citation2022) or to knowledge explicitly encoded (Homburg and Boochs Citation2019). As an example of the intrinsic approach, Zhang et al. (Citation2021) proposed a method that automatically identifies and corrects misplaced attributes (tags) in road networks without resorting to reference data.
As data quality improves, OSM becomes more and more popular in wider applications. One such area where OSM has the advantages over other data sources is the disaster response and management (Ahmouda, Hochmair, and Cvetojevic Citation2018; Fohringer et al. Citation2015; Sakaki, Okazaki, and Matsuo Citation2010). A key example is the Humanitarian OpenStreetMap Team (HOT), where OSM has been playing an increasingly important role (Soman et al. Citation2020). On the other hand, the use of OSM by GIS professionals such as national mapping agencies and data providers is also increasing (Haklay et al. Citation2014; Olteanu-Raimond et al. Citation2017). Leading companies such as Wikipedia, Amazon, Facebook, and Flickr also use OSM to provide location-based services.Footnote1
Meanwhile, corporate editors (CEs) hired/paid by companies like Apple, Facebook, and Mapbox have been intensively involved around 2016 and presented a new form of contribution (Dickinson, Patel, and Sarkar Citation2022; Sarkar and Anderson Citation2022). Corporate editors can make large number of edits in a short time, presenting a highly productive force. Different from non-profit editors who are mainly driven by altruistic purposes (Budhathoki and Haythornthwaite Citation2013), CEs are compensated and hence motived by their employers (for-profit groups). Currently their focus is more on features like road networks (Anderson, Sarkar, and Palen Citation2019; Dickinson, Patel, and Sarkar Citation2022) and is shown to significantly improve the quality of these features (Patel et al. Citation2023; Veselovsky et al. Citation2022). They are also different from the early organizational contributions such as TIGER import in the USA and AND/BAG import in the Netherlands (Anderson, Sarkar, and Palen Citation2019), because CEs are editing the map by people rather than importing data by scripts. In the future, the CE community is likely to change the territory and direction of the OSM project if it outweighs the non-profit community or becomes a mainstream of contribution (Sarkar and Anderson Citation2022).
Corporate involvement also fostered a surge of volunteered editors (different from the paid CEs). Location-based games (e.g. Pokémon GO and Ingress since 2017) began to use OSM as their map services, which attracts a sizeable number of new users to use and contribute to OSM.Footnote2 Autonomous vehicle manufactures present another example. In 2019, a Tesla owner found that OSM may have been used in Tesla’s routing and self-driving system.Footnote3 This finding further attracted an inburst of new mappers, probably Tesla owners, to join OSM and map parking aisles to enhance their driving experience (Anderson and Sarkar Citation2020).
As the reliance on crowd-sourced mapping projects such as OSM increases, the sustainability of such projects become crucial (Zhang et al. Citation2021). Our main research question is therefore: can projects like OSM reliably provide their services in the long run? Here, we aim to answer this question by tracking individual trajectories of editing behavior. Related work is reviewed in the following.
1.1. Literature review
The understanding of contributors’ motivations and editing patterns is at the core of crowdsourcing projects (Budhathoki and Haythornthwaite Citation2013). Many studies explored and analyzed user behavior in relation to the crowdsourcing platforms. For example, Panciera, Halfaker, and Terveen (Citation2009) analyzed the behavioral patterns of Wikipedia contributors and found that power users are born, not made, meaning that they become active or highly active very quickly instead of upgrading step-by-step. Thebault-Spieker, Hecht, and Terveen (Citation2018) explored contribution behavior in OpenStreetMap and found a spatial version of such a ‘born, not made’ character.
An understanding of contributor behavior patterns can also be applied to other respects. Bégin, Devillers, and Roche (Citation2013) for instance argued that an understanding of contributor behavior can help assess the quality of VGI data. Scholars have also revealed that patterns of contributor behavior can reflect the inner state of the platform. For example, Xia et al. (Citation2021) found that although OSM suffered from contributor loss, the retention of new editors remained stable throughout its history. Halfaker et al. (Citation2013) analyzed the negative impact of Wikipedia’s quality control tools on contributor recruiting, and revealed that the use of such tools was the main cause of editor loss and hence the decline. The decline of Wikipedia should be vigilant for the OSM community. It is important to ask if OSM already appears a similar trend and can the project continue to thrive?
On the one hand, online community is highly dynamic and changes drastically over time; all editors, no matter how active they used to be, eventually stop contributing (Halfaker et al. Citation2013). Although the OSM community seems to grow as a whole by looking at basic numbers, this does not give too much insight into its inner status and does not have any predictive power. To better understand the community and predict its future trajectory, it is necessary to break down the community and inspect the behavior of its sub-groups (Neis and Zipf Citation2012). To this end, users can be distinguished by their activity levels according to the power-law distribution, or the 1-9-90 rule (Thebault-Spieker, Hecht, and Terveen Citation2018). However, a classification based on static criteria such as total edits can be limited: (1) users contributing heavily in the past may no longer be active years later and (2) a static classification cannot distinguish between user activities with temporal variations.
On the other hand, studies show that the success of an online community is related to the number of participants it maintains (Ducheneaut Citation2005; Halfaker et al. Citation2013), especially the small minority of power users (Peddibhotla and Subramani Citation2007). The critical mass theory in sociology adds that when a committed minority of a community reaches a critical size the community becomes self-sustaining and creates further growth (Iacopini et al. Citation2022; Oliver and Marwell Citation1988; Oliver, Marwell, and Teixeira Citation1985). However, recent studies on online community suggest that it is not just the number, and not even the number of power users, that matters. For example, Solomon and Wash (Citation2014) inspected over a thousand WikiProjects and revealed a counter-intuitive correlation: it is the critical mass of people, especially the majority of users who infrequently contribute, makes a crowd-sourcing project sustain long-term growth; such growth can be counteracted by the overwhelming participation of power users in early stages. This seems to be against the general wisdom that power users are critical to an online community (Panciera, Halfaker, and Terveen Citation2009; Peddibhotla and Subramani Citation2007). Does the OSM community bear a similar character? If this is the case, what user policy should OSM make for its future success?
1.2. Problem statement and research questions
This paper aims to study the sustainable status of the OSM community over time. With its evolution OSM might cease to grow, not because the map is completed but due to the increasing regulations introduced which could be harmful for new users to socialize into the system (see, e.g. Halfaker et al. Citation2013). It is now important for the OSM community to consider this sustainability issue. To our knowledge, this is the first time such an issue is identified and addressed for the OSM community.
We argue that inspecting the increase or decrease of users and content (i.e. edits uploaded) does not give too much insight into the sustainable status of OSM, as it would be too late once we see the decreasing trend. For example, OSM could still be able to sustain itself even when it slows down or stops the growth of its user base. On the other hand, the project can be already in its early declining stage but the number of editors and content are still rising for some time (so no one realizes that). This can be the case if new users keep coming in but fail to socialize into the center of the community, and the remaining active users are currently contributing but will eventually leave the project. Therefore, it is important to device intrinsic measures to reveal and monitor the inner status of OSM which cannot be predicted by the number indicators widely used in previous work.
Our contributions lie in the proposal of inner cycle of OSM career stages (i.e. user’s transition between different activity levels, see Section 3.2 for details), which models the process of socializing new users into core members of the community, and the measures to quantify the transitions of individual editors between career stages (see Section 3.3). Transition analysis (e.g. turnover time and rate for editors to transition to higher career stages) can reflect the sustainable status of OSM and can be used as early warning indicators (e.g. a low and decreasing turnover rate). In addition, critical mass theory (see Section 3.4) is tested on OSM for the first time to unravel what factors drive the project to sustain, if at all? This should lead to recommendations as to how the community can be self-sustaining in the future.
Specifically, our analysis of historical data (see Section 2) differs from the existing studies. That is, we keep track of individual trajectories of all editors during their OSM career during 2005–2021 and calculate the dates when each editor transitioned to different career stages (i.e. activity levels). With this we are able to measure the time and rate of the transition (see Section 3.3). The sheer amount and richness of such individual-level information allow us to reveal the dynamics of career transitions over the full history of OSM, which signals the sustainable status of OSM. This is only possible with intrinsic measures that go beyond basic statistics.
Finally, our main research objective can be formulated into four research questions:
How quick and at what rate do OSM editors transition to different career stages in the OSM history?
What are the turnover (transition between two career stages) time and rate for editors of different types (e.g. editors ranked top 1%, top 9%, and top 90%)? Are active editors born to be active?
Are active editors durable in their OSM lifespan? How long do different types of editors remain active in OSM?
How well does the OSM project grow (accelerating or decelerating) in terms of changes (edits) uploaded by users? Does the community need more content or editors to become self-sustaining? which editors?
2. Data acquisition
We use OSM changeset to answer the research questions. Changeset records temporal and spatial information of edits uploaded by OSM contributors during OSM’s history and is continuously updated. These include user ID, bounding box (BBox) of edits (rectangle geometry), creation and closing time, number of edits, and others (five random records are shown in ). The dataset can be used to understand when, where, and how many edits have been submitted to the OSM database and hence the user behavior.
Table 1. Examples of the original changeset data (only relevant fields are shown).
In this paper, a full sample of changeset data was collected for over 16 years (April 2005–July 2021), amounting to 107,025,268 records in total. The data were obtained from the official Planet OSM websiteFootnote4 and imported into a local PostgreSQL database for subsequent processing and analysis. Two pre-processing steps were performed after data entry, including data cleaning and temporal aggregation. The former removes the data items with missing or abnormal values (e.g. missing id and #edits in ). The latter aggregates the number of edits by an editor on a monthly basis, forming a table containing 4,756,200 records ().
Table 2. Examples of the monthly-edits-per-user table.
3. Methodology
3.1. Overall analytical framework
Our analytical framework () mainly consists of two parts: (1) we propose inner cycle of career stages and transition analysis to inspect and monitor the sustainable status of OSM; (2) we use critical mass theory to identify what factors make OSM to sustain.
To begin with, we define career stages and analyze how users transition between them during the full history of OSM (RQ1). To compare and analyze contributors with different activity levels, we follow the static classification in Thebault-Spieker, Hecht, and Terveen (Citation2018) and divide the contributors into top 1%, top 9%, and top 90% contributors (1-9-90 rule) in terms of the total edits they made. Combined with the concept of inner cycle of career stages, it is interesting to see how users of different (static) activity levels transition between career stages (RQ2) and how durable they are after transitioning to certain stages (RQ3).
3.2. Inner cycle of career stages
Here we propose the inner cycle of career stages to model the user socialization process in the OSM community (). To retain a sustainable pool of core contributors, new users need to be continuously socialized into the community. Career trajectories can be different: some move from the periphery to the center while others end up with leaving the community (Halfaker et al. Citation2013). To explore the dynamics of editors’ career trajectories and to reveal the sustainable status of OSM, we distinguish between five career stages according to users’ dynamic activity levels (i.e. monthly edits uploaded), and track the transition of individual users between stages.
Figure 2. Proposed concept of inner cycle of OSM career stages (a) and possible career trajectories between periphery and core of the community (C wanders near the periphery longer than B before entering the core; whereas A is a passer-by).

The career stages include Registered user (REG), First-time Editor (FE), New Editor (NE), Active Editor (AE), and Highly Active Editor (HAE), using the criteria in . These stages are motivated by many studies (e.g. Neis and Zipf Citation2012; Neis, Zielstra, and Zipf Citation2013; Thebault-Spieker, Hecht, and Terveen Citation2018). Our approach complements these studies as most previous classifications are static (i.e. based on total edits per user). Here, FE is chosen as it identifies real contributors in OSM. NE is a static concept as users become new editor only once when they uploaded 100 edits, which is similar to Neis and Zipf (Citation2012). AE and HAE are dynamic status that reflects instantaneous activity levels of users. We set the criterion of HAE empirically to 10,000 edits/month so that no users in the top 90% group (i.e. the least active group) can reach the HAE stage; it was not easy to find a threshold that clearly isolates the top 1% from the top 9%. Then the criterion for AE was chosen as a middle value between NE and HAE such that most top 1% and 9% contributors can transition to this stage. Since these categories are empirical (as in most previous studies), our goal is to make the criteria simple and effective. We also tried different thresholds and the main findings hold the same.
Table 3. Contributor career stages in terms of activity level (monthly edits).
A similar term called ‘life cycle phases’ was coined by Bégin, Devillers, and Roche (Citation2018), where phases are defined by how long a user stayed (survived) in the OSM community. Once a user survived to a later phase, the user will not go back to earlier phases. The concept does not necessarily reflect users’ activity levels. Our proposed career stages are dynamic status and reflect the instantaneous vitality (activity level) of a user. Editors usually go back and forth between different stages during their lifespan, metaphorically mimicking career transitions in real-world enterprises. This gives an inner view as to how an organization sustains for a certain period.
3.3. Transition measures
Transition measures include time and rate for editors to transition between career stages (i.e. turnover time and rate) and duration after transition. Turnover is a notion in employee management (Saporta and Farjoun Citation2003), and we use it to refer to transitions from lower to higher stages (turnover of promotion). To carry out the analysis, we calculate the dates when the editor transitions to each of the five career stages (e.g. when a user’s activity level qualifies the stage criterion in ) for the first time using data in the monthly-edit-per-user table (). This produces a so-called user versus transition-date matrix (). The matrix tracks every editor’s career trajectory during 2005–2021.
Figure 3. User versus transition-date matrix records the date when each editor transitions to a career stage for the first time; the dimension is 1,716,176 users × 196 months.

Turnover time is the time taken for a contributor to transition from activity class G to G + 1 and is measured for a specific month in our analysis. It can be calculated as follows:
(1)
(1) where TG + 1 denotes the first time when the contributor becomes class G + 1 and TG denotes the first time when the contributor becomes class G. For example, if we want to measure the turnover time for NE-AE type of transition in 2009/09 (TG + 1), we do the following: (1) find all the users who transitioned to AE in this month (e.g. user_id = {24, 35}); (2) for each user we find the time when the user transitioned to NE (located to the left in the matrix) and mark it as TG, and then turnover time for this user can be obtained using Equation (1); (3) we obtain turnover times for all the users at AE stage in this month. Such a measure is carried out for all types of transitions and for all months.
Turnover rate is the rate at which contributors move from class G to G + 1. We define turnover rate as the ratio between the number of editors transitioned to class G + 1 (NG + 1) and the total number of editors at class G in each month (NG):
(2)
(2) Again, if we want to measure the turnover rate for NE–AE type of transition in 2009/09, we do the following: (1) for each row in this month we look back in time and count the users (NG + 1) whose previous stage (activity level) is NE (user_id = {24, 35, 54, 3612}); (2) count how many of them (NG) transitioned to AE this month (red AE in ); (3) calculate turnover rate in this month using Equation (2). Turnover rate is measured for all types of transitions and for all months.
With the user versus transition-date matrix, we also measure (1) the times a user becomes AE and HAE, (2) intervals between adjacent status of becoming active, and (3) durations of being active. This gives insight into how long a user keeps active after becoming active for the first time.
3.4. Critical mass theory
Critical mass theory is formalized into a production function, which can be used to explain the relationships between individual contributions to a group and the collective output of the group (Oliver, Marwell, and Teixeira Citation1985; Raban, Moldovan, and Jones Citation2010). Online communities have either accelerating, linear, or decelerating production. For a community with accelerating production function, contributions in later stages have greater return for the community.
We will model the growth of OSM data revision (editing) and contributors, respectively. As the whole dataset spans over 16 years and can be a mixture of different growth patterns, we segment the sequence data into sub-sequences. For each sub-sequence, we calculate the cumulative percentage of this sequence’s total edits or contributors up to the end of this sequence. To examine if the growth is acceleration or deceleration, we fit a second-order polynomial model (Solomon and Wash Citation2014) for each sub-sequence:
(3)
(3) where Y is the community output (number of edits or editors);
describes the rate of growth (not relevant for properties of acceleration);
indicates the degree of acceleration (positive) or deceleration (negative); R2 is used to measure the goodness-of-fit of the model. Such analysis (see Section 4.4) can get insight into the growth patterns of the OSM project in different times and how the growth of editors relates to the growth of revisions (edits).
To further answer which critical mass may help sustain the OSM community (edits or editors), we fit a negative binomial regression model to evaluate effects of the number of editors or edits at year one on the number of revisions at several years later, with statistical soundness (see Section 4.4.2). Such statistics can be further used to estimate which activity classes have greater impact on a community’s future growth (see Section 4.4 for details).
4. Results and discussion
4.1. Exploration of OSM growth
The statistics of the number of OSM contributors converted to each dynamic level are shown in . As of July 2021, OSM has accumulated 7,770,880 registers, of which only 22% of contributors made at least one edit and became first-time editors (FE). This means that over 3/4 of OSM registers are the ‘silent majority’ and have not contributed at all. New editors (NE) who have contributed 99.8% of total edits accounted for 32.6% of all FE. The number of contributors transitioned to active editors (AE) and highly active editors (HAE) accounted for 10.3% and 2.0% of all FE, and have contributed 98.5% and 92.7% of total edits, respectively.
Table 4. Statistics of the number of contributors and edits.
The above statistics confirm the participation inequality in the OSM community observed by Xia et al. (Citation2021). From our change set perspective, the vast majority (98.5%) of the OSM changes was made by a tiny portion of active editors (2.3% of all registered users).
depicts the growth of editors per category (e.g. NE, AE, HAE) who are transitioned from other categories for the first time during 2005–2021 on a monthly basis. The curves are raw data and not smoothed by moving averages. In general, the increment of editors for all activity classes steadily increased over time. Although fluctuations exist for the increment, the number of editors of all classes are generally boosting in an acceleratory manner.
Figure 4. Monthly increment of editors transitioned to each activity class during 2005–2021 (a); growth in AE (b) and HAE (c) are visually enlarged for clarity (the three phases divided by dash lines are explorative rather than definitive).

To ease subsequent explorations, we segment the 16 years of growth into three phases, where the splitting point also can be confirmed by important events in OSM and other researchers (Bégin, Devillers, and Roche Citation2018; Sarkar and Anderson Citation2022; Xia et al. Citation2021). In early development (2005–2007), newly added editors get increased slowly, where contributors registered before 2007 were ‘innovator’; the sudden increase from 2007 to 2009 may be attributed to the fact that OSM got more and more popularized when ‘early adopters’ began to advocate OSM in the world (Bégin, Devillers, and Roche Citation2018). Together, years before 2009 are labeled early growth in . From 2009 to 2016, the increment of FE still grows but the pace of growth slows down; while the increment of AE and HAE appears to hold steady, indicating that the turnover rate of editors in the two classes is more or less constant.
Around 2016, the increment of first-time editors suddenly jumped to a high level and leveled off (the fast growth phase in a). This may be attributed to the intensive involvement of corporate editors (CEs) since 2016 (Anderson and Sarkar Citation2020; Anderson, Sarkar, and Palen Citation2019; Sarkar and Anderson Citation2022). As CEs usually contributed many edits during a short duration, this might also explain the more prominent increase in active and highly active editors later in this stage (b and c), who were transitioned from the large swarm of FEs.
To summarize, we show that OSM is still attracting a steady stream of new contributors, who seem to increasingly transition themselves to upper activity classes over 16 years. The four curves of increment in are highly similar (with Pearson's coefficient p > 0.8), and all show an upward trend. To get more insight, we will study quantitatively how contributors transition to different career stages (i.e. activity levels)
4.2. Transitions between OSM career stages
Although transitions between any two activity classes are possible (see a), we will focus on the four basic transition types in our study: REG-FE, FE-NE, NE-AE, and AE-HAE. Since a contributor’s registration time cannot be obtained in an automatic, batch mode, representative contributors were sampled to analyze the REG-FA type of transition. The remaining three types of transition are analyzed for all the editors in our changeset data.
4.2.1. Turnover time distribution
30,000 users were sampled in the four career stages to analyze their REG-FE turnover time. These 120,000 users’ registration dates were crawled from the user homepages on OSM website. Cumulative distribution functions (CDF) of the turnover time in the four groups are shown in . It indicates that over 60% of the contributors who eventually became FE finished their first edits on the day of registration, nearly 85% of the contributors made their transition within 30 days, and more than 95% of the contributors finished their turnover in less than 365 days. If a registered user does not make one edit within a year, the probability for the user to revise the map is significantly low. Surprisingly, our result suggests that users of higher activity levels have lower probability of making their first edits in shorter time. For instance, less editors in HAE contributed the first edit on their registration days than editors in FE (cf. a and d). Such a phenomenon needs further study.
Figure 5. CDF of the REG–FE turnover time of editors in different stages: (a) first-time editor, (b) new editors, (c) active editors, and (d) highly active editor.

(a) shows the distribution of turnover time for editors to transition to the three stages at a yearly bin. For all types of transitions, 86%–93% of the contributors went to a higher stage within a year. Apparently, transitions to HAE are slower than transitions to NE or AE. This could be the side effect of setting the threshold value too high for the HAE level. Likewise, (b) depicts the distribution of the turnover time at a monthly bin. It shows that nearly 50%–80% of the contributors transitioned into higher stages within a month. The monthly view confirms the above-mentioned finding: the transition from AE to HAE is significantly longer than the other transition types. Additionally, the three curves in (b) are well fitted to power-law models.
4.2.2. Turnover time dynamics
To study how turnover time varies over time, we analyzed, for all editors of a stage in current month (horizontal axis in ), the time needed to take them to a higher class (turnover time). Then first quartile, median, and third quartile of their turnover time are plotted over 16 years in . Error bars are placed in the enlarged inserts. Significant fluctuations are present in the early phase of OSM (before 2007) due to the small user base. Meaningful findings can be supported in later, more stable phases (e.g. after 2009). Generally, the turnover time becomes shorter with the evolution of OSM regardless of the transition types. FE-NE and NE-AE types of transitions display a similar pattern: editors tend to transition to a higher activity class within a month on average. Turnover time for the AE-HAE type is significantly longer at all quantiles (c, insert). Since 2016, the transition seems to be accelerated: the third quantile of turnover time reduced to less than a month for FE-NE and NE-AE types, and less than 3 months for AE-HAE. This is correlated with the surge of corporate editors as described in Anderson, Sarkar, and Palen (Citation2019).
4.2.3. Turnover time in different users
To see how editors ranked in top 1%, 9%, and 90% (see Section 2.2.1) transition between career stages, we study the turnover time in different static groups (). Will active users finish their inner cycle more quickly than less active users?
Overall, the turnover time in all 1-9-90 groups for three types of transitions is decreasing over time. The quartiles in the boxplot get close to 0 in later stages of OSM development, indicating that editors completed their transitions to higher classes in the same month they became the previous class. The transition from AE to HAE still took longer than other types of transitions. This is consistent with previous analysis for all editors (see Section 4.2.2). Horizontally, transitions to higher classes (e.g. AE-HAE) generally took longer than transitions to the lower ones (e.g. NE-AE). Longitudinally, editors that contributed more in total (e.g. top 1% editors) finished their transitions significantly quicker than those contributed less (e.g. top 9% editors). Such a trend is even clear in the early years of OSM.
4.2.4. ‘Born or made’ dilemma
Thebault-Spieker, Hecht, and Terveen (Citation2018) showed that OSM edits tend to have geographic biases in their contribution, which are ‘born, not made’. This means that ‘born’ power users become active shortly after they made the first contribution, rather than being socialized into the core members step-by-step. For example, most corporate editors are ‘born’ to be active editors. Our previous results confirm that active users in OSM also exhibit this ‘born, not made’ character. First, during the lifespan of an editor, the turnover time is generally short, e.g. most transitions to a higher stage were completed in the same month this editor has just become the previous stage. If a contributor does not become active early in their lifespan, the probability of transition to a higher activity level is rather low. In other words, there is no editors who become active in a slow pace. Second, editors who made more contributions in total, transitioned to a higher stage more quickly, i.e. they are always more active throughout their OSM lifespan.
4.2.5. Turnover rate analysis
Turnover rate shows what proportions of editors in an activity class transition to a higher class (). The general trend of turnover rate for three types of transitions is similar. The rate is very high in the early days of OSM and declines quickly until around 2009. This corresponds to the ‘innovators’ and ‘early adopters’ periods coined in Bégin, Devillers, and Roche (Citation2018). After 2009, the turnover rate levels off. Specifically, the turnover rate for FE-NE type of transition displays an early decrease followed by a slight increase to around 40%. For NE-AE, the rate stabilizes at around 30%. For AE-HAE, the rate seems to decrease slightly even for later stages.
Further analysis reveals the difference of turnover rates in the top 1%, 9%, and 90% editors. Except for the early fluctuations (before 2009), the turnover rates of three types of transitions are relatively stable. Almost all top 1% editors eventually became highly active editors. For top 9% editors, all of them completed the FE-NE transition, most of them became active editors, and 15%–20% of them transitioned to highly active editors. For the 90% remaining editors, 20%–40% of them finished 100 edits, less than 10% became active editors, and no one became highly active. After 2016, the turnover rate for FE-NE transition experienced significant increase (, all contributors), which might be explained by the large inflow of corporate editors since then (Anderson, Sarkar, and Palen Citation2019).
4.3. How long do editors remain active?
This question is related to the studies concerning retention and attrition (Mahmud et al. Citation2022; Xia et al. Citation2021) but with a different focus on sustainability. To answer this question, we focus on active and highly active editors and explore the occurrences of editors being active (see Section 4.3.1), intervals between the status of being active (see Section 4.3.2), and active durations (see Section 4.3.3).
4.3.1. Occurrences of being active
We analyzed the number of times editors became active (or highly active), as shown in . First, the occurrences follow power-law distribution meaning that becoming active/highly active is an intermittent (or burst) behavior. A majority of editors (about 60% AE and 45% HAE) became active (or highly active) only once in their lifespan. Few users become active editors many times. Nevertheless, the result suggests that highly active editors generally had more chances of becoming highly active and showed greater dedication to OSM.
4.3.2. Intervals between being active
The CDF of the intervals in shows that the time interval for a user to become active (or highly active) in consecutive times was rather short: more than 70% of the times the interval was within a month, about 90% of the times the interval was less than 6 months, and within a year nearly 95% of cases editors became active/highly active twice. Though not being significantly different from each other, the time intervals for HAE were slightly shorter than those for AE, indicating again that HAEs are more frequent workers.
4.3.3. Durations of being active
To study how long AE and HAE stay active (i.e. monthly edits > 1,000), in our study, if the interval between adjacent status of becoming active is less than a year, we define this interval as being continuously active. Continuous active intervals of an editor are added up as the editor’s active duration. CDFs of active durations for AE and HAE are depicted in . For active editors, more than 75% of them kept active for less than a year, and nearly 85% kept active for less than 2 years. For highly active editors, more than 55% were continuously active for less than 1 year and nearly 75% stayed in active for less than 2 years.
Taken together, our results indicate that highly active editors are more dedicated and persistent contributors, and they remain active significantly longer than active editors. However, few editors stayed in active status more than 5 years. This confirms the observation by Halfaker et al. (Citation2013) that all users eventually stop to contributing.
4.4. What makes OSM sustain?
4.4.1. Modeling OSM growth
First, we model the grow of OSM content (edits) as well as contributors in different stages of OSM development. We divided the whole historical data into six phases (). The fitting results show that most phases exhibit accelerating growth of total edits as well as editors (β2 > 0 with R2 > 0.99). The modeling predicts that OSM was self-sustaining in terms of both content and contributors even in recent years.
Table 5. Growth of content (edits) and editors as predicted by production functions.
It is worth noting that accelerating growth of editors does not ensure an accelerating growth of edits (2010–2013). Even when the growth of editors is decelerating, the growth of content can exhibit an accelerating pattern (2016–2019). Hence, what exactly is the critical mass that helps sustain the OSM project?
4.4.2. What is critical?
To answer the above question, we fitted regression models to predict revisions (edits) made to OSM after 5 years based on the number of editors and that of edits. The coefficients of the two terms (editors or edits) can be used to explain which one is the critical mass (more important). We fitted the model to data starting from each month and the output 5 years later, obtaining 195 samples from April 2005 to June 2016. As the number of edits after 5 years is over dispersed (the variance much larger than the mean), we used a negative binomial regression model (see Section 2.2.4).
The model (, left column) shows a statistically significant effect of the number of editors at year 1 on the number of revisions after 5 years, holding the number of edits after 1 year constant. On the other hand, holding the number of editors at year 1 constant, the number of edits after 1 year had a little effect on the number of edits after 5 years. To put this into perspective, if 50 editors increase their contributions from 1000 to 5000 edits at year 1, OSM would have increased 290 edits after 5 years. However, if the 5000 edits were contributed by 100 editors, there would be 127,132 more edits at year 5.
Table 6. Effect of 1 year of growth in editors and edits (left column), or growth in first-time editors and active editors (right column), on 5 years’ growth in revisions.
This implies that people is more likely the critical mass that makes OSM sustain. Getting more people with less contribution each leads to better long-term growth of OSM than getting large amount of early contributions from a small group of people.
To further explore the effect of user activity on the future production, we fitted again a negative binomial regression taking the number of editors of different activity classes as input. Finally, we evaluated only first-time editors and active editors since editors of other classes did not pass the multicollinearity test. The results (, right column) showed that the number of AE at year 1 had a slightly larger impact than the number of FE (an order of magnitude larger), on the number of edits 5 years later. This is somewhat different from Solomon and Wash (Citation2014), who showed that it is the majority of non-power user that makes the community in WikiProjects sustain longer. Apparently OSM is different from the wiki projects in that both types of editors are important for OSM’s future growth.
5. Conclusion and outlook
Sustainability is identified in this paper as a fundamental issue for the OSM community after data quality. To address this issue, we provide a new perspective and a set of new tools: (1) the inner cycle of career stages (including transition analysis) that models the socialization process within OSM, and for revealing and monitoring the sustainable status of OSM, and (2) the critical mass theory for identifying driving factors that make OSM sustain in the long term. Moreover, the proposed intrinsic measures (e.g. turnover time and rate) can be used as early warning indicators for spotting the tendency of a potential decline, which cannot be predicted by existing approaches. The methodology has wider applications in online communities beyond OSM. Specifically, the methodology was used to analyze the full sample of historical changeset of OSM (2005–2021) and the main findings and implications are summarized as follows.
First, the transitions of individual users between career stages are highly dynamic during the full history of their OSM lifespan. We reveal for the first time that turnover time keeps reducing over time, implying an accelerating socialization process going on within OSM, and that turnover rate tends to stabilize from 2009 to most recently. This is a sign that OSM has been self-sustaining for a long time and does not show a tendency of decline. The ever-faster socialization process can be attributed to two possible reasons: (1) OSM is becoming easier for new users to socialize into the center of the community and (2) professional editors who are faster and more dedicated in contributing are increasingly involved.
However, the acceleration may also have a negative implication. That is, editors joining OSM later in time become more short-lived than before as the time for a new user to become a core member has been shortened. This can be compounded by our findings concerning the limited duration after transitioning to active stages (e.g. no one stayed active for more than 5 years). The question is that, it is not yet clear if such an accelerated socialization is correlated with the increasing involvement of more professional editors (e.g. corporate editors), and if professional editors persist longer than normal users after becoming core members. If this is not the case, OSM would have to meet the challenge of recruiting new users in an ever-faster pace. Either way, further research is required in the future.
Then the critical mass theory predicts that people is far more critical than the content (edits contributed per person) in sustaining the long-term growth of OSM. This finding implies that relying on a group of core members to make more contributions is not as sustainable as recruiting more people and each making small contributions. This contrasts with the common wisdom established for OSM. We show further that both active and non-active editors are important for OSM’s future growth. A recommendation is that, carefully adopting quality control mechanisms and fostering a welcoming editing experience for newcomers is far more important than relying on large number of early contributions from a small group of power users for OSM to self-sustain in the long term.
In the future, it is interesting to carry out an in-depth analysis of how professional editors (e.g. CEs) impact the future trajectory of OSM. Since many companies and organizations have disclosed the names (OSM id) of CEs, their contribution behavior and impact can be studied independently (Anderson, Sarkar, and Palen Citation2019).
Acknowledgements
We are grateful to the reviewers whose comments substantially improved this paper.
Disclosure statement
No potential conflict of interest was reported by the author(s).
Data availability statement
Data supporting the findings of this study can be made available upon request.
Additional information
Funding
Notes
1 OpenStreetMap usage: https://en.wikipedia.org/wiki/OpenStreetMap#Usage
References
- Ahmouda, Ahmed, Hartwig Hochmair, and Sreten Cvetojevic. 2018. “Analyzing the Effect of Earthquakes on OpenStreetMap Contribution Patterns and Tweeting Activities.” Geo-spatial Information Science 21 (1): 1–18. doi:10.1080/10095020.2018.1498666.
- Anderson, Jennings, and Dipto Sarkar. 2020. “Curious Cases of Corporations in OpenStreetMap.” In Proceedings of the Academic Track at State of the Map 2020. https://doi.org/10.5281/zenodo.3923050.
- Anderson, Jennings, Dipto Sarkar, and Leysia Palen. 2019. “Corporate Editors in the Evolving Landscape of OpenStreetMap.” ISPRS International Journal of Geo-Information 8 (5): 232. doi:10.3390/ijgi8050232.
- Bégin, Daniel, Rodolphe Devillers, and Stéphane Roche. 2013. “Assessing Volunteered Geographic Information (VGI) Quality Based on Contributors' Mapping Behaviours.” ISPRS International Archives of the Photogrammetry, Remote Sensing and Spatial Information Sciences, XL-2/W1. doi:10.5194/isprsarchives-XL-2-W1-149-2013.
- Bégin, Daniel, Rodolphe Devillers, and Stéphane Roche. 2018. “The Life Cycle of Contributors in Collaborative Online Communities – The Case of OpenStreetMap.” International Journal of Geographical Information Science 32 (8): 1611–1630. doi:10.1080/13658816.2018.1458312.
- Borkowska, Sylwia, and Krzysztof Pokonieczny. 2022. “Analysis of OpenStreetMap Data Quality for Selected Counties in Poland in Terms of Sustainable Development.” Sustainability 14 (7), doi:10.3390/su14073728.
- Budhathoki, Nama R., and Caroline Haythornthwaite. 2013. “Motivation for Open Collaboration:Crowd and Community Models and the Case of OpenStreetMap.” American Behavioral Scientist 57 (5): 548–575. doi:10.1177/0002764212469364.
- Dickinson, Corey, Jugal Patel, and Dipto Sarkar. 2022. “Corporate Editing and its Impact on Network Navigability Within OpenStreetMap.” In State of the Map 2022 (SotM 2022). https://doi.org/10.5281/zenodo.7004583.
- Ducheneaut, Nicolas. 2005. “Socialization in an Open Source Software Community: A Socio-Technical Analysis.” Computer Supported Cooperative Work (CSCW) 14 (4): 323–368. doi:10.1007/s10606-005-9000-1.
- Fohringer, J., D. Dransch, H. Kreibich, and K. Schröter. 2015. “Social Media as an Information Source for Rapid Flood Inundation Mapping.” Natural Hazards and Earth System Sciences 15 (12): 2725–2738. doi:10.5194/nhess-15-2725-2015.
- Goodchild, Michael F. 2007. “Citizens as Sensors: The World of Volunteered Geography.” GeoJournal 69 (4): 211–221. doi:10.1007/s10708-007-9111-y.
- Haklay, Mordechai, Vyron Antoniou, Sofia Basiouka, Robert Soden, and Peter Mooney. 2014. Crowdsourced Geographic Information Use in Government. London: World Bank.
- Halfaker, Aaron, R. Stuart Geiger, Jonathan Morgan, and John Riedl. 2013. “The Rise and Decline of an Open Collaboration System How Wikipedia’s Reaction to Popularity Is Causing Its Decline.” American Behavioral Scientist 57 (5): 664–688. doi:10.1177/0002764212469365.
- Heipke, Christian. 2010. “Crowdsourcing Geospatial Data.” ISPRS Journal of Photogrammetry and Remote Sensing 65 (6): 550–557. doi:10.1016/j.isprsjprs.2010.06.005.
- Homburg, Timo, and Frank Boochs. 2019. “Situation-Dependent Data Quality Analysis for Geospatial Data Using Semantic Technologies.” In Business Information Systems Workshops. BIS 2018. https://doi.org/10.1007/978-3-030-04849-5_49.
- Honarparvar, Mohammad Malek, Sara Saeedi, and Steve Liang. 2021. “Towards Development of a Real-Time Point Feature Quality Assessment Method for Volunteered Geographic Information Using the Internet of Things.” International Journal of Geo-Information 10: 1–27. doi:10.3390/ijgi10030151.
- Iacopini, Iacopo, Giovanni Petri, Andrea Baronchelli, and Alain Barrat. 2022. “Group Interactions Modulate Critical Mass Dynamics in Social Convention.” Communications Physics 5 (1): 64. doi:10.1038/s42005-022-00845-y.
- Li, Yinxiao, Jennings Anderson, and Yiqi Niu. 2021. “Vandalism Detection in OpenStreetMap via User Embeddings.” In Proceedings of the 30th ACM International Conference on Information & Knowledge Management, 3232–3236. Virtual Event, Queensland, Australia: Association for Computing Machinery.
- Mahmud, Zarif, Aarjav Chauhan, Dipto Sarkar, and Robert Soden. 2022. “Revisiting Engagement in Humanitarian Mapping: An Updated Analysis of Contributor Retention in OpenStreetMap.” In Extended Abstracts of the 2022 CHI Conference on Human Factors in Computing Systems, Article 251. New Orleans, LA, USA: Association for Computing Machinery.
- Mohammadi, Nazila, and Amin Sedaghat. 2019. “A Framework for Classification of Volunteered Geographic Data Based on User's Need.” Geocarto International 36 (1): 1–15. doi:10.1080/10106049.2019.1641562.
- Neis, Pascal, and Dennis Zielstra. 2014. “Recent Developments and Future Trends in Volunteered Geographic Information Research: The Case of OpenStreetMap.” Future Internet 6 (1): 76–106. doi:10.3390/fi6010076.
- Neis, Pascal, Dennis Zielstra, and Alexander Zipf. 2013. “Comparison of Volunteered Geographic Information Data Contributions and Community Development for Selected World Regions.” Future Internet 5 (2): 282–300. doi:10.3390/fi5020282.
- Neis, Pascal, and Alexander Zipf. 2012. “Analyzing the Contributor Activity of a Volunteered Geographic Information Project — The Case of OpenStreetMap.” ISPRS International Journal of Geo-Information 1 (2): 146–165. doi:10.3390/ijgi1020146.
- Oliver, Pamela Elaine, and Gerald Marwell. 1988. “The Paradox of Group Size in Collective Action: A Theory of the Critical Mass. II. *.” American Sociological Review 53 (1): 1. https://doi.org/10.2307/2095728
- Oliver, Pamela, Gerald Marwell, and Ruy Teixeira. 1985. “A Theory of the Critical Mass: I. Interdependence, Group Heterogeneity, and the Production of Collective Action.” American Journal of Sociology 91 (3): 522–556. doi:10.1086/228313.
- Olteanu-Raimond, Ana-Maria, Glen Hart, Giles M. Foody, Guillaume Touya, Tobias Kellenberger, and Demetris Demetriou. 2017. “The Scale of VGI in Map Production: A Perspective on European National Mapping Agencies.” Transactions in GIS 21 (1): 74–90. doi:10.1111/tgis.12189.
- Panciera, K., A. Halfaker, and L. Terveen. 2009. “Wikipedians Are Born, Not Made: A Study of Power Editors on Wikipedia.” In Proceedings of the ACM 2009 International Conference on Supporting Group Work, 51–60. ACM.
- Patel, Jugal, Corey Dickinson, Raja Sengupta, and Dipto Sarkar. 2023. “Intrinsic Data Quality of Corporate Contributions to OpenStreetMap: Assessing Completeness.” In Proceedings of Spatial Knowledge and Information Canada 2023, SKI 2023, short paper 12. https://skiconference.ca/2023/papers/SKI2023_paper_12.pdf.
- Peddibhotla, Naren B., and Mani R. Subramani. 2007. “Contributing to Public Document Repositories: A Critical Mass Theory Perspective.” Organization Studies 28 (3): 327–346. doi:10.1177/0170840607076002.
- Raban, D. R., M. Moldovan, and Q. Jones. 2010. “An Empirical Study of Critical Mass and Online Community Survival.” In Proceedings of the 2010 ACM Conference on Computer Supported Cooperative Work, 71-80. ACM.
- Sakaki, Takeshi, Makoto Okazaki, and Yutaka Matsuo. 2010. “Earthquake Shakes Twitter Users: Real-Time Event Detection by Social Sensors.” In Proceedings of the 19th International Conference on World Wide Web, 851–860. Raleigh, NC: Association for Computing Machinery.
- Saporta, Ishak, and Moshe Farjoun. 2003. “The Relationship Between Actual Promotion and Turnover among Professional and Managerial-Administrative Occupational Groups.” Work and Occupations 30 (3): 255–280. doi:10.1177/0730888403253892.
- Sarkar, Dipto, and Jennings T. Anderson. 2022. “Corporate Editors in OpenStreetMap: Investigating co-Editing Patterns.” Transactions in GIS 26 (4): 1879–1897. doi:10.1111/tgis.12910.
- Solomon, Jacob, and Rick Wash. 2014. “Critical Mass of What? Exploring Community Growth in WikiProjects.” In Proceedings of the 8th International Conference on Weblogs and Social Media, ICWSM 2014, vol. 8: 476–484. doi:10.1609/icwsm.v8i1.14546.
- Soman, Satej, Anni Beukes, Cooper Nederhood, Nicholas Marchio, and Luís M. A. Bettencourt. 2020. “Worldwide Detection of Informal Settlements via Topological Analysis of Crowdsourced Digital Maps.” ISPRS International Journal of Geo-Information 9 (11): 685. doi:10.3390/ijgi9110685.
- Thebault-Spieker, Jacob, Brent Hecht, and Loren Terveen. 2018. “Geographic Biases are ‘Born, not Made': Exploring Contributors’ Spatiotemporal Behavior in OpenStreetMap.” In Proceedings of the 2018 ACM International Conference on Supporting Group Work, 71–82. Sanibel Island, FL: Association for Computing Machinery.
- Veselovsky, Veniamin, Dipto Sarkar, Jennings Anderson, and Robert Soden. 2022. “An Automated Approach to Identifying Corporate Editing.” Proceedings of the International AAAI Conference on Web and Social Media 16 (1): 1052–1063. doi:10.1609/icwsm.v16i1.19357.
- Wang, M., Q. Li, Q. Hu, and M. Zhou. 2013. “Quality Analysis of Open Street map Data.” International Archives of the Photogrammetry, Remote Sensing and Spatial Information Sciences – ISPRS Archives 40: 155–158. doi:10.5194/isprsarchives-XL-2-W1-155-2013.
- Xia, Qiongyan, Guan Luo, Xiang Zhang, and Liang Zhou. 2021. “Volunteer Editing Behavior Retention Analysis of OpenStreetMap.” Journal of Geomatics 44 (2): 90–93, 97. doi:10.3969/j.issn.1672-5867.2021.02.022.
- Zacharopoulou, Dimitra, Andriani Skopeliti, and Byron Nakos. 2021. “Assessment and Visualization of OSM Consistency for European Cities.” ISPRS International Journal of Geo-Information 10 (6): 361. doi:10.3390/ijgi10060361.
- Zhang, Hongyu, and Jacek Malczewski. 2018. “Accuracy Evaluation of the Canadian OpenStreetMap Road Networks.” International Journal of Geospatial and Environmental Research 5: Article 1. https://dc.uwm.edu/ijger/vol5/iss2/1.
- Zhang, Xiang, Tianfu Wang, Delin Jiao, Zhiying Zhou, Jianwei Yu, and Xiao Cheng. 2021. “Detecting Inconsistent Information in Crowd-Sourced Street Networks Based on Parallel Carriageways Identification and the Rule of Symmetry.” ISPRS Journal of Photogrammetry and Remote Sensing 175: 386–402. doi:10.1016/j.isprsjprs.2021.03.014.
- Zhang, Yuheng, Qi Zhou, Maria Antonia Brovelli, and Wanjing Li. 2022. “Assessing OSM Building Completeness Using Population Data.” International Journal of Geographical Information Science 36 (7): 1443–1466. doi:10.1080/13658816.2021.2023158.
- Zhou, Qi, Yuheng Zhang, Ke Chang, and Maria Brovelli. 2022. “Assessing OSM Building Completeness for Almost 13,000 Cities Globally.” International Journal of Digital Earth 15 (1): 2400–2421. doi:10.1080/17538947.2022.2159550.