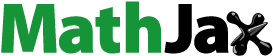
ABSTRACT
Interferometric Synthetic Aperture Radar (InSAR) is capable of detecting crust deformation. However, the accuracy is limited by spatiotemporal changes in the lower troposphere. In this paper, we constructed a periodic zenith total delay negative exponential function (PZTD-NEF) model of atmospheric spatiotemporal variation characteristics based on ERA-5 data to alleviate the temporal oscillation bias introduced by tropospheric delay and improve the accuracy of time series InSAR (TS-InSAR) inversion of surface deformation. We evaluated the model’s performance using the phase standard deviation (STD), atmospheric delay correlation coefficient with topography and the spatial structure function. The results were compared with a linear topography-dependent empirical model, generic atmospheric correction online service (GACOS) and ERA-5 methods. Our method reduces the STD of the phase of 83% of the interferograms by 12.8%. For vertical stratification delay correction, the correlation between the proposed method and the Linear, GACOS, and ERA-5 reached 0.734, 0.708, and 0.729, respectively. We found that accounting for spatiotemporal variation characteristics of tropospheric delay can alleviate the seasonal oscillations of vertical stratification delay and improve the accuracy of the deformation time series solution by 40.04%. We also used the Kunming continuous operation reference station system (KMCORS) to verify the displacement results of our method.
1. Introduction
The development of interferometric synthetic aperture radar (InSAR) technology has enabled efficient wide-area deformation monitoring (such as inverse displacement mapping) of various geophysical processes, such as volcanoes and earthquakes (Cheloni et al. Citation2014; Lanari et al. Citation2010; Wilkinson et al. Citation2012; Yang et al. Citation2017), slow tectonic movements (Cavalié and Jónsson Citation2014; Lanari et al. Citation2007; Walters et al. Citation2013), and urban surface displacement caused by human factors (Casu, Manzo, and Lanari Citation2006; Du et al. Citation2017; Pepe et al. Citation2008). Correspondingly, InSAR technology enables wide-area, round-the-clock Earth observation, unlike traditional geodetic methods like the Global Positioning System (GPS) and leveling measurement, which can only provide observation data at discrete spatial points. Advanced time-series InSAR (TS-InSAR) techniques can accurately extract the temporal evolution of surface deformation with millimeter precision from continuously observed SAR images (Berardino et al. Citation2002; Ferretti, Prati, and Rocca Citation2001). Despite the popular utilization of TS-InSAR in surface displacement monitoring, some limitations exist due to spatiotemporal decorrelation, inaccurate orbits, and tropospheric delays (Hooper et al. Citation2012; Peltzer et al. Citation2001; Wright, Parsons, and Fielding Citation2001; Zebker and Villasenor Citation1992). As control of satellite orbits becomes increasingly more accurate, orbital errors in interferograms are becoming controlled (e.g. TerraSAR-X, Sentinel-1). Furthermore, decorrelation is reducible by adaptive phase optimization (Jiang, Ding, Hanssen, et al. Citation2015; Jiang, Ding, Li, et al. Citation2014). Unfortunately, tropospheric delay still restricts TS-InSAR surface displacement monitoring accuracy. Studies have shown that a 20% change in relative humidity may result in a surface deformation monitoring error of 10–14 cm (Zebker, Rosen, and Hensley Citation1997). In our study, we propose an improved method to mitigate the effects of tropospheric artifacts on TS-InSAR surface displacement measurements.
Tropospheric delay-correction methods can be categorized into three groups: empirical modification, statistical correction, and prediction. Empirical modification aim to mitigate tropospheric effects by modeling the relationship between terrain height and InSAR phase (Bekaert, Hooper, and Wright Citation2015; Liao et al. Citation2013; Remy et al. Citation2003). Empirical approaches may be less effective when the deformation signal is expected to be related to the terrain or when the tropospheric signal changes in the spatial scene (Delacourt, Briole, and Achache Citation1998). Bekaert, Walters, et al. (Citation2015) proposed a power-law method that takes into account the characteristics of atmospheric space variability. Unfortunately, the model requires sounding balloon data for empirical estimation of parameters, which may not match the SAR acquisition time (Zhu, Li, and Tang Citation2017). Statistical methods, such as Stacking (Sandwell and Sichoix Citation2000; Xiao et al. Citation2022), temporal and spatial filtering (Ferretti, Prati, and Rocca Citation2001; Ferretti et al. Citation2011; Hooper Citation2008; Zhao et al. Citation2016), are used to estimate atmospheric delay by applying geostatistical or adjustment algorithms to InSAR phase. Unfortunately, statistical methods are often ineffective because they assume an average random nature of spatiotemporal tropospheric delays, which necessitates a large number of SAR images for obtaining convincing results. Notably, some studies have shown that water vapor content in air varies periodically, so it is essential to consider the impact of large seasonal tropospheric delays in long-term TS-InSAR observations (Doin et al. Citation2009; Dong et al., Citation2019; Tang et al. Citation2018). Prediction methods are the most direct atmospheric correction methods, such as GPS (Onn and Zebker Citation2006; Williams, Bock, and Fang Citation1998; Yu et al. Citation2018), multi-spectral techniques for obtaining water vapor data (Barnhart and Lohman Citation2013; Li et al. Citation2012; Puysségur Citation2007), and numerical weather prediction (NWP) models (Foster et al. Citation2006; Foster et al. Citation2013; Gong et al. Citation2015; Guo et al. Citation2022; Jolivet et al. Citation2011; Jung, Kim, and Park Citation2014; Parker et al. Citation2015; Ulmer and Adam Citation2017). However, the performance of prediction correction depends on the spatiotemporal resolution of the available external data as well as the intrinsic accuracy of the model, so the availability of external information is often the key to the success of these methods.
Current research on InSAR atmospheric delay methods generally estimate vertical stratification and turbulent delays by modeling them. In contrast to the complex stochastic properties of turbulent delays, vertical stratification delays are altitude dependent. Empirical modeling is a simple and effective method that can be used in practical applications to construct phase and elevation without the need for atmospheric parameters as a priori knowledge (Cavalié et al. Citation2007; Wicks et al., Citation2002). Unfortunately, this approach does not account for spatial variation of the tropospheric properties (Bekaert, Hooper, and Wright Citation2015). Another approach is to use meteorological reanalysis data to model atmospheric delays and elevation function methods that consider spatially varying characteristics of the troposphere with global coverage. Considering that these methods allow the estimation of atmospheric variables such as temperature, pressure, and relative humidity at regular time intervals on global or regional spatial grids, which have the advantage of being free to be applied over global coverage (Bekaert, Hooper, and Wright Citation2015; Cao, Jónsson, and Li Citation2021). The two main challenges of atmospheric delay-correction using NWP are: (1) accurately and simultaneously describing the spatiotemporal variation characteristics of the troposphere and (2) solving the inconsistency between SAR spatial resolution and NWP spatial resolution (interpolation accuracy problem). The first challenge requires consideration of the complex seasonal variations of the atmosphere in the vertical profile. According to Emardson et al. (Citation2003), horizontal changes in the troposphere have a correlation time of one day or less, while the average vertical stratification delay of the troposphere has a correlation time ranging from one to three days. Therefore, in the case of strong terrain, the assumption that time is not correlated does not hold. Li et al. (Citation2018) attempted to analyze the vertical profile of the atmosphere with seasonal variation (annual and semi-annual components) and proposed IGGtrop_SH models to describe the temporal and spatial variation of tropospheric delay. The second challenge pertains to the mismatch between the low spatial resolution of the external weather data and high resolution of SAR. The accuracy of current tropospheric delay models is still limited by the relatively low horizontal resolution of the external weather data used for modeling (e.g. Foster et al. Citation2013; Jung, Citation2014). Therefore, reasonable and effective interpolation methods are crucial for improving accuracy.
We propose a new TS-InSAR tropospheric delay-correction method that accounts for the characteristics of spatiotemporal variation. Firstly, we establish a periodic zenith total delay negative exponential function (PZTD-NEF) model based on the ERA5 meteorological reanalysis data covering the study area, we constrain the model reference point at the surface, and use the decreasing elevation factor to describe the atmospheric stratification delay changes. Then, we establish annual and semi-annual period fitting functions for the reference points and elevation decline factor of each time-series grid point respectively, and estimate the model parameters using the least squares method. To further mitigate the residual delay, we treat it as a random signal in time and use temporal filtering to reduce the tropospheric delay more effectively. We tested the model’s performance in the Kunming area of China and successfully detected local ground deformation. The efficiency of atmospheric delay-correction was evaluated using three metrics: phase standard deviation (STD), phase-elevation relationship and the spatial structure function, which evaluates the reduction in the long-wavelength atmospheric effect.
2. Method
2.1. Accounting for characteristics of atmospheric spatiotemporal variation
The SAR signals in the neutral atmosphere is influenced by the propagation medium, which can delay the phase. In general, the atmospheric refractivity N can be used to express the delay change of radar signals through the atmosphere (Bekaert, Hooper, and Wright Citation2015).
(1)
(1) where P represent the atmospheric pressure of the total air, e represent the partial pressure of water vapor, T represent the absolute temperature.
,
,
, which represent the refractive index constants (Smith and Weintraub Citation1953; Thayer Citation1974).
Equation (1) specifically accounts for the hydrostatic and wet delay in the troposphere, while ignoring the impacts of the ionosphere and liquid water. This is because the study uses a C-band sensor with a radar wavelength of approximately 5.6 cm, which is relatively insensitive to ionospheric delays, and liquid water is typically only significant in saturated atmospheres and its presence is often limited and dependent on various factors such as temperature, pressure, and composition (Hanssen Citation2001). For SAR measurements, the atmospheric delay represents the integral of the refractive index of air from the ground point to the satellite in the line-of-sight (LOS) direction:
(2)
(2) where
represent the atmospheric delay of SAR in the LOS direction (one-way),
represent the radar incidence angle,
represent the radar wavelength, and
represent a conversion factor to convert from pseudo-range increase to phase delay (Bekaert, Hooper, and Wright Citation2015).
As ECMWF's latest generation of meteorological reanalysis products, ERA-5 provides detailed atmospheric parameters on a 0.25° spatial grid per hour, surpassing other meteorological reanalysis products (for example, NARR, ERA-I, and sounding balloon data). Considering that the imaging time of Sentinel-1A is 23:05 UTC, we select the ERA-5 atmospheric reanalysis stratification data and the corresponding surface data at 23:00 UTC in the range of 97°–107°E and 21°–30°N from 2017 to 2020 for integral calculation. (a) demonstrates a negative exponential relationship between ZTD and elevation. Therefore, we established a zenith total delay negative exponential function (ZTD-NEF) model to describe this change (Huang, Liu, and Yao Citation2012). (a) shows the atmospheric grid points at (E102, N25) as a function of elevation. We observe that as SAR signals propagate in the troposphere, the delay and elevation tend to decrease this change (Huang, Liu, and Yao Citation2012).
(3)
(3) where
is the formula coefficient, h represents the elevation in km, and
represents the elevation decline factor in cm/km
Figure 1. (a) ERA-5 grid ZTD-NEF fitting. (b) Annual and semi-annual period distribution of surface ZTD. (c) Annual and semi-annual period distribution of the elevation decline factor. (d) Absolute ZTD correlation between NEF-ZTD calculated values and real values. (e) Differential ZTD correlation between NEF-ZTD calculated values and real values.

Therefore, ZTD at different heights at any grid can be formulated as.
(4)
(4)
(5)
(5) where
represents the reference
(here, it is surface
) and
represents the ground elevation. In the time dimension, we use the ERA-5 daily average grid data from 2017 to 2020 to calculate the daily average
and the
time series.
(b,c) shows the atmospheric delay time series variation of the ERA-5 grid point in the study area, and we observe that the model parameters and
exhibit annual cycle changes, so we use the annual and semi-annual period functions for fitting, and determine the model coefficient according to the least squares method (Li et al. Citation2018).
(6)
(6)
(7)
(7) where
represents the model parameters of the
,
represents the model parameters of the
, and
represents a day of the year. The final NEF-ZTD model can be combined as:
(8)
(8) We evaluated the model performance using the ZTD calculated by directly integrating ERA-5 meteorological parameters as true values, and we compared 36 absolute ZTD values and 630 combined differential ZTD values, with the elevation uniformly located in the 11th layer. We plotted the correlation between the results of the two calculations in (e,f). It can be seen that for both absolute ZTD and differential ZTD, the values calculated by our model were in high agreement with the true values with correlation coefficients of 0.9379 and 0.9383, respectively.
Due to the spatial variability of the tropospheric delay, there will be errors in directly using the weighting strategy to calculate ZTD at any elevation position (Cao, Jónsson, and Li Citation2021). Our proposed strategy uses the 30 m resolution unit of Shuttle Radar Topography Mission Digital Elevation Model (SRTM DEM) as the model input. For the atmospheric delay at any time and input location (The red asterisk symbol in ), we calculate the atmospheric delay at the same elevation from a PZTD-NEF model with four surrounding grids (B1, B2, B3 and B4) already established, assuming that the atmosphere varies uniformly and horizontally at the same altitude while considering the spatial location, and then interpolating the output data into the same grid system of the SRTM DEM by using the inverse distance weighting method.
Thus, the atmospheric delay phase at point p with elevation of q can be expressed as:
(9)
(9) where
is the predicted value of atmospheric delay at point p with elevation q,
is the measurement value of known points,
represents the number of sample points around the prediction point participating in the interpolation point (here, n = 4,
represents the distance of the predicted point from the known sample point), and k is the specified power (here,
). shows the atmospheric delay product of the two images obtained using our method.
2.2. Improved TS-InSAR method
To alleviate the seasonal oscillation biases into TSInSAR-measured deformation caused by atmospheric delay, we propose an improved TS-InSAR method that accounts for spatiotemporal variations in the atmosphere. We performed conventional Differential InSAR (D-InSAR) processing based on the GAMMA software, including format conversion, image registration, and generating interferograms. Although multi-looking helps reduce decorrelation noise, studies have indicated that it leads to loss of detail and is associated with misreading of deformed and non-deformed areas, especially in complex terrain (Ferretti et al. Citation2011). Therefore, we use the adaptive coherence estimation algorithm proposed by Jiang (Citation2014). The Minimum Cost Flow (MCF) algorithm is used to unwrap the interferometric phases (Hooper and Zebker Citation2007), obtaining the differential interferometric phase by subtracting the topography phase of the SRTM DEM model at a resolution of 30 m from the interferogram. Then, high coherence points are selected based on the coherence threshold for time series analysis, so the differential interferometric phase of the target point in any interferogram
is composed of:
(10)
(10) where
indicates the residual topographic component caused by imprecise DEM;
and
represent the two SAR image acquisition times A and B, respectively.
represents the deformation component of the radar LOS direction from
to
;
denotes the atmospheric delay component from
to
; and
represents the residual out-of-phase noise.
We build observational models for and
(11)
(11) where
represents the DEM error,
indicates the oblique distance of the radar from the observation,
and
are the spatial and temporal baselines of the interferogram, respectively,
represents the linear deformation velocity, and
represents the nonlinear deformation.
We use the PZTD-NEF model from the previous section to mitigate the tropospheric delay in each interferogram, so we can assume that the residual tropospheric delay is due to turbulent mixing delay. Then, the phase due to linear deformation velocity and DEM error is calculated by using the time-domain phase regression method and step-by-step multiple iterations. The remaining residual phase includes LOS non-deformation, residual atmospheric disturbance delay, and residual noise phase. Since nonlinear deformation has different characteristics than atmosphere and noise in the spatial and temporal domains, the residual atmospheric delay is a complex random high frequency signal in the time dimension, which, however, is correlated in a certain space range and is a smooth low frequency signal. The nonlinear deformation presents a low frequency signal in both time and space dimensions, while noise is an unrelated high frequency signal in both time and space dimensions; therefore, we can separate the nonlinear deformation by spatial and temporal filtering (Hooper et al., Citation2004).
Adding this to the linear deformation velocity yields the final deformation phase, which can be expressed as:
(12)
(12) where
is an
matrix, the primary image position is 1, the secondary image position is –1, and the rest of the positions are 0;
represents the unknown parameter to be solved and denotes the deformation phase matrix of the selected high coherence point.
When and A is a full-rank matrix, equation (12) can be solved using the least squares criterion to yield:
(13)
(13) If A is a rank loss matrix and there is no unique solution to the equation in equation (12), the generalized matrix of A can be obtained by singular value decomposition (SVD), thereby obtaining the least norm solution of the φ. illustrates the flow of data.
2.3. Study area and datasets
The Dian-chi basin of Kunming and its surrounding mountains in southwest China were selected as the experimental area (24°50′44.16″∼25°09′13.08″ N, 102°25′16.16″ ∼102°49′38.75″ E). The studied area is denoted by the green rectangle in (a). From the perspective of geological structure, the study area is located in the largest Quaternary inherited fault basin on the Yunnan Plateau, with large terrain fluctuations. We expect the displacement estimation of the differential interferogram to be affected by the significant vertical stratification delay. Previous reports state that frequent land subsidence in the study area is primarily caused by seasonal rainfall, groundwater extraction, and active tectonics (Li et al. Citation2020). Some sedimentation that develops near the Dian-chi Lake basin may be affected by water vapor, with the turbulent mixing effects in this area expected to be greater than that of stratification. Moreover, the typical highland mountain monsoon climate and complex topographical factors were the prime reasons for selecting this area.
Figure 5. (a) Coverage of the Kunming study area and topographic map. (b) Temporal and spatial baselines distribution of Sentinel-1A.

In this study, datasets from 36 C-band Sentinel-1A satellites (Path 62, Frame 509) were obtained. The time series covers March 15, 2017-February 16, 2020 (1068 days). Sentinel-1A satellite data has the characteristics of high orbital accuracy, short revisit period, high image resolution (range direction of 2.3 m, azimuth direction of 13.9 m) and convenient data acquisition, and has been widely used in the detection and analysis of land deformation, landslides, earthquakes, volcanoes and other disasters by TS-InSAR technology (Bekaert et al. Citation2020; Jelének and Kopaková-Strnadová Citation2016; Wang et al. Citation2020; Xu et al. Citation2016). We reduced the influence of SAR imaging parameter changes on the monitoring results, by using VV polarization with an incidence angle of about 39.3° uniformly (Garestier, Dubois-Fernandez, and Champion Citation2008). We processed the iw2 subset of Path 62-frame 509 datasets. In order to obtain better interferometric coherence, we set the temporal–spatial baseline thresholds to 60 days and 300 meters respectively forming a total of 46 small baseline networks. (b) shows the details, such as the acquisition time, temporal baseline, and spatial baselines of all Sentinel-1A data acquired in this study area. We also acquired Precise Orbit Ephemerides (POE) data, which is utilized to minimize the influence of orbital inaccuracies on subsequent data processing. In addition, we accessed the meteorological reanalysis products dataset through the ECMWF public service to obtain hourly atmospheric physical parameters from 2017 to 2020, including air pressure, temperature, geopotential for tropospheric delay modeling.
3. Results
3.1. Seasonal variation characteristics of TS-InSAR tropospheric delay
illustrates the seasonal fluctuations in the tropospheric delay caused by the elevation decline factor, surface ZTD, and seasonal changes in climate in the vertical profile model. The observed phases of TS-InSAR also have spatially relative properties since we made observations relative to a spatial reference point. To analyze the variation characteristics of TS-InSAR tropospheric delay at different elevations, we selected a reference point with an elevation of 2448 m and used Equation 2 for modeling and then converted the delay units from rad to cm by conversion factor −λ/4π. The tropospheric delay amplitudes of the other four points relative to the reference point were 0.6 cm (), 1.5 cm (
), 3.6 cm (
), and 13.8 cm (
), as shown in . Therefore, the amplitude of the TS-InSAR tropospheric delay depends on the elevation difference between the two points, the larger the difference, the greater the amplitude of the delay. The seasonality of the delay is noticeable; if the elevation difference is small, the seasonal fluctuations in the delay are relatively weak.
Figure 6. InSAR temporal tropospheric delay oscillation trend over time under different elevation differences. Blue dots were derived from the ERA-5 reanalysis data. (e) The position and elevation differences between the four ERA-5 grids and the ERA-5 reference grid.

Estimated average deformation velocity of the Kunming are shown in , using interferograms obtained from both the original and delay-corrected methods. The star mark indicates the reference point. At the spatial scale, we found that the two temporal series results were highly consistent. Especially for artificial construction areas (A1 and A2 region), the deformation velocity estimated by the new and the conventional methods is not significantly over- or underestimated. However, in mountainous areas far from artificial construction, the velocity maps estimated by conventional methods clearly have spatial distribution signals related to elevation, such as the B1 region in (a), mainly due to the effect of vertical stratification delays. In (b), tropospheric delay is mitigated in the velocity map estimated by our method. Most of the regions are stable, with the velocity estimated by TS-InSAR close to 0 mm/a. In we plot the cumulative deformations of the Kunming area at different times, and we see that the subsidence of the Kunming area becomes more and more serious as time advances.
Figure 7. Average deformation velocity map of Kunming area of improved TS-InSAR. (a) No atmospheric correction and (b) new atmospheric correction method. Groups 1 (A1 to D1) and 2 (A2 to D2) show details of the local deformation velocity for selected areas with original and delay-corrected methods, respectively.

Figure 8. Cumulative deformation at different time periods. (a) No atmospheric correction and (b) new atmospheric correction method.

We plot the deformation time series of four points with the same name in , namely P1, P2, P3, and P4. P1 and P2 are in a mountainous area, while P3 and P4 are in dense urban areas. We observed the impact of atmospheric delay in the deformation time series at different elevation. The elevation difference between P3 and P4 relative to the reference point being only 15 and 22 m, respectively, indicating a small influence of vertical stratification delay, we found that the difference in deformation velocity before and after atmospheric delay correction was not significant in the deformation time series. At P1 and P2, there is an elevation drop of approximately 300 m to the reference point. The seasonal oscillations in the deformation time series, calculated using the original method, had a maximum oscillation of ∼4 mm. The deformation oscillation displays a similar trend as the ZTD oscillation, wherein peaks and troughs appear in July–August and January–February each year. The deformation time series results also show that the mountainous area is clearly affected by the tropospheric delay effect ().
Figure 9. Time series of seasonal atmospheric delays and displacements at points P1, P2, P3 and P4 observed in the Sentinel-1A dataset in the Kunming area, and their elevations reported, with the dashed line and triangle representing uncorrected result, solid line and circular representing the corrected result. (a) Mountainous area; (b) Artificial construction area.

3.2. Overall assessment of tropospheric delay improvement
The Linear method estimates vertical stratification delay by establishing a functional relationship between local or global elevation and interferometric phases (), having the apparent drawback of not track such variability tropospheric variation at a relatively flat terrain. We also used the ERA product-based numerical weather prediction (NWP) approach proposed by Jolivet et al. (Citation2014), which uses spline interpolation in the vertical direction instead of constructing a linear or exponential model related to elevation, to compute atmospheric delays at pixel elevations. In addition, we used the generic atmospheric correction online service (GACOS) product developed by Yu et al. (Citation2018), which utilizes an iterative tropospheric decomposition algorithm to interpolate tropospheric delay on the mesh to each InSAR point, rather than directly interpolating weather products in the transverse and vertical directions (Bekaert, Hooper, and Wright Citation2015).
shows an example of an estimated tropospheric delay with different delay-correction methods in the Kunming area. The first interferogram is from the dry winter of 2019 (7 October–24 November), the water vapor content in the air is relatively low, and the coherence of interferogram is also higher, reaching 0.75 overall. Because urban surface displacement is slow, and no major geological disasters (such as earthquakes and landslides) have occurred, we assume there is no significant surface deformation within two months, and that the deformation signal in the interferogram is negligible.
Figure 10. Two cases of tropospheric correction of interferograms: (a) is the first interferogram (October 07 and November 24, 2019), and (b–e) is the interferogram corrected by the four correction methods; (f–j) histogram statistics of the original interferogram and the corrected interferograms for the four correction methods; (k) is the second interferogram (September 23 and October 15, 2017), (l–o) is the same as (b–e), (p–t) is the same as (f–j).

We detected a strong tropospheric signal in the eastern mountains of the original interferogram. Although GACOS and the ERA-5 method almost alleviated the effect of this part of the atmospheric delay, there were still anomalies on the northwest side. The Linear method appeared less successful for mountainous areas, and the atmospheric delay phase remained in the eastern mountainous areas. The results of our proposed method are in close agreement with those obtained from GACOS, Furthermore, the reduction in the STD of the phase before and after tropospheric delay correction demonstrates that the proposed method significantly improves atmospheric delay. On average, the STD decreased from 1.87 rad (0.83 cm) to 1.41 rad (0.62 cm), which is a greater improvement compared with that provided by the Linear method (1.81 rad (0.81 cm)), GACOS (1.49 rad (0.66 cm)), and ERA-5 (1.57 rad (0.70 cm)).
The second interferogram is from 23 September 2017 and 15 October 2017, considering that the rainy season is from July-September and that water vapor in the air leads to complex atmospheric delay changes, we observe that there is a relatively strong stochastic tropospheric signal in the original interferogram. The Linear method assumes a uniform scale factor parameter across the spatial scale, implying a uniform delay for water vapor content's vertical stratification delays. However, if the elevation changes throughout the scene, the wet delay changes with elevation and its rate (relative to the reference altitude delay) increases with the amount of water vapor. Therefore, the interferogram correction effect of wet delay accounting for the main body is not good (the STD of the interferometric phase after correction by the Linear method is reduced by 3.54%). In contrast, the GACOS method uses the exponential function to model the stratified delay, and the ITD model can reconstruct the longwave tropospheric delay related to altitude better, so there is a significant improvement for the mountain area in the red circle (the STD of the interferometric phase after correction by the GACOS method is reduced by 12.63%). Although the ERA-5 method based on a global atmospheric model achieves a larger amount of delay correction, we find that it is ineffective in areas with flat topography, and this may be due to the bilinear interpolation that the method employs in the horizontal space without taking into account the horizontal non-uniformity of the atmospheric delay. The proposed atmospheric delay-correction method was comparable to that of GACOS and ERA-5 method, and the correction amplitude of the interferometric phase STD was nearly identical. Additionally, the proposed method led to a 14.14% reduction in the STD of interferometric phase. Compared with winter, due to severe turbulence and coherent short-scale tropospheric signals in summer, inaccurate signals may be introduced after correction by the proposed method, which may be due to the proposed method assuming that the atmosphere changes uniformly in the horizontal direction at the same altitude, and uses inverse distance weights to interpolate unknown points, but this does not consider small-scale turbulence changes.
We performed a statistical evaluation and comparison of 46 small-baseline interferograms, generated from 36 SAR images acquired between March 2017 and February 2020. In , the STD of the interferograms before and after correction demonstrate that the proposed method (red histogram) generally outperforms other corrections in mitigating spatial variations in tropospheric delays, resulting in lower values. provides a quantitative comparison of the three correction methods, confirming that all correction methods reduce the spatial variation of tropospheric signals in the data. However, the proposed method shows the greatest improvement, and reducing the STD from 1.6 rad (0.71 cm) to 1.27 rad (0.57 cm) on average, with the interferogram improvement rate reaching 83%.
Figure 11. Change of the STD and absolute average mean value of the 46 interferograms before and after using the three correction methods.

Table 1. Quantitative evaluation and comparison of calibration methods based on 46 interferograms.
3.3. Performance comparison to mitigate vertical stratification delay
We used the correlation coefficient between phase and elevation to evaluate the mitigation of vertical stratification delays. Theoretically, the lower the absolute correlation coefficient between the interferometric phase and elevation, the better the mitigation of the vertical stratification component. In , we show an example of four delay-corrected methods to mitigate vertical stratification delays.
Figure 12. Two cases of phase and elevation analysis of interferograms with plotted scatter density distributions. (The red line indicates the linear relationship between the fitted phase and elevation. The first row of interferograms was observed on April 22, 2019, and June 9, 2019, and is dominated by vertical stratification delay, and the second row of interferograms was observed on August 18, 2017 and September 23, 2017, and is dominated by turbulent mixing delay.)

The first interferogram was from 22 April 2019, and 9 June 2019, before any tropospheric correction. The original interferogram had significant topographically dependent phases, mainly caused by vertical stratification delays. On correcting them using Linear, GACOS, ERA-5 and the proposed method, the absolute correlation coefficient between interferometric phase and elevation was reduced from 11.030 rad/km (∼4.905 cm/km) to 1.785 rad/km (∼0.794 cm/km (Linear)), 1.46 rad/km (∼0.649 cm/km (GACOS)), 1.7786 rad/km (∼0.791 cm/km (ERA-5)) and 1.301 rad/km (∼0.579 cm/km (proposed method)). We randomly selected the interferogram phase of 110,000 target points and plotted the correlation between the new method and the corrections of the other three methods, as shown in (a–c). We found good agreement between the corrected phase of the new methods and other three methods which indicates that all four methods can alleviate the vertical stratification delays, and the Linear method is closer to the proposed method, with a correlation coefficient of 0.734. In the second interferogram from 18 August 2017 and 23 September 2017, as shown in (d–f). We found that the topographically relevant phase in the original interferogram was very low, only 0.981 rad/km (∼0.436 cm/km), mainly due to the delay of atmospheric turbulence. Although there was no significant difference in the absolute correlation coefficient between the interferogram phase and elevation corrected by Linear, GACOS, ERA-5 and the method in this paper, which was −0.028 rad/km (∼−0.012 cm/km), 1.192 rad/km (∼0.530 cm/km), 0.907 rad/km (∼0.389 cm/km) and 1.615 rad/km (∼0.719 cm/km). However, combined with (d–f), we find that after the correction of the three methods, there are significant differences in the interferogram phase, the GACOS method shows a negative correlation with the other two methods, and the ERA-5 method has the highest correlation with the proposed method, but the correlation coefficient is only 0.492. This shows that the three methods are not ideal for correcting turbulence delay, and the rough spatiotemporal resolution of ERA-5 may lead to the deterioration of the interferogram phase.
Figure 13. Comparison of the new method with three other methods (a–c from the first interferogram in , d–f from the second interferogram in , the green line indicates the linear fit of different methods).

Unlike linear empirical methods that estimate the tropospheric delay at a global or local scale factor by considering the linear relationship between tropospheric delay and elevation, this approach is easy to implement; however, the scale factor highly depends on the spatial extent of the SAR scene and can sometimes be confused with the linear deformation signal (Cavalié et al. Citation2008). Atmospheric delay-correction methods that consider external meteorological data have the advantage of not requiring a priori information on surface deformation, but they are usually limited by the spatial and temporal resolution of the external data. In this study, we also evaluated the performance of three atmospheric delay correction methods based on ERA-5 reanalysis data (GACOS, ERA-5, and new method). In , we compare the coefficients of correction between the interferometric phase and elevation as a function of STD reduction when correcting a given interferogram using the new method, GACOS and ERA-5. Note that we have masked the coherence points at elevations below 2100 m to avoid confusion due to deformation signals in flat terrain.
Figure 14. Coefficients of correction between interferometric phase and elevation as a function of STD reduction when correcting a given interferogram using the new method, GACOS and ERA-5.

We found that when the absolute value of the correlation coefficient between the interferometric phase and elevation was larger, the three methods significantly improved the tropospheric delay in the interferogram. The new method positively improves the interferograms by 91% (42/46), with a phase STD reduction of 9.5%, in contrast to interferograms improvements of 85% by GACOS and 61% by ERA-5. When the coefficient of correlation was close to zero, the topography did not correlate with the interferometric phase, suggesting significant turbulent delay in the troposphere preventing all of the three methods from performing well in terms of correction. The new method considers the spatial variability in the atmospheric delay by constraining the reference ZTD to the surface and building a negative exponential function related to elevation to characterize the atmospheric vertical profile, rather than estimate the atmospheric delay at pixel elevation based on spline interpolation. In addition, the inverse distance weighted (IDW) method that considers the correlation of atmospheric delay with distance in the horizontal direction performs better than bilinear interpolation. However, these methods still do not consider the no uniformity of water vapor in the atmosphere, which leads to the low improvement by the three methods for turbulent delays.
3.4. Estimation of long-wavelength atmospheric signals
The spatial structure function (SSF) conveys scale- and distance-dependent information about atmospheric effects and is particularly suitable for assessing the mitigation performance of long-wave atmospheric signals. We leverage the fact that the atmospheric signal at a point in space is related to its distance from a reference point and assume that when spatially varying tropospheric delays have a second-order intrinsic smoothness, the spatial autocorrelation can be examined and quantified by using a semi-variogram function:
(14)
(14) where
is the observed phase value at s, and
is the observed value at another distance point. When there is spatial autocorrelation between the object attributes, the variance will gradually level off with distance.
First, we investigated the reduction in long-wavelength atmospheric signals within different spatial scales. For each spatial scale, the spatial scaling factor was set from 1 to 5 with a step size of 0.5, and we randomly selected 10,000 observations from those with coherence greater than 0.8 for variance estimation. (a) shows the maximum variance value estimates for all 46 interferogram-averaged phase values estimated at different spatial scaling factors. We observed that the variance value tended to stabilize with an increase in the spatial scaling factor; however, as the atmospheric delay signal was manifested as a long-wavelength signal, the attenuation of the long-wavelength atmospheric delay signal could not be accurately estimated at overly large scaling factors. After comprehensive consideration, we chose a scale scaling of 2, which is half of the maximum distance (with a window of 22.874 km), as the limit of the maximum distance.
Figure 15. SSF results of the interferogram from 20190422-20190509. (a) Plot of the maximum variance value versus the spatial scale factor. (b-f) semi-variograms of the original interferogram, GACOS correction, ERA-5 correction, Linear correction and new method.

(b–e) shows the performance results of the four atmospheric delay correction methods for a set of interferograms selected for a spatial scale window of 2, where an exponential model is used to describe the discrete changes in the variance values. We found that the variance values of the original interferograms did not stabilize and still showed spatial dependence even at spatial distances over 25 km, which indicates that the original interferograms were affected by long-wavelength atmospheric signals. After atmospheric delay correction, however, the spatial processes were correlated at short distances (around 7 km), and there was no spatial dependence at distances over 7 km, which indicates that the long-wavelength atmospheric signals were mitigated, and the interferograms are mainly presented as small-scale signals, with all four delay-corrected methods achieving consistent results. In addition, the new method was more effective in correcting long-wavelength signals, and the decorrelation distance was reduced from 16.012 km2 to 6.152 km2, instead of 6.412 km2 (Linear), 7.004 km2 (GACOS), and 7.24 km2 (ERA-5).
4. KMCORS verification
To evaluate the performance of the new method, we collected Kunming continuous operation reference station system (KMCORS) data from stations CHG0 and KMCH located in the Kunming city, which together make up the regional GNSS network, covering the period from 16 January 2019 to 30 February 2020. We first use the GAMIT developed by the Massachusetts Institute of Technology in the United States to solve the observational values in the entire time series based on cubic spline interpolation and nearest neighbor linear interpolation and then the three dimensional (3D) KMCORS measurements were projected onto the Sentinel-1A LOS direction as the actual values. The difference between TS-InSAR and KMCORS in solving time series is calculated. shows the time series results of the TS-InSAR at two stations. We found that the deformation time series obtained by the original method differed significantly from the KMCORS results, with root mean square error (RMSE) of 6.58 and 10.09 mm for CHG0 and KMCH station. In contrast, the corrected RMSE of the four tropospheric delay methods was 5.29 and 6.46 mm (Linear), 5.89 and 8.06 mm (GACOS), 5.54 and 6.46 mm (ERA-5), 5.22 and 6.05 mm (proposed method), respectively. This suggests that all three approaches mitigate tropospheric delay. The proposed method performed best, producing the largest improvement of about 40.04%. It is worth noting that the difference between the elevation and reference point of CHG0 and KMCH stations is only 9.4 and 34.5 m, respectively, indicating that the influence of vertical layering delay is small, while turbulence delay can affect both mountains and plains. The proposed method has less deviation from the KMCORS results after correction, which also indicates better performance than other methods in mitigating tropospheric turbulent mixing delay.
Figure 16. Time series analysis of ground displacement (related to 17 SAR acquisitions from January 16, 2019 to February 30, 2020) at two KMCORS stations (KMCH and CHG0). Gray indicates the time series of the KMCORS station, black dots represent the original InSAR time series displacement without tropospheric correction, and green, blue, and red represent the displacement time series corrected by Linear, GACOS, ERA-5 and the proposed method, respectively.

We also compared the displacement difference between KMCH-CHG0. Quantitative comparison of the four correction methods ( and ) confirms that, all four atmospheric delay correction methods are able to improve the accuracy of the deformation solution, and for the comparison of the deformation difference with the two KMCORS points, we find that the new method gives the best correction result with an RMSE of 5.43 mm, instead of 8.94 mm (Original), 7.30 mm (Linear), 6.82 mm (GACOS), and 5.51 mm (ERA-5).
Table 2. Comparison of displacement difference between two positions by GNSS and TS-InSAR.
Table 3. Displacement difference between KMCH-CHG0 counted by different methods, with KMCORS results as the true values.
5. Discussion
As can be seen from , atmospheric delay has distinct annual and semi-annual cycle characteristics, so the assumption that it is not time-dependent does not always hold. The proposed method is different from the statistical or tuning algorithm of conventional TS-InSAR and is based on the ERA-5 atmospheric data. We compared the performance of correcting atmospheric delay with the Linear, GACOS and other numerical weather prediction (NWP) models based on ERA methods. We found that the new method can estimate the tropospheric delay signal related to terrain effectively. However, since the resolution of SAR images reaches the meter level (e.g. Sentinel-1A is 20 m×5 m), it is clear that the low-resolution ERA-5 reanalysis data used in this method means that it may not be possible to capture the turbulent mixing effect at small scales. Our solution considers elevation attribution when solving the atmospheric delay at unknown points and eliminates the atmospheric delay error caused by elevation differences during interpolation. However, turbulent mixing may be more complex in the spatial dimension than previously thought, and stochastic models considering turbulent delays are recommended as a future research topic.
ERA-5 reanalysis data can provide atmospheric parameters with high temporal resolution (1 h intervals) to observe the time-dimensional characteristics of tropospheric delay. Current correction methods for InSAR atmospheric delays are widely focused on spatial dimensional modelling, but there are still very few reports on modelling atmospheric delays taking into account both the temporal and spatial dimensional properties of atmospheric delays. Our proposed method constrains the ZTD model by fitting the periodic changes of variables in the atmospheric model over time rather than a linear interpolation method that assumes linear changes in atmospheric conditions between the two periods of ERA-5 meteorological reanalysis products. Regardless of the advantages of PZTD-NEF method, the rapid changes in atmospheric conditions challenge the application of PZTD-NEF, especially in some disasters such as mudslides and landslides caused by rainfall. Future machine learning models with higher nonlinear fitting performance and higher temporal resolution output meteorological data will help solve this problem.
In deformation monitoring, our approach mitigates the presence of seasonal oscillation bias in the InSAR deformation time series caused by changes in elevation factors, however, this may not work well in areas where there is no significant change in topography. Future development of atmospheric delay models based on PZTD-NEF with a multi-feature factor weighting strategy using deep learning as a framework will help to address this issue. In addition, we introduce adaptive phase optimization proposed by Jiang et al. (Citation2015) instead of traditional multi-looking, which not only improves the spatial density of observation points, helping to observe the spatial distribution of displacement but also avoids misreading of deformed and non-deformed regions compared with conventional TS-InSAR methods. We do this on the basis that the APS estimate is independent of the differential interferograms, which is particularly important for monitoring low-coherence regions. Whether it is conventional SBAS-InSAR or phase-optimized distributed scatterer InSAR (DS-InSAR) technology, the atmospheric delay-correction method proposed in this paper can be supplemented by displacement measurement. Our method provides scientific solutions and references for subsequent studies of such deformations.
6. Conclusion
Here, we propose a tropospheric delay correction method based on ERA-5 data from one of the NWP models to improve the accuracy of surface displacement measurements in the area around Kunming, China, taking into account the spatial and temporal variability of the atmosphere. To evaluate the effectiveness of the new method, we also evaluate the performance of the model in TS-InSAR systematically for the first time by comparing it with Linear, ERA-5 and GACOS methods. After correction by the proposed method, the original STD of 83% of the interferogram decreased by an average of 12%. Our method mitigates the seasonal oscillations of stratification delays and reduces the phase STD by an average of 9.5% in steep mountainous areas with elevations higher than 2100 m. We also evaluated the ability of the new method to mitigate long-wavelength atmospheric signals by means of the spatial structure function, and the corrected decorrelation distance was reduced from 16 km to 6.1 km. By comparing with the time series observation of the KMCORS station, we found that our proposed method alleviates the temporal oscillation introduced by the seasonal atmospheric delay and improves the accuracy of the deformation solution by 40.04%. According to the improved TS-InSAR results, a total of four major ground settlement zones are detected, and groundwater pumping, building loads and engineering construction are the main factors causing large-scale settlement. Additionally, the proposed method is independent of the InSAR dataset and can be integrated into conventional SBAS-InSAR and other TS-InSAR techniques. As a result, reliable monitoring results can be obtained even in complex terrain and vegetated areas.
Our method focuses on seasonal fluctuations in the stratified atmosphere, which is well-corrected for mountainous regions with significant elevation differences. However, the high volatility of water vapor over horizontal space still deserves attention, and further consideration should be given in the future to incorporate the non-uniformity of atmospheric delays into the IDW calculations to further improve the accuracy of the new method. Finally, although we used multiple evaluation criteria to validate the effectiveness of the new model, it was also confirmed that different tropospheric correction techniques present their own limitations. Fortunately, the NWP-based weather modeling approach is independent of interferograms, and in the future, optimal correction methods for combinations of multiple weather models should be investigated to improve the accuracy of atmospheric delay estimates in single interferogram.
Acknowledgements
The authors would like to acknowledge ESA for providing the Copernicus Sentinel-1A dataset and ECMWF for the ERA-5 data. Thanks to Andy Hooper for developing and releasing StaMPS as well as David Bekaert for developing open source TRAIN for atmospheric delay correction. Thanks to Yunnan Geo-Environmental Monitoring Institute for providing GAMMA software for SAR data processing. In addition, we would like to thank the Yunnan Basic Mapping Technology Center for providing the CORS data. We would also like to thank the three peer reviewers and the editor-in-chief for their guidance on the manuscript.
Disclosure statement
No potential conflict of interest was reported by the author(s).
Additional information
Funding
References
- Barnhart, W. D., and R. B. Lohman. 2013. “Characterizing and Estimating Noise in InSAR and InSAR Time Series with MODIS.” Geochemistry, Geophysics, Geosystems 14 (10): 4121–4132. https://doi.org/10.1002/ggge.20258.
- Bekaert, D., A. L. Handwerger, P. Agram, and D. B. Kirschbaum. 2020. “InSAR-based Detection Method for Mapping and Monitoring Slow-Moving Landslides in Remote Regions with Steep and Mountainous Terrain: An Application to Nepal.” Remote Sensing of Environment 249 (1): 111983. https://doi.org/10.1016/j.rse.2020.111983.
- Bekaert, D., A. Hooper, and J. T. Wright. 2015. “A Spatially Variable Power law Tropospheric Correction Technique for InSAR Data.” Journal of Geophysical Research: Solid Earth 120: 1345–1356. https://doi.org/10.1002/2014JB011558.
- Bekaert, D., R. J. Walters, T. J. Wright, A. J. Hooper, and D. J. Parker. 2015. “Statistical Comparison of InSAR Tropospheric Correction Techniques.” Remote Sensing of Environment 170: 40–47. https://doi.org/10.1016/j.rse.2015.08.035.
- Berardino, P., G. Fornaro, R. Lanari, and E. Sansosti. 2002. “A New Algorithm for Surface Deformation Monitoring Based on Small Baseline Differential SAR Interferograms.” IEEE Transactions on Geoscience and Remote Sensing 40 (11): 2375–2383. https://doi.org/10.1109/TGRS.2002.803792.
- Cao, Y., Ś. Jónsson, and Z. W. Li. 2021. “Advanced InSAR Tropospheric Corrections from Global Atmospheric Models That Incorporate Spatial Stochastic Properties of the Troposphere.” Journal of Geophysical Research: Solid Earth 126: 1–20. https://doi.org/10.1029/2020JB020952.
- Casu, F., M. Manzo, and R. Lanari. 2006. “A Quantitative Assessment of the SBAS Algorithm Performance for Surface Deformation Retrieval from DInSAR Data.” Remote Sensing of Environment 102: 195–210. https://doi.org/10.1016/j.rse.2006.01.023.
- Cavalié, O., M. P. Doin, C. Lasserre, and P. Briole. 2007. “Ground Motion Measurement in the Lake Mead Area, Nevada, by Differential Synthetic Aperture Radar Interferometry Time Series Analysis: Probing the Lithosphere Rheological Structure.” Journal of Geophysical Research: Solid Earth 112 (B3): 1–18. https://doi.org/10.1002/2013GL058170.
- Cavalié, O., and S. Jónsson. 2014. “Block-Like Plate Movements in Eastern Anatolia Observed by InSAR.” Geophysical Research Letters 41: 26–31. https://doi.org/10.1002/2013GL058170.
- Cavalié, O., C. Lasserre, M. P. Doin, G. Peltzer, J. Sun, X. Xu, and Z. K. Shen. 2008. “Measurement of Interseismic Strain Across the Haiyuan Fault (Gansu, China), by InSAR.” Earth and Planetary Science Letters 275: 246–257. https://doi.org/10.1016/j.epsl.2008.07.057.
- Cheloni, D., R. Giuliani, E. D'Anastasio, S. Atzori, R. J. Walters, L. Bonci, N. D'Agostino, M. Mattone, S. Calcaterra, and P. Gambino. 2014. “Coseismic and Post-Seismic Slip of the 2009 L'Aquila (Central Italy) MW 6.3 Earthquake and Implications for Seismic Potential Along the Campotosto Fault from Joint Inversion of High-Precision Levelling, InSAR and GPS Data.” Tectonophysics 622: 168–185. https://doi.org/10.1016/j.tecto.2014.03.009.
- Delacourt, C., P. Briole, and J. A. Achache. 1998. “Tropospheric Corrections of SAR Interferograms with Strong Topography. Application to Etna.” Geophysical Research Letters 25: 2849–2852. https://doi.org/10.1029/98GL02112.
- Doin, M., C. Lasserre, G. Peltzer, O. Cavalié, and C. Doubre. 2009. “Corrections of Stratified Tropospheric Delays in SAR Interferometry: Validation with Global Atmospheric Models.” Journal of Applied Geophysics 69: 35–50. https://doi.org/10.1016/j.jappgeo.2009.03.010.
- Dong, J., L. Zhang, M. S. Liao, and J. Y. Gong. 2019. “Improved correction of seasonal tropospheric delay in InSAR observations for landslide deformation monitoring.” Remote Sensing of Environment 233: 111370. https://doi.org/10.1016/j.rse.2019.111370.
- Du, Z., L. Ge, A. H.-M. Ng, X. Li, and L. Li. 2017. “Monitoring of Ground Deformation in Liulin District, China Using InSAR Approaches.” International Journal of Digital Earth 11 (3): 264–283. https://doi.org/10.1080/17538947.2017.1322151.
- Emardson, T. R., M. Simons, and F. H. Webb. 2003. “Neutral atmospheric delay in interferometric synthetic aperture radar applications: Statistical description and mitigation.” Journal of Geophysical Research: Solid Earth 108 (B5). https://doi.org/10.1029/2002JB00178.
- Ferretti, A., A. Fumagalli, F. Novali, C. Prati, F. Rocca, and A. Rucci. 2011. “A New Algorithm for Processing Interferometric Data-Stacks: SqueeSAR.” IEEE Transactions on Geoscience and Remote Sensing 49 (9): 3460–3470. https://doi.org/10.1109/TGRS.2011.2124465.
- Ferretti, A., C. Prati, and F. Rocca. 2001. “Permanent Scatterers in SAR Interferometry.” IEEE Transactions on Geoscience and Remote Sensing 39 (1): 8–20. https://doi.org/10.1109/36.898661.
- Foster, J., B. Brooks, T. Cherubini, C. Shacat, S. Businger, and C. L. Werner. 2006. “Mitigating Atmospheric Noise for InSAR Using a High Resolution Weather Model.” Geophysical Research Letters 33: L16304. https://doi.org/10.1029/2006GL026781.
- Foster, J., B. Brooks, T. Cherubini, C. Shacat, S. Businger, and C. L. Werner. 2013. “The Utility of Atmospheric Analyses for the Mitigation of Artifacts in Insar.” Journal of Geophysical Research: Solid Earth 118: 748–758. https://doi.org/10.1002/jgrb.50093.
- Garestier, F., P. C. Dubois-Fernandez, and I. Champion. 2008. “Forest Height Inversion Using High-Resolution P-Band Pol-InSAR Data.” IEEE Transactions on Geoscience and Remote Sensing 46: 3544–3559. https://doi.org/10.1109/TGRS.2008.922032.
- Gong, W., F. J. Meyer, C.-W. Lee, Z. Lu, and J. Freymueller. 2015. “Measurement and Interpretation of Subtle Deformation Signals at Unimak Island from 2003 to 2010 Using Weather Model-Assisted Time Series InSAR.” Journal of Geophysical Research: Solid Earth 120: 1175–1194. https://doi.org/10.1002/2014JB011384.
- Guo, S., X. Zuo, W. Wu, F. Li, Y. Li, X. Yang, S. Zhu, and Y. Zhao. 2022. “Ground Deformation in Yuxi Basin Based on Atmosphere-Corrected Time-Series InSAR Integrated with the Latest Meteorological Reanalysis Data.” Remote Sensing 14: 5638. https://doi.org/10.3390/rs14225638.
- Hanssen, R. F. 2001. “Remote Sensing and Digital Image Processing, in Radar Interferometry: Data Interpretation and Error Analysis.” Earth and Environmental Science 2: 200–210.
- Hooper, A. 2008. “A Multi-Temporal InSAR Method Incorporating Both Persistent Scatterer and Small Baseline Approaches” Geophysical Research Letters 35 (16). https://doi.org/10.1029/2008GL034654.
- Hooper, A., D. Bekaert, K. Spaans, and M. Arıkan. 2012. “Recent Advances in SAR Interferometry Time Series Analysis for Measuring Crustal Deformation.” Tectonophysics, 514–517, 1–13. https://doi.org/10.1016/j.tecto.2011.10.013.
- Hooper, A., and H. A. Zebker. 2007. “Phase Unwrapping in Three Dimensions with Application to InSAR Time Series.” Journal of the Optical Society of America A 24: 2737–2747. https://doi.org/10.1364/JOSAA.24.002737.
- Hooper, A., H. A. Zebker, P. Segall, and B. Kampes. 2004. “A new method for measuring deformation on volcanoes and other natural terrains using InSAR persistent scatterers.” Geophysical Research Letters 31 (23): 1–5. https://doi.org/10.1029/2004GL021737.
- Huang, L. K., L. Liu, and C. Yao. 2012. “A Zenith Tropospheric Delay Correction Model Based on the Regional CORS Network.” Geodesy and Geodynamics 3: 53–62. https://doi.org/10.3724/SP.J.1246.2012.00053.1.
- Jelének, J., and V. Kopaková-Strnadová. 2016. “Synergic use of Sentinel-1 and Sentinel-2 Data for Automatic Detection of Earthquake-Triggered Landscape Changes: A Case Study of the 2016 Kaikoura Earthquake (Mw 7.8), New Zealand.” Remote Sensing of Environment 265: 112634. https://doi.org/10.1016/j.rse.2021.112634.
- Jiang, M., X. Ding, R. F. Hanssen, R. Malhotra, and L. Chang. 2015. “Fast Statistically Homogeneous Pixel Selection for Covariance Matrix Estimation for Multitemporal InSAR.” IEEE Transactions on Geoscience and Remote Sensing 53: 1213–1224. https://doi.org/10.1109/TGRS.2014.2336237.
- Jiang, M., X. Ding, Z. Li, X. Tian, C. Wang, and W. Zhu. 2014. “InSAR Coherence Estimation for Small Data Sets and Its Impact on Temporal Decorrelation Extraction.” IEEE Transactions on Geoscience and Remote Sensing 52: 6584–6596. https://doi.org/10.1109/TGRS.2014.2298408.
- Jiang, M., X. Ding, Z. Li, X. Tian, C. Wang, and W. Zhu. 2014. “InSAR Coherence Estimation for Small Data Sets and Its Impact on Temporal Decorrelation Extraction.” IEEE Transactions on Geoscience and Remote Sensing 52: 6584–6596. https://10.1109/TGRS.2014.2298408.
- Jolivet, R., P. S. Agram, N. Y. Lin, M. Simons, M. P. Doin, G. Peltzer, and Z. Li. 2014. “Improving InSAR Geodesy Using Global Atmospheric Models.” Journal of Geophysical Research: Solid Earth 119: 2324–2341. https://doi.org/10.1002/2013JB010588.
- Jolivet, R., R. Grandin, C. Lasserre, M.-P. Doin, and G. Peltzer. 2011. “Systematic InSAR Tropospheric Phase Delay Corrections from Global Meteorological Reanalysis Data.” Geophysical Research Letters 38: L17311. https://doi.org/10.1029/2011GL048757.
- Jung, J., D. J. Kim, and S. E. Park. 2014. “Correction of Atmospheric Phase Screen in Time Series InSAR Using WRF Model for Monitoring Volcanic Activities.” IEEE Transactions on Geoscience and Remote Sensing 52 (5): 2678–2689. https://doi.org/10.1109/TGRS.2013.2264532.
- Jung, J., D. J. Kim, and S.E. Park. 2014. “Correction of Atmospheric Phase Screen in Time Series InSAR Using WRF Model for Monitoring Volcanic Activities.” IEEE Transactions on Geoscience and Remote Sensing 52 (5): 2678–2689. https://10.1109/TGRS.2013.2264532.
- Lanari, R., P. Berardino, M. Bonano, F. Casu, A. Manconi, M. Manunta, M. Manzo, et al. 2010. “Surface Displacements Associated with the L'Aquila 2009 Mw 6.3 Earthquake (Central Italy): New Evidence from SBAS-DInSAR Time Series Analysis.” Geophysical Research Letters 37: L20309. https://doi.org/10.1029/2010GL044780.
- Lanari, R., F. Casu, M. Manzo, and P. Lundgren. 2007. “Application of the SBAS-DInSAR Technique to Fault Creep: A Case Study of the Hayward Fault, California.” Remote Sensing of Environment 109 (1): 20–28. https://doi.org/10.1016/j.rse.2006.12.003.
- Li, S., Z. Wang, L. Yuan, X. Li, Y. Huang, and R. Guo. 2020. “Mechanism of Land Subsidence of Plateau Lakeside Kunming City Cluster (China) by MT-InSAR and Leveling Survey.” Journal of Coastal Research 115 (SI): 666–675. https://doi.org/10.2112/JCR-SI115-173.1.
- Li, Z. W., W. B. Xu, G. C. Feng, J. Hu, C. C. Wang, X. L. Ding, and J. J. Zhu. 2012. “Correcting Atmospheric Effects on InSAR with MERIS Water Vapour Data and Elevation-Dependent Interpolation Model.” Geophysical Journal International 189: 898–910. https://doi.org/10.1111/j.1365-246x.2012.05432.x.
- Li, W., Y. Yuan, J. Ou, and Y. He. 2018. “IGGtrop_SH and IGGtrop_rH: Two Improved Empirical Tropospheric Delay Models Based on Vertical Reduction Functions.” IEEE Transactions on Geoscience and Remote Sensing 56 (9): 5276–5288. https://doi.org/10.1109/TGRS.2018.2812850.
- Liao, M., H. Jiang, W. Yong, W. Teng, and Z. Lu. 2013. “Improved Topographic Mapping Through High-Resolution SAR Interferometry with Atmospheric Effect Removal.” ISPRS Journal of Photogrammetry and Remote Sensing 80: 72–79. https://doi.org/10.1016/j.isprsjprs.2013.03.008.
- Onn, F., and H. A. Zebker. 2006. “Correction for Interferometric Synthetic Aperture Radar Atmospheric Phase Artifacts Using Time Series of Zenith wet Delay Observations from a GPS Network.” Journal of Geophysical Research: Solid Earth 111. https://doi.org/10.1029/2005JB004012.
- Parker, A. L., J. Biggs, R. J. Walters, S. K. Ebmeier, T. J. Wright, N. A. Teanby, and L. Zhong. 2015. “Systematic Assessment of Atmospheric Uncertainties for InSAR Data at Volcanic Arcs Using Large-Scale Atmospheric Models: Application to the Cascade Volcanoes, United States.” Remote Sensing of Environment 170: 102–114. https://doi.org/10.1016/j.rse.2015.09.003.
- Peltzer, G., F. Crampé, S. Hensley, and P. Rosen. 2001. “Transient Strain Accumulation and Fault Interaction in the Eastern California Shear Zone.” Geology 29 (11): 975–978. https://doi.org/10.1130/0091-7613(2001)029<0975:TSAAFI>2.0.CO;2.
- Pepe, A., M. Manzo, F. Casu, G. Solaro, and S. Pepe. 2008. “Surface Deformation of Active Volcanic Areas Retrieved with the SBAS-DInSAR Technique: An Overview.” Annals of Geophysics 51 (1): 247–258. https://doi.org/10.4401/ag-3046.
- Puysségur, B. 2007. “Tropospheric Phase Delay in Interferometric Synthetic Aperture Radar Estimated from Meteorological Model and Multispectral Imagery.” Journal of Geophysical Research: Solid Earth 112: B05419. https://doi.org/10.1029/2006JB004352.
- Remy, D., S. Bonvalot, P. Briole, and M. Murakami. 2003. “Accurate Measurements of Tropospheric Effects in Volcanic Areas from SAR Interferometry Data: Application to Sakurajima Volcano (Japan).” Earth and Planetary Science Letters 213 (3-4): 299–310. https://doi.org/10.1016/S0012-821X(03)00331-5.
- Sandwell, D. T., and L. Sichoix. 2000. “Topographic Phase Recovery from Stacked ERS Interferometry and a low-Resolution Digital Elevation Model.” Journal of Geophysical Research: Solid Earth 105: 28211–28222. https://doi.org/10.1029/2000JB900340.
- Smith, E.k., and S. Weintraub. 1953. “The Constants in the Equation for Atmospheric Refractive Index at Radio Frequencies.” Proceedings of the IRE 41: 1035–1037. https://doi.org/10.1109/JRPROC.1953.274297.
- Tang, W., P. Yuan, M. Liao, and T. Balz. 2018. “Investigation of Ground Deformation in Taiyuan Basin, China from 2003 to 2010, with Atmosphere-Corrected Time Series InSAR.” Remote Sensing 10 (9): 1499–1422. https://doi.org/10.3390/rs10091499.
- Thayer, G. 1974. “An Improved Equation for the Radio Refractive Index of Air.” Radio Science 9: 803–807. https://doi.org/10.1029/RS009i010p00803.
- Ulmer, F. G., and N. Adam. 2017. “Characterisation and Improvement of the Structure Function Estimation for Application in PSI.” ISPRS Journal of Photogrammetry and Remote Sensing 128: 40–46. https://doi.org/10.1016/j.isprsjprs.2017.03.005.
- Walters, R. J., J. R. Elliott, Z. Li, and B. Parsons. 2013. “Rapid Strain Accumulation on the Ashkabad Fault (Turkmenistan) from Atmosphere-Corrected InSAR.” Journal of Geophysical Research: Solid Earth 118: 3674–3690. https://doi.org/10.1002/jgrb.50236.
- Wang, L., P. Marzahn, M. Bernier, and R. Ludwig. 2020. “Sentinel-1 InSAR Measurements of Deformation Over Discontinuous Permafrost Terrain, Northern Quebec, Canada.” Remote Sensing of Environment 248: 111965. https://doi.org/10.1016/j.rse.2020.111965.
- Wicks, C.W., D. Dzurisin, S. Ingebritsen, W. Thatcher, Z. Lu, and J. Iverson. 2002. “Magmatic activity beneath the quiescent Three Sisters volcanic center, central Oregon Cascade Range, USA.” Geophysical Research Letters 29 (7): 26-1–26-4. https://10.1029/2001GL014205.
- Wilkinson, M. W., K. J. W. McCaffrey, G. P. Roberts, P. A. Cowie, R. J. Phillips, M. Degasperi, E. Vittori, and A. M. Michetti. 2012. “Distribution and Magnitude of Post-Seismic Deformation of the 2009 L'Aquila Earthquake (M6.3) Surface Rupture Measured Using Repeat Terrestrial Laser Scanning.” Geophysical Journal International 189 (2). https://doi.org/10.1111/j.1365-246X.2012.05418.x.
- Williams, S., Y. Bock, and P. Fang. 1998. “Integrated Satellite Interferometry: Tropospheric Noise, GPS Estimates and Implications for Interferometric Synthetic Aperture Radar Products.” Journal of Geophysical Research: Solid Earth 103: 27051–27067. https://doi.org/10.1029/98JB02794.
- Wright, T., B. Parsons, and E. Fielding. 2001. “Measurement of Interseismic Strain Accumulation Across the North Anatolian Fault by Satellite Radar Interferometry.” Geophysical Research Letters 28: 2117–2120. https://doi.org/10.1029/2000GL012850.
- Xiao, R., C. Yu, Z. Li, M. Jiang, and X. He. 2022. “Insar Stacking with Atmospheric Correction for Rapid Geohazard Detection: Applications to Ground Subsidence and Landslides in China.” International Journal of Applied Earth Observation and Geoinformation 115: 103082. https://doi.org/10.1016/j.jag.2022.103082.
- Xu, W., S. Jónsson, J. Ruch, and Y. Aoki. 2016. “The 2015 Wolf Volcano (Galápagos) Eruption Studied Using Sentinel-1 and ALOS-2 Data.” Geophysical Research Letters 43. https://doi.org/10.1002/2016GL06982.
- Yang, Y., Q. Chen, Q. Xu, Y. Zhang, Q. Yong, and G. Liu. 2017. “Coseismic Surface Deformation of the 2014 Napa Earthquake Mapped by Sentinel-1A SAR and Accuracy Assessment with COSMO-SkyMed and GPS Data as Cross Validation.” International Journal of Digital Earth 10: 1197–1213. https://doi.org/10.1080/17538947.2017.1299806.
- Yu, C., Z. Li, N. T. Penna, and P. Crippa. 2018. “Generic Atmospheric Correction Model for Interferometric Synthetic Aperture Radar Observations.” Journal of Geophysical Research: Solid Earth 123: 9202–9222. https://doi.org/10.1029/2017JB015305.
- Zebker, H. A., P. A. Rosen, and S. Hensley. 1997. “Atmospheric Effects in Interferometric Synthetic Aperture Radar Surface Deformation and Topographic Maps.” Journal of Geophysical Research: Solid Earth 102: 7547–7563. https://doi.org/10.1029/96JB03804.
- Zebker, H. A., and J. Villasenor. 1992. “Decorrelation in Interferometric Radar Echoes.” IEEE Transactions on Geoscience and Remote Sensing 30: 950–959. https://doi.org/10.1109/36.175330.
- Zhao, R., Z. W. Li, G. C. Feng, Q. J. Wang, and J. Hu. 2016. “Monitoring Surface Deformation Over Permafrost with an Improved SBAS-InSAR Algorithm: With Emphasis on Climatic Factors Modeling.” Remote Sensing of Environment 184C: 276–287. https://doi.org/10.1016/j.rse.2016.07.019.
- Zhu, B. Y., J. Li, and W. Tang. 2017. “Correcting InSAR Topographically Correlated Tropospheric Delays Using a Power Law Model Based on ERA-Interim Reanalysis.” Remote Sensing 9 (8): 765. https://doi.org/10.3390/rs9080765.