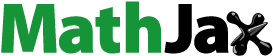
ABSTRACT
Offshore small-scale fisheries, constituting 31% of global marine captures, are crucial for the promotion of sustainable fisheries. However, current fishery monitoring, assessments, and management remain incomplete. Nighttime remote sensing provides a critical perspective for monitoring fishery activities. Thus, in this study, a dynamic threshold fishing vessel detection (DTFVD) model was developed to assess and manage the spatiotemporal variations of offshore fisheries. Results demonstrated that the DTFVD model provides superior accuracy in nighttime fishery monitoring and can successfully identify a robust seasonal bimodal distribution of the Yellow-Bohai Sea's fisheries. By analysing the impacts of climate, environmental, and policy factors, this study reveals that ocean dynamics affect the seasonal and geographic distribution of offshore nighttime fisheries by enhancing ocean mixing and ecological processes. The effects of the COVID-19 lockdowns and Chinese summer fishing moratorium on offshore fisheries were also determined by nighttime remote sensing. According to future climate warming predictions, the habitats for the recruited migratory fish population may gradually move northward and disappear under climate risks, leading to the disappearance of suitable fishing areas for nighttime fisheries. Therefore, this study suggests integrating nighttime remote sensing into offshore fishery monitoring and assessment efforts for climate-adapted fishery management strategies.
1. Introduction
As one of humanity's most critical socioeconomic-related activities in the ocean, marine capture provided up to 78.8 million tonnes of capture fisheries production worldwide in 2020 (FAO Citation2022), playing an integral role in the global food supply and future bluefood transformation (Tigchelaar et al. Citation2022). Offshore small-scale fisheries, which account for more than 31% of the world's marine capture production, are critical to maintaining the current and future sustainable utilization of living marine resources (Gutierrez et al. Citation2023; Melnychuk et al. Citation2021). However, rich fishery resources and intensive fishery activities have also led to frequent illegal, unreported and unregulated (IUU) fishing and fishery conflicts in offshore waters (Tian et al. Citation2022b). Monitoring and accessing the spatiotemporal variation mechanism of offshore fisheries is the basis for effective management and conservation measures under changing marine ecological structures, the challenge of IUU fisheries, and the decline of fishery resources in traditional offshore fishing grounds attributed to pressures including overfishing and climate change (Boyce et al. Citation2022; Cinner et al. Citation2022). The number of fishing vessels operating in offshore waters, mostly smaller than 12 m, is as high as 3.32 million, accounting for 81% of the global fishing fleet (FAO Citation2022). Automatic identification systems (AIS) and vessel monitoring systems (VMS) are commonly used in the tracking and management of fishing vessels. However, effectively monitoring offshore fishing vessels proves to be a challenge as AIS and VMS are only suitable for fishing vessels over 150 tonnes and only for some territorial waters, respectively (James et al. Citation2018; Kroodsma et al. Citation2018). Therefore, it is essential to develop efficient spatiotemporal monitoring data to brtter understand the variation mechanism of offshore fishery and promote the sustainable exploitation of fisheries.
The increasing number of satellites in space provides new means for the monitoring of human socio-economic activities. With the decline in the average trophic level of marine ecosystems, nighttime fisheries have begun to spread offshore, using high-power electric lights to attract phototactic pelagic fish such as Japanese flying squid (Todarodes pacificus) and Pacific saury (Cololabis saira) (Elvidge et al. Citation2018; Liu et al. Citation2015). The development of nighttime remote sensing technology, using satellite sensors to observe and map the nighttime lights (NTL) from Earth, can be traced back to the 1970s (Elvidge et al. Citation2022). The Visible Infrared Imager Radiometer Suite (VIIRS) sensor onboard the Suomi National Polar-orbiting Partnership satellite was launched in 2011. It is fitted with a specific optical spectrum band (spanning ∼0.5–0.9 μm) denoted as the Day/Night Band (DNB) to sense extremely low levels of light at night (Miller et al. Citation2012). The daily global coverage, near real-time acquisition, and broad detection range facilitate the precise identification of night-light fishing vessels via DNB images (Miller et al. Citation2013; Rogers et al. Citation2023). Therefore, the 742 m resolution DNB images, which are available to the public, are frequently used in the spatiotemporal dynamic mapping of nighttime fisheries in both offshore waters and distant regions (Cozzolino and Lasta Citation2016; Elvidge et al. Citation2015; Hsu et al. Citation2019; Tian et al. Citation2022a; Tian et al. Citation2022b). However, the advanced low-light detection capabilities of DNB images allow the sensing of light from fishery activities and gas flares, but also the moonlight reflected from clouds, sea fog, snow and ice, particularly during full moons (Elvidge et al. Citation2013). Therefore, the accuracy of night-light fishing vessel detection may be affected by noise such as gas flares in the sea and moonlight reflected from clouds or sea fog. The previous fixed empirical threshold segmentation algorithms needed to consider the moon phase's variation and cloud cover fully (Chen et al. Citation2019; Tian et al. Citation2022b). There is a lack of research on the spatiotemporal distribution of offshore fishery based on more precise quantitative and geographical night-light fishing vessel detection data.
In addition to more accurate monitoring data, numerous factors affecting the distribution of offshore nighttime fisheries should also be considered. Nighttime remote sensing has unique advantages and an irreplaceable role in the assessment of the influence of large-scale human activities on fisheries (Levin et al. Citation2020). The impact of coronavirus (COVID-19) lockdowns on local and global fisheries has been extensively explored by matching remote sensing-based fishery activities and catch data since the virus outbreak in December 2019 (He et al. Citation2021; March et al. Citation2021; Tian et al. Citation2022b). Despite the mounting concerns over the decline in capture production during COVID-19, limited attention has been focused on the effects of the COVID-19 lockdowns on the spatiotemporal distribution of offshore fisheries in neighboring countries. Fishing closure, which aims to manage and protect fishing resources, establishes deliberate restrictions on fishery activities in offshore waters, unlike an emergency lockdown in response to the epidemic (Dimarchopoulou et al. Citation2018; Richerson, Leonard, and Holland Citation2018). It requires accurate fishery monitoring and assessments that consider the local conditions, the life history characteristics and spatiotemporal distribution mechanisms of fishery resources in various waters. DNB images have been employed to assess the effectiveness of fishery closures in Vietnam, the Philippines, and the Beibu Gulf of China, and more importantly, can be generalized to the spatially refined fishery management in other waters (Elvidge et al. Citation2018; Tian et al. Citation2022b).
Furthermore, nighttime remote sensing provides a unique perspective for assessing the habitat changes of marine living resources, which are inextricably linked to the spatiotemporal distribution of fisheries activities. In this process, such changes play a vital role in the marine environment and oceanographic features at different scales (Arostegui et al. Citation2022; Mondal et al. Citation2021b; Mondal et al. Citation2022b; Smith, Capinha, and Kramer Citation2022; Vayghan et al. Citation2020). The warming planet, however, is profoundly affecting marine environmental factors and the global species distribution, thus creating more uncertainties for current and future fisheries sustainability (Mondal et al. Citation2021a; Mondal and Lee Citation2023b; VanDerWal et al. Citation2013). The Yellow-Bohai Sea, one of the western Pacific marginal seas, produced 2.92 million tons fishery resources in 2019 (Jin et al. Citation2005). This region extensively employs light purse seine fisheries for mackerel, anchovy, and squid (Li et al. Citation2014). The Yellow-Bohai Sea is a semi-enclosed shallow body of water, and thus its temperatures have rapidly increased over the past few decades, exacerbating the changes to its fishery resources caused by climate change (Belkin Citation2009; Boyce et al. Citation2022). Previous studies have shown that seasonal ocean fronts form in the Yellow-Bohai seas under the influence of marine dynamic processes such as the Yellow Sea warm current (YSWC), the Yellow Sea cold water mass (YSCWM), and coastal currents (Diao et al. Citation2021; Lü et al. Citation2010). These frontal areas effectively promote ocean mixing and lead to high zooplankton productivity, facilitating offshore fishing ground formation (Oh et al. Citation2019). Future global warming will degrade the habitats of most commercial fish species in the Yellow-Bohai Sea, and thus climate-appropriate fishery management strategies are necessary (Ma et al. Citation2022). Despite having one of the highest fishing intensities in the world and its high sensitivity to climatic change, the fishery resource surveys and assessment in the Yellow-Bohai Sea are constrained by the survey scope, sampling method, and financial support. Nighttime remote sensing can undoubtedly inspire the application of large-scale marine monitoring data to explore the spatiotemporal distribution mechanism of offshore fishing vessels and promote spatially refined fishery management under the influence of climate change, oceanographic features, and fishery policies.
Therefore, this study aims to: (1) develop a dynamic threshold fishing vessel detection (DTFVD) model to extract accurate fishery data; (2) map and access the spatiotemporal variation mechanism of offshore nighttime fisheries, and (3) promote spatially refined sustainable fishery management strategies under the changing climate and policy factors of the Yellow-Bohai Sea.
2. Materials and methods
2.1. Study area
The Yellow-Bohai Sea (33°N–41°N, 117°E–127°E) is surrounded by the Chinese mainland and the Korean Peninsula (). The study area is divided into five regions, namely, the Bohai Sea (BS), the Northern Yellow Sea (NYS), the Western Yellow Sea (WYS), the Central Yellow Sea (CYS), and the Eastern Yellow Sea (EYS), based on the National Marine Functional Zoning of China and the China–Korea Fishery Agreement signed in 2000 (Ministry of Foreign Affairs of the People's Republic of China Citation2020; Natural Resources of the People’s Republic of China Citation2012). The line connecting the Laotie Mountain in Liaoning Province and the Penglai Cape in Shandong Province separates the BS from the NYS north of 37°N. The CYS refers to the China–Korea Fishery Agreement waters between 33-37°N and 122-124°E, where the distribution of fishing vessels has gradually attracted the attention of fishery management agencies since the signing of the fishery agreement (Straka et al. Citation2015). Furthermore, the boundary of the CYS allows us to explore the spatial distribution patterns of nighttime fisheries under the different management policies of neighboring nations (Shim, Tariq, and Chowell Citation2021; Xue Citation2005; Zhao et al. Citation2020). In addition to the EYS, the current fishery activities of different gears in the Yellow-Bohai Sea are affected by the Chinese summer fishing moratorium. Based on the closed seasons shown in , we explore the impact of the fishing moratorium on Yellow-Bohai Sea’s nighttime fishery activities (Zhu Citation2009).
Figure 1. Map of the Yellow-Bohai Sea. The dashed golden lines separate the Bohai Sea (BS), Northern Yellow Sea (NYS), Western Yellow Sea (WYS), Central Yellow Sea (CYS), and Eastern Yellow Sea (EYS). The areas with black shadows denote the China-Korea fishery agreement waters, and the red points are gas flares.

2.2. Data collection
2.2.1. DNB images
DNB images with a 742 m resolution and corresponding cloud masking data from January 1, 2013, to May 31, 2021, were used to extract DTFVDs in the Yellow-Bohai Sea, distributed in version 5 of the Hierarchal Data Format. These data were obtained from the NOAA Comprehensive Larger Array-Data Stewardship System (https://www.avl.class.noaa.gov/) between March and December 2020.
2.2.2. Moon phase index and vector data
The moon phase properties in the study area were calculated to explore the influence of lunar phase changes on the number and thresholds of DTFVD using the Marine Geospatial Ecology Tools in the ArcGIS 10.7 (Esri) toolbox. In this study, regardless of the difference between the first and last quarter moons, the moon phase properties were converted to a moon phase index ranging from 0 (new moon) to 1 (full moon) (Roberts et al. Citation2010).
To remove the interference from land lights and burning sources on the identification of DTFVDs, vector data, including land-sea boundaries and active fire data in the Yellow-Bohai Sea, were acquired from the Nature Earth dataset (http://www.naturalearthdata.com/) and NASA’s Fire Information for Resource Management System (https://firms.modaps.eosdis.nasa.gov/) on July 13, 2020, respectively (Sun et al. Citation2020).
2.2.3. AIS-based fishing vessels
The AIS-based daily fishing effort dataset, which contains 0.1° gridded fishing effort data for each registered vessel, was downloaded from the Global Fishing Watch online repository (https://globalfishingwatch.org/datasets-and-code/) on March 17, 2021 to test the effectiveness of NTL images in the spatial monitoring of fishery activities. When extracting AIS-based fishing vessels from multiple fishing locations of the same fishing vessel, only the locations with the most extensive daily fishing hours were considered. Location data with fishing hours equal to 0 were excluded from the analysis.
2.2.4. Marine environmental factors
In this study, marine environmental factors, including water depth, sea surface temperature (SST), sea surface chlorophyll-a concentration (SSC), sea surface salinity (SSS), sea level anomalies (SLA), surface current velocity (SCV), SST_front and SSS_front, were collected or calculated to explore the impacts of oceanographic features on the distribution of nighttime fisheries in the Yellow-Bohai Sea. The gridded bathymetry data were accessed from the British Oceanographic Data Centre (https://www.bodc.ac.uk/) on July 29, 2022. Monthly SST and SSC data with a 4 km spatial resolution were downloaded from NOAA Ocean Watch (https://oceanwatch. pifsc.noaa.gov/) on October 11, 2022. The missing pixels in the SSC data due to cloud cover were filled by the mean value of surrounding pixels. Monthly SSS and SLA data were obtained from the Copernicus Marine Service (https://resources.marine.copernicus.eu/) on October 11, 2022, with resolutions of 0.083° and 0.25°, respectively. The SCV was calculated as follows:
(1)
(1) where
is the surface current velocity of grid
in month
; and
and
are the northward and eastward sea water velocities of grid
in month
derived from the Copernicus Marine Service, with a resolution of 0.083°. SST_front and SSS_front were extracted from monthly SST and SSS data by a mesoscale front detection algorithm, and a series of local thresholds and morphological methods were used to generate seasonally persistent fronts (Xing et al. Citation2023a; Xing et al. Citation2023b).
Monthly sea ice thickness data with a resolution of 0.083°, downloaded from the Copernicus Marine Services on October 12, 2022, was used to map sea ice masks in the Yellow-Bohai Sea, considering that the distribution of sea ice in the middle and upper latitudes during winter can limit nighttime fishing activities.
2.2.5. IPCC-RCP scenarios
The Representative Concentration Pathways (RCPs), a series of integrated concentration and emission scenarios, were used to describe future atmospheric composition concentrations under different emissions scenarios of human activities in the twenty-first century (Moss et al. Citation2010). Based on greenhouse gas emissions with or without climate change policy interventions, RCPs consist of four emission scenarios (RCP 8.5, RCP 6.0, RCP 4.5, and RCP 2.6), in which RCP 8.5 without human intervention will lead to the fastest future warming. Moreover, RCP 2.6 has the most negligible impact on global warming (Hurtt et al. Citation2011). To predict the seasonal spatiotemporal distribution of nighttime fisheries in the Yellow-Bohai Sea by the end of this century, projected monthly SST, SSS, and SCV raster datasets of RCP 2.6 and RCP 8.5 were acquired at a resolution of 0.083° from Bio-ORACLE (https://www.bio-oracle.org) on December 9, 2022. These environmental raster files were seasonally fuzed and used as input data to generate future fishery distribution based on the optimal model built in R 4.3.0 (R Core Team).
2.3. NTL data preprocessing
outlines the approach used to monitor and analyze the spatiotemporal distribution mechanism of nighttime fisheries in the Yellow-Bohai Sea. The original radiance values of the DNB images, generally between 10−11 and 10−8 W/cm2/sr, were converted to nW/cm2/sr by multiplying by 109 to simplify the data processing. The geometric correction was implemented using the Build Geographic Lookup Table (GLT) and Georeference from GLT in ENVI 5.3 toolbox (Exelis Visual Information Solutions) to provide the pixel-wise geographical coordinates corresponding to the raw DNB image. The flashes of lightning, horizontal light stripes in the DNB image, were then filtered out by strip masks (Elvidge et al. Citation2015). Pixels glowing in the same position for continuous months were considered as persistent bright noises from lighthouses and aquaculture facilities and were eliminated by circular masks. The bright pixels located in shipping lanes and anchorage masks were also removed. Furthermore, a 2 km buffer mask based on the land-sea boundaries and active fire points was constructed to remove the interferences from land and gas fires. Finally, monthly sea ice coverage was used as a mask to remove the areas in NTL images where fishing boats are unable to operate.
2.4. DTFVD model
In the DTFVD model, the spike median index (SMI) was calculated to amplify the difference between light sources and background pixels (Elvidge et al. Citation2015). Based on the coastal nighttime fishery monitoring model developed by Tian et al. in Citation2022, night-light fishing vessels in the Yellow-Bohai Sea in 2013 were first extracted to explore the relationships between the moon phase index and the detection threshold (Tian et al. Citation2022b). As the moon phase index increased, the detection number of fishing vessels decreased significantly (R2 = 0.58, p < 0.0001) (Figure A1(a)), while the detection threshold increased exponentially (R2 = 0.93, p < 0.0001) (Figure A1(b)), particularly when the moon phase index was more significant than 0.7. Therefore, we derived an adaptive threshold to remove dark background pixels using the following formula:
(2)
(2) where
and
are the daily moon phase index and adaptive initial threshold in the DTFVD, respectively. A focal statistics tool was subsequently used to calculate the largest SMI of luminous pixels within a circular neighborhood of a 2-pixel radius. To extract the target pixels with a local maximum SMI from surrounding halos, the image before the application of the focal statistics was subtracted from the image after the application of the focal statistics, and the zero-value pixels were marked as target fishing vessels. To ensure the accuracy of the DTFVD, the negative results were reextracted by adjusting the threshold slightly under visual verification, and only the vessels detected based on cloud-free NTL images with a moon phase index less than 0.7 were extracted as final DTFVD data.
2.5. Spatiotemporal merging of DTFVDs
Offshore fishing vessels that have been provided the ability to refrigerate their catches typically operate constantly, resulting in minimal changes in the number and distribution of nighttime fishery activities over a certain period. Therefore, the monthly average number of DTFVDs in the Yellow-Bohai Sea was calculated using the following formulation:
(3)
(3) where
is the average number of DTFVDs in a given month;
is the number of days in the current month without cloud cover with a moon phase index less than 0.7; and
is the daily number of DTFVDs. To highlight the spatial differences of nighttime fisheries within the same comparison period, the kernel density (0.1° search radius) and radiance raster of DTFVDs with a 0.05° spatial resolution were normalized and averaged. These spatiotemporal merging data were used to explore the distribution patterns of DTFVDs and the impact of management policies.
2.6. Establishment and evaluation of the fishery distribution model
The spatiotemporal variation of fishery activities is closely related to the distribution of fish species, whose abundance and migration are affected by the nonlinear effects of marine environmental changes. Therefore, to assess the relationship between the marine environment and nighttime fisheries in the Yellow-Bohai Sea, the logarithmic kernel density and radiance of DTFVDs after 0.5° resampling were taken as response variables affected by the environmental changes. The predictive variables consisted of eight environmental factors, including depth, SCV, SLA, SSC, SST, SST_front, SSS and SSS_front.
Random forest (RF) models have been demonstrated to perform well in fish habitat prediction, providing a new perspective and means to explore fishery activities (Xing et al. Citation2022; Zhang et al. Citation2022a). Therefore, in addition to traditional statistical regression models such as the generalized linear model (GLM) and generalized additive model (GAM), we also constructed an RF model based on emerging machine learning. These models used to quantitatively analyse the impacts of environmental factors on the distribution of DTFVDs are collectively referred to as the fishery distribution model. Prior to the modeling, Pearson’s correlation coefficients were examined to exclude predictive variables with collinearity, where SLA and depth were filtered due to the strong correlation with SST and SSC, respectively (R > 0.7, Figure A2). By incrementally adding non-collinear environmental variables, a total of 768 seasonal fishery distribution models were constructed, in which the RFs, GLMs and GAMs were implemented respectively in the R package ‘random forest’, ‘glm’ and ‘mgcv’ (Guisan, Edwards, and Hastie Citation2002; Liaw and Wiener Citation2002; Wood Citation2006). The parameter ‘num.trees’ in the RFs was set to 500, and the GLMs and GAMs are described as follows:
(4)
(4)
(5)
(5) where g is the link function that establishes the relationship between the response variables and predictor variables;
is the response variable (logarithmic kernel density and logarithmic radiance of DTFVDs);
is the predictive variable (depth, SCV, SLA, SSC, SST, SST_front, SSS and SSS_front); n is the number of predictive variables;
and
are the model constants;
is the smoothing function for
; and
is the random error.
The deviance explained was used to evaluate the explanatory rate of different combinations of environmental factors to the fishery distribution model, and the optimal model (with the highest deviance explained) was selected to forecast the future nighttime fishery distribution. All fishery distribution models were created using DTFVD data from 2013–2018 to exclude the effect of COVID-19 on fishery activities. The performance of the best model was assessed by comparing the anticipated and actual values of the response variables in 2019.
2.7. Evaluating the impact of environmental factors
The percentage increase of the mean square error (%IncMSE) was used to investigate the role of various environmental factors in the optimal fishery distribution model, which was implemented in the R package ‘imp’, and the higher the value, the more critical the environmental variable. Using the R package ‘iml’, the range and optimum value of the positive effects of these environmental factors on the distribution of nighttime fisheries were further explored based on Accumulated Local Effects (ALE) plots, which can eliminate the effect of the other variables in machine learning (Molnar Citation2018).
3. Results
3.1. Improved accuracy of DTFVD data
depicts the findings of DTFVD in the Yellow-Bohai Sea, January 2013 as an example. Compared with the 31-day raw DNB composite image ((a)), the average number of bright pixels and normalized average radiance of the cloud-free DNB composite image are significantly higher ((b)), and are not affected by cloud coverage. Therefore, the average number of fishing vessels detected from cloudless DNB images significantly increased to approximately 985 per day, 14.31 and 2.6 times, respectively, compared to AIS data and 31-day raw DNB composite images ((f)). The increased detection capability also allowed for the accurate mapping of nighttime fishery activities in the Yellow-Bohai Sea. Compared with the results of AIS data ((c)) and raw DNB images ((e)), the normalized kernel density distribution of DTFVDs is more spatially explicit, particularly ensuring the monitoring of fishery activities in the CYS and EYS ((d)).
Figure 3. Performance of dynamic threshold fishing vessel detection (DTFVD) model in the Yellow-Bohai Sea in January 2013. (a) Normalized radiance of 31-day raw Day/Night Band (DNB) composite image. (b) Normalized radiance of cloudless DNB composite image. (c) Normalized kernel density of raw DNB-based fishing vessels. (d) Normalized kernel density of DTFVDs. (e) Normalized kernel density of automatic identification systems (AIS)-based fishing vessels. (f) Comparison of the average number of AIS-based fishing vessels, raw DNB-based fishing vessels and DTFVDs in the Yellow-Bohai Sea in January 2013.

3.2. Fluctuations in the number of DTFVDs in the Yellow-Bohai Sea
presents the fluctuations in the number of DTFVDs in the Yellow-Bohai Sea from 2013 to 2021. First of all, the time span of cloud-free NTL data in most months is approximately 50%, which ensures the representativeness of the detection results ((a)). The number of DTFVDs in the Yellow-Bohai Sea exhibited an apparent seasonal bimodal fluctuation that underwent four stages:
a small peak in spring (March to May, with an average of 972 DTFVDs per day);
a trough in summer (June to August, with an average of 782 DTFVDs per day);
a significant peak in autumn (September to November, with an average of 1729 DTFVDs per day); and
a trough in winter (December to February, with an average of 937 DTFVDs per day) ((b)).
Figure 4. (a) Monthly proportion of cloudless DNB images in the Yellow-Bohai Sea from 2013–2021. (b) Fluctuations in the average number of DTFVDs in the Yellow-Bohai Sea from 2013–2021. (c) Fluctuations in the average number of DTFVDs in different waters of the Yellow-Bohai Sea from 2013–2021. The colored whiskers represent the standard error of the mean value.

However, regional differences were observed in the average number of DTFVDs, with the following trend: EYS (401 per day) > BS (243 per day) > WYS (175 per day) > CYS (144 per day) > NYS (137 per day). Moreover, the seasonal fluctuations of the number of DTFVDs varied with the location of the water ((c)). The number of DTFVDs in the BS was less than 300 on most days, with minor fluctuations. In the NYS, the average number of DTFVDs was high in autumn (269 per day), yet only reached around 100 per day in other seasons. The fluctuations in the average number of DTFVDs in the WYS exhibited an apparent seasonal bimodal distribution, with the highest in September (368 per day) and the lowest in June (47 per day). Compared with other waters, CYS had the most significant fluctuation in the average number of DTFVDs, with the highest in October (363 per day) and lowest in June (12 per day). In the EYS, between February and October, the average number of DTFVDs increased gradually until it peaked at 575 per day and subsequently declined.
3.3. Seasonal spatial distribution of DTFVDs in the Yellow-Bohai Sea
Similar to the fluctuations in number, the normalized density of DTFVDs in the Yellow-Bohai Sea from 2013–2021 also exhibited an apparent seasonal bimodal distribution: a small expansion in spring and a significant expansion in autumn ((a1–a4)). In terms of the overall distribution, these vessels spread to offshore waters in spring and then shrink to coastal waters in summer. Nighttime fisheries are most widely distributed during autumn, covering the whole Yellow-Bohai Sea with the highest density, while in winter, the majority of DTFVDs are concentrated near the boundaries of the fisheries agreement area (CYS). Regarding regional distribution, nighttime fishery activities in the BS were concentrated in a ‘clump-like’ distribution, while DTFVDs in the NYS exhibited the lowest density distribution. In the EYS, most DTFVDs were observed along the coast and on Jeju Island all year round.
Figure 5. Seasonal normalized density (a1-a4) and radiance (b1-b4) distribution of the DTFVDs in the Yellow-Bohai Sea. The gold dashed lines represent the boundaries of different waters.

Despite the apparent seasonal pattern, the normalized radiance distribution of nighttime fisheries in the Yellow-Bohai Sea is distinct to that of the density ((b1–b4)). In spring, DTFVD normalized radiance was typically low, and only sporadic fishery activities with high-power lights existed in the CYS and the coastal waters of EYS. During summer, the DTFVDs exhibited a ‘strip-like’ distribution and were generally observed in the center of the EYS. In autumn, nighttime fishery activities with high radiance were observed throughout the entire CYS and EYS waters and part of the southern NYS. In winter, however, with the exception of the waters around Jeju Island, the DTFVDs with high-power lights were mainly concentrated in the northern CYS, which is highly consistent with the boundaries of the fisheries agreement area.
In summary, the spatial distribution of DTFVDs in the Yellow-Bohai Sea exhibited an obvious seasonal trend that experienced two key stages:
a spring-summer coastal distribution dominated by DTFVDs with low-power lights;
an autumn-winter offshore distribution dominated by DTFVDs with high-power lights
3.4. Performance of optimal seasonal nighttime fishery distribution model
The Yellow-Bohai Sea's nighttime fisheries activities follow seasonal trends. Hence, the density and radiance distribution models of the DTFVDs were built for the spring−summer, and autumn−winter periods, respectively. The RF model developed using all non-collinear environmental factors (SST, SSC, SSS, SCV, SST_front, and SSS_front) outperformed the other fishery distribution models based on GLM, GAM and RF in terms of logarithmic density and radiance predictions in different seasons. The deviance, a quantitative assessment of the impact of environmental factors on the fishery distribution, explained by the optimal RF models (based on all non-collinear environmental variables) for the density of DTFVDs in spring and summer, the density of DTFVDs in autumn and winter, the radiance of DTFVDs in spring and summer, and the radiance of DTFVDs in autumn and winter was determined as 23.2%, 38.2%, 32.5%, and 45.6%, respectively ( (a1–a2)). Correlation analysis reveals a significant positive correlation (0.6 < R < 0.7, p < 0.0001) between the fishery activity predictions of the four optimal distribution models and the actual values in 2019 ( (b1–b2)).
3.5. Relationship between environmental variables and the seasonal distribution of DTFVDs
The %IncMSE value of the optimal distribution models reveals that the environmental variables exert a similar influence on the density distribution and radiation distribution of nighttime fisheries across different seasons in the Yellow-Bohai Sea. The most important environmental variables affecting DTFVD density and radiance distribution are SST, SSS, SSC, and SST_front, which are significantly more critical than SCV and SSS_front ((a)).
Figure 7. Impact of environmental variables on the seasonal distribution of DTFVDs. (a) Importance of environmental factors in the optimal fishery distribution models in different seasons. (b) Overlap of DTFVDs and the four most critical environmental variables in different seasons. Red dotted lines and gold solid lines represent the location of SST_fronts and the optimum values of critical environmental variables with positive effects on the nighttime fishery distribution, respectively.

The overlap results of DTFVDs and environmental variables show that the seasonal fishery activities in the Yellow-Bohai Sea are highly consistent with the spatial distribution of the optimum values of SST, SSS, Log10 SSC, and SST_front, which have positive effects on the distribution of nighttime fisheries ((b)). The optimum SST, SSS, and SSC values in spring and summer were 15°C, 32 psu and 0.4 mg/m3, and 19°C, 32.5 psu and 0.1 mg/m3 in autumn and winter, respectively. The fishery activities of DTFVDs in spring and summer were generally outside the SST_front, where the SSS is higher than 30 psu and varies rapidly, and the SSC is higher than 0.3 mg/m3. In contrast, the distribution of DTFVDs in autumn and winter was typically inside the SST_front, where the SST exceeds 13°C.
4. Discussion
4.1. Impact of marine dynamic processes on the distribution of nighttime fisheries
The migration and distribution of fish are influenced by the distribution of baits, SST, SSS, and mesoscale oceanographic phenomena such as eddies and fronts, which further affect the spatiotemporal variation of fishery activities (Arostegui et al. Citation2022; Mondal et al. Citation2022a; Mondal et al. Citation2023a; Oh et al. Citation2019; Sihombing et al. Citation2023). In the Yellow-Bohai Sea, the shift of the monsoon drives the seasonal reversal of sea surface wind and current directions, and generates oceanographic features such as YSCWM and YSWC (He, Zhu, and Sheng Citation2022; Zhang et al. Citation2018). By developing a more spatially explicit DTFVD model (), a corresponding seasonal distribution pattern of the quantity variations and spatial distribution of nighttime fisheries was also extracted and mapped in the Yellow-Bohai Sea ( and ). Therefore, seasonal fishery distribution models were constructed by integrating nighttime remote sensing and marine environmental data. Our results indicated that the RF models outperformed the traditional regression approaches such as GLMs and GAMs (). This may be attributed to the advantages of the RF model, including the adoption of a machine-learning approach for nonlinear relationships and avoiding the overfitting of the training data (Zhang et al. Citation2022a). The RF model has also been shown to provide well-controlled variable selection and coefficient estimations, and is becoming increasingly popular in predicting fishery distribution (Xing et al. Citation2022). Therefore, based on the well-performing RF models, this study reveals that marine fronts are also essential for explaining the seasonal distribution of DTFVDs (). Previous studies have demonstrated that the unique oceanographic phenomena in the Yellow-Bohai Sea dominate the seasonal variations of marine environmental factors, including fronts and upwellings (Fan et al. Citation2018; Li et al. Citation2016; Yuan et al. Citation2013). Despite being previously reported, further research is required to determine how seasonal marine fronts affect fish migration and fishery distribution (Diao et al. Citation2021; Zhao et al. Citation2022). The results of this study reveal that the seasonal persistent SST_fronts in the Yellow-Bohai Sea are closely related to the distribution of DTFVDs and the environmental conditions suitable for fishing. This provides a basis for interpreting the spatiotemporal distribution mechanism and promoting spatially refined management of nighttime fisheries based on oceanographic phenomena, ocean mixing, and fish life history ().
Figure 8. Spatiotemporal distribution mechanism of nighttime fisheries in the Yellow-Bohai Sea in (a) spring and summer, and (b) autumn and winter under the influence of the marine environment.

The thermocline is formed in spring and summer with the rise of SST, hindering the water exchange in the Yellow-Bohai Sea. In the nearshore waters, however, the tidal current facilitates the thorough mixing of the YSCWM edge with the surface warm water, forming continuous seasonal upwellings and SST_fronts (Diao et al. Citation2021; Lü et al. Citation2010). As a result of this tidal mixing, water bodies can interchange vertically, allowing cold, nutrient-rich water to rise from the bottom to the surface, improving primary productivity and producing fish bait close to the front areas (Xing et al. Citation2021). With the rise of SST in the Yellow-Bohai Sea, pelagic fish, the main target of nighttime fisheries, began to migrate from the wintering ground to coastal waters affected by diluted water for spawning and breeding in spring. Most hatchlings remain in plankton-rich coastal waters for summer baiting and fattening to obtain the energy required for overwintering (Zhang et al. Citation2020; Zhang et al. Citation2022b). SSS emerged as the most significant driving factor of nocturnal fishery activities in the Yellow-Bohai Sea during this time as migratory fish seek suitable spawning and baiting areas in spring and summer ((a)).
In autumn and winter, the north–south YSMC appears in the middle of the Yellow Sea, driven by the reversal of the sea surface wind and current direction, while the cold currents from the north to the south occur in the coastal areas. As the temperature dropped, YSWC replaced YSCWM as the dominant oceanographic feature in the Yellow-Bohai Sea, and also intersected with coastal cold currents to form SST_fronts, which enhanced ocean mixing, improved primary productivity and promoted the formation of fishing grounds (Lie et al. Citation2019; Tang et al. Citation2004). After autumn, pelagic fish in the Yellow-Bohai Sea began to migrate in clusters from coastal feeding grounds to the south-central Yellow Sea and Jeju Island waters with high water temperatures for overwintering. Therefore, SST became the primary driving factor affecting the distribution of nighttime fisheries during this period ((a)) (Chen et al. Citation2023).
In general, tidal currents drive the ocean mixing of the Yellow-Bohai Sea in spring and summer, forming persistent upwellings and SST_fronts with rich plankton at the edge of the YSCWM. As a result, nighttime fisheries were concentrated on the more landward side of the SST_fronts, which had a lower SSS ((a)). The ocean mixing in autumn and winter was caused by the intersection of cold and warm waters from the coastal current and YSWC, and the ensuing abundant plankton attracted the fishery resources during winter migration. During this time, the nighttime fisheries in the Yellow-Bohai Sea were distributed on the offshore side of the SST_fronts, where the SST was higher than 13°C ((b)). Therefore, seasonal persistent SST fronts can play a crucial environmental role in improving the spatially refined management of offshore fishery resources. Management agencies may concentrate on the location of seasonal SST fronts while patrolling fisheries and conserving resources.
4.2. Impact of human management policies on the distribution of nighttime fisheries
Human activities, such as holidays and management policies, have also been shown to affect the number and distribution of fishing vessels at different spatiotemporal scales. Such policies are receiving more attention from regulatory bodies compared to cultural events due to their role in the conservation of marine ecology and fishery resources (Kroodsma et al. Citation2018; Tian et al. Citation2022b). Therefore, this study explored the impact of fishery policies on the spatiotemporal distribution of DTFVDs in the Yellow-Bohai Sea by assessing the efficiency of the Chinese summer fishing moratorium and the COVID-19 lockdown. Variations in the number of DTFVDs in the Yellow Sea revealed the regional variances in the effects of management measures from surrounding nations. The existence of effective management policies is the main reason for the fluctuations in the number of fishing vessels in different fishing areas under the same marine environmental conditions. Although the quantity and density of nighttime fisheries in the whole Yellow-Bohai Sea decreased significantly in summer, demonstrates that the trends in the quantity changes and distribution of DTFVDs in different waters of the Yellow Sea varied before, during and after the closed fishing season. Under the influence of the Chinese summer fishing moratorium, the number of DTFVDs in the WYS and CYS decreased by 48% and 72%, respectively, and the density distribution also exhibited a general decline. This trend was reversed after the fishing ban, particularly in the CYS water, where the number and density of DTFVDs increased sharply, driven by environmental changes and the overwintering fish migrations in the Yellow-Bohai Sea (). In contrast, under similar environmental conditions, the number of DTFVDs in EYS waters was unaffected by the fishery closure and increased consistently in summer. The high-density fishery distribution also shifted from coastal waters to offshore waters. These observations demonstrate how Chinese fishermen actively participate in management policies. With the exception of several EYS waters (particularly those surrounding Jeju Island), the Yellow-Bohai Sea's summertime fishery intensity is managed effectively, which is conducive to the recovery of offshore fishery resources.
Figure 9. Impact evaluation of Chinese summer fishing moratorium on nighttime fisheries in the Yellow-Bohai Sea. Proportion of changes in the number of DTFVDs (a) in the WYS, (b) in the CYS, and (c) in the EYS. (d) Changes in the distribution of DTFVDs during and before the fishing ban. (e) Changes in the distribution of DTFVDs after and during the fishing ban.

To maintain the sustainable development of offshore fisheries under the threat of climate change and human activity, China has taken a series of practical measures to control the number and catch of fishing vessels (Ministry of Agriculture and Rural Affairs of the People's Republic of China Citation2021). This study has proven the effectiveness of these measures in the Yellow-Bohai Sea. The seasonal fishing ban, however, will decrease the revenue of fishermen, making it challenging to strike a balance between sustainable fisheries and economic growth. Given that fishing efforts are under control and the marine ecology is maturing, eco-friendly nocturnal fisheries can address this problem (Xu et al. Citation2022). Allowing legal night-light fishing vessels equipped with a positioning system to operate during the fishing ban can boost coastal economies and help regulatory agencies combat illegal, unreported and unregulated (IUU) fisheries. The restriction of fishing efforts by the surrounding nations also affects the role of the summer fishery closure in the Yellow-Bohai Sea's sustainable fisheries. reveals that the average density and radiance of DTFVDs in the WYS are much lower than those in the EYS, indicating that less nocturnal fishing may occur in Chinese waters compared to South Korean waters. With the movement of migratory common-pool fishery resources, DTFVDs have gradually become concentrated near the boundary of common fishery areas in autumn and winter. A bilateral cooperation mechanism based on mutual understanding, timely communication and reasonable fishery management can effectively avoid fishery disputes (Xue Citation2005; Yu and Mu Citation2006).
The distribution of nighttime fisheries across different waters is influenced by the duration and effectiveness of management policies. To deal with the initial wave of the epidemic, China and South Korea both implemented quarantine measures in early 2020. These measures were later relaxed once the epidemic was successfully under control (Shim, Tariq, and Chowell Citation2021). This study revealed that COVID-19 largely affected the Yellow Sea's nighttime fisheries from February to April, consistent with the concentrated outbreak of the epidemic in winter. The lockdown in 2020 and 2021 reduced the number of DTFVDs by 11% and 19%, respectively, compared to the pre-lockdown years (2013–2019). In contrast, the density distribution of these vessels declined in large clumps and rose only in rare locations () (Wang et al. Citation2020). Due to the differences in duration and intensity of lockdowns, however, the management policies of neighboring countries exerted different impacts on nighttime fishery activities. COVID-19 first hit Chinese nighttime fisheries, as evidenced by the decline in the number of DTFVDs in NYS, WYS, and CYS in 2020 in February, while this number in EYS started to decline in March. Unlike NYS, WYS, and CYS, the quantity of DTFVDs in EYS increased in March 2021 compared to before the COVID-19 outbreak. The variation in the number of DTFVDs between different waters suggests that the COVID-19's effects on South Korean nighttime fisheries essentially disappeared in early 2021 and the lockdowns started to loosen. Management practices can be assessed using the distribution of DTFVDs as an indicator. The variations in the spatiotemporal distribution of nighttime fisheries in the Yellow Sea under the impact of COVID-19 revealed that management policies with the same purpose have broad prospects in bilateral and even multilateral maritime cooperation, such as establishing common marine protected areas, combating IUU fisheries, and tackling climate change.
4.3. Impact of future climate change on the distribution of nighttime fisheries
Under the threat of climate change and overfishing, the United Nations’ Sustainable Development Goal (SDG) 14, which includes the purpose of maintaining fish stocks at biologically sustainable levels, protecting coastal and marine areas, and eliminating overfishing, has received extensive attention from fishery scientists (Lee et al. Citation2023; Mondal et al. Citation2023c). Research on fishery management and resource conservation based on species distribution models provides motivation for this study aimed at serving fishery decision-making and SDGs by analysing the distribution patterns of nighttime fisheries under the influence of multiple factors (Mondal et al. Citation2023b; Mondal and Lee Citation2023a). Based on the excellent response of Yellow-Bohai Sea’s nighttime fishery activities ( and ), regulatory agencies can timely obtain and manage the spatial distribution of fishing vessels using large-scale monitoring technology. However, this management model can only control the distribution of recognized fisheries and has a certain lag, and thus implementing fishery management and marine protection measures that are climate change-adapted proves to be a challenge. The key to achieving SDG 14 is to combat overfishing by identifying the spatial and temporal distribution of fishery-intensive areas and its relationship with current and future marine environment and climate change. Global warming will cause the distribution range of marine species to expand poleward at about 72 km per decade, and the corresponding fishery activities will also change (Poloczanska et al. Citation2013). The prediction of species distribution models and the refined management of marine fisheries on spatial and temporal scales, which have been widely utilized to predict fish habitat, can protect current and future marine ecosystems from climate risks (Lee et al. Citation2022a; Lee et al. Citation2022b; Zhang et al. Citation2022a). Therefore, in order to promote the spatially refined management of Yellow-Bohai Sea’s nighttime fishery both currently and in the future under SDG 14, this study not only successfully revealed the spatiotemporal variation mechanism of DTFVDs (), but also developed the optimal fishery distribution model based on the predicted density distribution of nighttime fisheries under different RCP scenarios ().
Figure 11. Present and future density distribution predictions of nighttime fisheries in the Yellow-Bohai Sea. (a) Range and period of present Chinese summer fishing moratorium. (b1) Present density distribution predictions of DTFVDs in spring and summer. (b2) Future density distribution predictions of DTFVDs in spring and summer under RCP 2.6. (b3) Future density distribution predictions of DTFVDs in spring and summer under RCP 8.5. (c1) Present density distribution predictions of DTFVDs in autumn and winter. (c2) Future density distribution predictions of DTFVDs in autumn and winter under RCP 2.6. (c3) Future density distribution predictions of DTFVDs in autumn and winter under RCP 8.5.

According to the results of the present prediction, the eastern coastal waters of the Yellow-Bohai Sea are suitable for nighttime fisheries in spring and summer. In contrast, CYS and its surrounding waters are ideal fishing grounds in autumn and winter, particularly around Jeju Island. However, the DTFVD dataset failed to identify apparent nighttime fishery activities in the northeast of the Yellow Sea, possibly because most low-power fishing vessels from North Korea are difficult to identify by optical remote sensing technology (Park et al. Citation2020). Although spring and summer are critical periods to protect the supplementary population of fishery resources, the summer fishery closure has yet to be implemented in the eastern waters of the Yellow-Bohai Sea, which is facing more significant fishing pressure ((a)). Therefore, it is necessary to expand the present marine protection area or the range of summer fishing moratorium, to realize the sustainable development of economic fish species in the Yellow-Bohai Sea. Improving monitoring efficiency and reducing supervision costs can effectively reduce the financial burden and stimulate enthusiasm for the seasonal fishing moratorium (Ding, Lu, and Xue Citation2021). Nighttime remote sensing provides an efficient and convenient means for monitoring and managing the fishery distribution in vast waters, revealing that the suitable fishery areas of DTFVDs in different seasons are highly consistent with the optimum isolines of critical environmental variables ((b1-c1)). Therefore, fishery supervision in spring and summer based on optimum isolines (32 psu and 15°C) will not only help to improve the monitoring efficiency of fishing vessels in the Yellow-Bohai Sea but also aid in advancing the scientific planning of marine protected areas. In autumn and winter, fishery agencies should focus attention on distributing 32.5 psu and 19°C isolines in the waters near Jeju Island to protect the wintering populations.
The distribution of SST ranges appropriate for nighttime fisheries will keep moving northward as a result of future rising greenhouse gas emissions in the Yellow-Bohai Sea ((b2-c2)). According to the RCP 8.5 scenario, the SST of the Yellow-Bohai Sea in spring and summer will exceed 15°C at the end of this century, decreasing spawning and feeding grounds for migratory fish, as well as reducing suitable areas for nighttime fishery. With the expansion of wintering grounds, suitable fishery areas for DTFVDs in autumn and winter will be extended to the coastal waters at the end of this century under the 8.5 scenario. The ideal environment during the early life history stage is the most critical element impacting the recruitment of fish stocks, as opposed to overwintering conditions (Liu et al. Citation2021). On the whole, climate change will pose a greater risk for the suitable spawning grounds and stock recruitments of migratory fish in the Yellow-Bohai Sea, thus affecting the catch and distribution of nighttime fisheries. NTL images can be used to monitor and predict the hotspots of fishery distributions to achieve effective climate-adapted fishery management and development. The findings suggest that lowering emissions will improve food security, stabilize the marine ecology and lessen climatic risks for fish species (Boyce et al. Citation2022). Moreover, scientific fisheries guidance based on NTL imagery and fishery distribution models can reduce fuel consumption and emissions. This will not only help preserve marine biodiversity but also increase the resilience of nighttime fisheries under climate change.
5. Conclusion
In order to comprehensively monitor and evaluate the spatiotemporal distribution of fishing vessels in the Yellow-Bohai Sea, a monthly DTFVD dataset with high spatial and quantitative precision was established using nighttime remote sensing images. An obvious seasonal distribution pattern of DTFVDs in the Yellow-Bohai Sea was revealed, with the spring-summer coastal and autumn-winter offshore distributions dominated by low- and high-power fishing vessels, respectively. The frequency and activity of offshore nighttime fisheries peaked in autumn, covering almost the entire Yellow-Bohai Sea. Marine dynamic processes play a critical role in the ecological consequences and this spatiotemporal distribution pattern. This is highlighted by the integration of large-scale fisheries monitoring and environmental remote sensing data presented in this study. The seasonal distribution of SST, SSS, SSC, and SST_fronts can be used to promote spatially refined monitoring and management of offshore fisheries. This study proves that fishery closures such as summer fishing moratoriums may successfully regulate the number of DTFVDs and support sustainable fisheries in the Yellow-Bohai Sea. Nighttime remote sensing will be irreplaceable in future socioeconomic-related activity predictions and climate-adapted management models in the ocean. The results of this study can aid the spatially refined management, assessment, and conservation of the world's offshore fishing resources. Larger areas and more spatiotemporal big data will be considered in future studies on nighttime fishery conservation and sustainable development.
Author contributions
H. Tian constructed the DTFVD and fishery distribution models and wrote the paper. H. Sun preprocessed the NTL images. J.C. Li guided the oceanographic characteristics of the Yellow-Bohai Sea. Q.W. Xing calculated the oceanographic fronts. Y.J. Tian reviewed and edited the manuscript. Y. Liu conceived the study and analysed and edited the manuscript.
Acknowledgements
We especially thank the Global Fishing Watch for their support of the AIS data.
Disclosure statement
No potential conflict of interest was reported by the author(s).
Data availability statement
The data used in this study are available by contacting the corresponding author.
References
- Arostegui, M. C., P. Gaube, P. A. Woodworth-Jefcoats, D. R. Kobayashi, and C. D. Braun. 2022. “Anticyclonic Eddies Aggregate Pelagic Predators in a Subtropical Gyre.” Nature 609 (7927): 535–540. https://doi.org/10.1038/s41586-022-05162-6.
- Belkin, I. M. 2009. “Rapid Warming of Large Marine Ecosystems.” Progress in Oceanography 81 (1-4): 207–213. https://doi.org/10.1016/j.pocean.2009.04.011.
- Boyce, D. G., D. P. Tittensor, C. Garilao, S. Henson, K. Kaschner, K. Kesner-Reyes, A. Pigot, et al. 2022. “A Climate Risk Index for Marine Life.” Nature Climate Change 12 (9): 854–862. https://doi.org/10.1038/s41558-022-01437-y.
- Chen, G., Y. Liu, Y. Tian, and H. Tian. 2019. “Use of VIIRS DNB Satellite Images to Detect Nighttime Fishing Vessel Lights in Yellow Sea.” Proceedings of the 3rd International Conference on Computer Science and Application Engineering – CSAE, 1-5.
- Chen, Y., X. Shan, H. Gorfine, F. Dai, Q. Wu, T. Yang, Y. Shi, and X. Jin. 2023. “Ensemble Projections of Fish Distribution in Response to Climate Changes in the Yellow and Bohai Seas, China.” Ecological Indicators 146: 109759. https://doi.org/10.1016/j.ecolind.2022.109759.
- Cinner, J. E., I. R. Caldwell, L. Thiault, J. Ben, J. L. Blanchard, M. Coll, A. Diedrich, et al. 2022. “Potential Impacts of Climate Change on Agriculture and Fisheries Production in 72 Tropical Coastal Communities.” Nature Communications 13 (1): 3530. https://doi.org/10.1038/s41467-022-30991-4.
- Cozzolino, E., and C. A. Lasta. 2016. “Use of VIIRS DNB Satellite Images to Detect Jigger Ships Involved in the Illex Argentinus Fishery.” Remote Sensing Applications: Society and Environment 4: 167–178. https://doi.org/10.1016/j.rsase.2016.09.002.
- Diao, X., G. Si, C. Wei, and F. Yu. 2021. “Structure and Formation of the South Yellow Sea Water Mass in the Spring of 2007.” Journal of Oceanology and Limnology 40 (1): 55–65. https://doi.org/10.1007/s00343-021-0206-y.
- Dimarchopoulou, D., A. Dogrammatzi, P. K. Karachle, and A. C. Tsikliras. 2018. “Spatial Fishing Restrictions Benefit Demersal Stocks in the Northeastern Mediterranean Sea.” Scientific Reports 8 (1): 5967. https://doi.org/10.1038/s41598-018-24468-y.
- Ding, L., M. Lu, and Y. Xue. 2021. “Driving Factors on Implementation of Seasonal Marine Fishing Moratorium System in China Using Evolutionary Game.” Marine Policy 133: 104707. https://doi.org/10.1016/j.marpol.2021.104707.
- Elvidge, C. D., K. Baugh, T. Ghosh, M. Zhizhin, F.-C. Hsu, T. Sparks, M. Bazilian, et al. 2022. “Fifty Years of Nightly Global low-Light Imaging Satellite Observations.” Frontiers in Remote Sensing 3, https://doi.org/10.3389/frsen.2022.919937.
- Elvidge, C. D., K. Baugh, M. Zhizhin, and F.-C. Hsu. 2013. “Why VIIRS Data are Superior to DMSP for Mapping Nighttime Lights.” Proceedings of the Asia-Pacific Advanced Network 35 (0): 62–69. https://doi.org/10.7125/APAN.35.7.
- Elvidge, C. D., T. Ghosh, K. Baugh, M. Zhizhin, F.-C. Hsu, N. S. Katada, W. Penalosa, and B. Hung. 2018. “Rating the Effectiveness of Fishery Closures With Visible Infrared Imaging Radiometer Suite Boat Detection Data.” Frontiers in Marine Science 5: 132. https://doi.org/10.3389/fmars.2018.00132.
- Elvidge, C. D., M. Zhizhin, K. Baugh, and F.-C. Hsu. 2015. “Automatic Boat Identification System for VIIRS Low Light Imaging Data.” Remote Sensing 7 (3): 3020–3036. https://doi.org/10.3390/rs70303020.
- Fan, H., X. Wang, H. Zhang, and Z. Yu. 2018. “Spatial and Temporal Variations of Particulate Organic Carbon in the Yellow-Bohai Sea Over 2002-2016.” Scientific Reports 8 (1): 7971. https://doi.org/10.1038/s41598-018-26373-w.
- FAO. 2022. The State of World Fisheries and Aquaculture 2022. Rome: Towards Blue Transformation.
- Guisan, A., Jr., T. C. Edwards, and T. Hastie. 2002. “Generalized Linear and Generalized Additive Models in Studies of Species Distributions: Setting the Scene.” Ecological Modelling 157: 89–100. https://doi.org/10.1016/S0304-3800(02)00204-1.
- Gutierrez, N. L., S. Funge-Smith, G. Gorelli, M. M. Mancha-Cisneros, O. Defeo, A. F. Johnson, and M. C. Melnychuk. 2023. Production and Environmental Interactions of Small-Scale Fisheries. in: FAO, Duke University & WorldFish. 2023. Illuminating Hidden Harvests: The Contributions of Small-Scale Fisheries to Sustainable Development. Rome: FAO; Durham, USA, Duke University; Penang, Malaysia, WorldFish.
- He, B., F. Yan, H. Yu, F. Su, V. Lyne, Y. Cui, L. Kang, and W. Wu. 2021. “Global Fisheries Responses to Culture, Policy and COVID-19 from 2017 to 2020.” Remote Sensing 13 (22), https://doi.org/10.3390/rs13224507.
- He, Z., S. Zhu, and J. Sheng. 2022. “Numerical Study of Circulation and Seasonal Variability in the Southwestern Yellow Sea.” Journal of Marine Science and Engineering 10 (7), https://doi.org/10.3390/jmse10070912.
- Hsu, F.-C., C. D. Elvidge, K. Baugh, M. Zhizhin, T. Ghosh, D. Kroodsma, A. Susanto, et al. 2019. “Cross-Matching VIIRS Boat Detections with Vessel Monitoring System Tracks in Indonesia.” Remote Sensing 11 (9): 995. https://doi.org/10.3390/rs11090995.
- Hurtt, G. C., L. P. Chini, S. Frolking, R. A. Betts, J. Feddema, G. Fischer, J. P. Fisk, et al. 2011. “Harmonization of Land-use Scenarios for the Period 1500–2100: 600 Years of Global Gridded Annual Land-use Transitions, Wood Harvest, and Resulting Secondary Lands.” Climatic Change 109 (1-2): 117–161. https://doi.org/10.1007/s10584-011-0153-2.
- James, M., T. Mendo, E. L. Jones, K. Orr, A. McKnight, and J. Thompson. 2018. “AIS Data to Inform Small Scale Fisheries Management and Marine Spatial Planning.” Marine Policy 91: 113–121. https://doi.org/10.1016/j.marpol.2018.02.012.
- Jin, X., X. Zhao, T. Meng, and Y. Cui. 2005. Biological Resources and Habitat of the Yellow-Bohai Sea. Beijing, People’s Republic of China: Science Press. in Chinese.
- Kroodsma, D. A., J. Mayorga, T. Hochberg, N. A. Miller, K. Boerder, F. Ferretti, A. Wilson, et al. 2018. “Tracking the Global Footprint of Fisheries.” Science 359: 904–908. https://doi.org/10.1126/science.aao5646.
- Lee, M.-A., S. Mondal, S.-Y. Teng, M.-L. Nguyen, P. Lin, J.-H. Wu, and B. K. Mondal. 2023. “Fishery-Based Adaption to Climate Change: The Case of Migratory Species Flathead Grey Mullet (Mugil cephalus L.) in Taiwan Strait, Northwestern Pacific.” Peer J 11, https://doi.org/10.7717/peerj.15788.
- Lee, M.-A., S. Mondal, J.-H. Wu, and M. Boas. 2022a. “Total Catch Variability in the Coastal Waters of Taiwan in Relation to Climatic Oscillations and Possible Impacts.” Journal of Taiwan Fisheries Society 49 (2): 127–143. https://doi.org/10.29822/JFST.202206_49(2).0006.
- Lee, M.-A., S. Mondal, J.-H. Wu, Y.-H. Huang, and M. Boas. 2022b. “Cyclic Variation in Fishing Catch Rates -Influenced by Climatic Variability in the Waters Around Taiwan.” Journal of Taiwan Fisheries Society 49 (2): 113–125. https://doi.org/10.29822/JFST.202206_49(2).0005.
- Levin, N., C. C. M. Kyba, Q. Zhang, A. Sánchez de Miguel, M. O. Román, X. Li, B. A. Portnov, et al. 2020. “Remote Sensing of Night Lights: A Review and an Outlook for the Future.” Remote Sensing of Environment 237: 111443. https://doi.org/10.1016/j.rse.2019.111443.
- Li, G., X. Chen, L. Lei, and W. Guan. 2014. “Distribution of Hotspots of Chub Mackerel Based on Remote-Sensing Data in Coastal Waters of China.” International Journal of Remote Sensing 35 (11-12): 4399–4421. https://doi.org/10.1080/01431161.2014.916057.
- Li, J., G. Li, J. Xu, P. Dong, L. Qiao, S. Liu, P. Sun, and Z. Fan. 2016. “Seasonal Evolution of the Yellow Sea Cold Water Mass and its Interactions with Ambient Hydrodynamic System.” Journal of Geophysical Research: Oceans 121 (9): 6779–6792. https://doi.org/10.1002/2016JC012186.
- Liaw, A., and M. Wiener. 2002. “Classification and Regression by Random Forest.” R News 2: 18–22.
- Lie, H.-J., K.-H. Oh, C.-H. Cho, and J.-H. Moon. 2019. “Wintertime Large Temperature Inversions in the Yellow Sea Associated With the Cheju and Yellow Sea Warm Currents.” Journal of Geophysical Research: Oceans 124 (7): 4856–4874. https://doi.org/10.1029/2019JC015180.
- Liu, Y., S.-I. Saitoh, T. Hirawake, H. Igarashi, and Y. Ishikawa. 2015. “Detection of Squid and Pacific Saury Fishing Vessels Around Japan Using VIIRS Day/Night Band Image.” Proceedings of the Asia-Pacific Advanced Network 39: 28–39. https://doi.org/10.7125/APAN.39.3.
- Liu, Y., X. Xia, Y. Tian, I. D. Alabia, S. Ma, P. Sun, and S.-I. Saitoh. 2021. “Influence of Spawning Ground Dynamics on the Long-Term Abundance of Japanese Flying Squid (Todarodes Pacificus) Winter Cohort.” Frontiers in Marine Science 8: 659816. https://doi.org/10.3389/fmars.2021.659816.
- Lü, X., F. Qiao, C. Xia, G. Wang, and Y. Yuan. 2010. “Upwelling and Surface Cold Patches in the Yellow Sea in Summer: Effects of Tidal Mixing on the Vertical Circulation.” Continental Shelf Research 30 (6): 620–632. https://doi.org/10.1016/j.csr.2009.09.002.
- Ma, S., B. Kang, J. Li, P. Sun, Y. Liu, Z. Ye, and Y. Tian. 2022. “Climate Risks to Fishing Species and Fisheries in the China Seas.” Science of the Total Environment 857 (Pt 1): 159325. https://doi.org/10.1016/j.scitotenv.2022.159325.
- March, D., K. Metcalfe, J. Tintore, and B. J. Godley. 2021. “Tracking the Global Reduction of Marine Traffic During the COVID-19 Pandemic.” Nature Communications 12 (1): 2415. https://doi.org/10.1038/s41467-021-22423-6.
- Melnychuk, M. C., H. Kurota, P. M. Mace, M. Pons, C. Minto, G. C. Osio, O. P. Jensen, et al. 2021. “Identifying Management Actions That Promote Sustainable Fisheries.” Nature Sustainability 4 (5): 440–449. https://doi.org/10.1038/s41893-020-00668-1.
- Miller, S. D., S. P. Mills, C. D. Elvidge, D. T. Lindsey, T. F. Lee, and J. D. Hawkins. 2012. “Suomi Satellite Brings to Light a Unique Frontier of Nighttime Environmental Sensing Capabilities.” Proceedings of the National Academy of Sciences 109 (39): 15706–15711. https://doi.org/10.1073/pnas.1207034109.
- Miller, S., W. Straka, S. Mills, C. Elvidge, T. Lee, J. Solbrig, A. Walther, A. Heidinger, and S. Weiss. 2013. “Illuminating the Capabilities of the Suomi National Polar-Orbiting Partnership (NPP) Visible Infrared Imaging Radiometer Suite (VIIRS) Day/Night Band.” Remote Sensing 5 (12): 6717–6766. https://doi.org/10.3390/rs5126717.
- Ministry of Agriculture and Rural Affairs of the People’s Republic of China. 2021. “Notification of the Ministry of Agriculture and Rural Affairs on printing and distributing the 14th Five-Year National Fishery Development Plan.” Accessed 6 March 2022. http://www.moa.gov.cn/govpublic/YYJ/202201/t20220106_6386439.htm.
- Ministry of Foreign Affairs of the People’s Republic of China. 2020. “Fisheries Agreement between the Government of the People’s Republic of China and the Government of the Republic of Korea.” Accessed June 18, 2021. https://www.mfa.gov.cn/web/wjb_673085/zfxxgk_674865/gknrlb/tywj/tyqk/200904/t20090409_9276864.shtml.
- Molnar, C. 2018. “iml: An R Package for Interpretable Machine Learning.” Journal of Open Source Software 3 (26), https://doi.org/10.21105/joss.00786.
- Mondal, S., Y.-C. Lan, M.-A. Lee, Y.-C. Wang, B. Semedi, and W.-Y. Su. 2022a. “Detecting the Feeding Habitat Zone of Albacore Tuna (Thunnus Alalunga) in the Southern Indian Ocean Using Multisatellite Remote Sensing Data.” Journal of Marine Science and Technology 29 (6): 794–807. https://doi.org/10.51400/2709-6998.2559.
- Mondal, S., and M.-A. Lee. 2023a. “Habitat Modeling of Mature Albacore (Thunnus Alalunga) Tuna in the Indian Ocean.” Frontiers in Marine Science 10, https://doi.org/10.3389/fmars.2023.1258535.
- Mondal, S., and M.-A. Lee. 2023b. “Long-Term Observations of Sea Surface Temperature Variability in the Gulf of Mannar.” Journal of Marine Science and Engineering 11 (1), https://doi.org/10.3390/jmse11010102.
- Mondal, S., M.-A. Lee, Y.-K. Chen, and Y.-C. Wang. 2023b. “Ensemble Modeling of Black Pomfret (Parastromateus Niger) Habitat in the Taiwan Strait Based on Oceanographic Variables.” PeerJ 11, https://doi.org/10.7717/peerj.14990.
- Mondal, S., M.-A. Lee, Y.-C. Wang, and B. Semedi. 2021a. “Long-term Variation of sea Surface Temperature in Relation to sea Level Pressure and Surface Wind Speed in Southern Indian Ocean.” Journal of Marine Science and Technology 29: 784–794. https://doi.org/10.51400/2709-6998.2558.
- Mondal, S., M.-A. Lee, J.-S. Weng, K. E. Osuka, Y. K. Chen, and A. Ray. 2023a. “Seasonal Distribution Patterns of Scomberomorus Commerson in the Taiwan Strait in Relation to Oceanographic Conditions: An Ensemble Modeling Approach.” Marine Pollution Bulletin 197, https://doi.org/10.1016/j.marpolbul.2023.115733.
- Mondal, S., A. Ray, M.-A. Lee, and M. Boas. 2023c. “Projected Changes in Spawning Ground Distribution of Mature Albacore Tuna in the Indian Ocean Under Various Global Climate Change Scenarios.” Journal of Marine Science and Engineering 11 (8), https://doi.org/10.3390/jmse11081565.
- Mondal, S., A. H. Vayghan, M.-A. Lee, Y.-C. Wang, and B. Semedi. 2021b. “Habitat Suitability Modeling for the Feeding Ground of Immature Albacore in the Southern Indian Ocean Using Satellite-Derived Sea Surface Temperature and Chlorophyll Data.” Remote Sensing 13 (14), https://doi.org/10.3390/rs13142669.
- Mondal, S., Y.-C. Wang, M.-A. Lee, J.-S. Weng, and B. K. Mondal. 2022b. “Ensemble Three-Dimensional Habitat Modeling of Indian Ocean Immature Albacore Tuna (Thunnus Alalunga) Using Remote Sensing Data.” Remote Sensing 14 (20), https://doi.org/10.3390/rs14205278.
- Moss, R. H., J. A. Edmonds, K. A. Hibbard, M. R. Manning, S. K. Rose, D. P. van Vuuren, T. R. Carter, et al. 2010. “The Next Generation of Scenarios for Climate Change Research and Assessment.” Nature 463 (7282): 747–756. https://doi.org/10.1038/nature08823.
- Natural Resources of the People’s Republic of China. 2012. “National Marine Function Zoning (2011-2020).” Accessed 18 June 2021. http://f.mnr.gov.cn/201806/t20180621_1830454.html.
- Oh, Y., D.-W. Kim, Y.-H. Jo, J.-D. Hwang, and C.-Y. Chung. 2019. “Spatial Variability of Fishing Grounds in Response to Oceanic Front Changes Detected by Multiple Satellite Measurements in the East (Japan) sea.” International Journal of Remote Sensing 41 (15): 5884–5904. https://doi.org/10.1080/01431161.2019.1685722.
- Park, J., J. Lee, K. Seto, T. Hochberg, B. A. Wong, N. A. Miller, K. Takasaki, et al. 2020. “Illuminating Dark Fishing Fleets in North Korea.” Science Advances 6 (30): eabb1197. https://doi.org/10.1126/sciadv.abb1197.
- Poloczanska, E. S., C. J. Brown, W. J. Sydeman, W. Kiessling, D. S. Schoeman, P. J. Moore, K. Brander, et al. 2013. “Global Imprint of Climate Change on Marine Life.” Nature Climate Change 3 (10): 919–925. https://doi.org/10.1038/nclimate1958.
- Richerson, K., J. Leonard, and D. S. Holland. 2018. “Predicting the Economic Impacts of the 2017 West Coast Salmon Troll Ocean Fishery Closure.” Marine Policy 95: 142–152. https://doi.org/10.1016/j.marpol.2018.03.005.
- Roberts, J. J., B. D. Best, D. C. Dunn, E. A. Treml, and P. N. Halpin. 2010. “Marine Geospatial Ecology Tools: An Integrated Framework for Ecological Geoprocessing with ArcGIS, Python, R, MATLAB, and C++.” Environmental Modelling & Software 25 (10): 1197–1207. https://doi.org/10.1016/j.envsoft.2010.03.029.
- Rogers, M. A., S. D. Miller, C. J. Seaman, J. Torres, D. Hillger, E. Szoke, and W. E. Line. 2023. “VIIRS After 10 Years – A Perspective on Benefits to Forecasters and End-Users.” Remote Sensing 15 (4), https://doi.org/10.3390/rs15040976.
- Shim, E., A. Tariq, and G. Chowell. 2021. “Spatial Variability in Reproduction Number and Doubling Time Across two Waves of the COVID-19 Pandemic in South Korea, February to July, 2020.” International Journal of Infectious Diseases 102: 1–9. https://doi.org/10.1016/j.ijid.2020.10.007.
- Sihombing, R. I., A. Ray, S. Mondal, and M.-A. Lee. 2023. “Habitat Modelling of Escolar Fish (Lepidocybium Flavobrunneum, Smith 1843) in the Southwestern Indian Ocean Using Remote Sensing Data.” International Journal of Remote Sensing, 1–20. https://doi.org/10.1080/01431161.2023.2235644.
- Smith, A. M., C. Capinha, and A. M. Kramer. 2022. “Predicting Species Distributions with Environmental Time Series Data and Deep Learning.” bioRxiv. https://doi.org/10.1101/2022.10.26.513922.
- Straka, W., C. Seaman, K. Baugh, K. Cole, E. Stevens, and S. Miller. 2015. “Utilization of the Suomi National Polar-Orbiting Partnership (NPP) Visible Infrared Imaging Radiometer Suite (VIIRS) Day/Night Band for Arctic Ship Tracking and Fisheries Management.” Remote Sensing 7 (1): 971–989. https://doi.org/10.3390/rs70100971.
- Sun, C., Y. Liu, S. Zhao, and S. Jin. 2020. “Estimating Offshore oil Production Using DMSP-OLS Annual Composites.” ISPRS Journal of Photogrammetry and Remote Sensing 165: 152–171. https://doi.org/10.1016/j.isprsjprs.2020.05.019.
- Tang, D. L., I. H. Ni, F. E. Müller-Karger, and I. S. Oh. 2004. “Monthly Variation of Pigment Concentrations and Seasonal Winds in China's Marginal Seas.” Hydrobiologia 511 (1): 1–15. https://doi.org/10.1023/B:HYDR.0000014001.43554.6f.
- Tian, H., Y. Liu, Y. Tian, I. D. Alabia, Y. Qin, H. Sun, J. Li, S. Ma, and S.-I. Saitoh. 2022a. “A Comprehensive Monitoring and Assessment System for Multiple Fisheries Resources in the Northwest Pacific Based on Satellite Remote Sensing Technology.” Frontiers in Marine Science 9: 808282. https://doi.org/10.3389/fmars.2022.808282.
- Tian, H., Y. Liu, Y. Tian, Y. Jing, S. Liu, X. Liu, and Y. Zhang. 2022b. “Advances in the use of Nighttime Light Data to Monitor and Assess Coastal Fisheries Under the Impacts of Human Activities and Climate and Environmental Changes: A Case Study in the Beibu Gulf.” Marine Policy 144, https://doi.org/10.1016/j.marpol.2022.105227.
- Tigchelaar, M., J. Leape, F. Micheli, E. H. Allison, X. Basurto, A. Bennett, S. R. Bush, et al. 2022. “The Vital Roles of Blue Foods in the Global Food System.” Global Food Security 33, https://doi.org/10.1016/j.gfs.2022.100637.
- VanDerWal, Jeremy, H. T. Murphy, A. S. Kutt, G. C. Perkins, B. L. Bateman, J. J. Perry, and A. E. Reside. 2013. “Focus on Poleward Shifts in Species’ Distribution Underestimates the Fingerprint of Climate Change.” Nature Climate Change 3: 239–243. https://doi.org/10.1038/nclimate1688.
- Vayghan, A. H., M.-A. Lee, J.-S. Weng, S. Mondal, C.-T. Lin, and Y.-C. Wang. 2020. “Multisatellite-Based Feeding Habitat Suitability Modeling of Albacore Tuna in the Southern Atlantic Ocean.” Remote Sensing 12: 2515. https://doi.org/10.3390/rs12162515.
- Wang, C., P. W. Horby, F. G. Hayden, and G. F. Gao. 2020. “A Novel Coronavirus Outbreak of Global Health Concern.” The Lancet 395 (10223): 470–473. https://doi.org/10.1016/S0140-6736(20)30185-9.
- Wood, S. N. 2006. “Generalized Additive Models: An Introduction with R.” Journal of Statistical Software 16.
- Xing, Q., H. Yu, Y. Liu, J. Li, Y. Tian, A. Bakun, C. Cao, H. Tian, and W. Li. 2022. “Application of a Fish Habitat Model Considering Mesoscale Oceanographic Features in Evaluating Climatic Impact on Distribution and Abundance of Pacific Saury (Cololabis Saira).” Progress in Oceanography 201: 102743. https://doi.org/10.1016/j.pocean.2022.102743.
- Xing, Q., H. Yu, H. Wang, and S.-I. Ito. 2023a. “An Improved Algorithm for Detecting Mesoscale Ocean Fronts from Satellite Observations: Detailed Mapping of Persistent Fronts Around the China Seas and Their Long-Term Trends.” Remote Sensing of Environment 294, https://doi.org/10.1016/j.rse.2023.113627.
- Xing, Q., H. Yu, H. Wang, and H. Yu. 2023b. “A Sliding-Window-Threshold Algorithm for Identifying Global Mesoscale Ocean Fronts from Satellite Observations.” Progress in Oceanography 216, https://doi.org/10.1016/j.pocean.2023.103072.
- Xing, Q., H. Yu, H. Yu, H. Wang, S.-I. Ito, and C. Yuan. 2021. “Evaluating the Spring-Neap Tidal Effects on Chlorophyll-a Variations Based on the Geostationary Satellite.” Frontiers in Marine Science 8, https://doi.org/10.3389/fmars.2021.758538.
- Xu, L., P. Song, Y. Wang, B. Xie, L. Huang, Y. Li, X. Zheng, and L. Lin. 2022. “Estimating the Impact of a Seasonal Fishing Moratorium on the East China Sea Ecosystem from 1997 to 2018.” Frontiers in Marine Science 9 (865645). https://doi.org/10.3389/fmars.2022.865645.
- Xue, G. 2005. “Bilateral Fisheries Agreements for the Cooperative Management of the Shared Resources of the China Seas: A Note.” Ocean Development & International Law 36 (4): 363–374. https://doi.org/10.1080/00908320500308767.
- Yu, Y., and Y. Mu. 2006. “The new Institutional Arrangements for Fisheries Management in Beibu Gulf.” Marine Policy 30 (3): 249–260. https://doi.org/10.1016/j.marpol.2004.12.006.
- Yuan, D., Y. Li, F. Qiao, and W. Zhao. 2013. “Temperature Inversion in the Huanghai Sea Bottom Cold Water in Summer.” Acta Oceanologica Sinica 32 (3): 42–47. https://doi.org/10.1007/s13131-013-0287-3.
- Zhang, R., Y. Liu, H. Tian, S. Liu, K. Zu, and X. Xia. 2022a. “Impact of Climate Change on Long-Term Variations of Small Yellow Croaker (Larimichthys Polyactis) Winter Fishing Grounds.” Frontiers in Marine Science 9, https://doi.org/10.3389/fmars.2022.915765.
- Zhang, Z., F. Qiao, J. Guo, and B. Guo. 2018. “Seasonal Changes and Driving Forces of Inflow and Outflow Through the Bohai Strait.” Continental Shelf Research 154: 1–8. https://doi.org/10.1016/j.csr.2017.12.012.
- Zhang, W., Z. Ye, Y. Tian, H. Yu, S. Ma, P. Ju, and Y. Watanabe. 2022b. “Spawning Overlap of Japanese Anchovy Engraulis Japonicus and Japanese Spanish Mackerel Scomberomorus Niphonius in the Coastal Yellow Sea: A Prey–Predator Interaction.” Fisheries Oceanography 31 (4): 456–469. https://doi.org/10.1111/fog.12595.
- Zhang, W., H. Yu, Z. Ye, Y. Tian, Y. Liu, J. Li, Q. Xing, and Y. Jiang. 2020. “Spawning Strategy of Japanese Anchovy Engraulis Japonicus in the Coastal Yellow Sea: Choice and Dynamics.” Fisheries Oceanography 30 (4): 366–381. https://doi.org/10.1111/fog.12523.
- Zhao, L., D. Yang, R. Zhong, and X. Yin. 2022. “Interannual, Seasonal, and Monthly Variability of Sea Surface Temperature Fronts in Offshore China from 1982–2021.” Remote Sensing 14 (21), https://doi.org/10.3390/rs14215336.
- Zhao, Y., K. Zhang, X. Xu, H. Shen, X. Zhu, Y. Zhang, Y. Hu, and G. Shen. 2020. “Substantial Changes in Nitrogen Dioxide and Ozone After Excluding Meteorological Impacts During the COVID-19 Outbreak in Mainland China.” Environmental Science & Technology Letters 7 (6): 402–408. https://doi.org/10.1021/acs.estlett.0c00304.
- Zhu, Y. 2009. “Research on the Effects of China’s Summer Fishing Moratorium - A Perspective of Institutional Analysis.” (Ph.D. Thesis). Fisheries College, Ocean University of China. (in Chinese with English Abstract).
Appendices
Figure A1. Relationships between the moon phase index and (a) average detection number, and (b) average detection threshold of DTFVDs in the Yellow-Bohai Sea in 2013 (excluding the data during closed fishing season).

Table A1. Closed seasons of different fishing nets in the Yellow-Bohai Sea after 2013.