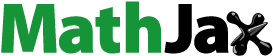
ABSTRACT
Frequent water quality anomalies severely affect the ecological environment, hindering the protection, restoration, and sustainable use of inland freshwater ecosystems in Sustainable Development Goals (SDG) 15.1. Thus, there is an urgent need to conduct real-time monitoring and early warnings of various water quality anomalies. However, current remote sensing indices of water quality anomalies are aimed at only one specific water anomaly event in water regions, which makes it difficult to achieve high-precision and on-orbit detection of sudden water quality anomalies in complex geographical environments with the limited storage and computing resources of satellites. Therefore, this study proposed a remote sensing index for the detection of multi-type water quality anomalies in complex geographical environments by analyzing the spectral differences between different earth’s surfaces. The validation results indicated that the proposed index achieved satisfactory performance for the synchronous detection of most water quality anomalies and could be used directly in complex geographical environments to detect water anomalies. Overall, this study contributes to the realization of real-time water quality anomaly detection methods in complex geographical environments, which increases the possibility of on-orbit water quality anomaly detection.
1. Introduction
In 2015, the United Nations adopted the 2030 Agenda for Sustainable Development, which contains 17 Sustainable Development Goals (SDGs) and 169 targets (Guo Citation2020). SDG 15.1 proposed ensuring the conservation, restoration and sustainable use of terrestrial and inland freshwater ecosystems and their services. However, the frequent occurrence of various water quality anomaly events in recent years (e.g. water blooms, green tides, red tides, black and odorous water, floods, oil spills, and water pollution) has severely affected the stability and sustainable development of inland freshwater ecosystems. Under the pressure of SDG 15.1, it is urgent to conduct real-time monitoring and early warnings of various water anomaly events.
First, it is necessary to illustrate the meaning of water quality anomalies. Currently, there is no unified definition of water quality anomalies in the existing literature. By referring to the definitions of anomalies in the fields of environment (NNU_Group Citation2011), computer vision (Gu, Fei, and Sun Citation2020), and the existing definition of earth’s surface anomalies (Wei et al. Citation2023), water quality anomalies could be defined as ‘the phenomenon threatening the natural or social environment, which destroys the stable balanced state of water-covered areas conforming to the historical evolutionary law or suddenly transforms non-water areas into water-covered areas, under the single or double influence of natural and human factors’.
Existing near-real-time detection methods for water quality anomalies generally use multi-source remote sensing satellites to form a virtual constellation to improve the temporal resolution. For example, Landsat satellites have been combined with other optical satellites such as MODIS (Doña et al. Citation2015; Min et al. Citation2012) and Sentinel-2 (Pahlevan et al. Citation2019; Tulbure et al. Citation2022), and with radar satellites such as COSMO-SkyMed (Tong et al. Citation2018) and Sentinel-1 (DeVries et al. Citation2020). However, they require a long process from task planning, satellite operation control, and ground data transmission to information extraction, which generally takes one to four days (Caballero et al. Citation2022; Tulbure et al. Citation2022; Wang et al. Citation2002). Therefore, the existing methods are confronted with the problems of long service chains, complex processes, and slow responses. Their timeliness is far from meeting the actual needs of emergency services for water quality anomalies characterized by minute-level responses.
Many studies have shown that on-orbit detection using remote sensing data is an essential way to realize the real-time detection of earth’s surface anomalies (Liu et al. Citation2020; Shao et al. Citation2019). Based on the algorithms stored in satellites, it can complete the detection of earth’s surface anomalies on orbit and directly transmit the detection results to the subscriber mobile terminal, which can markedly simplify the detection process and improve the response speed (Wei et al. Citation2023). Remote sensing features are the basis for constructing on-orbit detection methods for earth’s surface anomalies (Wei et al. Citation2023; Yu et al. Citation2020). Especially in recent years, on-orbit data preprocessing technologies have been greatly developed and many methods have been proposed, such as on-orbit radiometric calibration (Huang et al. Citation2023; Jiang, Zou, and Chen Citation2022), on-orbit geometric calibration (Wang et al. Citation2020), and on-orbit atmospheric correction (Li et al. Citation2022). These progresses make it possible to construct the remote sensing indices on orbit. Therefore, it is necessary to construct a remote sensing index for the real-time detection of water quality anomalies that can effectively identify water quality anomaly information and adapt to the limited storage and computing resources of satellites.
Currently, there are various remote sensing indices used to reflect the characteristics of water quality anomalies, such as the normalized difference algae index (NDAI) (Shi and Wang Citation2009), Forel Ule index (FUI) (Li et al. Citation2016; Yang et al. Citation2022), floating algae index (FAI) (Zhao et al. Citation2014), MODIS red tie index (MRI) (Soto et al. Citation2015), and black and odorous water index (BOI) (Yao et al. Citation2019). However, these remote sensing indices can distinguish water quality anomalies only from normal water but cannot achieve reliable performance in distinguishing water quality anomalies from non-water areas (Alharbi Citation2023; Handler et al. Citation2023). For example, the FAI values of green tides and blooms are similar to those of vegetation-covered areas (Hu et al. Citation2010; Jia, Zhang, and Dong Citation2019), and the BOI values of black and odorous water are similar to those of artificial surfaces (Yao et al. Citation2019). This is because these remote sensing indices are generally composed of a few bands where water quality anomalies are markedly different from normal water, but non-water areas are similar to the water quality anomalies in these bands. Therefore, the current remote sensing indices of water quality anomalies can only be used for water regions, and it is necessary to obtain the spatial distribution of water areas before using these indices of water quality anomalies (Kislik et al. Citation2022; Wang et al. Citation2021). However, all land cover products or land cover classification using remote sensing data inevitably introduce classification errors. For example, the user accuracies of water in ESRI Land Cover 2020, FROM_GLC 10 (2017), and Global Land 30 are approximately 83.00% (Karra et al. Citation2021), 86.39% (Gong et al. Citation2019), and 84.70% (Chen et al. Citation2015), respectively. If water quality anomaly detection is conducted based on current land cover products, it is inevitable that some non-water areas will be misidentified as water quality anomalies and generate nonnegligible identification errors. Therefore, it is difficult for current remote sensing indices to effectively and rapidly identify water quality anomalies in complex geographical environments.
Furthermore, current remote sensing indices of water quality anomalies are applicable to only one specific water quality anomaly event, such as green tides (Han et al. Citation2022), black and odorous water, floods (Hagos et al. Citation2020), and oil spills (Lu et al. Citation2013). If the detection of water quality anomalies is carried out on orbit, the remote sensing index of each water quality anomaly event must be combined into a large remote sensing feature system, which complicates the detection method of water quality anomalies and contradicts the detection results owing to misclassification from different indices. Meanwhile, almost all remote sensing indices can judge only whether the regions of interest are water quality anomalies in the real-time acquired remote sensing data and cannot judge whether they are long-term stable water quality anomalies or sudden water quality anomalies. If they are used to detect sudden water quality anomalies, it is necessary to construct a large prior knowledge base reflecting the normal earth’s surface states (Wei et al. Citation2023) based on the remote sensing feature system to determine whether the regions of interest are sudden. The enormous amount of prior knowledge required undoubtedly challenges the limited storage and computing resources of satellites.
On the whole, under the conditions of limited storage and computing resources in satellites, the current remote sensing indices of water quality anomalies cannot realize high-precision and on-orbit detection of sudden water quality anomalies in complex geographical environments. Therefore, this study proposed a remote sensing index for the detection of multi-type water quality anomalies in complex geographical environments. First, this study analyzed the spectral characteristics of normal water, water quality anomalies, and non-water areas. Then, a unified remote sensing index of water quality anomalies was constructed based on their spectral characteristics. Finally, the proposed index was validated under different scenarios to prove its effectiveness.
Overall, the main contribution of this study is proposing an index for various water quality anomaly detection instead of combining various remote sensing indices, which can substantially reduce the volume and complexity of detection methods and increase the possibility of real-time water quality anomaly detection in complex geographical environments. The structure of the paper is as follows: Section 2 presents the spectral characteristics of different earth’s surfaces and the construction of the proposed index. Section 3 compares the detection results for different water quality anomalies using the proposed index with those of other traditional indices. Section 4 discusses the differences in spectral characteristics of different earth’s surfaces and the advantages, limitations, and prospects of the proposed method in this study. Section 5 summarizes the conclusions.
2. Methodology
2.1. Spectral characteristic analysis of different earth’s surfaces
To analyze the spectral characteristics of different earth’s surfaces, this study constructed spectral reflectance curves based on Sentinel-2 multispectral data. Specifically, this study randomly selected 600, 1200, and 800 sample points from normal water areas, water quality anomalies, and non-water areas, respectively. The selected water quality anomaly events covered all types of water quality anomalies, including water blooms, green tides, red tides, black and odorous water, floods, oil spills, and water pollution. The selected non-water areas also covered common land cover types, including vegetation, barren land, artificial surface, ice and snow. Then, this study constructed the spectral reflectance curves of different earth’s surfaces based on the selected points. The reasonable maximum and minimum reflectance values of different earth’s surfaces in each band were determined by the quartile distance method (Equations (1-2)), and all abnormal reflectance values were eliminated. Finally, the mean reflectance of different earth’s surfaces in each band was calculated using EquationEquation (3(3)
(3) ), and the spectral reflectance curves of different earth’s surfaces () were constructed based on these mean reflectance.
(1)
(1)
(2)
(2)
(3)
(3)
max(bi, rk) and min(bi, rk) represent the maximum and minimum reflectance values of the rkth earth’s surface in the bith band, respectively. Q1 and Q3 represent the first and third quartile distances, respectively. fmean(bi, rk) represents the mean reflectance of the rkth earth’s surface at the bith band. v(bi, rk, n) represents the reflectance of the rkth earth’s surface in the bith band and nth random point. n represents the number of random points on the rkth earth’s surface.
The reflectance values of normal water in each band are relatively low, showing a rapidly decreasing trend from the blue band as the wavelength increases. The reflectance values of the water quality anomalies in each band were higher than those of normal water, and the spectral reflectance curves for each water quality anomaly were apparently different. Specifically, the largest reflection peaks of both green tides and water blooms were in the near infrared (NIR) band, and the smaller reflection peaks were in the green band. The spectral reflection peak of red tides was in the red band, and the reflectance values decreased rapidly with increasing wavelength after reaching this peak. The reflectance of black and odorous water in each band was relatively similar, and there were no evident reflection peaks or absorption valleys. The reflection peak of the light-yellow floods was in the red edge 1 (RE1) band and the absorption valley was in the SWIR1 and SWIR2 bands. The reflection peak of light-green floods was in the green band, and its reflectance values gradually decreased with increasing wavelength. The reflectance of the oil spills (black oil slicks) in each band was relatively low, with little difference between the bands. The reason for analyzing only the spectral characteristics of black oil slicks is that they are the first state of oil spills, and monitoring them will help achieve the goal of early warning. Similarly, water pollution is a complex and diverse anomaly, and only one type of water pollution is shown in . To compensate for this deficiency, this study found that the reflection peaks of water pollution were primarily distributed in the red, green, and NIR bands, as reported in related literature (Li et al. Citation2018; Liu Citation2008; Xu, Deng, and Liu Citation2007). Overall, the spectral characteristics of water anomalies were considerably different from those of normal water. Their reflection peaks shifted to larger wavelengths (such as green, red, RE1, and NIR bands), and the differences between the blue and red bands were much lower than those of normal water.
The reflectance values of the non-water areas in each band were higher than those of the normal water and most water quality anomalies, and the spectral reflectance curves of each non-water area were different. Specifically, the largest reflection peak of vegetation was in the NIR narrow 2 (NIRn2) band, and a smaller reflection peak was in the green band, which is similar to that of water blooms and green tides. However, their reflectance values in the SWIR1 and SWIR2 bands were apparently higher than those in the red, green, and blue bands, which distinguished them from water blooms and green tides. The reflectance of barren land and artificial surfaces showed a rapidly increasing trend with increasing wavelength. The spectral reflectance curve of ice and snow showed a decreasing trend with increasing wavelength, but the reflectance values in most bands were apparently higher than those of other earth’s surfaces. Overall, the spectral characteristics of non-water areas were considerably different from those of normal water and water quality anomalies, and the wavelengths of the reflectance peaks in non-water areas, except for ice and snow, were larger than those of water quality anomalies and normal water.
2.2. The construction of remote sensing index for water quality anomaly detection
Based on the analysis of spectral characteristics of different earth’s surfaces, this study constructed a comprehensive remote sensing index for the detection of water quality anomalies (WAI). To construct the WAI, this study first constructed a remote sensing index (WAI_0, EquationEquation (4(4)
(4) )) that reflected normal water information based on the high reflection and absorption bands of normal water.
(4)
(4)
Blue and SWIR1 represent the blue and shortwave infrared 1 bands of Sentinel-2 multispectral data. Then, the high reflectance bands of the water quality anomalies were subtracted from those of normal water to separate the water quality anomalies from normal water. Based on the spectral characteristic analysis, the reflection peaks of the water quality anomalies were in the green, red, RE1, and NIR bands. However, considering the availability of the remote sensing index for different satellite sensors (e.g. Landsat satellites), this study selected only the green, red, and NIR bands and constructed WAI_1 based on WAI_0 (EquationEquation (5(5)
(5) )).
(5)
(5)
Green, NIR, and Red represent the green, near-infrared, and red bands of Sentinel-2 multispectral data, respectively. For normal water, all the items in the numerator of EquationEquation (5(5)
(5) ) were positive. For water quality anomalies, because their reflection peaks moved to larger wavelengths, there were both positive and negative numbers in the numerator terms of EquationEquation (5
(5)
(5) ). Therefore, the WAI_1 values of the water quality anomalies were lower than those of normal water.
For barren land, because the reflectance gradually increased with increasing wavelength, each item in the numerator of EquationEquation (5(5)
(5) ) was negative. Their WAI_1 values were lower than those of the water quality anomalies. However, due to the large reflectance difference between the blue, green, and NIR bands during high-intensity green tides and water blooms, their WAI_1 values were also negative and may have been lower than those of barren land. To solve this problem, this study added normalization of the blue and SWIR 2 bands based on WAI_1 (EquationEquation (6
(6)
(6) )), which made the WAI_2 values of water quality anomalies higher than those of barren land. This is because the reflectance values of normal water and water quality anomalies in the SWIR2 band were lower than or close to their reflectance in the blue band, whereas the reflectance values of non-water areas except ice and snow, in the SWIR2 band were higher than their reflectance in the blue band:
(6)
(6) SWIR2 represents the shortwave infrared 2 band of Sentinel-2 multispectral data. For the vegetation-covered areas, most items in the numerator of EquationEquation (6
(6)
(6) ) were negative with relatively higher absolute values, and only the ‘(green–red)’ item was positive with a lower absolute value. Therefore, their WAI_2 values are also lower than those of the water quality anomalies.
As the spectral characteristics of most artificial surfaces were similar to those of barren land, WAI_2 could still be used to distinguish between water quality anomalies and most artificial surfaces. However, WAI_2 may not be suitable for some special artificial surfaces, such as bright white and blue artificial surfaces. Their reflectance in the red, green, and blue bands was relatively high, and the difference in reflectance from that in the shortwave infrared bands was small, which made their WAI_2 values close to those of the water quality anomalies. Based on the Google Earth Engine (GEE) platform, this study selected a large number of images containing bright white and blue artificial surfaces at different locations and different imaging times. Then, 1500 sample points were randomly selected from the areas of bright white and blue artificial surfaces to extract the blue band reflectance. In addition, approximately 1800 sample points were randomly selected when constructing the spectral curves of normal water and water quality anomalies, and their reflectance in the blue band was counted. According to statistics, the reflectance of approximately 99% of bright white and blue artificial surfaces is greater than 0.13, which is also higher than that of normal water and water quality anomalies. Meanwhile, the reflectance of ice and snow in the blue band is higher than that of almost all earth’s surfaces. Therefore, this study set the earth’s surfaces with reflectance in the blue band higher than 0.13 as non-water areas, and the final remote sensing index for the detection of water quality anomalies was obtained by combining this condition with WAI_2 (EquationEquation (7(7)
(7) )).
(7)
(7)
‘⌊⌋’ and ‘⌈⌉’ represent round down and round up.
2.3. Validation of the proposed WAI
2.3.1. Specific water quality anomaly detection experiments
To validate the proposed remote sensing index, this study randomly selected eight different water quality anomaly events, six different non-water areas, and a normal water area as the experimental areas based on Sentinel-2 data with a 10-m spatial resolution. The selected water quality anomaly events covered all common water quality anomaly types, including water blooms, green tides, red tides, black and odorous water, light-yellow floods, light-green floods, oil spills, and water pollution. The selected non-water and normal water areas also covered most land cover types, including forests, grasslands with high vegetation coverage, grasslands with low vegetation coverage, barren land, artificial surfaces, ice and snow, and water. lists the anomaly types, data acquisition time, and location information for the selected experimental areas.
Table 1. The acquisition time and location information of the selected Sentinel-2 data.
For experimental areas with water quality anomalies, grayscale images of the WAI were first calculated based on Sentinel-2 multispectral data. Then, this study obtained grayscale images of water quality anomaly indices applicable to one special water quality anomaly event. Specifically, the FAI, EVI, RI, BOI, modified normalized difference water index (MNDWI) (Xu Citation2006), normalized difference water index (NDWI) (Gao Citation1996), fluorescence index (FI) (Lin et al. Citation2018), and water pollution difference index (WPDI) (Zhang and Zhang Citation2017) were used to detect water blooms, green tides, red tides, black and odorous water, light-yellow floods, light-green floods, oil spills, and water pollution.
To observe the separation degrees of non-water areas, normal water, and water quality anomalies in different remote sensing indices more intuitively, this study drew frequency distribution maps of different earth’s surfaces with different remote sensing indices. Then, this study determined suitable segmentation thresholds according to the value distribution ranges of different earth’s surfaces with different remote sensing indices, and completed the identification of water quality anomalies. Finally, this study summarized the value ranges of the WAI in water quality anomalies and initially formed a recommended threshold range.
2.3.2. Accuracy assessment
For the detection accuracy assessment of water quality anomalies, this study randomly and evenly selected 260, 320, 400, 250, 400, 600, 250, and 220 validation points in the regions with water blooms, green tides, red tides, black and odorous water, light-yellow floods, light-green floods, oil spills, and water pollution, respectively. Then, the water quality anomaly types of the validation points were determined visually (i.e. whether they were water anomalies) by comparing the target images and their multiperiod historical images (Sentinel-2 or images with a higher spatial resolution). Finally, a confusion matrix was constructed to calculate the precision, recall, and F1-score of water anomaly detection using Equations (8-10):
(8)
(8)
(9)
(9)
(10)
(10) where TP represents the number of validation points that are water quality anomalies and are correctly identified as water quality anomalies, FP represents the number of validation points that are non-water anomalies but are incorrectly identified as water quality anomalies, and FN represents the number of validation points that are water quality anomalies but are incorrectly identified as non-water anomalies. In addition, because there were no water quality anomalies in the experimental areas with normal water and non-water areas, the precision, recall, and F1-score could not be calculated.
3. Results
shows natural color images, grayscale images of remote sensing indices, and the detection results of water quality anomalies. For the grayscale images of the WAI, the brightness of normal water was the highest, followed by water quality anomalies and non-water areas. Their brightness was substantially different and well-delimited, which made it easy to distinguish them. For the remote sensing indices applicable to one special water quality anomaly event, the difference in brightness between the water quality anomalies and normal water was clear. However, the difference between the water quality anomalies and non-water areas was weak. It is difficult to distinguish water quality anomalies from non-water areas using these remote sensing indices.
Figure 2. The natural color images, grayscale images of remote sensing indices, and detection results of water quality anomalies. White represents the water quality anomalies, and black represents the non-water quality anomalies in the detection results.

These characteristics are illustrated in . For the WAI, there was almost no overlap in the frequency curves among the different earth’s surfaces. However, the frequency curves of water quality anomalies generally overlap with those of non-water areas in the existing remote sensing indices, which indicated that the gray values of water quality anomalies are similar to those of non-water areas. For example, the gray values of water blooms were similar to those of low-vegetation cover and artificial surfaces using FAI. For the EVI and RI, the differences between green tides, red tides, and the regions with high vegetation cover were not clear. There were no significant differences in the BOI, FI and WPDI between black and odorous water, oil spills, polluted water, and artificial surfaces. In the experimental areas with floods, although the NDWI and MNDWI could distinguish between water quality anomalies and non-water areas, there were problems. For example, the MNDWI could not distinguish between normal water and water quality anomalies, and the NDWI could detect only a few water anomaly quality events.
Figure 3. The frequency distribution of non-water areas, normal water areas, and water quality anomalies for different remote sensing indices.
The red lines in represent the segmentation thresholds of different water quality anomalies. These red lines were located at the intersections of different frequency curves, which could effectively distinguish between the different earth’s surfaces. Based on these segmentation thresholds, this study obtained the detection results of water quality anomalies (). The water quality anomaly detection results based on WAI were consistent with the spatial distribution of the visual interpretation. Although these these currently existing remote sensing indices detected most water quality anomalies, they also misidentified many non-water areas as water quality anomalies. From the perspective of accuracy, the precision values of the WAI were above 84.00%, the recall values were above 90.00%, and the F1-scores were above 0.88 in all experimental areas with water quality anomalies (). The recall values of the currently existing remote sensing indices were also above 90.00%. However, most of their precision values were below 84.00% (as low as 32.24%), and the F1-scores were similarly uneven, with most of them being below 0.88.
Table 2. The precision, recall, and F1-scores of different water quality anomaly detection results.
shows that the segmentation thresholds between non-water areas and water quality anomalies using the WAI were approximately −0.11 to −0.15, and the segmentation thresholds between water quality anomalies and normal water were approximately 0.70–1.05. Therefore, the median values of the above two value ranges (−0.125 and 0.875) were selected to form the recommended threshold range for the detection of water quality anomalies. Based on the recommended threshold range, this study generated detection results for water quality anomalies in experimental areas with different non-water areas and normal water (). The grayscale images showed that the WAI could distinguish between different land cover types, and the boundaries of different earth’s surfaces were relatively clear. The detection results showed that the WAI could effectively distinguish between normal water, water quality anomalies, and non-water areas, and there were almost no misidentified areas. Only when the experimental area was artificial surfaces were a few slightly gray or dark brown artificial surfaces misidentified as water quality anomalies, which might have been caused by the fact that the colors of these earth’s surfaces were darker and their reflectance was similar in each band, bringing their WAI values higher than those of normal artificial surfaces and closer to water quality anomalies.
4. Discussion
4.1. The spectral characteristic differences between different earth’s surfaces
Based on the analysis of spectral reflectance curves for different earth’s surfaces, this study found that the spectral characteristics of water quality anomalies were clearly different from those of normal water and non-water areas. Compared to normal water, the spectral reflection peaks of the water quality anomalies moved to larger wavelengths. This phenomenon might be caused by the fact that the content of non-water substances (such as plankton, suspended sediment, nutrient salt, and other pollutants) in water continually increased with the occurrence of water quality anomalies, and the spectral reflectance curves of water quality anomalies no longer reflected only the water information but also the comprehensive information of water and other substances. The wavelengths of the reflectance peaks for the non-water substances were much larger than those for normal water, and the mixtures of water and non-water substances had larger values than those of normal water. The higher the content of non-water substances, the greater the difference between the spectral characteristics of normal water and water quality anomalies. Similarly, because most non-water areas contained no water information, the wavelengths of their reflection peaks were larger than those of the water quality anomalies and normal water. It should be noted that ice and snow are other forms of water, and their reflectance also show a decreasing trend with increasing wavelength. However, the reflectance values in most bands were much higher than those of normal water and water quality anomalies, which made them clearly different from normal water and water quality anomalies.
4.2. The advantages of the proposed method
Based on the spectral characteristics of different earth’s surfaces, this study proposed a comprehensive remote sensing index (WAI) for the detection of water quality anomalies. Compared with existing water quality anomaly indices, the WAI has the following three advantages and innovations.
First, WAI can be directly used to detect water quality anomalies in complex geographical environments. The existing remote sensing indices for the detection of water quality anomalies are applicable only to water regions and do not have the ability to distinguish between water quality anomalies and non-water areas. Therefore, if existing water anomaly indices are directly applied to detect water quality anomalies in complex geographical environments, many non-water areas will be misidentified as water quality anomalies. In contrast, WAI integrates the spectral characteristics of water quality anomalies and non-water areas, thus greatly increasing the ability to distinguish between water quality anomalies and non-water areas.
Second, WAI can effectively detect most water quality anomalies, which is more suitable for on-orbit processing. In recent years, index methods (Alharbi Citation2023; Handler et al. Citation2023) and deep learning methods (Li, Martinis, and Wieland Citation2019; Zhang et al. Citation2022) have been widely used to detect different anomaly events. Especially for the deep learning methods, although they are relatively complex, they generally achieve good performances (Zhang and Cheng Citation2020; Zhao, Sui, and Liu Citation2023). For the detection of some water quality anomaly events (e.g. floods), the precision exceeded 90% (Shastry et al. Citation2023), which was higher than the 85% precision of the proposed method. However, both the index methods and deep learning methods are applicable to only one specific water quality anomaly event and lack a comprehensive method for all water quality anomalies. In deep learning methods, if all the water quality anomalies are detected, a more complex model must be constructed. However, the computing resources of satellites are limited, which makes it difficult to run extremely complex models. Moreover, spatial–temporal generalization is still a major problem for deep learning methods (Morais et al. Citation2021; Ye et al. Citation2022), and effectively identifying water quality anomalies at different locations and times remains a great challenge. For index methods, it is also necessary to integrate all existing water quality anomaly indices into a large remote sensing feature system. Accordingly, this inevitably increases the volume and complexity of on-orbit water quality anomaly detection methods, particularly for the detection of sudden water quality anomalies. This is because the detection of sudden water quality anomalies not only needs to determine whether the regions of interest are water quality anomalies in the real-time acquired remote sensing data but also whether they are sudden according to the prior knowledge reflecting their normal earth’s surface states. If there are many remote sensing indices, the prior knowledge will be extremely large and complex, which is a significant challenge for the limited storage of current satellites. In contrast, WAI is a universal remote sensing index that can detect most water quality anomalies. Its complexity is significantly lower than that of deep learning methods. Even in the detection of sudden water quality anomalies, it is only necessary to construct a prior knowledge based on the historical data of WAI, which significantly reduces the volume of prior knowledge. Overall, WAI is more adaptable to the limited storage and computing resources of satellites, which greatly improves the possibility of on-orbit processing for water quality anomaly detection. Furthermore, on-orbit processing is an important basis for real-time remote sensing detection, which can significantly simplify the detection process and improve the response speed (Wei et al. Citation2023). This implies that the proposed method could significantly improve the timeliness of water quality anomaly detection and make it faster than existing methods.
Third, WAI can be applied to multiple satellites, which can be used to form a virtual constellation to observe the earth’s surfaces. Because WAI was constructed using the blue, red, green, NIR, SWIR1, and SWIR2 bands, satellites containing these bands, such as the Sentinel 2 A/B and Landsat series satellites, could calculate WAI. These satellites can be used to form a virtual constellation, thereby reducing the satellite revisit period and improving the time resolution. For example, the temporal resolutions of Landsat 8 and 9 are 16 days, and those of Sentinel 2A/B are 10 days. If these four satellites were combined to observe the earth’s surfaces, the time resolution could be reduced to an average of approximately three days. If other satellites that meet the above conditions are continued to be introduced into the virtual constellation, the temporal resolution may be higher. This will contribute to the high-frequency monitoring of water quality anomalies.
4.3. The limitations and future works of the proposed method
There are also some limitations to the WAI. First, the recommended parameter (i.e. 0.13) for the WAI is based on statistics and it is applicable in most cases. However, with significant changes in the imaging conditions, this parameter may no longer be applicable. If the blue band reflectance of bright white and blue artificial surfaces is less than 0.13, they may be misidentified as water quality anomalies. If the blue band reflectance of water quality anomalies is slightly higher than 0.13, they may be misidentified as non-water areas. Although such situations are rare, they may have affected the detection results to some extent. Second, water quality anomalies are complex and diverse, and some sudden water quality anomalies are similar to long-term stable water quality anomalies or normal water. For example, floods are usually similar to long-term muddy water. It is difficult to effectively identify these sudden water quality anomalies using only WAI, which requires the support of prior knowledge that reflect the normal earth’s surface states. Third, the input data of the proposed index are surface reflectance data, which can not be realized on-orbit process at present. However, on-orbit data processing has become one of the important directions of remote sensing data processing and many methods have been proposed (Huang et al. Citation2023; Li et al. Citation2022; Wang et al. Citation2020). With the technology development, it is possible to generate usable correction data and different remote sensing indices on different satellites. This also means that the proposed index has a great potential for on-orbit application.
Therefore, the future works will mainly focus on three points. The first is how to change the parameter of WAI from a fixed value to an adaptive parameter. Second, it is necessary to construct a prior knowledge base that reflect the normal earth’s surface states according to the historical WAI data to identify sudden water quality anomalies. Third, with the development of on-orbit data processing technology, we will investigate the on-orbit generation of WAI.
5. Conclusion
To realize high-precision and on-orbit detection of water quality anomalies in complex geographical environments using a simple and effective remote sensing index, this study proposed a new index called the WAI for the detection of multitype water quality anomalies. The validation results indicated that the WAI could effectively detect most water quality anomalies with high accuracy and could be directly used to distinguish between non-water areas, normal water areas, and water quality anomalies in different complex geographical environments. Compared to the currently existing remote sensing indices of water quality anomalies, the WAI is a universal remote sensing index that can be used for most water quality anomalies and different complex geographical environments. The WAI not only has the potential for the on-orbit detection of water quality anomalies but also provides real-time data support for the conservation, restoration and sustainable use of inland freshwater ecosystems, which will contribute to the realization of SDG 15.1.
Acknowledgement
The authors appreciate the editors and anonymous reviewers for their valuable suggestions.
Disclosure statement
No potential conflict of interest was reported by the author(s).
Additional information
Funding
References
- Alharbi, B. 2023. “Remote Sensing Techniques for Monitoring Algal Blooms in the Area Between Jeddah and Rabigh on the Red Sea Coast.” Remote Sensing Applications: Society and Environment 30: 100935. https://doi.org/10.1016/j.rsase.2023.100935.
- Caballero, I., A. Román, T. S. Antonio, and G. Navarro. 2022. “Water Quality Monitoring with Sentinel-2 and Landsat-8 Satellites During the 2021 Volcanic Eruption in La Palma (Canary Islands).” Science of The Total Environment 822: 153433. https://doi.org/10.1016/j.scitotenv.2022.153433.
- Chen, J., J. Chen, A. P. Liao, X. Cao, L. J. Chen, X. H. Chen, C. Y. He, et al. 2015. “Global Land Cover Mapping at 30m Resolution: A POK-Based Operational Approach.” ISPRS Journal of Photogrammetry and Remote Sensing 103: 7–27. https://doi.org/10.1016/j.isprsjprs.2014.09.002.
- DeVries, B., C. Q. Huang, J. Armston, W. L. Huang, J. W. Jones, and M. W. Lang. 2020. “Rapid and Robust Monitoring of Flood Events Using Sentinel-1 and Landsat Data on the Google Earth Engine.” Remote Sensing of Environment 240: 111664. https://doi.org/10.1016/j.rse.2020.111664.
- Doña, C., N. B. Chang, V. Caselles, J. M. Sánchez, A. Camacho, J. Delegido, and B. W. Vannah. 2015. “Integrated Satellite Data Fusion and Mining for Monitoring Lake Water Quality Status of the Albufera de Valencia in Spain.” Journal of Environmental Management 151: 416–426. https://doi.org/10.1016/j.jenvman.2014.12.003.
- Gao, B. C. 1996. “NDWI – A Normalized Difference Water Index for Remote Sensing of Vegetation Liquid Water from Space.” Remote Sensing of Environment 58 (3): 257–266. https://doi.org/10.1016/S0034-4257(96)00067-3.
- Gong, P., H. Liu, M. N. Zhang, C. C. Li, J. Wang, H. B. Huang, N. Clinton, et al. 2019. “Stable Classification with Limited Sample: Transferring a 30-m Resolution Sample set Collected in 2015 to Mapping 10-m Resolution Global Land Cover in 2017.” Science Bulletin 64 (6): 370–373. https://doi.org/10.1016/j.scib.2019.03.002.
- Gu, M., J. Fei, and S. Sun. 2020. “Online Anomaly Detection with Sparse Gaussian Processes.” Neurocomputing 403 (1): 383–399. https://doi.org/10.1016/j.neucom.2020.04.077.
- Guo, H. 2020. “Big Earth Data Facilitates Sustainable Development Goals.” Big Earth Data 4 (1): 1–2. https://doi.org/10.1080/20964471.2020.1730568.
- Hagos, G. G., M. M. Assefa, B. Kevin, and G. G. Azage. 2020. “Linear Spectral Unmixing Algorithm for Modelling Suspended Sediment Concentration of Flash Floods, Upper Tekeze River, Ethiopia.” International Journal of Sediment Research 35 (1): 79–90. https://doi.org/10.1016/j.ijsrc.2019.07.007.
- Han, H. B., Y. Li, Z. L. Wang, W. Song, and X. J. Ma. 2022. “Tempo-spatial Distribution and Species Composition of Green Algae Micro-Propagules in the Coastal Waters of Qinhuangdao After Green Tides Bloom in 2020.” Estuarine, Coastal and Shelf Science 276: 108054. https://doi.org/10.1016/j.ecss.2022.108054.
- Handler, A. M., J. E. Compton, R. A. Hill, S. G. Leibowitz, and B. A. Schaeffer. 2023. “Identifying Lakes at Risk of Toxic Cyanobacterial Blooms Using Satellite Imagery and Field Surveys Across the United States.” Science of The Total Environment 869: 161784. https://doi.org/10.1016/j.scitotenv.2023.161784.
- Hu, C., Z. Lee, R. Ma, K. Yu, D. Li, and S. Shang. 2010. “Research Progress on Remote Sensing Monitoring of Phytoplankton in Eutrophic Water.” Journal of Lake Sciences 33 (5): 1299–1314. https://doi.org/10.18307/2021.0502.
- Huang, D., X. Li, X. B. Zheng, W. Wei, Q. Zhang, and F. X. Guo. 2023. “Evaluation and Application of On-Orbit Calibration of the Automated Vicarious Calibration System.” IEEE Transactions on Geoscience and Remote Sensing 61: 1–15. https://doi.org/10.1109/TGRS.2023.3309658.
- Jia, T. X., X. Q. Zhang, and R. C. Dong. 2019. “Long-Term Spatial and Temporal Monitoring of Cyanobacteria Blooms Using MODIS on Google Earth Engine: A Case Study in Taihu Lake.” Remote Sensing 11 (19): 2269. https://doi.org/10.3390/rs11192269.
- Jiang, G. M., Y. Zou, and H. Chen. 2022. “Assessment and Correction of the On-Orbit Radiometric Calibration in FY-3D MERSI-2 Thermal Infrared Channels.” IEEE Transactions on Geoscience and Remote Sensing 60: 1–10. https://doi.org/10.1109/TGRS.2022.3215806.
- Karra, K., C. Kontgis, Z. Statman-Weil, J. C. Mazzariello, M. Mathis, and S. P. Brumby. 2021. “Global Land Use/Land Cover with Sentinel-2 and Deep Learning.” Paper presented at 2021 IEEE International Geoscience and Remote Sensing Symposium IGARSS, Brussels, Belgium.
- Kislik, C., I. Dronova, T. E. Grantham, and M. Kelly. 2022. “Mapping Algal Bloom Dynamics in Small Reservoirs Using Sentinel-2 Imagery in Google Earth Engine.” Ecological Indicators 140: 109041. https://doi.org/10.1016/j.ecolind.2022.109041.
- Li, Z. Q., W. Z. Hou, Z. W. Qiu, B. Y. Ge, Y. Q. Xie, J. Hong, Y. Ma, et al. 2022. “Preliminary On-Orbit Performance Test of the First Polarimetric Synchronization Monitoring Atmospheric Corrector (SMAC) On-Board High-Spatial Resolution Satellite Gao Fen Duo Mo (GFDM).” IEEE Transactions on Geoscience and Remote Sensing 60: 1–14. https://doi.org/10.1109/TGRS.2021.3110320.
- Li, Y., S. Martinis, and M. Wieland. 2019. “Urban Flood Mapping with an Active Self-Learning Convolutional Neural Network Based on TerraSAR-X Intensity and Interferometric Coherence.” ISPRS Journal of Photogrammetry and Remote Sensing 152: 178–191. https://doi.org/10.1016/j.isprsjprs.2019.04.014.
- Li, J. S., S. L. Wang, Y. H. Wu, B. Zhang, X. L. Chen, F. F. Zhang, Q. Shen, et al. 2016. “MODIS Observations of Water Color of the Largest 10 Lakes in China Between 2000 and 2012.” International Journal of Digital Earth 9 (8): 788–805. https://doi.org/10.1080/17538947.2016.1139637.
- Li, J., Q. Yu, Y. Q. Tian, B. L. Becker, P. Siqueira, and N. Torbick. 2018. “Spatio-temporal Variations of CDOM in Shallow Inland Waters from a Semi-Analytical Inversion of Landsat-8.” Remote Sensing of Environment 218: 189–200. https://doi.org/10.1016/j.rse.2018.09.014.
- Lin, Y. Y., E. Hu, C. S. Sun, M. Li, L. Gao, and L. H. Fan. 2018. “Using Fluorescence Index (FI) of Dissolved Organic Matter (DOM) to Identify non-Point Source Pollution: The Difference in FI Between Soil Extracts and Wastewater Reveals the Principle.” Science of The Total Environment 862: 160848. https://doi.org/10.1016/j.scitotenv.2022.160848.
- Liu, Y. 2008. “Remote Sensing Method of Water Pollution and Application on Water Pollution Monitoring in ShaoXing Area.” (Master diss.). Zhejiang University.
- Liu, Y., Y. Dai, G. Liu, J. Yang, L. Tian, and H. Li. 2020. “Distributed Space Remote Sensing and Multi-Satellite Cooperative On-Board Processing.” Paper Presented at 2020 International Conference on Sensing, Measurement & Data Analytics in the era of Artificial Intelligence (ICSMD), Xi’an, People’s Republic of China.
- Lu, Y., X. Li, Q. Tian, G. Zheng, S. Sun, Y. Liu, and Q. Yang. 2013. “Progress in Marine oil Spill Optical Remote Sensing: Detected Targets, Spectral Response Characteristics, and Theories.” Marine Geodesy 36 (3): 334–346. https://doi.org/10.1080/01490419.2013.793633.
- Min, J. E., J. H. Ryu, S. Lee, and S. H. Son. 2012. “Monitoring of Suspended Sediment Variation Using Landsat and MODIS in the Saemangeum Coastal Area of Korea.” Marine Pollution Bulletin 64 (2): 382–390. https://doi.org/10.1016/j.marpolbul.2011.10.025.
- Morais, T. G., R. F. M. Teixeira, M. Figueiredo, and T. Domingos. 2021. “The use of Machine Learning Methods to Estimate Aboveground Biomass of Grasslands: A Review.” Ecological Indicators 130: 108081. https://doi.org/10.1016/j.ecolind.2021.108081.
- NNU_Group. 2011. Environmental Abnormality, Concept & Semantic, OpenGMS, https://geomodeling.njnu.edu.cn/repository/concept/47a991ba-c99b-4c22-bcc5-10c0163e49ca.
- Pahlevan, N., S. K. Chittimalli, S. V. Balasubramanian, and V. Vellucci. 2019. “Sentinel-2/Landsat-8 Product Consistency and Implications for Monitoring Aquatic Systems.” Remote Sensing of Environment 220: 19–29. https://doi.org/10.1016/j.rse.2018.10.027.
- Shao, Z. F., Y. Pan, C. Y. Diao, and J. J. Cai. 2019. “Cloud Detection in Remote Sensing Images Based on Multiscale Features-Convolutional Neural Network.” IEEE Transactions on Geoscience and Remote Sensing 57 (6): 4062–4076. https://doi.org/10.1109/TGRS.2018.2889677.
- Shastry, A., E. Carter, B. Coltin, R. Sleeter, S. McMichael, and J. Eggleston. 2023. “Mapping Floods from Remote Sensing Data and Quantifying the Effects of Surface Obstruction by Clouds and Vegetation.” Remote Sensing of Environment 291: 113556. https://doi.org/10.1016/j.rse.2023.113556.
- Shi, W., and M. H. Wang. 2009. “Green Macroalgae Blooms in the Yellow Sea During the Spring and Summer of 2008.” Journal of Geophysical Research: Oceans 114 (C12): 207–211. https://doi.org/10.1029/2009JC005513.
- Soto, I. M., J. Cannizzaro, F. E. Muller-Karger, C. Hu, J. Wolny, and D. Goldgof. 2015. “Evaluation and Optimization of Remote Sensing Techniques for Detection of Karenia Brevis Blooms on the West Florida Shelf.” Remote Sensing of Environment 170: 239–254. https://doi.org/10.1016/j.rse.2015.09.026.
- Tong, X. H., X. Luo, S. G. Liu, H. Xie, W. Chao, S. Liu, S. J. Liu, et al. 2018. “An Approach for Flood Monitoring by the Combined use of Landsat 8 Optical Imagery and COSMO-SkyMed Radar Imagery.” ISPRS Journal of Photogrammetry and Remote Sensing 136: 144–153. https://doi.org/10.1016/j.isprsjprs.2017.11.006.
- Tulbure, M. G., M. Broich, V. Perin, M. Gaines, J. C. Ju, S. V. Stehman, T. Pavelsky, et al. 2022. “Can we Detect More Ephemeral Floods with Higher Density Harmonized Landsat Sentinel 2 Data Compared to Landsat 8 Alone?” ISPRS Journal of Photogrammetry and Remote Sensing 185: 232–246. https://doi.org/10.1016/j.isprsjprs.2022.01.021.
- Wang, Y. F., Z. N. Gong, Y. Zhang, and S. Su. 2021. “Extraction and Application of Forel -Ule Index Based on Images from Muliple Sensors.” Remote Sensing for Natural Resources 33 (3): 262–271. https://doi.org/10.6046/zrzyyg. 2020324.
- Wang, M., B. B. Guo, X. X. Long, L. Xue, Y. F. Cheng, S. Y. Jin, and X. Zhou. 2020. “On-orbit Geometric Calibration and Accuracy Verification of GF-6 WFV Camera.” Acta Geodaetica et Cartographica Sinica 49 (2): 171–180. https://doi.org/10.11947/j.AGCS.2020.20190265.
- Wang, S. X., Y. L. Liu, Y. Zhou, and C. J. Wei. 2002. “Study on the Method of Establishment of Normal Water Extent Database for Flood Monitoring Using Remote Sensing.” Paper presented at IEEE International Geoscience and Remote Sensing Symposium, Toronto, ON, Canada.
- Wei, H. S., K. Jia, Q. Wang, B. Cao, J. B. Qi, W. Z. Zhao, and J. W. Yang. 2023. “Real-time Remote Sensing Detection Framework of the Earth’s Surface Anomalies Based on a Priori Knowledge Base.” International Journal of Applied Earth Observation and Geoinformation 122: 103429. https://doi.org/10.1016/j.jag.2023.103429.
- Xu, H. Q. 2006. “Modification of Normalised Difference Water Index (NDWI) to Enhance Open Water Features in Remotely Sensed Imagery.” International Journal of Remote Sensing 27: 3025–3033. https://doi.org/10.1080/01431160600589179.
- Xu, J. H., M. J. Deng, and G. D. Liu. 2007. “Application of Remote Sensing Techniques to Water Pollution Monitoring.” Research of Soil and Water Conservation 14 (5): 324–326. 330.
- Yang, X. Q., R. Q. Tong, L. Ma, J. Li, S. Q. Wang, and L. Q. Tian. 2022. “Monitoring Water Color Anomaly of Lakes Based on an Integrated Method Using Landsat-8 OLI Images.” International Journal of Digital Earth 15 (1): 1567–1587. https://doi.org/10.1080/17538947.2022.2122609.
- Yao, Y., Q. Shen, L. Zhu, H. J. Gao, H. Y. Cao, H. Han, J. G. Sun, et al. 2019. “Remote Sensing Identification of Urban Black-Odor Water Bodies in Shenyang City Based on GF-2 Image.” National Remote Sensing Bulletin 23 (2): 230–242. https://doi.org/10.11834/jrs.20197482.
- Ye, W. J., J. M. Lao, Y. J. Liu, C. C. Chang, Z. W. Zhang, H. Li, and H. H. Zhou. 2022. “Pine Pest Detection Using Remote Sensing Satellite Images Combined with a Multi-Scale Attention-UNet Model.” Ecological Informatics 72: 101906. https://doi.org/10.1016/j.ecoinf.2022.101906.
- Yu, S. H., Y. B. Yu, X. J. He, M. Lu, P. Wang, X. D. An, and X. C. Fang. 2020. “On-Board Fast and Intelligent Perception of Ships with the “Jilin-1” Spectrum 01/02 Satellites.” IEEE Access 8: 48005–48014. https://doi.org/10.1109/ACCESS.2020.2979476.
- Zhang, L., and B. Cheng. 2020. “Transferred CNN Based on Tensor for Hyperspectral Anomaly Detection.” IEEE Geoscience and Remote Sensing Letters 17 (12): 2115–2119. https://doi.org/10.1109/LGRS.2019.2962582.
- Zhang, L., B. Cheng, S. Tan, and Y. Wang. 2022. “Fractional Fourier Transform Based Joint Adaptive Subspace Detection for Hyperspectral Anomaly Detection.” IEEE Geoscience and Remote Sensing Letters 19: 1–5. https://doi.org/10.1109/LGRS.2022.3150929.
- Zhang, C., and C. Zhang. 2017. “Monitoring of Water Pollution Based on Water Pollution Difference Index.” Beijing Surveying and Mapping 2: 102–105. https://doi.org/10.19580/j.cnki.1007-3000.2017.02.024.
- Zhao, B. F., H. G. Sui, and J. Y. Liu. 2023. “Siam-DWENet: Flood Inundation Detection for SAR Imagery Using a Cross-Task Transfer Siamese Network.” International Journal of Applied Earth Observation and Geoinformation 116: 103132. https://doi.org/10.1016/j.jag.2022.103132.
- Zhao, J., M. Temimi, H. Ghedira, and C. M. Hu. 2014. “Exploring the Potential of Optical Remote Sensing for oil Spill Detection in Shallow Coastal Waters-a Case Study in the Arabian Gulf.” Optics Express 22 (11): 13755–13772. https://doi.org/10.1364/OE.22.013755.