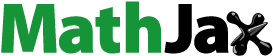
ABSTRACT
Accurate monitoring of the leaf area index (LAI) and aboveground biomass (AGB) using remote sensing at a fine scale is crucial for understanding the spatial heterogeneity of vegetation structure in mountainous ecosystems. Understanding discrepancies in various retrieval strategies considering topographic effects or not is necessary to improve LAI and AGB estimations over mountainous areas. In this study, the performances of the look-up table method (LUT) using radiative transfer model (RTM), machine learning algorithms (MLAs), and hybrid RTM integrating RTM and MLAs based on Landsat surface reflectance (SR) before and after topographic correction were compared and analyzed. The results show that topographic correction improves the accuracies of retrieval methods involving RTM more significantly than the MLAs, meanwhile, it reduces the performance variability of different MLAs. Based on the topographically corrected Landsat SR, the random forest (RF) combined with RTM improves the retrieval accuracy of RTM-based LUT by 7.7% for LAI and 13.8% for AGB, and reduces the simulation error of MLA by 15.1% for LAI and 20.1% for AGB. Compared with available remote sensing products, the hybrid RTM based on Landsat SR with topographic correction has better feasibility to capture LAI and AGB variation at 30 m scale over mountainous areas.
1. Introduction
Grasslands, as a significant component of Earth's terrestrial ecosystems, cover over 30% of the global land area. They hold significant importance in maintaining the regional ecological balance and human survival (Sun et al. Citation2022). The Chinese Loess Plateau is characterized by complex topography, diverse site conditions, intensive ecological restoration, and water and soil conservation, making the restored grassland more susceptible to disturbances in mountainous areas (He et al. Citation2022). It is well known that Leaf Area Index (LAI) and Aboveground Biomass (AGB) serve as pivotal indicators for characterizing the vegetation structure and productivity (Klemas Citation2013; Yu, Evans, and Malleson Citation2018) and for researching ecohydrological processes such as hydrological cycling, carbon cycling, and ecosystem succession (Dong et al. Citation2013; Baez-Gonzalez et al. Citation2005). Therefore, accurate monitoring of the grassland LAI and AGB at a high resolution is crucial for evaluating ecological restoration effects over the rugged mountainous area on the Loess Plateau, which is beneficial for achieving sustainable ecological, economic, and social development in northern China.
Accurate estimation of the LAI and AGB benefits from increasingly abundant remote sensing data sources and a variety of model methods. Among these approaches, statistical model inversion methods are one of the most common and convenient approaches (Dorigo et al. Citation2007; Danner et al. Citation2021). This approach typically relies on establishing statistical regression models based on the relationship between the surface reflectance (SR) or vegetation indices (VIs) and field survey data, and it has advantages such as simplicity, flexibility, and high efficiency (Nguy-Robertson et al. Citation2014). Because machine learning algorithms (MLAs) are capable of constructing efficient predictive models by autonomously learning features and patterns from a large amount of data, they have been widely used in the retrieval of biophysical parameters, providing significant opportunities for remote sensing-based applications (Verrelst et al. Citation2015; Maxwell, Warner, and Fang Citation2018; Weiss, Jacob, and Duveiller Citation2020). MLAs can learn complex relationship features from a large amount of training data to estimate the variables within relevant spectral features (Wang, Xiao, and Liu Citation2017). Therefore, MLAs have a great potential for handling large spatial datasets. Nevertheless, establishing a reliable MLA model necessitates a substantial volume of training data, and relying solely on field sampling significantly hinders its application (Kwon et al. Citation2017). Field sampling, which is often laborious and time-consuming, is typically constrained by limited time and spatial scope, thereby compromising the timeliness and comprehensiveness of sampled areas and greatly constraining the powerful computational capability and generalization ability of the MLAs, thereby affecting their predictive performance (Ma and Liang Citation2022).
By coupling the PROSPECT (Jacquemoud et al. Citation1995) and SAIL (Verhoef Citation1984) models, a canopy-level radiative transfer model (RTM), known as the PROSAIL model, was generated to simulate the vegetation spectral reflectance by inputting vegetation structure parameters such as the LAI, leaf chlorophyll content, and dry matter weight (Quan et al. Citation2017). Over the past three decades, LAI and AGB estimations have been continuously and successfully conducted for various ecosystems (such as forests (Sinha et al. Citation2020), agriculture (Wang et al. Citation2022), and grasslands (He et al. Citation2019)) based on radiation transfer models (Yin et al. Citation2015; Fang et al. Citation2019). However, most physical model-based vegetation parameter inversions are conducted through the construction of a look-up table (LUT) (Ganguly et al. Citation2012). In the inversion process, all combinations of the reflectance in the LUT are iteratively cycled using the cost function as a medium to derive the parameter set corresponding to the pixel being processed. However, each pixel requires iterative cycling using the data in the LUT, which slows down the calculation speed and reduces the efficiency (Houborg and McCabe Citation2018).
In comparison to the RTM and MLAs, it has been reported that hybrid RTM methods that combine the RTM and MLAs have superior efficiencies in the inversion of vegetation biophysical parameters. In the strategy of the hybrid RTM, a massive training dataset is first constructed based on physical constraints and prior knowledge, and then, MLAs are used to establish the nonlinear relationships between the SR and vegetation structural parameters based on the training dataset (Verrelst et al. Citation2015). The processing of large-scale remote sensing scenes can be completed in a matter of minutes or seconds, greatly enhancing the processing efficiency. However, in mountainous areas, the shading and multiple scattering caused by terrain undulation lead to serious spectral distortion of pixel-scale satellite observations, and the finer the spatial resolution of the remote sensing images is, the greater the topographic effect is (Roupioz et al. Citation2014; Wilson and Jetz Citation2016; Yu et al. Citation2020). Therefore, topographic effects must not be ignored in retrieving accurate vegetation structural parameters under complex terrain conditions, especially for sunny slopes and shaded slopes (Pasolli et al. Citation2015), when using high-resolution and medium-resolution remote sensing imagery, e.g. Landsat, Sentinel, and Worldview imagery.
To mitigate this challenge, in recent years, researchers have developed high-dimensional RTMs that consider the topographic effects and have conducted studies on vegetation parameter inversion over mountainous areas (Jin et al. Citation2017; Qi et al. Citation2022; Cheng et al. Citation2022). However, due to the rare availability of complicated parameters in mountainous areas and the low computational efficiency, the widespread and practical application of high-dimensional RTMs remains challenging. Another more practical method includes two steps (Wu, Zhao, and Qin Citation2022; Xie et al. Citation2021). 1) The SR on a hilly slope is converted into the SR on a flat surface using topographic correction methods (e.g. C and SCS + C). 2) The vegetation structural parameters are estimated using radiative transfer models suitable for flat areas. However, few existing studies have focused on estimating vegetation structural parameters in mountainous areas using high-resolution satellite imagery, and there is a lack of a deeper understanding of the discrepancies between various retrieval algorithms based on SR with and without topographic correction.
Therefore, the aims of this study were 1) to assess the impacts of topographic effects on the accuracy of LAI and AGB estimates; 2) to compare and analyze the performances of different retrieval strategies based on the corrected SR; and 3) to develop an optimal vegetation parameter retrieval method for mountainous areas.
2. Materials
2.1. Study area
The study area is located in the typical hilly and gully region on the Loess Plateau, which is located in the Yan'an and Yulin districts, Shaanxi Province, China (32°03'–33°10'N, 108°25'–109°30'E) (). The climate in this area is a typical temperate semi-arid climate with annual an average temperature of 9.5–11 °C and an annual average precipitation of 300–550 mm. Due to historically intensive human activities in these mountainous areas, which have resulted in serious vegetation degradation, soil and water loss, and environmental deterioration, the Chinese government has implemented national ecological restoration projects, including converting cropland into forest and grassland, afforestation, and grazing prohibition, since 1999 (Jia et al. Citation2014). From 1990 to 2020, the proportion of grassland area on the Loess Plateau increased from 30.6 to 45.7%. Around 2000, the area of farmland converted to forests and grassland increased from 1.51 to 12.72%. The grassland area of the study area is 14,441.71 km2, accounting for 76.63% (Wang et al. Citation2023). The structure and function of the herbaceous vegetation have been significantly improved over the last three decades. Currently, although grassland is the dominant vegetation type in this semi-arid zone, the mountainous grassland is characterized by fragmented landscapes and high spatial heterogeneity as a result of the complex terrain and human activities. The dominant herbaceous species are primarily Artemisia capillaris, Artemisia lavandulaefolia, and Lespedeza bicolor.
Figure 1. Location of the study area and distribution of sampling points. (a) Geographic location of the Loess Plateau and the study area; (b) Location distribution of 33 sampling points; (c) Leaf area index and leaf chlorophyll content were measured at each sampling site; (d) Histograms of the distribution of the LAI and AGB values.

2.2. Data
2.2.1. Field observations
The field survey for this study was conducted from July 20, 2022, to August 1, 2022, coinciding with the peak growth phase of the herbaceous vegetation. A 30 m × 30 m plot boundary was constructed manually, and a Trimble Real-time Kinematic (RTK) global positioning system (GPS) was used to determine the coordinates of the four corners of the plot to match the Landsat pixels. Three small plots (1 m × 1 m) with different degrees of coverage were further selected within the 30 m × 30 m plot. All leaves and branches of plants in each small plot were harvested manually, and the total area of all leaves was measured via scanning. The average of the LAI values of the three small plots represents the plot-level LAI at the 30 m scale. The AGB of each small plot was obtained by determining the dry matter content of the harvested leaves and branches after 24 h of drying at 105 °C in an oven. The average AGB density of the three small plots represents the plot-level AGB density at the 30 m scale. In addition, the plot-level leaf chlorophyll content was calculated by averaging 20 in-situ measurements, which were randomly distributed in the 30 m × 30 m plot using SPAD-502PLUS and the calibration method developed by Markwell et al. (Markwell, Osterman, and Mitchell Citation1995). The plot-level leaf angle was determined using a traditional angle measuring instrument. Finally, a total of 33 plots (30 m × 30 m) were investigated during the field survey.
2.2.2. Landsat8 OLI surface reflectance
Landsat-8 operational land imager (OLI) SR data (L1T) was obtained from the Google Earth Engine (GEE) cloud platform. Detailed information is shown in .
Table 1. The detailed information of Landsat 8 OLI.
2.2.3. DEM data
The digital elevation model (DEM) employed for the topographic correction uses the global high-resolution dataset AW3D30 DEM (https://www.eorc.jaxa.jp/ALOS/en/aw3d30/data/index.htm) compiled and released by the Japanese Earth Observation Satellite (Advanced Land Observing Satellite, ALOS) for the period from 2006 to 2011. With a horizontal resolution of 30 m and a vertical accuracy of 5 m, it provides coverage for 99% of the Earth's landmasses and islands. This dataset possesses characteristics such as high precision, high resolution, global coverage, and consistency. The version we used was (AW3D30_v3.2), which was released in February 2022.
2.2.4. Land cover product
The land cover product dataset used in this study was the first annual China Land Cover Dataset (CLCD) derived from Landsat on the GEE platform (https://zenodo.org/record/4417810). It was produced using a post-processing method that combines spatiotemporal filtering and logical reasoning techniques, and has a reliable overall classification overall accuracy of 79.30% in China (Yang and Huang Citation2021). The CLCD classifies the land into nine categories: cropland, forest, shrub, grassland, barren, impervious, water, snow/ice, and wetland, in China. Because this study focused on grasslands, all land cover types except grasslands were masked.
2.2.5. GLASS LAI, MODIS LAI and CCI AGB
The Global Land Surface Satellite (GLASS) LAI product was generated by a Bidirectional Long Short-Term Memory (Bi-LSTM) Temporal Recurrent Neural Network Model and moderate-resolution imaging spectroradiometer (MODIS) reflectance data (http://www.glass.umd.edu/Download.html). The temporal resolution of GLASS LAI is 8 days, and the spatial resolution is 250 m. The MOD15A2H LAI product is an 8-day composite dataset at 500 m resolution (https://lpdaac.usgs.gov/products/mod15a2hv006/). To match the acquisition time of the Landsat imagery, epoch of the 201st day (July 20th) of the year in 2022 was selected for LAI estimates comparison.
European Space Agency's Climate Change Initiative (CCI) Biomass project provides global maps of AGB at 100m scale annually scales for five epochs (2010, 2017, 2018, 2019 and 2020), which is produced by Envisat’s ASAR and JAXA’s Advanced Land Observing Satellite (ALOS-1 and ALOS-2), along with earth observation sources (https://climate.esa.int/en/odp/#/project/biomass). To match the acquisition time of the Landsat imagery, the year of 2020 was selected for AGB estimates comparison.
3. Methodology
3.1. Overall methodology
The experimental workflow of this study is illustrated in . First, Sun-Canopy-Sensor + C-correction (SCS + C) topographic correction was applied to Landsat 8 OLI imagery using AW3D30 DEM data. Subsequently, RTM-based LUT, hybrid RTM methods, and MLAs were used to conduct LAI and AGB inversion. The differences between various inversion strategies with and without topographic correction were compared and analyzed. Finally, diverse validation methods were used to assess the accuracy and reliability of the inversion results.
3.2. SCS + C algorithm
Before conducting LAI and AGB inversions, it is crucial to perform effective topographic correction on Landsat 8 OLI imagery. Currently, various topographic correction methods have been developed, including cosine correction, Minnaert correction, and SCS + C correction. Among them, the SCS + C topographic correction algorithm has been demonstrated to effectively mitigate the impact of the uneven reflectance caused by terrain factors in areas with topographic variations on remote sensing imagery (Soenen, Peddle, and Coburn Citation2005). It leverages the slope, aspect, solar zenith angle, and azimuth angle to describe the terrain effects. In this study, the AW3D30 DEM was used to extract the necessary terrain parameters for the SCS + C topographic correction. The mathematical equation of the algorithm is as follows:
(1)
(1) where
is the reflectance with topographic correction,
is the reflectance without topographic correction,
is the topographic slope,
is the solar zenith angle,
is the local incidence angle, and C is the ratio of the regression coefficients between illumination and reflectance in different bands.
3.3. Setting PROSAIL model
Since the field measurement area (30 m × 30 m) does not completely match the pixel size (30 m) of the Landsat8 OLI imagery, the reflectance data of each plot was obtained by calculating the weighted average based on the pixel area ratio of the plots (Shi et al. Citation2021).
In this study, the PROSAIL model was used to construct a dataset of the canopy reflectance-LAI and AGB. The PROSAIL model is developed by coupling the leaf optical properties model PROSPECT and the canopy reflectance model SAIL for arbitrarily inclined leaves (Baret et al. Citation1992; Ding et al. Citation2016; Wang et al. Citation2022). The PROSPECT model is mainly used to describe the physical and chemical characteristics of the leaves. This model includes parameters such as the leaf chlorophyll content, leaf dry matter content, and leaf equivalent water thickness. It can be calculated as follows:
(2)
(2) where
is the wavelength,
is the leaf reflectance at
,
is the leaf transmittance at λ.
is the leaf chlorophyll content,
is the leaf dry matter content,
is the brown pigment fraction,
is the leaf equivalent water thickness,
is the leaf carotenoid content,
is the leaf anthocyanin content, and N is the leaf structure index.
The SAIL model is primarily used to describe the reflectance and scattering characteristics of the entire vegetation canopy, including parameters such as the LAI and average leaf angle. It can be calculated as follows:
(3)
(3) where
is the wavelength,
is the solar zenith angle,
is the observation zenith angle,
is the sun-sensor azimuth angle, LAI is the leaf area index, ALA is the average leaf angle,
and
are simulated using the PROSPECT model,
is the soil (background) reflectance at
, and
is the proportion of scattered light from the sky to the total incident light.
The determination of the parameter values in the PROSAIL model is essential for the physical model inversion of the grassland LAI and AGB. Based on field survey data and prior knowledge, taking into account factors such as computational resources and time costs, we conducted multiple experiments to ultimately determine the values and ranges of input parameters listed in , totaling 127,260 parameter combinations.
Table 2. Input parameter values or ranges and sampling step size for the PROSAIL model.
3.4. RTM-based LUT retrieval
The RTM-based LUT method has been widely used in vegetation remote sensing. This method is based on a forward model. In the experiment, LAI and Cm are used as inputs for the PROSAIL model, and the AGB can be represented as the product of the LAI and Cm (Jin et al. Citation2019). To construct an RTM-based LUT, 127,260 parameter combinations drive the PROSAIL model to obtain a continuous simulated spectrum from 400 to 2500 nm. By matching the radiance spectral responses (RSR) with the band settings of the Landsat8 OLI, it transforms the continuous spectrum to Landsat 8 OLI sensor-corresponding band reflectance (Soudani et al. Citation2006), a canopy reflectance-LAI and AGB LUT with a size of 127,260, has been established.
Through iterative cycles with a selected cost function, the LAI and AGB corresponding to the combination of the reflectance that the minimum cost function was chosen as the optimal inversion value for that pixel. It can be calculated as follows:
(4)
(4) where n is the number of bands,
is the band reflectance of the Landsat8 OLI, and
is the simulated reflectance of the PROSAIL model.
However, due to the ill-posedness and instability of the LUT inversion method, the LAI and AGB obtained from the minimum cost function do not necessarily represent the optimal solution. To address this issue, we used a multi-solution averaging approach, and the average values of the top 50 correspondences of the LAI and AGB with the minimum cost function were considered to be the best estimates.
3.5. Machine learning algorithms
Regarding this article, it is advisable to consider using various MLAs. Artificial neural network (ANN) method is a widely used MLA. It consists of a group of interconnected neurons, which process information in a grouped manner and store it between nodes or neurons with serial connections. Each neuron is connected to other neurons in this context, and training and learning are performed by modifying the weights of their connections (Baret, Clevers, and Steven Citation1995; Fang and Liang Citation2003). Support vector regression (SVR) is a regression algorithm based on support vector machines. It transforms the data into a higher dimensional space using a kernel function and finds an optimal hyperplane that can nonlinearly map the input variables, thereby solving nonlinear problems (Cortes and Vapnik Citation1995; Mountrakis, Im, and Ogole Citation2011). Random forest (RF) regression algorithm, introduced by Breiman in 2001, is an ensemble method that uses multiple decision trees as the foundation and employs averaging predictions to achieve improved performance. This algorithm has the advantage of feature importance assessment, thereby facilitating an in-depth understanding of the feature selection process (Breiman Citation2001; Wu et al. Citation2021). Extreme gradient boosting (XGB) is a supervised gradient boosting algorithm that forms new decision trees through iterative processes to fit the residuals of the previously predicted and actual values of the training samples. It combines the advantages of bagging ensemble learning (Chen and Guestrin Citation2016; Jahromi et al. Citation2023). Least absolute shrinkage and selection operator regression (LASSO) is a method based on ordinary least squares with regularization in linear regression analysis. It uses L1 regularization to penalize the complexity of the model to optimize the model (Tibshirani Citation1996). Each algorithm possesses its own merits and limitations, necessitating the consideration of factors such as the accuracy, computation time, and interpretability when selecting an algorithm. All MLAs use Landsat 8 SR (blue band, red band, NIR band, SWIR1 band, and SWIR2 band) corresponding to field survey points as feature input. with a sample size of 33. And use 3-fold cross validation for model training and testing.
All MLAs involved in this study were implemented using the Scikit-learn (sklearn) library in Python. All inversion methods involving MLA use Gridsearchcv and 5-fold cross validation to determine hyperparameters (), apart from the parameters listed in the , all other settings are set to their default values.
Table 3. Parameter settings to determine the optimal hyperparameters for involving MLAs.
3.6. Hybrid RTM retrieval methods
The hybrid RTM combines the PROSAIL model with five MLAs and uses the individual strengths of each MLA to create a more useful model. In this study, hybrid RTM methods that couple PROSAIL with five MLAs were used to estimate the grassland LAI and AGB. Specifically, the PROSAIL model was used to simulate the reflectance data and biophysical parameters, and MLAs were used to establish the model of the relationship between the reflectance and grassland LAI and AGB. Then, the LAI and AGB of the study area were estimated using the trained machine learning model. Hybrid RTM retrieval methods use the features simulated by the PROSAIL model for Landsat 8, including the blue band, red band, NIR band, SWIR1 band, and SWIR2 band, with a sample size of 127,260. To validate the quality of the dataset and the performance of the MLAs, the dataset simulated using the PROSAIL model was split into a training set and a testing set at a ratio of 7:3.
3.7. Evaluation strategy
To compare the performance of the RTM-based LUT and the hybrid RTM, 33 field observations were used to verify the models. The accuracy and reliability of inverting the LAI and AGB with and without topographic correction using the RTM-based LUT and hybrid RTM were compared.
To evaluate the performance of the MLAs and hybrid RTM based on different datasets, 33 field observations were used as the input of the MLAs, and three-fold cross-validation was used for the evaluation. The hybrid RTM was trained using the simulated data from the PROSAIL model and was evaluated using the validation set of the MLAs through three-fold validation, and the average was used as the final evaluation result. The performances of the MLAs and hybrid RTM for inverting the LAI and AGB with and without topographic correction were assessed.
To comprehensively evaluate the reliability and accuracy of the inversion of each method, the coefficient of determination (R²) and unbiased root mean square error (ubRMSE) were selected to evaluate the performances of the models. The R² value can be calculated as follows:
(5)
(5) During the inversion process, the RMSE cannot reflect the actual situation, but there may be some biases. To solve this problem, the bias can be used to eliminate the bias of the RMSE, and the ultimate result is the ubRMSE. It can be calculated as follows:
(6)
(6) where
is the observed value of the LAI and AGB,
is the predicted value of the LAI and AGB,
is the arithmetic mean of the observed values of the LAI and AGB, and N is the number of verification samples.
Generally, a higher R² and lower ubRMSE indicate that the model has a better accuracy and reliability.
4. Results
4.1. Topographic correction results
Topography plays an important role in LAI and AGB retrievals. presents a visual comparison of the results of employing the SCS + C algorithm in partial regions within the study area with and without topographic correction, presented in the RGB color composite of the SWIR1-NIR-Red. In this process, the differences between the vegetation and non-vegetation can be made more pronounced.
The visual assessment reveals that the original image exhibits an uneven surface with significant differences between the shady and sunny slopes, displaying pronounced topographical effects. These factors subsequently affect the LAI and AGB retrievals on rugged surfaces. Compared with the original images (a–d), the application of the SCS + C topographic correction (e–h) to the Landsat8 SR data is visually successful. The SCS + C topographic correction algorithm can reduce most of the radiometric difference between the slopes facing the sun and those facing away from the sun, which allows for the attainment of the equivalent flat SR.
illustrates the changes in spectral values of selected pixels (sunny slope and shaded slope) at different slopes before and after topographic correction. After topographic correction, the spectral reflectance values on different slopes shows a decrease on the sunny slope and an increase on the shaded slope. Moreover, as the slope increases, the effect of topographic correction becomes more pronounced.
Figure 4. The changes in spectral values of selected pixels (sunny slope and shaded slope) at different slopes before and after topographic correction. (The circumference represents the aspect, the radius represents the slope, and the red and black dots represent the slope and aspect of the selected pixels.)

By extracting the reflectance values of Landsat8 OLI imagery (Green band, Blue band, Red band, NIR band, SWIR1 band, and SWIR2 band) from the field survey points before and after topographic correction, the correlation between the Landsat8 SR and LAI, as well as the AGB, was evaluated ().
Figure 5. Heatmaps of the correlation between the LAI and AGB before (a) and after (b) topographic correction for different spectral bands.

After topographic correction, the positive correlation between the NIR band and LAI is enhanced, increasing from 0.22 to 0.32. The negative correlation between the NIR band and AGB is weakened, changing from −0.12 to −0.011. The changes in correlation between other bands and LAI and AGB are relatively small, staying within 0.04. This is because the NIR band exhibits a strong sensitivity to the LAI, as well as the AGB, and can capture the structural and physiological information about the vegetation. It is important to note that correlation can only reflect the strength and direction of linear relationships between variables, and cannot provide detailed information about causal relationships or other non-linear relationships. Therefore, in order to gain a more comprehensive understanding of these changes, the Landsat8 SR data with and without topographic correction were used to conduct different retrieval strategies to analyze the topographic effects on the LAI and AGB retrievals.
4.2. Comparison of RTM-based LUT and hybrid RTM retrieval schemes
shows the accuracies of the retrieved LAI and AGB with and without topographic correction compared with the field observations. The ubRMSE values of the LAI (0.39 m2/m2) and AGB (79.08 g/m2) estimated using the RTM-based LUT based on the SR without topographic correction decreased to 0.26 m2/m2 and 60.67 g/m2 after topographic correction, indicating that the topographic correction was able to improve the estimation accuracy of the RTM-based LUT by 33.9% for the LAI and by 23.3% for the AGB. In addition, it can be seen that the topographic correction was also able to improve the accuracies of the LAI and AGB estimations using the hybrid RTM. Based on the Landsat SR after topographic correction, the RF integrating the RTM emerged as the most accurate method for retrieving the LAI and AGB values (LAI: R2 of 0.69 and ubRMSE of 0.24 m2/m2; AGB: R2 of 0.80 and ubRMSE of 52.33 g/m2), surpassing the performance of the RTM-based LUT (decrease in ubRMSE of 7.7% for LAI and of 13.8% for AGB).
4.3. Comparison of machine learning and hybrid RTM retrieval schemes
presents the evaluation indicators of the accuracies of the LAI and AGB retrievals conducted using the MLAs and hybrid RTM methods and compares the results obtained with and without topographic correction. The ubRMSE values of the LAI (0.27 m2/m2) and AGB (61.89 g/m2) for the MLAs estimations with the best performance among the five MLAs using the Landsat SR after topographic correction are lower than those of the MLA estimations obtained using the Landsat SR without topographic correction (0.28 m2/m2 for LAI and 64.15 g/m2 for AGB), indicating that the topographic correction was able to improve the estimation accuracy of the MLA by 3.6% for the LAI and by 3.5% for the AGB.
Figure 7. Three-fold cross-validation of the MLAs and hybrid RTM inversions of the LAI (a-b) and AGB (c-d) using the Landsat SR before and after topographic correction.

The ubRMSE values of the LAI (0.23 m2/m2) and AGB (49.08 g/m2) for the hybrid RTM estimations show that it had the best performance among the five hybrid RTM methods when using the Landsat SR after topographic correction. They are notably lower than those of the best hybrid RTM estimations achieved without topographic correction (0.26 m2/m2 for LAI and 61.89 g/m2 for AGB), indicating that the topographic correction was able to improve the estimation accuracy of the MLA by 11.5% for the LAI and by 20.7% for the AGB.
Based on the Landsat SR after topographic correction, the RF integrated with the RTM was the most accurate method for retrieving the LAI and AGB. For the LAI, it achieved an impressive R2 of 0.71 and a low ubRMSE of 0.23 m2/m2. For the AGB, it excelled, with an R2 of 0.80 and a minimal ubRMSE of 49.48 g/m2. It significantly outperformed the MLA with the best performance (LASSO) for the LAI retrieval, with an ubRMSE reduction of 15.1%, and it exceeded that of the MLA with the best performance (RF) for the AGB retrieval, with an ubRMSE reduction of 20.1%.
Additionally, after topographic correction, among various LAI inversion strategies, the ubRMSE of decreased by 0.33 m2/m2, the average ubRMSE of the hybrid RTM decreased by 0.25 m2/m2, both greater than the ubRMSE of MLAs (average decrease of 0.11 m2/m2). Among various AGB inversion strategies, the ubRMSE of decreased by 0.23 g/m2, the average ubRMSE of the hybrid RTM decreased by 0.16 g/m2, both greater than the ubRMSE of MLAs (average decrease of 0.06 g/m2).
4.4. Comparison of LAI and AGB estimates and remote sensing products
To verify the reliability of the RF integrated with the RTM for LAI, regression analysis was conducted by comparing LAI values obtained from the field observations with those extracted from the LAI estimates derived from Hybrid RTM RF, GLASS LAI, and MODIS LAI at the survey plots to obtain the correlation between the field observations and each remote sensing product. Taking into account the differences in the spatial scales, the GLASS LAI and MODIS LAI were resampled into 30 m resolution. The results are presented in .
Figure 8. Accuracy assessment of the estimated LAI (a) obtained using the GLASS LAI (b) and MODIS LAI (c) based on in-situ measurements in 2022.

The results indicate that the RF integrated with the RTM for the LAI was in better agreement with the in-situ measurements (R2 of 0.69 and ubRMSE of 0.24 m2/m2). In contrast, both the GLASS LAI and MODIS LAI exhibited lower levels of agreement. Notably, the RF integrated with the RTM for the LAI resulted in a significant 38% decrease in the ubRMSE compared with the GLASS LAI and a 29% decrease compared with the MODIS LAI. The GLASS LAI and MODIS LAI exhibited poor consistency with the field observations, which is mainly attributed to factors such as the spatial heterogeneity in the mountainous areas, the preprocessing of the satellite observations, and the retrieval methods.
The Hybrid RTM LAI estimate over study area was resampled into 250m and 500m, respectively to compare with GLASS LAI and MODIS LAI products pixel-by-pixel over the whole study area, as illustrated in the . According to the results, the correlation coefficients between the LAI results obtained using the RF integrated with the RTM and the GLASS LAI and MODIS LAI are 0.56 and 0.61, respectively, and both P-values are less than 0.05. This demonstrates the existence of a significant positive correlation, further confirming the robustness of the RF integrated with the RTM for LAI retrieval in mountainous areas.
The spatial distribution map of GLASS LAI, MODIS LAI and Hybrid RTM LAI and AGB was shown in the . It can be clearly seen that LAI values are relatively low since the grassland land cover types are predominant in the arid and semi-arid region. The greater LAI values are predominately distributed in the southern area, and the lower LAI values are primarily distributed in the northwestern area. Moreover, the spatial distribution of the Hybrid RTM estimates is more consistent with the MODIS LAI, meanwhile the spatial distribution of the estimated AGB is consistent with the distribution of LAI to some degree.
Figure 10. Visual comparisons of the LAI (a) and AGB (d) obtained using the RF integrated with the RTM for the inversion with the GLASS LAI (b) and MODIS LAI (c). The white pixels in the images are non-grassland pixels.

It should be noted that there is no AGB value in the CCI biomass product in the study area, which demonstrated that retrieval of AGB in mountainous area using SAR data is even more difficult for herbaceous vegetation with low AGB than for forest with high AGB. Therefore, Hybrid RTM AGB is able to fill the gap of current AGB product for the vegetation AGB with low value over the hilly Loess Plateau with the arid and semi-arid climatic condition.
5. Discussion
5.1. Impact of topographic correction on Landsat SR over mountainous areas
In complex topographic areas, the accuracy of remote sensing LAI products often falls short of application requirements (Jin et al. Citation2017). Previous studies have demonstrated that the differences in different LAI products are more significant in mountainous areas with complex topography (Xie et al. Citation2019). Topographic factors contribute to over 50% of the uncertainty in mountainous vegetation parameter retrieval (Yu, Li, and Liu Citation2019). Topographic factors (e.g. slope and aspect) dominate the spatial variations in the LAI retrieved from topographically uncorrected reflectance data, which easily leads to unjustifiable spatial differences in the LAI across difficult surfaces (Chen et al. Citation2020). Therefore, eliminating topographic effects from multispectral images is of great significance for retrieving the structural parameters of vegetation in mountainous areas (Wen et al. Citation2018).
a illustrates the impact of topographic correction on the Landsat8 OLI SR. The correlation between the cosi and NIR band reflectance is weaker after topographic correction, indicating a reduction in the topographic effects on the Landsat SR. Furthermore, we compared the results of the NIR band reflectance variations with the slope direction before and after topographic correction (b). It was found that the slope direction, particularly slopes facing the sun and slopes facing away from the sun, significantly impacts the variations in the NIR band compared with that before topographic correction. The degree of variation in the NIR band reflectance with slope direction is lower after topographic correction. The NIR band reflectance on the sunny slopes decreases, while that on the shaded slopes is compensated for, resulting in a more uniform distribution of the NIR band reflectance along the slope direction.
Figure 11. Comparison of the fitting results between the reflectance of NIR band and cosi before and after topographic correction (a). Variation of the NIR band reflectance with slope direction before and after topographic correction (b). The circumference denotes the slope direction, and the radius denotes the reflectance.

5.2. RTM-based LUT vs. hybrid RTM
The PROSAIL model is based on flat terrain, thus limiting its applicability in mountainous areas. It was found that the LAI and AGB retrieved based on the RTM had varying degrees of overestimation and underestimation. However, after topographic correction, this phenomenon was somewhat mitigated. This may be related to the fact that high LAI values and high AGB values are mainly distributed on slopes facing the sun, while low LAI values and low AGB values are typically distributed on shaded slopes (Jin et al. Citation2019). Moreover, spectral data from the red, NIR, and shortwave infrared bands are prone to being limited by signal saturation at moderately large LAI values. As a result, an underestimation of the retrieved LAI values sometimes occurs. Similar findings have been reported in a previous study (Sinha et al. Citation2020).
Although the RTM-based LUT is considered to be a more robust mechanism for retrieving canopy structural parameters compared with empirical models, the it is susceptible to limitations related to the retrieval methods and complexity of the canopy-radiation interaction processes (Houborg and McCabe Citation2018). While a higher accuracy can be achieved by setting a shorter parameter step size, this method consumes more computational resources. Hybrid RTM methods can leverage MLAs to establish the complex nonlinear relationships between the reflectance and the parameters to be retrieved in the LUT, enabling rapid inversion over large regions (Wang et al. Citation2022).
5.3. Machine learning algorithms vs. hybrid RTM
It was found that topographic correction improved the accuracies of the LAI and AGB estimation derived from both MLAs and hybrid RTM. By comparing sensitivities of the LAI and AGB estimates of different MLAs to the topographic effects, the ANN algorithm exhibited higher sensitivity to topographic effects, resulting in a significant decrease in ubRMSE by 25.0% for LAI and 11.6% for AGB. In contrast, the SVR algorithm displayed lower sensitivity, with only a marginal decrease in ubRMSE by 0.4% for LAI and 2.5% for AGB. In addition, the variance of the ubRMSE for the LAI and AGB retrieval was decreased by 80.5% and 74.3%, respectively, when topographically corrected Landsat SR was employed in the MLAs and hybrid RTM methods. This indicates that topographic correction can reduce the performance variability of different MLAs caused by the topographic effects.
It is well known that the performance of MLA retrieval is limited by the amount of samples, whereas it is very difficult to provide sufficient samples for retrieving the LAI and AGB over mountainous areas due to collecting field observations is challenging in the rugged loess hilly and gully region. The accuracy evaluation by three-fold cross validation strategy indicated that the commonality and robustness of estimation model derived from MLA is lower than that of Hybrid RTM, especially for the mountainous areas, which is consistent with the study results of Sun et al. (Citation2023).
In contrast, compared with MLAs, the hybrid RTM only requires a small number of field observations for calibrating radiative transfer model to establish the sufficient sample datasets (Lu et al. Citation2016). This greatly overcomes the limitation of MLAs requiring a large amount of field observations for retrieval. It has been proved that Hybrid RTM not only overcome the limitations of acquiring sample data but also achieve reliable and retrieval of vegetation parameters in the course of the extended application, demonstrating its higher practical value (Döpper et al. Citation2022).
5.4. Limitations of the study and prospects for future work
While existing topographic correction algorithms can partially alleviate topographic factors, their effectiveness varies depending on factors such as the sensor, wavelength, land surface type, and geographic area. Each method has certain strengths and weaknesses, and there is no one optimal method that applies to all images and all regions. The land cover type and spectral bands have significant impacts on the corrective effects of these techniques. Consequently, it is essential to select the best topographic correction method based on the land cover type and spectral bands.
Due to the harsh natural environment of the Loess Plateau hilly region, it is challenging to obtain a sufficient number of samples in mountainous areas. In the future, we will also collect more samples and open-source data to expand the number of sample plots in sparse regions. To refine the parameter input range of the mechanistic model more accurately for further validation and improvement.
6. Conclusions
Topographic correction using the SCS + C algorithm was successfully performed on the Landsat8 SR, and the performances of various retrieval strategies (RTM-based LUT, MLAs, and hybrid RTM) using the Landsat SR before and after topographic correction were also successfully assessed and compared. The results demonstrated that the LAI and AGB values retrieved from topographically corrected Landsat8 SR data outperformed those without topographic correction. Applying the SCS + C topographic correction algorithm can enhance the accuracy of retrieving the LAI and AGB in mountainous areas. It can also reduce the variability of the retrieval results of different algorithms. Compared with data-driven methods, methods based on the RTM are more sensitive to topography. In addition, the RF model has a stronger predictive performance compared to other MLAs, indicating a certain superiority for both MLAs and hybrid RTM methods. The RF integrated with the RTM for LAI has a higher spatial resolution and a closer spatial distribution than the existing LAI products. In addition, the RF integrated with the RTM for AGB fills the gaps in existing products.
Author contributions
Acquisition of financial support for the project leading to this publication, Z.W.; Application of statistical, mathematical, computational, or other formal techniques to analyze or synthesize study data, S.P. and Z.W.; Preparation, creation, and/or presentation of the published work by those from the original research group, specifically critical review, commentary, or revision, including pre- or post-publication stages, X.L., Z.W. and X.L.
Acknowledgements
The authors thank Yi Zhang, Miaomiao Cheng, Enjun Gong, Xu Li and Haolin Huang for assistance with LAI and measurements.
Disclosure statement
No potential conflict of interest was reported by the author(s).
Data availability statement
The code used in this study is available by contacting the corresponding author.
Additional information
Funding
References
- Baez-Gonzalez, Alma Delia, James R. Kiniry, Stephan J. Maas, Mario L. Tiscareno, Jaime C. Macias, Jose L. Mendoza, Clarence W. Richardson, Jaime G. Salinas, and Juan R. Manjarrez. 2005. “Large-Area Maize Yield Forecasting Using Leaf Area Index Based Yield Model.” Agronomy Journal 97 (2): 418–425. https://doi.org/10.2134/agronj2005.0418.
- Baret, F., J. Clevers, and M. D. Steven. 1995. “The Robustness of Canopy Gap Fraction Estimates from Red and Near-Infrared Reflectances: A Comparison of Approaches.” Remote Sensing of Environment 54 (2): 141–151. https://doi.org/10.1016/0034-4257(95)00136-O.
- Baret, F., S. Jacquemoud, G. Guyot, and C. Leprieur. 1992. “Modeled Analysis of the Biophysical Nature of Spectral Shifts and Comparison with Information Content of Broad Bands.” Remote Sensing of Environment 41 (2-3): 133–142. https://doi.org/10.1016/0034-4257(92)90073-S.
- Breiman, L. 2001. “Random Forests.” Machine Learning 45: 5–32. https://doi.org/10.1023/A:1010933404324.
- Chen, T., and C. Guestrin. 2016. “Xgboost: A Scalable Tree Boosting System.” Proceedings of the 22nd ACM SIGKDD International Conference on Knowledge Discovery and Data Mining 2016, 785–794. https://doi.org/10.1145/2939672.2939785.
- Chen, R., G. Yin, G. Liu, J. Li, and A. Verger. 2020. “Evaluation and Normalization of Topographic Effects on Vegetation Indices.” Remote Sensing 12 (14): 2290. https://doi.org/10.3390/rs12142290.
- Cheng, J., H. Yang, J. Qi, Z. Sun, S. Han, H. Feng, J. Jiang, et al. 2022. “Estimating Canopy-Scale Chlorophyll Content in Apple Orchards Using a 3D Radiative Transfer Model and UAV Multispectral Imagery.” Computers and Electronics in Agriculture 202: 107401. https://doi.org/10.1016/j.compag.2022.107401.
- Cortes, C., and V. Vapnik. 1995. “Support-vector Networks.” Machine Learning 20: 273–297. https://doi.org/10.1007/BF00994018.
- Danner, M., K. Berger, M. Wocher, W. Mauser, and T. Hank. 2021. “Efficient RTM-Based Training of Machine Learning Regression Algorithms to Quantify Biophysical & Biochemical Traits of Crops.” ISPRS Journal of Photogrammetry and Remote Sensing 173: 278–296. https://doi.org/10.1016/j.isprsjprs.2021.01.017.
- Ding, Y., H. Zhang, Z. Li, X. Xin, X. Zheng, and K. Zhao. 2016. “Comparison of Fractional Vegetation Cover Estimations Using Dimidiate Pixel Models and Look-up Table Inversions of the PROSAIL Model from Landsat 8 OLI Data.” Journal of Applied Remote Sensing 10 (3): 036022–036022. https://doi.org/10.1117/1.JRS.10.036022.
- Dong, Y., C. Zhao, G. Yang, L. Chen, J. Wang, and H. Feng. 2013. “Integrating a Very Fast Simulated Annealing Optimization Algorithm for Crop Leaf Area Index Variational Assimilation.” Mathematical and Computer Modelling 58 (3-4): 877–885. https://doi.org/10.1016/j.mcm.2012.12.013.
- Döpper, V., A. D. Rocha, K. Berger, T. Gränzig, J. Verrelst, B. Kleinschmit, and M. Förster. 2022. “Estimating Soil Moisture Content Under Grassland with Hyperspectral Data Using Radiative Transfer Modelling and Machine Learning.” International Journal of Applied Earth Observation and Geoinformation 2022 (110): 102817. https://doi.org/10.1016/j.jag.2022.102817.
- Dorigo, W. A., R. Zurita-Milla, A. J. de Wit, J. Brazile, R. Singh, and M. E. Schaepman. 2007. “A Review on Reflective Remote Sensing and Data Assimilation Techniques for Enhanced Agroecosystem Modeling.” International Journal of Applied Earth Observation and Geoinformation 9 (2): 165–193. https://doi.org/10.1016/j.jag.2006.05.003.
- Fang, H., F. Baret, S. Plummer, and G. Schaepman-Strub. 2019. “An Overview of Global Leaf Area Index (LAI): Methods, Products, Validation, and Applications.” Reviews of Geophysics 57 (3): 739–799. https://doi.org/10.1029/2018RG000608.
- Fang, H., and S. Liang. 2003. “Retrieving Leaf Area Index with a Neural Network Method: Simulation and Validation.” IEEE Transactions on Geoscience and Remote Sensing 41 (9): 2052–2062. https://doi.org/10.1109/TGRS.2003.813493.
- Ganguly, S., R. R. Nemani, G. Zhang, H. Hashimoto, C. Milesi, A. Michaelis, W. Wang, and P.S Votava. 2012. “Generating Global Leaf Area Index from Landsat: Algorithm Formulation and Demonstration.” Remote Sensing of Environment 2012 (122): 185–202. https://doi.org/10.1016/j.rse.2011.10.032.
- He, L., A. Li, G. Yin, et al. 2019. “Retrieval of Grassland Aboveground Biomass Through Inversion of the PROSAIL Model with MODIS Imagery.” Remote Sensing 11 (13): 1597. https://doi.org/10.3390/rs11131597.
- He, M., J. Wen, D. You, Y. Tang, S. Wu, D. Hao, X. Lin, and Z. Gong. 2022. “Review of Forest Leaf Area Index Retrieval Over Rugged Terrain Based on Remotely Sensed Data.” National Remote Sensing Bulletin, https://doi.org/10.11834/jrs.20210244.
- Houborg, R., and M. F. McCabe. 2018. “A Hybrid Training Approach for Leaf Area Index Estimation via Cubist and Random Forests Machine-Learning.” ISPRS Journal of Photogrammetry and Remote Sensing 135: 173–188. https://doi.org/10.1016/j.isprsjprs.2017.10.004.
- Jacquemoud, S., F. Baret, B. Andrieu, F. M. Danson, and K. Jaggard. 1995. “Extraction of Vegetation Biophysical Parameters by Inversion of the PROSPECT+ SAIL Models on Sugar Beet Canopy Reflectance Data. Application to TM and AVIRIS Sensors.” Remote Sensing of Environment 52 (3): 163–172. https://doi.org/10.1016/0034-4257(95)00018-V.
- Jahromi, M. N., S. Zand-Parsa, F. Razzaghi, S. Jamshidi, S. Didari, A. Doosthosseini, and H. R. Pourghasemi. 2023. “Developing Machine Learning Models for Wheat Yield Prediction Using Ground-Based Data, Satellite-Based Actual Evapotranspiration and Vegetation Indices.” European Journal of Agronomy 146: 126820. https://doi.org/10.1016/j.eja.2023.126820.
- Jia, X., B. Fu, X. Feng, G. Hou, Y. Liu, and X. Wang. 2014. “The Tradeoff and Synergy between Ecosystem Services in the Grain-for-Green Areas in Northern Shaanxi, China.” Ecological Indicators 43: 103–113. https://doi.org/10.1016/j.ecolind.2014.02.028.
- Jin, H., A. Li, J. Bian, X. Nan, W. Zhao, Z. Zhang, and G. Yin. 2017. “Intercomparison and Validation of MODIS and GLASS Leaf Area Index (LAI) Products Over Mountain Areas: A Case Study in Southwestern China.” International Journal of Applied Earth Observation and Geoinformation 55: 52–67. https://doi.org/10.1016/j.jag.2016.10.008.
- Jin, H., A. Li, W. Xu, Z. Xiao, J. Jiang, and H. Xue. 2019. “Evaluation of Topographic Effects on Multiscale Leaf Area Index Estimation Using Remotely Sensed Observations from Multiple Sensors.” ISPRS Journal of Photogrammetry and Remote Sensing 154: 176–188. https://doi.org/10.1016/j.isprsjprs.2019.06.008.
- Klemas, V. 2013. “Remote Sensing of Coastal Wetland Biomass: An Overview.” Journal of Coastal Research 29 (5): 1016–1028. https://doi.org/10.2112/JCOASTRES-D-12-00237.1.
- Kwon, S. K., H. S. Jung, W. K. Baek, and D. Kim. 2017. “Classification of Forest Vertical Structure in South Korea from Aerial Orthophoto and Lidar Data Using an Artificial Neural Network.” Applied Sciences 7 (10): 1046. https://doi.org/10.3390/app7101046.
- Lu, D., Q. Chen, G. Wang, L. Liu, G. Li, and E. Moran. 2016. “A Survey of Remote Sensing-Based Aboveground Biomass Estimation Methods in Forest Ecosystems.” International Journal of Digital Earth 9 (1): 63–105. https://doi.org/10.1080/17538947.2014.990526.
- Ma, H., and S. Liang. 2022. “Development of the GLASS 250-m Leaf Area Index Product (Version 6) from MODIS Data Using the Bidirectional LSTM Deep Learning Model.” Remote Sensing of Environment 273: 112985. https://doi.org/10.1016/j.rse.2022.112985.
- Markwell, J., J. C. Osterman, and J. L. Mitchell. 1995. “Calibration of the Minolta SPAD-502 Leaf Chlorophyll Meter.” Photosynthesis Research 46: 467–472. https://doi.org/10.1007/BF00032301.
- Maxwell, A. E., T. A. Warner, and F. Fang. 2018. “Implementation of Machine-Learning Classification in Remote Sensing: An Applied Review.” International Journal of Remote Sensing 39 (9): 2784–2817. https://doi.org/10.1080/01431161.2018.1433343.
- Mountrakis, G., J. Im, and C. Ogole. 2011. “Support Vector Machines in Remote Sensing: A Review.” ISPRS Journal of Photogrammetry and Remote Sensing 66 (3): 247–259. https://doi.org/10.1016/j.isprsjprs.2010.11.001.
- Nguy-Robertson, A. L., Y. Peng, A. A. Gitelson, T. J. Arkebauer, A. Pimstein, I. Herrmann, A. Karnieli, D. C. Rundquist, and D. J. Bonfil. 2014. “Estimating Green LAI in Four Crops: Potential of Determining Optimal Spectral Bands for a Universal Algorithm.” Agricultural and Forest Meteorology 192: 140–148. https://doi.org/10.1016/j.agrformet.2014.03.004.
- Pasolli, L., S. Asam, M. Castelli, L. Bruzzone, G. Wohlfahrt, M. Zebisch, and C. Notarnicola. 2015. “Retrieval of Leaf Area Index in Mountain Grasslands in the Alps from MODIS Satellite Imagery.” Remote Sensing of Environment 165: 159–174. https://doi.org/10.1016/j.rse.2015.04.027.
- Qi, J., D. Xie, J. Jiang, and H. Huang. 2022. “3D Radiative Transfer Modeling of Structurally Complex Forest Canopies Through a Lightweight Boundary-Based Description of Leaf Clusters.” Remote Sensing of Environment 283: 113301. https://doi.org/10.1016/j.rse.2022.113301.
- Quan, X., B. He, M. Yebra, C. Yin, Z. Liao, X. Zhang, and X. Li. 2017. “A Radiative Transfer Model-Based Method for the Estimation of Grassland Aboveground Biomass.” International Journal of Applied Earth Observation and Geoinformation 54: 159–168. https://doi.org/10.1016/j.jag.2016.10.002.
- Roupioz, L., L. Jia, F. Nerry, and M. Menenti. 2014. “Correction of Sub-Pixel Topographical Effects on Land Surface Albedo Retrieved from Geostationary Satellite (FengYun-2D) Observations.” IOP Conference Series: Earth and Environmental Science 17 (1): 012270. https://doi.org/10.1088/1755-1315/17/1/012270.
- Shi, Y., Z. Wang, L. Liu, C. Li, D. Peng, and P. Xiao. 2021. “Improving Estimation of Woody Aboveground Biomass of Sparse Mixed Forest Over Dryland Ecosystem by Combining Landsat-8, GaoFen-2, and UAV Imagery.” Remote Sensing 13 (23): 4859. https://doi.org/10.3390/rs13234859.
- Sinha, S. K., H. Padalia, A. Dasgupta, J. Verrelst, and J. P. Rivera. 2020. “Estimation of Leaf Area Index Using PROSAIL Based LUT Inversion, MLRA-GPR and Empirical Models: Case Study of Tropical Deciduous Forest Plantation, North India.” International Journal of Applied Earth Observation and Geoinformation 86: 102027. https://doi.org/10.1016/j.jag.2019.102027.
- Soenen, S. A., D. R. Peddle, and C. A. Coburn. 2005. “SCS+ C: A Modified sun-Canopy-Sensor Topographic Correction in Forested Terrain.” IEEE Transactions on Geoscience and Remote Sensing 43 (9): 2148–2159. https://doi.org/10.1109/TGRS.2005.852480.
- Soudani, K., C. François, G. Le Maire, V. Le Dantec, and E. Dufrêne. 2006. “Comparative Analysis of IKONOS, SPOT, and ETM+ Data for Leaf Area Index Estimation in Temperate Coniferous and Deciduous Forest Stands.” Remote Sensing of Environment 102 (1-2): 161–175. https://doi.org/10.1016/j.rse.2006.02.004.
- Sun, Q., Q. Jiao, X. Chen, H. Xing, W. Huang, and B. Zhang. 2023. “Machine Learning Algorithms for the Retrieval of Canopy Chlorophyll Content and Leaf Area Index of Crops Using the PROSAIL-D Model with the Adjusted Average Leaf Angle.” Remote Sensing 15 (9): 2264. https://doi.org/10.3390/rs15092264.
- Sun, J., Y. Wang, S. Piao, M. Liu, G. Han, J. Li, E. Liang, T. Lee, G. Liu, A. Wilikes, et al. 2022. “Toward a Sustainable Grassland Ecosystem Worldwide.” The Innovation 3 (4), https://doi.org/10.1016/j.xinn.2022.100265.
- Tibshirani, R. 1996. “Regression Shrinkage and Selection via the Lasso.” Journal of the Royal Statistical Society Series B: Statistical Methodology 58 (1): 267–288. https://doi.org/10.1111/j.2517-6161.1996.tb02080.x.
- Verhoef, W. 1984. “Light Scattering by Leaf Layers with Application to Canopy Reflectance Modeling: The SAIL Model.” Remote Sensing of Environment 16 (2): 125–141. https://doi.org/10.1016/0034-4257(84)90057-9.
- Verrelst, J., G. Camps-Valls, J. Muñoz-Marí, J. P. Rivera, F. Veroustraete, J. G. Clevers, and J. Moreno. 2015. “Optical Remote Sensing and the Retrieval of Terrestrial Vegetation bio-Geophysical Properties–A Review.” ISPRS Journal of Photogrammetry and Remote Sensing 108: 273–290. https://doi.org/10.1016/j.isprsjprs.2015.05.005.
- Wang, Z., M. Cheng, Y. Zhang, S. Peng, and H. Huang. 2023. “A 30 m Annual Land Cover Dataset of Chinese Loess Plateau from 1990 to 2020” (Dataset). Accessed number v.1. https://doi.org/10.5281/zenodo.10225564.
- Wang, X., X. Lu, X. Zhang, and Z. Yang. 2022. “Inversion of the Hybrid Machine Learning Model to Estimate Leaf Area Index of Winter Wheat from GaoFen-6 WFV Imagery.” Geocarto International 37 (27): 16307–16322. https://doi.org/10.1080/10106049.2022.2108906.
- Wang, T., Z. Xiao, and Z. Liu. 2017. “Performance Evaluation of Machine Learning Methods for Leaf Area Index Retrieval from Time-Series MODIS Reflectance Data.” Sensors 17 (1): 81. https://doi.org/10.3390/s17010081.
- Weiss, M., F. Jacob, and G. Duveiller. 2020. “Remote Sensing for Agricultural Applications: A Meta-Review.” Remote Sensing of Environment 236: 111402. https://doi.org/10.1016/j.rse.2019.111402.
- Wen, J., Q. Liu, Q. Xiao, Q. Liu, D. You, D. Hao, S. Wu, and X. Lin. 2018. “Characterizing Land Surface Anisotropic Reflectance Over Rugged Terrain: A Review of Concepts and Recent Developments.” Remote Sensing 10 (3): 370. https://doi.org/10.3390/rs10030370.
- Wilson, A. M., and W. Jetz. 2016. “Remotely Sensed High-Resolution Global Cloud Dynamics for Predicting Ecosystem and Biodiversity Distributions.” PLoS Biology 14 (3): e1002415. https://doi.org/10.1371/journal.pbio.1002415.
- Wu, L., Y. Peng, J. Fan, Y. Wang, and G. Huang. 2021. “A Novel Kernel Extreme Learning Machine Model Coupled with K-Means Clustering and Firefly Algorithm for Estimating Monthly Reference Evapotranspiration in Parallel Computation.” Agricultural Water Management 245: 106624. https://doi.org/10.1016/j.agwat.2020.106624.
- Wu, Z., C. Zhao, and Q. Qin. 2022. “Evaluating the Impact of Spatial Heterogeneity on the Prosail Model and Lai Inversion.” IGARSS 2022-2022 IEEE International Geoscience and Remote Sensing Symposium. IEEE, 6260–6263. https://doi.org/10.1109/IGARSS46834.2022.9884257.
- Xie, X., A. Li, X. Guan, J. Tan, H. Jin, and J. Bian. 2021. “A Practical Topographic Correction Method for Improving Moderate Resolution Imaging Spectroradiometer Gross Primary Productivity Estimation Over Mountainous Areas.” International Journal of Applied Earth Observation and Geoinformation 103: 102522. https://doi.org/10.1016/j.jag.2021.102522.
- Xie, X., A. Li, H. Jin, J. Tan, C. Wang, G. Lei, Z. Zhang, J. Bian, and X. Nan. 2019. “Assessment of Five Satellite-Derived LAI Datasets for GPP Estimations Through Ecosystem Models.” Science of The Total Environment 690: 1120–1130. https://doi.org/10.1016/j.scitotenv.2019.06.516.
- Yang, J., and X. Huang. 2021. “The 30 m Annual Land Cover Dataset and its Dynamics in China from 1990 to 2019.” Earth System Science Data 13 (8): 3907–3925. https://doi.org/10.5194/essd-13-3907-2021.
- Yin, G., J. Li, Q. Liu, W. Fan, B. Xu, Y. Zeng, and J. Zhao. 2015. “Regional Leaf Area Index Retrieval Based on Remote Sensing: The Role of Radiative Transfer Model Selection.” Remote Sensing 7 (4): 4604–4625. https://doi.org/10.3390/rs70404604.
- Yu, R., A. J. Evans, and N. Malleson. 2018. “Quantifying Grazing Patterns Using a new Growth Function Based on MODIS Leaf Area Index.” Remote Sensing of Environment 209: 181–194. https://doi.org/10.1016/j.rse.2018.02.034.
- Yu, W., J. Li, and Q. Liu. 2019. “Topographic Effects on Leaf Area Index Retrieval by Remote Sensing Approach.” IGARSS 2019-2019 IEEE International Geoscience and Remote Sensing Symposium. IEEE, 6539–6542. https://doi.org/10.1109/IGARSS.2019.8900658.
- Yu, W., J. Li, Q. Liu, G. Yin, Y. Zeng, S. Lin, and J. Zhao. 2020. “A Simulation-Based Analysis of Topographic Effects on LAI Inversion Over Sloped Terrain.” IEEE Journal of Selected Topics in Applied Earth Observations and Remote Sensing 13: 794–806. https://doi.org/10.1109/JSTARS.2020.2970999.