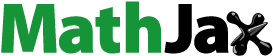
ABSTRACT
Assessing ecosystem services values (ESV) within land use/land cover (LULC) changes is crucial for promoting human well-being and sustainable development of regional ecosystems. Yet, the spatial relationship between LULC changes and ecosystem services is still unclear in Yemen. This study aimed to assess the impacts of LULC changes on ESV in Ibb City, over three decades (1990–2020), and to predict the ESV changes in 2050. The hybrid land use classification technique for classifying Landsat images, the CA-Markov model for LULC prediction, and the benefit transfer method (BTM) for assessment ESV were employed. Our findings revealed that there was a continuous increase in built-up areas and barren land, with a decrease in cultivated land and grassland, which are predicted to continue for the next 30 years. Consequently, the total ESV has decreased from US$ 68.5 × 106 in 1990 to US$ 65.2 × 106 in 2020 and is expected to further reduce to US$ 61.2 × 106 by 2050, reflecting the impact of urban expansion and socio-economic activities on ESV. The study provides insights for future ESV monitoring, which will contribute to the formulation of effective land-use strategies for more sustainable ecosystem services, particularly in rapidly urbanizing data-limited regions.
1. Introduction
Ecosystem services (ES) refer to the functions and activities of natural ecosystems and their organisms that provide human well-being and sustainable development; or are the benefits that humans obtain directly or indirectly through ecosystems (such as services of provisioning, regulatory, cultural, and supporting) (Costanza et al. Citation1997; Liu et al. Citation2019; Msofe et al. Citation2020). Consequently, the ES not only furnishes material services to humans such as food, water, and various raw materials but also provides non-material services such as climate and gas regulation, etc., which support system for sustaining life and establishing ecological conditions for human well-being (M. Das and Das Citation2019; Morshed et al. Citation2022). Globally, the values of Ecosystem services have deteriorated dramatically over time and across different geographical areas (M. Das and Das Citation2019). According to (Costanza et al. Citation1997; Citation2014), the total value of global ES during the period 2007–2011 was US$145–125 trillion per year. From this perspective, land use/land cover (LULC) change is the first and direct driving force of ES, and its change process plays a crucial role in changing the functions of ES (Jiang, Fu, and Lü Citation2020; Msofe et al. Citation2020). Thus, the study of the impact of LULC change on changing ecosystem service values (ESV) is of great significance for understanding regional environmental changes, maintaining ecological balance, and promoting the coordinated development of regional economy and environment (N. Li et al. Citation2021). In recent years, scholars have gradually begun to strengthen research in this area and achieved a series of research results, making the research on the value of ES develops from a static to a dynamic direction (Hasan et al. Citation2020). However, studies have shown that in the historical period, LULC changes were mostly caused by human socioeconomic activities, thus leading to changes in the value of ecosystem service (L. Gao et al. Citation2019). Therefore, as the global environment deteriorates and people's awareness of environmental protection increases, scholars and research organizations globally are increasingly aware of the impact of human activities on environmental changes (B. Shrestha, Ye, and Khadka Citation2019).
In the literature, diverse methodologies have been developed for the assessment of terrestrial ES. These approaches can be broadly classified into four groups including (a) revealed preference techniques; (b) stated preference methodologies; (c) cost-based methodologies; and (d) the benefits transfer method (BTM) (Chopra, Khuman, and Dhyani Citation2022). These methods differ in the source of information and resources (monetary and time) required. Among these methods, the BTM is the most widely employed approach on a global scale for valuing ES because of its feasibility and simplicity (M. Shrestha and Acharya Citation2021). Most notably, these researchers categorized the global biosphere was first classified into 16 sub-ecosystems and 17 ecosystem service functions, and the value of each ES was evaluated by BTM (Chatterjee et al. Citation2022). Although Costanza's estimates of ESV are considered to be rough approximations, they remain the most comprehensive and widely recognized set of estimates available for measuring changes in the value of services provided by various ecosystems (Belay, Melese, and Senamaw Citation2022) for ecosystem service assessment research. Following this groundbreaking estimation of global ESV, scholarly interest in the valuation of ES has witnessed marked attention on an international scale among researchers (Woldeyohannes et al. Citation2020). For instance, in Bangladesh, (Gashaw et al. Citation2018; Haque et al. Citation2023) evaluated the impact of LULC changes on ESV; in China, Liu et al. (Citation2019)Assessed the Impacts of urbanization-induced land-use changes on ESV; In India, (Basu et al. Citation2023; Chatterjee et al. Citation2022; M. Das and Das Citation2019; M. Das, Das, and Pandey Citation2022) analysis of ESV Based on LULC change; and in Ethiopia, (Gashaw et al. Citation2018; Tolessa, Senbeta, and Kidane Citation2017) monitored the impact of LULC change on ESV. In addition, many scholars have designed a variety of models to predict the future impact of LULC and ESV at different scales. For example, land change modeler in TerraSet (known as IDRISI) (Taiwo et al. Citation2023), machine learning algorithms (A. Al Kafy, Saha, et al. Citation2022), the Cellular Automata (CA) algorithms (Rahaman, Kafy, Faisal, et al. Citation2022), Multi-Layer Perceptron Neural Network and Markov Chain (MLPNN-M) (Basu et al. Citation2023; A. Wang et al. Citation2023), model future land use simulation (FLUS) model (Hou et al. Citation2021), artificial neural networks (ANNs) (Morshed et al. Citation2022), The Cellular Automata-Artificial Neural Network (CA-ANN) (A. Wang et al. Citation2023; A. Al Kafy et al. Citation2021b), RapidEye images (A. Al Kafy et al. Citation2022), and Markov chain and cellular automata models (J. Li et al. Citation2019; F. Zhang, Yushanjiang, and Jing Citation2019), etc. These models provide a strong foundation for evaluating the impacts on EVS of current and future LULC, as the input data requirements and simulation objects are different, the application effects have their advantages and disadvantages (A. Al Kafy et al. Citation2021a). The Markov model mainly has advantages at the regional macro scale, and the cellular automata (CA) model has advantages at the spatial unit scale (Y. Zhang et al. Citation2021). As a result, the integration of CA with a Markov model, commonly denoted as CA-Markov, within a GIS framework, has significantly minimized simulation inaccuracies (Rahaman, Kafy, Faisal, et al. Citation2022). This is the rationale behind employing this model in the present study for simulating perspective LULC changes and their associated ESV.
The majority of studies adopt value coefficients to approximate the ESV and create maps of their distribution through a combination of BTM with remote sensing and GIS estimation techniques (Liu et al. Citation2019). This approach is feasible for estimating ESV and suggests management alternatives for areas with a lack of data (Hoque et al. Citation2022). Furthermore, the Economics of Ecosystems and Biodiversity (TEEB) database has been established to identify value coefficients for different biomes from various regions worldwide, serving as the foundation for developing these coefficients (Msofe et al. Citation2020; Morshed et al. Citation2022). The TEEB database offers valuable insights into the valuation of ES across diverse ecosystems (Kornatowska and Sienkiewicz Citation2018). Despite efforts to estimate ESV for regions with data scarcity, there are few studies on the evaluation of ESV related to the dynamics of LULC change in the Middle East (Bindajam et al. Citation2021). In Yemen, rapid population growth and economic development are a problem of concern, where LULC types are transforming into built-up areas and influencing degradation of ecology and environment, which hinders the ability to Maintain and manage ecosystem services (Dammag, Jian, et al. Citation2023). One of the underlying factors for the degradation of Yemen’s ecosystems is the lack of integration of ecosystems’ values in decision-making at national and local levels, lack of acknowledgment that the ecosystem is a closed system, and that ecosystem deprecation feedback into slow economic growth (Masike Citation2014). Consequently, this study has entailed two areas of focus in this field. The primary focus has been given to assessing the impacts of historical LULC changes on ESV than projected changes in ESV in the future based on the predicted LULC pattern under different scenarios.
One such urban center grappling with the complexities of urbanization is Ibb city, which is located in the southwest highlands of Yemen, is the tourist capital of Yemen, and is the third-largest city of the Republic in terms of population (Al-Darwish et al. Citation2018). In recent years, the city has witnessed an influx of internally displaced persons (IDPs) as a result of recent conflicts in Yemen. The continuous intensification of LULC change towards agricultural land and urbanization due to population pressure, government policies, and climate change have led to ecological problems in the region, such as food production, biological control, the decrease in grassland coverage, and the degree of desertification (Dammag et al. Citation2023). Changes in Ibb's ecosystem and its services are closely related to maintaining regional ecological security and sustainable economic and social development (Dammag et al. Citation2023a). Rapid urbanization and increasing populations have led to fundamental changes in land use, which have widespread impacts on ES in the region. Therefore, understanding the impacts of LULC changes on ESV in Ibb City is essential to give public awareness and indicate policy directions about the loss/gain in ecosystem services. Previously, there is no study carried out in the study region concerning the impacts of LULC changes on ESV. Therefore, this study is considered crucial to reveal the detection impacts of LULC changes on ESV over the past and next three, which provides a basis for sustainable development and management of the ecology and environment in the study area. To achieve this, (1) to examine the temporal and spatial dynamics patterns of LUCC in the study area during the whole study period; (2) to estimate the impacts of LULC changes on ESV in monetary values in the study area over 1990–2020 periods. in addition, the study will predict the ESV in the future (2035, 2050) using the CA-Markov model to predict the trend and spatial structure of LULC classes; (3) to explore the changes in individual ESV due to the effects of dynamics in each LULC class; and (4) to assess the impact of LULC change on ESV through determining the elasticity of ESV across the entire period. This study offers a distinctive approach to estimating local-level ESV by leveraging the knowledge of local experts instead of relying solely on global databases, which may yield inaccurate or exaggerated results. In addition, the discussion offers suggestions for economic development and balancing regional differences of ESV that can inform decision-making processes and be utilized in other data-scarce regions and facing similar conditions.
2. Data and methods
2.1. Study area
The study area, Ibb city, is located in the southwestern highlands of central Yemen. It is the tourist capital of Yemen and is about 193 km away from Sana’a (the capital of Yemen) (Dammag et al. Citation2023). It is located between 13°54′ and 14° 73′ N latitude and 43° 08′ and 45° 21′ E longitude, as depicted in . Its importance is due to its role as a commercial and distribution center of regional importance (Al Sabahi et al. Citation2009). The area of Ibb city is about 5300 km2 distributed over twenty districts, and its population is estimated at 3,243,580 people, or 10.8% of the total population of Yemen, at an annual rate of 2.47% (Dammag et al. Citation2023b). It is the third-largest city in terms of population and is inhabited by about 78% rural population. Furthermore, it is known as one of the most productive food warehouses in the country, with the city’s agricultural production accounting for (5.6%) of Yemen’s total production, making it significant not just ecologically, but also socio-economically (Varisco Citation2018). In recent years, the city has suffered unexpected eco-environment degradation as a result of rapid urbanization, population growth, and the influx of IDPs. Due to the mountainous areas surrounding the city, as well as the volcanic soil and heavy amounts of rain throughout the year, the city produces wheat, coffee, fruits, and vegetables, and is famous for its simple handicrafts (Ward Citation2014). The diversity of agricultural products is due to the heavy rainfall throughout the year, which makes the soil fertile (Dammag et al. Citation2023). Therefore, Ibb City holds significant importance as an agricultural hub and primary source of food for the inhabitants of the neighboring regions (Dammag et al. Citation2023a). The city's lands contain some minerals, the most important of which are clay minerals used in the manufacture of cement and refractory bricks, mineral (zeolite) used in the manufacture of detergents, basalt used in the manufacture of building stone, and the mineral used in the manufacture of ornamental stones (Berghof foundation Citation2020).
Due to Ibb city location at an altitude of around 1,900 meters above sea level, it relishes a moderate climate throughout the year, and it is accompanied by heavy rains with coldness as a result of the monsoon rains saturated with water that blows from the southeast and southwest, as the annual precipitation rate is more than (1000 mm) on the western and southern mountainous heights at an altitude of (1500 m above sea level), with an annual mean temperature 18 ◦C (Https://weatherspark.com 2020). Geologically, it’s high in the southwest and low in the northeast, with undulating landforms, forming three types of terrain: middle mountains, low mountains, and river valleys (NIC Citation2015). The natural vegetation in the region depends on agricultural terraces, followed by marginal grasslands occasionally with trees (CEPF Citation2012). The area above 1500 m above sea level accounts for 49.40% of the administrative area. Most of these valley’s flow into the Tihama Plain in the west, while the valleys east of Ibb flow into the Gulf of Aden (Ward Citation2014).
2.2. Data acquisition and processing
The overall approach employed in this study () involved estimating ESV based on LULC change. Thus, LULC is the core data for this study, and the Landsat images were collected from the United States Geological Survey (USGS) official website (https://earthexplorer.usgs.gov)to elucidate LULC dynamics. Landsat images were comprised of Landsat TM (5) from 1990, ETM+ (7) from 2005, and Landsat OLI_TIRS (8) from 2020, which were interpreted for remote sensing images at a resolution of 30 m × 30 m. Track numbers 128/47 and 128/48 were obtained (). ERDAS's LULC change classification will use a 15-meter Google Earth connected to the software, allowing interpretation of the correct location of LULC for remote sensing blurry images. We selected Landsat data for the season of dry in each year because the cloud-free images were available during the season of dry. In addition, there was no rain during the season of dry, which ensures the actual presence of water bodies.
Table 1. Detail of data sources used for analysis of LULC in the study.
This study uses BTM to estimate ESV coefficients, which involves adjusting existing values or data from one location to estimate the ESV of other similar novel sites in the absence of site-specific evaluation information (Msofe et al. Citation2020). It is mainly useful when raw data cannot be collected due to financial or time restrictions (Aneseyee et al. Citation2022). The modification of ESV coefficients is performed based on BTM, which refers to the adaptation of existing values or data from one site to estimate ESV for other similar new sites in the absence of site-specific evaluation information (Msofe et al. Citation2020). This study considers the value of tropical areas with equivalent LULC classes for the geographical area studied to verify the significance of data extracted from the TEEB evaluation database.
ENVI 5.0 was used to perform atmospheric correction and geometric correction on the remote sensing images, and then supervised classification was used to interpret the remote sensing images. The socioeconomic data come from the ‘interviews’, and the precipitation data come from meteorological services. The process characteristics and reasons for LULC change from 1990 to 2050 at a 15-year interval were analyzed, including image processing methods, the creation of interpretation markers, and a remote sensing system for LULC classification. This study selected data from 1990 to 2005 as Phase I; Phase II data from 2005 to 2020; and Phase Final in 2035 and 2050 to analyze the impact of LULC change on the regional ESV in the study area.
2.3. LULC classification and simulation
Satellite images were pre-processed using ENVI 5.3 software for various corrections to ensure an accurate representation of biophysical phenomena. This pre-processing makes sure the imagery aligns correctly with the geographic coordinate system (Darem et al. Citation2023). Accurate land cover change detection requires meticulous radiometric and geometric corrections. Image classification involves sorting pixels in Landsat images into LULC types (Dammag et al. Citation2023). Specific bands from Landsat 5 TM and Landsat 8 OLI were used to produce false-color composite maps. The types of LULC were categorized into five classes, namely, cultivated land, grassland, built-up area, bare land, and waterbody by applying the maximum likelihood supervised classification method (). Then the process characteristics and reasons for LULC change during the study period 1990–2050 at a 15-year interval have been analyzed, and the spatiotemporal gain and loss for each type of land cover were calculated.
Table 2. Description of LULC classification classes.
The supervised maximum likelihood classifier (MLC) algorithm was applied post–pre-processing. This method is adaptable to various data and systems (Servadio and Convertino Citation2018). Some classifications faced challenges due to similar spectral characteristics, and post-classification refinement was employed for better accuracy. Mixed pixels, common in medium-resolution data, were corrected using additional data sources. For verification, 50 forms were created using public land use data and Google Earth maps. Then the accuracy of image classification maps was assessed to understand their reliability. An error/confusion matrix was employed a recommended method by many researchers (Jamal et al. Citation2022; Jodder et al. Citation2023), to compare categorized images with reference data. This matrix utilized reference points from multiple sources, including on-site observations, historical maps, and satellite imagery. For 2020, on-site observations with a 60CSx GPS map were used, encompassing an area of ∼100 m × 100 m (http://www.cso.gov.ye 2020). In total, 143 reference points formed the matrix, with specific allocations based on area coverage. This study used the CA-Markov model in GIS to simulate changes in LULC. The CA-Markov LULC matrix determined past trends and predicted future LULC configurations, transitions, and driving factors. This matrix calculated overall accuracy and the kappa coefficient (K) to measure the classification's accuracy, considering various types of errors. K values range from −1 to 1, indicating the type of land cover changes. Mathematically, the Kappa test measures the value of the coefficient in the point Equation (1)
(1)
(1) Where K is the kappa coefficient, and S is the number of columns and land cover class in the error matrix. Aii is the number of pixels in the first row and first column of the error matrix. Ai+ and A+i are the total number of pixels in row i and column i, respectively. r is the number of rows in the matrix.
The results revealed that in 1990, the overall accuracy and kappa coefficient were 87.4% and 0.84, respectively. In 2005, these figures reached 86.4% and 0.82, while in 2020 they reached 89.2% and 0.86. The overall classification showed strong performance across all maps, reaching an overall accuracy of 87.6% and a resulting kappa coefficient of 0.84 (). Therefore, the image processing method applied in this study has been effective in producing time-matched LULC data.
Table 3. Accuracy assessment of LULC classified for 1990, 2005 and 2020.
2.4. Assignment of ecosystem services values (ESV)
Most ESV evaluations use BTM based on universal or adjusted value coefficients developed by other researchers (Costanza et al. Citation1997; Pham and Lin Citation2023). This study has used the BTM because of its simplicity and parsimony in the approach and resources required for assessment, particularly in data-poor regions (Woldeyohannes et al. Citation2020). Although value proposition coefficients have been criticized due to the limitations and uncertainties in their local use, several researchers have used them in areas where data are scarce and improved upon initial values (Costanza et al. Citation1997) for ecosystem service assessment (Liu et al. Citation2019; Costanza et al. Citation2014). Furthermore, the availability of global datasets on LULC based on remote sensing and regional datasets based on validation and/or correction of the global data facilitates the application of BTM (Woldeyohannes et al. Citation2020). Therefore, we used this method in our study to evaluate ecosystem services for Ibb City. To calculate ESV associated with different types of LULC changes, we conducted a comparison between the LULC classifications obtained from the Landsat TM/ETM dataset and the biomes identified in the ES Assessment Model developed by Costanza et al. (Citation2014). The most relevant biome for each category was assigned as the proxy for that LULC type. For example, cropland biome for ‘Cultivated land’, desert land for ‘Bare land’, urban/village area for ‘Built-up area’, grass/rangelands ‘Grassland’, and lakes/rivers ‘Waterbody’. Despite the LULC categories and equivalent biomes do not precisely match, their use has been possible in many other studies (Morshed et al. Citation2022; Msofe et al. Citation2020; Rahman and Szabó Citation2021). LULC types, equivalent biome, and corresponding value coefficient over historical years 1990, 2005, and 2020, and projected years 2035 and 2050 for the study area are presented in .
Table 4. Corresponding biomes for LULC classes and ES coefficients based on the adjusted estimates.
In order to assess changes in ESV, all value coefficients were converted to US dollars (US$) per hectare per year, based on the 1994 currency value. Moreover, the study incorporated adjustments to the value coefficients using the Consumer Price Index (CPI) and the Producer Price Index (PPI) to account for changes in inflation and price levels over time (available at http://data.imf.org/) to analyze the impact of the temporal evolution of the coefficients on the total estimate of ESV change. In , we present specific information regarding the annual ecosystem service value coefficients adjusted for each LULC change scenario. The study area maps were created using other datasets in a GIS. Therefore, these unit values were more reliable for calculating ESV at the regional level.
Table 5. Details of coefficients for annual conservative adjustments and ecosystem service functions for each LULC type in 1994 US$ ha−1 year−1.
2.5. Calculation of ESV
Considering the LULC class, we made adjustments to the coefficient of ESV based on the fundamental equivalent table of ESV per unit area, as proposed by Yang et al. (Citation2020), and Costanza et al. (Citation1997; Citation2014). The total value of ecosystem services in a particular period, denoted as ESVt was determined by the following equation (2)
(2)
(2) Where ESVt is the total value of ecosystem services in an estimated period, Akt represents the area of LULC type ‘k’ (ha), and VCk is the ecological value coefficient (US$ ha−1 year−1) associated with LULC type ‘k’. System service value coefficient, ‘n’ is the number of LULC types (n = 5).
To calculate the change rate of ecosystem service value (ESVcr) was determined using the following equations Equation (3)
(3)
(3) Where, ‘ESVt1’ represents the total value of ecosystem services in the initial year ‘t1’ and ‘ESVt2’ in the recent year ‘t2’, respectively. A positive ESVcr indicates an increase in the amount of ecosystem service value, while a negative value suggests a decrease.
To estimate the secondary ecosystem service values (ESVft) for a specific item ‘f’ within a given period ‘t’, was calculated using the equation below Equation (4)
(4)
(4) Where VCfk represents the value per unit area of ecosystem service ‘f’ associated with LULC type ‘k’, ‘m’ represents the number of ecosystem service types, and ‘Aft’ represents the area (ha) of LULC type ‘k’ contributing to the ecosystem service ‘f’.
2.6. Calculating elasticity of ESV in response LULC changes
Describes elasticity as a measure that quantifies the responsiveness of one variable to changes in another variable (Yuan et al. Citation2019). We will use this approach to understand how the total ESV changes with changes in LULC by calculating the elasticity of the ESV generated by measuring the percentage change in the ESV in response to the percentage change in LULC (F. Zhang, Yushanjiang, and Jing Citation2019), using the following Equations (Equation5(5)
(5) ) and (Equation6
(6)
(6) )
(5)
(5)
(6)
(6) Where EL is the elasticity of change of total ESV concerning LULC change, ESVcr was explained above EquationEquation (3
(3)
(3) ), LTP is the percentage of land conversion denoting LULC change degree,
Ak is the converted area of LULC class ‘k’, and Li is the initial area of LULC type ‘k’.
2.7. Spatial autocorrelation analyses
The spatial autocorrelation model used in this study mainly represents the degree of spatial concentration of data and is conducive to fully understanding ESV's changing trends and internal mechanisms (F. Zhang, Yushanjiang, and Jing Citation2019). Global spatial autocorrelation is usually represented by Global Moran’s I, which can reveal overall whether the spatial distribution of a certain attribute in a region is correlated with neighboring areas and the degree of correlation (L. Li et al. Citation2022), but it is difficult to measure local spatial agglomeration and its spatial heterogeneity. To this end, it is still necessary to use Local Moran’s I to characterize the spatial differentiation characteristics of coupling coordination from the local scale (Shi et al. Citation2022). The Equations are shown respectively
(7)
(7)
(8)
(8) Where Ii and I represent the global Moran index and the local Moran index respectively; n is the number of spatial units; xi and xj are the observed values of coupling coordination degree of prefecture i and j respectively; x represents the average coupling coordination degree of the study area; Wij is the spatial weight matrix of prefectures i and j, a Queen first-order weight matrix formed by Geoda; the I value range is [−1,1], when I > 0 it is a positive spatial correlation, and the element attributes are clustered and distributed; when I < 0 It is a negative spatial correlation, and the feature attributes present a discretized distribution; when I = 0, it is a random distribution. According to the local Moran index, the coupling coordination level can be divided into four types: HH (High-High clustering), HL (High-Low clustering), LH (Low-High clustering), and LL (Low-Low clustering).
This study uses the Weight tool in GeoDa software to create a spatial weight matrix in Ibb City. It uses the global Moran’s I index and local LISA agglomeration map to analyze the spatial aggregation differences of ESV in Ibb City.
2.8. Sensitivity analysis
The degree of ESV is based on the value of ecological service functions by using the coefficient of sensitivity (CS) to determine whether an equivalent factor is appropriate (Su, Wei, and Lin Citation2020). In this study, the sensitivity index for each land type was determined by adjusting the equivalent factor specific to that land type by 50%, to evaluate the resulting change in the overall value of ecological services and to determine the coefficient of sensitivity for the given land type (N. Li et al. Citation2021). To confirm the representativeness of the ecosystem type for each land cover type and the accuracy of the obtained value coefficient (economic value per unit area), the CS was used to measure the accuracy of the value coefficient. If CS > l, it indicates that the estimated ESV is characterized by elasticity concerning the ecological value coefficient, and the accuracy is relatively low. Conversely, if CS < l, the ecosystem value is considered to be inelastic, indicating that the ecological value coefficient is precise and reliable (Haque et al. Citation2023). The higher the property is, the more accurately the ESV can be estimated. We then calculated the CS by Equationequation (9(9)
(9) ) (Belay, Melese, and Senamaw Citation2022; Hu et al. Citation2020)
(9)
(9) Where, ESV refers to an estimation of the overall ESV offered by a specific LULC type, whereas VC serves as an indicator for comparing the relative value of various land use types. ESVi and ESVj represent the estimated total value of ecosystem services before and after adjusting the ecological value coefficient for the LULC type in question. The variable K represents each LULC type.
3. Results
3.1. LULC changes
This study investigated quantifying the LULC dynamics from 1990 to 2020, and we used the LULC base map from 2005 and transition probabilities from 1990 to 2005 and chose the number of cycles 15 years to simulate the LULC for 2020 by using the CA-Markov model. Following the above process, the future LULC in 2035 and 2050 was predicted. The results showed an overall accuracy of 85.5% and a Kappa coefficient index of 0.80 between the actual LULC and the simulated LULC maps of 2020, which is a good agreement according to (Dey et al. Citation2021). Moreover, we conducted a validation to ascertain the significance of the association between actual and simulated LULC values for each category in 2020, employing the Chi-Square (χ²) test. The results yielded a χ² value of 26.87 for a degree of freedom (df) equal to 7. The result showed that the validation unit includes five statistical coefficients for kappa: kappa no (Kno) with a value of 0.9132, kappa no (Klocation) with a value of 0.8943, kappa location strata (Klocation Strat) with a value of 0.9304, and standard kappa (Kstandard) with a value of 0.8426. This shows that the CA-Markov model has high accuracy to simulate future LULC changes in the study area based on pre-processed data and factors that have an impact on land use changes, such as natural, social, and economic policies. To reduce randomness in the simulation process, this study defines conversion rules for land use types along with influencing factors such as natural conditions, planning policies, climate change, the eco-environment, and other influencing factors. and and illustrates the patterns of spatial distribution of LULC from 1990 to 2050 in the study area. From 1990 to 2005, the built-up areas, grassland, barren land, and waterbody increased by 11.8, 56, 359.1, and 2.2 km², at an annual rate of 2.4, 3.7, 23.9, 0.06 km²/year, respectively. Conversely, vegetated land decreased by 451.7 km² with a rate of 4.2 km²/year. During (2005–2020), the barren land decreased by 121 km², at an annual rate of change of 8.1 km²/year. On the other hand, the vegetated land expanded by 63 km², with a rate of 4.2 km²/year, also grassland increased by 27 km², at an annual change rate of 1.8 km². Built-up areas continued to grow, adding 29 km² (1.9 km²/year), and the waterbody area increased by 1.6 km² (0.1 km²/year). The eco-environment has improved due to some environmental restoration projects implemented by some organizations in the region.
Table 6. Area and proportion of different LULC classes.
The study area has experienced a notable increase in average temperature by 0.25°C per decade, the highest in the area, leading to a decline in biodiversity and vegetation cover. While annual precipitation remained relatively stable in the early 1990s, it approached the multi-year average in the 2000s, peaking in 2005 and 2020, and dropping significantly in 2010. This decline in rainfall has led to water deficits, a critical factor in the degradation of vegetation land, and the increase of barren land. Population trends in the region have shown an increasing trend due to the return of migrants after the Gulf War in 1990, with a growth rate of 4.5% in the first period and 4.4% in the second. Since 2015, population overcrowding due to war and the continuous influx of displaced people has brought about substantial changes in the economic and social fabric, mode of production, and way of life. This shift towards a non-agricultural population has led to a 7.4% decrease in the proportion of agricultural GDP in the region. In addition, national policies and institutions have played a role in shaping LULC changes, where some areas lack a system for registering land titles and documents.
In 2035, cropland, grassland, and barren lands are predicted to decrease totaling a reduction of 36.8, 10.8, and 249.3 km², respectively. Conversely, the built-up area is projected to increase by 50.2 km², driven by rapid population growth in Ibb City, primarily due to rural-urban migration and IDPs. Additionally, the volume of water bodies is anticipated to increase at 1.6 km² due to the expansion of dams and water barriers as outlined in the Water Resources Office's future plan. The decline in vegetation, grasslands, and barren lands is expected to persist in 2050, reaching 1286.7, 397.6, and 3423.7 km², respectively. However, the built-up area is predicted to increase by 184.7 km² and waterbody by 1.9 km².
3.2. Variation in ecosystem services value
3.2.1. Estimation of changes in ESV
The changes in ESV were evaluated from 1990 to 2020 periods, and its predicted amount in the years 2035 and 2050, the total ESV in , and the area of different LULC types in . Please note that we have converted the land area unit of measurement from km2 to hectares since calculating the coefficients of ESV. It can be observed in that the total estimated ESV has reduced from US$ 68.5 million in 1990 to US$ 66.8 million in 2005 and US$ 65.2 Mn in 2020, and is predicted to further decrease to US$ 63.8 Mn in 2035 and US$ 61.2 million in 2050.
Table 7. Estimated ESV for each LULC type of the different reference years (US$ Million/year).
clearly shows that water bodies were the highest contributor to total ESV, followed by grassland, and vegetation land 1990 and 2020 periods. On the other hand, built-up areas and bare land did not contribute to ESV. According to , the ESV trend shows a declining change process. A gradual decrease in ESV was observed for vegetation land, and grassland over the study period 1990–2005 and 2005–2020. A similar trend in the provision of services is predicted to continue from 2020 to 2035, except that the provision of cultivated land services has consistently decreased and ranked third. The sudden decline in cultivated land and its environmental and social value is attributed to heavy commercial investments in the area. It is also projected that waterbodies will continue to contribute the most to total ESV by 2050, followed by grasslands and croplands. Since waterbodies were the highest contributor to total ESV, the characteristics of the total ESV change process are dominated by the characteristic change process of ESV derived from waterbodies.
According to the result, as presented in and , the annual rate of changes of ESV for different LULC classes during the period 1990–2050. A negative sign in the value indicates a decrease in the ESV. In 1990, total changes to ESV over the first study period were approximately US$ 1.7 Mn (2.5%) of the present value in 1990. The total value of ecosystem services experienced a decrease of US$1.6 million (2.4%,) compared to the year 1990. As previously stated, the total ESV underwent a change of about US$3.3 million between 1990 and 2020, which signifies a 4.8% decrease from its 1990 value. Due to the encroachment of built-up areas on cropland and grassland. On the contrary, the values of the water body showed an increase of approximately US$2.3 million (4.8%). Meanwhile, changes in the total value of ecosystem services are expected to continue to decline by approximately US$ 1.4 million (2.2%) and US$ 2.6 million (4.1%) in 2035 and 2050. As a result, the total value of ES over the full prediction period decreased by US$4.0 million, which means 6.1% of the value that existed in 2020.
Table 8. Total ecosystem service valuation (ESV) (US$ in millions) estimated for each LULC classes.
Although the ESV change for each LULC class shows a significant decrease in the value of cropland and grassland, the ESV change for built-up and bare land is absent because they do not derive the ESV. It is also noted that the LULC classes that occurred during the historical periods from 1990 to 2020 have lost US$ 3.3 Mn, and the projected changes in LULC across the periods 2020–2050 will decrease by US$ 4.0 million if the current condition is not enhanced in terms of land management practices (). Interestingly, the percentage changes in the estimated ESV for each type of LULC change were comparable across the study period. However, the primary factor contributing to the overall decrease in the total ESV over the entire study period was the conversion of a significant area of cultivated land and grassland into construction land. This conversion was driven by economic development and the indiscriminate implementation of construction projects in the study area. This underscores the significance of conserving and effectively managing natural resources, along with promoting sustainable LULC practices, to uphold and enhance the provision of ecosystem services.
Overall, the projected trends in ecosystem service values suggest that there is a need for continued efforts to protect and manage natural resources in the study area, to ensure the provision of important environmental services, and to support sustainable development.
3.2.3. Changes in the value of individual functions of ecosystem services
In this study, the general trends in the estimated value of individual ecosystem functions (ESVft) were calculated for each specific period, and their aggregate changes were based on EquationEquation (4(4)
(4) ). In addition, we calculated the effect of LULC changes on individual ecosystem function change over the historical (1990–2020) and projected (2020–2050) period at a 15-year interval; their total contribution represents approximately US$ 68.5, 66.8, 65.2, and 63.8 61.2 million in 1990, 2005, 2020, 2035, and 2050, respectively.
These 17 ecosystem services were divided into four main ecosystem services: regulating (seven types), provisioning (five types), supporting (four types), and cultural (two types). Understanding how each ecosystem function contributes to the overall ESV is made easier with the help of the value of individual ecosystem functions (Rahman and Szabó Citation2021). It was revealed that out of the 17 ecosystem services included in the study, 14 of them showed a significant decrease when measured by EquationEquation (4(4)
(4) ). shows that throughout the study period, regulating services made the largest contribution to the total ESV, followed by provisioning, supporting, and cultural services.
Table 9. Estimated annual value of ecosystem functions (ESVf in US $ million per year).
In 1990, regulating services 40.1% accounted for the largest annual share of total ESV, followed by provisioning, support, and cultural services which means 31.2%, 21.8%, and 6.9%, respectively, yet, supporting services increased to 22.9% and 23.6%, in 2005 and 2020. On the contrary, provisioning, regulating, and culture services decreased to 31.0%, 40.0%, and 6.0% in 2005; 31.4%, 39.6%, and 5.4% in 2020. The increase of supporting services is predicted to continue at about 24.3% and 25.5% in the periods 2035 and 2050, while provisioning, regulating, and cultural services will continue to decline which means 32.5%, 38.0%, and 4.1%, respectively, in 2050. clearly shows that the most significant downward trend in total ESV was regulating services (US$ 1.7 million), followed by cultural services (US$ 1.2 Mn), and provisioning services (US$ 0.9 million) in 2020. The contributions of cultural and Supporting services are predicted to decrease by (US$ 1.0 and 0.6 Mn), but the regulating services continue to increase by (10.1%) across the period 2020–2050 ().
Over the periods 1990–2020, the ecosystem service functions of food production, biological control, and pollination contributed significantly to the total economic values of ESV concerning LULC changes which mean 43.1% (US$ 2.5 million), 67.9% (US$ 1.9 million), and 46.5% (US$ 2.0 Mn), respectively. On the contrary, most of the ecosystem services were reduced as follows, from (high to low): water support (US$ 1.1 million ≈ 22.4%), erosion control (US$ 1.1 Mn ≈ 19.3%), and raw materials (US$ 0.9 million ≈ 23.7%), medical services (US$ 0.9 million ≈ 20.9%), waste treatment (US$ 0.7 million ≈ 14.6%), and climate regulation (US$ 0.6 million ≈ 15.8%), as shown in .
Table 10. Change rate in value (US$ million) of ecosystem service functions from 1990 to 2050.
Although the contribution to total ESV for food production, biological control, and pollination remained increasing over the period 1990–2020, their contributions are predicted to decrease i.e. 18.1% (US$ 1.5 million), 34.1% (US$ 1.6 million), and 30.2% (US$ 1.9 Mn), respectively, in 2050. The highest declines in ESV are expected for gas regulation (US$ 1.5 million), water supply (US$ 0.7 Mn), waste treatment (US$ 0.7 million), and raw materials (US$ 0.7 million) over 2020–2050. In the case of ESV supportive and cultural groups, the nutrient cycle and recreation function had a negative role in the total ESV with apparently equal contributions of (US$ 0.7 Mn) over the period 1990–2020. However, their contribution is expected to decrease by (US$ 0.6 million), and entertainment (US$ 0.5 million) in 2050. As mentioned earlier, grassland destruction as a result of uncontrolled urbanization has had a significant impact on habitat services. This impact is directly related to the food supply, which in turn affects biological control and pollination services (Morshed et al. Citation2022; F. Zhang, Yushanjiang, and Jing Citation2019). Additionally, the estimated erosion control function of ecosystem services is compelling, given the challenging topography of the region, particularly in the southern part. The fact that most land uses are agricultural may indicate adaptations of populations to a rough terrain environment, particularly erosion control.
3.2.4. Impact of LULC change on ESV
The ESV resilience was calculated for each LULC type using Equation (5, 6) to show the effects of LULC changes on the total ESV. The results revealed that LULC change had varied effects on different ES throughout the study periods. It can be observed that certain ESV experienced an increase, while others underwent a decrease as a consequence of LULC change as illustrated in . The elasticity implies that a 1% conversion in land use would result in an average change of US$ 291.7 and 203.8 million in total ESV over the period 1990–2020 and 2020–2050.
The matrix revealed that the area affected by LULC changes was 777.0 and 223.6 km2, respectively, over 1990–2020 and 2020–2050. Across the period 1990–2020, cultivated land, which was mainly the result of urbanization, decreased by 3.4 km2 and led to a decrease of US$ 1.3 million. The conversion of cultivated land to grassland and bare land, with an approximate area of 32.7 and 97.5 km2 respectively, had a significant impact on the estimated ESV. This LULC change resulted in a decrease in ESV by approximately US$24.7 million for the conversion to grassland and US$68.2 million for the conversion to bare land. Furthermore, the decrease in grassland area contributed to a reduction in ESV by US$83.4 Mn. Conversely, 6.8% and 61.7% of built-up land underwent conversion to cropland and grassland, increasing from US$13.4 and US$13.2 million respectively, as presented in .
Table 11. Transition matrix of land use and ESVs in the study area from 1990 to 2020 (km2)
During the predicted period 2020–2050, the transition matrix showed a series of expected decreases in cultivated land by 85.0 km2 and grassland by 21.7 km2 resulting in a decrease in total ESV by US$ 117.0 and 42.8 million, which is the most significant change in the type of transition that predicts the decline in ESV. clearly reports a decrease in agricultural land is also expected by 85 km2, due to the conversion of 113.2 km2 into bare land and 61.1 km2 to grassland, resulting in a decrease of US$ 78.3 and 32.6 million. Meanwhile, converting 50.1% and 13.7% of the built-up area were into cropland and grassland, an increase of US$ 28.3 and 17.4 million, respectively. At the same time, grassland would decrease due to the conversion of 39.5 km2 to bare land and 9.4 km2 to built-up land resulting in a reduction of US$ 6.7 and 2.6 million in 2050. This fully reflects the enormous impact of LULC changes caused by human activities on ESV. Therefore, it is recommended to implement strict control measures in the conversion of bare land into construction land in future development plans, maintain the existing area of cultivated land, and consider strategies to increase the area of water bodies.
Table 12. Transition matrix of land use and ESVs in the study area from 2020 to 2050 (km2).
3.5. Spatial autocorrelation results
3.5.1. Global spatial autocorrelation of ESV
In order to better show the spatial evolution characteristics of ESV, this study used the ‘Queen’ adjacency method, a Moran scatterplot was created to obtain a spatial weight matrix to analyze the ESV of Ibb City from 1990 to 2020, and predicted from 2020 to 2050. The scattered points were mainly distributed in the HH and LL quadrants as shown in . indicates that Moran's I index of ESV is 0.62, 0.67, and 0.69, and the predicted is 0.73, and 0.78 respectively. After selecting 999 replacements for the number of randomization iterations, the calculated Z scores are all greater than 2.58, and the p values are all 0.01. At the same time, Moran’s I values are all positive, indicating that ESV has global spatial aggregation characteristics and is constantly increasing.
Table 13. ESV global spatial autocorrelation indices for 1990–2050.
3.5.2. Local spatial autocorrelation of ESV
A five-phase LISA agglomeration map was obtained by further exploring ESV's local spatial agglomeration characteristics in the study area. It can be seen from the local LISA map in that the insignificant areas are mainly distributed in plain areas, ESV had spatial differences during the study time point, and the agglomeration characteristics showed an LL Mainly agglomeration. In 1990, the study area was still a traditional agricultural city. The study area was mostly LL agglomeration, mainly distributed in the central northern, eastern, and south parts, where the land use types were mostly cultivated land, and grassland. At this time, HH agglomeration was more scattered, mainly concentrated in the central, northern, and other regions; LH and HL types occupy a larger area Small and scattered overall. In 2005, the study area entered a period of rapid development, and the LL agglomeration areas were significantly reduced, mainly concentrated in the east and scattered in the north and southwest parts. At this time, the HH agglomeration areas were further increased and scattered to central and northern parts. The Moran's I index in 2020 is 0.68, which has increased compared with 2005. The proportion of HH types has increased, mainly concentrated in the central and northern and a part of the southern. At the same time, the LL agglomeration areas have decreased further shrink, mainly concentrated in the east and scattered in the northwest part of the study area.
It can be seen from that under the ecological security scenario in 2035 and 2050, ecosystem services are generally distributed mainly in HH and LL clusters. The HH types are mainly distributed in the central and northern parts of the study area. The LL agglomeration areas are mainly distributed in the east and scattered in the north and southwest parts; secondly, the HL agglomeration is a more widely distributed district, mainly distributed in the northwest of the study area. It mainly shows that high-value areas of ecosystem services are surrounded by low-value areas, indicating that the ecosystem service capacity of this agglomeration area is higher than that of surrounding areas, and plays a vital role in the supply of ecosystem services in the region; the smallest distribution range is the LH agglomeration area, mainly distributed in the waterbodies of the study area, showing that areas with low value of ecosystem services are replaced by high Surrounded by value areas, it means that the ecosystem service capacity of the agglomeration area is lower than that of surrounding areas, which has a certain inhibitory effect on the improvement of the regional ecosystem service capacity, is not conducive to regional development, and requires focused management and control.
Overall, the global Moran's I index showed a trend of first decreasing and then increasing from 2000 to 2020. Except for insignificant types, the study area is dominated by HH types, which are mainly distributed in the mountainous part with a better natural ecological environment in the north, such areas are rich in natural resources and have a good ecological environment. Soil and water conservation and natural resource protection can effectively improve the ESV of such gathering areas; LL areas have a certain overlap with construction land, and other types of distribution are more scattered and account for a relative proportion. There are few, and focusing on the construction of green infrastructure within the city and the construction of ecological network patterns can effectively improve ESV levels; LH and HL types are scattered near the urban fringe areas of the central city. There is a negative spatial correlation in some areas. There is a certain irrationality in the layout of land use types in such areas. The construction of ecological corridors should be strengthened to maintain the integrity of regional ecological structure-process-function.
3.6. Sensitivity analysis of ecosystem service value
A sensitivity analysis is a useful technique for assessing the impact of changes in input parameters or assumptions on the output of a model or analysis (Chu-Agor et al. Citation2011; Rahman and Szabó Citation2021). A sensitivity analysis was calculated to determine the CS value by utilizing EquationEquation (7(7)
(7) ), intending to evaluate the potential influences of adjusting the service value coefficient for chosen LULC types, the ±50% adjustment in the service value coefficient was based on the recommendation proposed by Costanza et al. (Citation1997; Citation2014). The CS of the different LULC types in the study area is less than 1 in all cases, the sensitivity of cultivated land and grassland towards total ESV in all indicators was less than 0.2, and the lowest value is 0.05 (). Generally, the total ESV is inelastic, which proves that the value coefficient selected in this study is more appropriate for the study area. Adjustment to the modified value coefficient for the waterbody had a larger significant impact on the estimated ESV than the value coefficient for other LULC types. When the value coefficient for the waterbody was increased by 50%, the estimated ESV also increased by about 31.7%. The CS values ranged from 0.05 to 0.1 for cropland, to higher than 0.6–0.95 for waterbody when value coefficients for these LULC classes were adjusted by 50%. In contrast, the results of the analysis indicate that while there was a ± 50% change in the grassland value coefficient, the overall change in total ESV was less than ±5.1%. This indicates that the ESV estimates using the ESV coefficients were relatively robust.
Table 14. Estimated total values of ecosystem services and coefficient of sensitivity (CS) after adjusting the ecosystem service value coefficient (VC) by 50%.
4. Discussion
4.1. LULC change dynamics
This study provides to measure of LULC changes and their impacts on ecosystem services over the entire study period. Our findings regarding the dynamics of LULC change are in close conformity with those of other studies (Jodder et al. Citation2023; Rahaman, Kafy, Saha, et al. Citation2022). Overall, vegetated land and barren land were the major LULC classes and constituted 92% of the total land cover. Urbanization is increasing in the study area, partly due to financial incentives for farmers to build on their land, leading to rapid urban expansion and environmental concerns (Dammag, Jian, et al. Citation2023; Dey et al. Citation2021). To achieve Sustainable Development Goal (SDG) 11, which seeks to balance urbanization with environmental protection, the study advocates for strengthened land supervision, legislation to resolve disputes, and the revitalization of land registration systems. Despite past governmental regulations on land use, there's an urgent need for stricter land supervision and sustainable policies, especially given the growing popularity of the khat tree and its effects on groundwater. Population growth, economic shifts, and GDP changes are significant drivers of LULC changes, expected to rise by 4.2%–5% in the coming years, emphasizing the need for careful regional development planning. The study aligns with findings by A. Al Kafy et al. (Citation2022) regarding LULC changes in the tropical catchment of Rajshahi, Bangladesh, where the built-up area is predicted to increase by 9.39 and 13.34 km2 in 2029 and 2039, respectively. The city's GDP experienced fluctuations, notably a decline during the Arab Spring revolution but saw a resurgence in the real estate sector recently. The terrain, climate, and overgrazing are other long-term LULC change contributors, suggesting measures like water conservation efforts. Overgrazing and the rise in livestock population have adverse effects on the ecological environment and biodiversity, advocating for ecological resettlement projects (Msofe, Sheng, and Lyimo Citation2019). Policy factors play a crucial role in regional LULC changes, with short-term changes influenced by socioeconomic factors, and natural factors having a longer-term impact (A. Al Kafy, Faisal, et al. Citation2022). To ensure sustainable land use, there's a call for increased governmental investment, infrastructure development, and robust policy implementation (Rahaman, Kafy, Faisal, et al. Citation2022). This study not only addresses the specific characteristics of the study area and the availability of relevant remote sensing and auxiliary data but also incorporates an analysis of SDGs 11, 15, and 17, which could provide insights that can inform sustainable land use planning and policy-making, ensuring the balance between development and conservation, and addressing the specific needs and challenges of the Ibb City community.
4.2. Changes in the total ecosystem service values
Based on remote sensing images and GIS analysis, we conducted a comprehensive study to quantify the changes in ESV in response to LULC change in Ibb city over the entire study period from 1990 to 2050. Our findings observed an increase in certain ecosystem services and a decline in others over the study period was primarily attributed to the patterns and impacts of LULC changes driven by urbanization, economic development, and population growth. The increase in the built-up area led to the conversion of croplands and grasslands, which have high ecological values to urban areas with comparatively lower ecological value. This urban expansion is directly revealed in the decline of provisioning and regulatory services, which are spatially concentrated around urbanized areas. Conversely, certain supporting services showed an increase, due to the relative abundance of water bodies in the study area and their high-value coefficients for individual ecosystem service functions (Liu et al. Citation2019; Wu Citation2021). Therefore, preserving natural areas is vital for maintaining ecosystem functions, thus strategies for conserving and restoring cropland, and grasslands are recommended. In light of Ibb City being a key foodshed, the adoption of sustainable agricultural practices, including agroforestry, organic farming, and water-efficient irrigation, is imperative for the enhancement of provisioning services and food production (Morshed et al. Citation2022; Yuan et al. Citation2019). Regulatory services, such as water supply and erosion control, are vital in the context of Ibb City’s topography and climate, especially given the challenges posed by urban expansion and climate change. Supporting services like biological control and pollination are significant for maintaining biodiversity and agricultural productivity, which are key to the local economy and food security.
According to our study, the built-up area increased from 0.38% to 4.1% in 2050, at the expense of cropland and grassland. Since the built-up areas have no value for ecosystem service, the total ESV has decreased during the past three decades as a result of urbanization (Chatterjee et al. Citation2022; Haque et al. Citation2023). In addition, the impact of climate drought and irrational land use is also greater. If this trend is not effectively controlled, it will have an extremely adverse impact on maintaining ecosystem stability, and the protection and improvement of the ecological environment in the region, as Malaysian (Rahaman, Kafy, Faisal, et al. Citation2022), Bangladesh (A. A. Kafy et al. 2021), India (Basu et al. Citation2023). Therefore, implementing climate change adaptation measures, such as developing urban green spaces and water conservation strategies, is crucial. Policymakers must also prioritize biodiversity and ecosystem protection, combating land degradation and biodiversity loss through protected areas and sustainable grazing practices.
In terms of the total value of ecosystem service functions, as LULC intensity has increased, the value of supporting services has shown a consistent year-by-year increase. On the other hand, provisioning services, regulation services, and cultural services have exhibited a downward trend over time. The supporting services contributed approximately 21.8%, 22.9%, 23.6%, 24.3%, and 25.5% of total ESV in the historical years 1990, 2005, and 2020, and the projected years 2035 and 2050. The primary explanation for the significant contribution of supporting services is the abundance of water bodies and their high-value coefficient for each individual ecosystem service function (Grammatikopoulou and Vačkářová Citation2021). Although the value of functions such as food production, erosion control, and water supply were three major contributors in 1990, the values of erosion control and water supply have shown a decreasing trend over the study period. Specifically, the individual value of erosion control services decreased by 28.1% (US$ 1.6 Mn), while the value of water supply services decreased by 36.7% (US$ 1.8 million) in 2050. In contrast, the individual value of biological control services increased by US$ 3.5 Mn and Pollination by US$2.8 million. When considering the analysis of LULC change, it becomes apparent that differences in the ESV coefficients result in corresponding variations in the impact of LULC change on the estimated ESV. The changing trend of each LULC type aligns with the changing trend of the corresponding ESV (A. Das and Basu Citation2020; Morshed et al. Citation2022; F. Zhang, Yushanjiang, and Jing Citation2019).
The results showed that human activities are the main factors leading to changes in LULC types and ESV, so relevant departments should take corresponding measures to promote sustainable and coordinated regional economic development and strengthen ecological environment protection (Berihun et al. Citation2021; Chen et al. Citation2022). In addition, we found there is a trade-off relationship between supply services and other ecosystem services, which is vital for promoting sustainable development in the regional economy, safeguarding the ecological environment, and ensuring the sustainable provision of ecosystem service (Luo et al. Citation2022), mainly because there is a certain competition between the land used for food and raw material production and other land uses. As a result, reducing LULC types such as cropland and grassland can lead to a significant reduction in total ESV, ultimately resulting in decreased biodiversity, increased land degradation, local warming, and habitat loss (C. Li et al. Citation2021; Olén et al. Citation2021). Ibb City has a large area of hills, and its ecosystem is relatively fragile and most sensitive to human disturbance (Dammag et al. Citation2023). Therefore, to maintain a high level of ESV and enable it to serve humans long-term in a sustainable manner, an integrated approach to land management policies is needed, one that comprehensively considers ecological, social, and economic factors, and addresses the trade-offs between different land uses. This calls for increased governmental investment and infrastructure development, supporting sustainable land use practices. Lastly, establishing a robust system for monitoring and evaluating the effectiveness of these strategies will be key to ensuring their successful implementation and adjustment over time. Engaging local communities and stakeholders in the policymaking process, coupled with educational initiatives, will foster community support and participation. These recommendations aim to strike a balance between developmental needs and ecological conservation, ensuring sustainable resource management and the well-being of the Ibb City community.
4.2. Limitations and future scope of study
This study assessed LULC changes in Ibb's ESV from 1990 to 2050, encountering uncertainties mainly due to data acquisition challenges.(Msofe et al. Citation2020; Niu et al. Citation2022). Firstly, the use of BTM for ESV, although practical in data-scarce regions like ours, introduces uncertainties. These arise from applying value coefficients derived from different ecological and socio-economic contexts, which may not accurately reflect the unique characteristics of Ibb City. Therefore, we recommend developing a value coefficient taking into account the local context. For Yemen, there is no specific value coefficient for different types of LULC. So, future studies can focus on developing the value coefficient in light of the local contest of Yemen. Secondly, the CA-Markov model, employed for predicting future LULC changes, inherently assumes that past trends will continue into the future. This assumption may not hold in the face of rapid socio-economic and environmental changes, potentially leading to less accurate future land cover projections (X. Gao et al. Citation2021; Yang et al. Citation2020). The static nature of value coefficients used in BTM may not accurately represent the temporal changes in ES in Ibb City. To partially mitigate these limitations, our study incorporated local expert knowledge wherever possible to adjust and validate the transferred values. Nonetheless, we acknowledge that the results obtained through BTM should be interpreted with caution, considering these limitations and uncertainties. The findings provide a valuable, understanding of ESV in Ibb City, highlighting the need for more Future studies should enhance ESV evaluation methods, clarify parameter connotations and values, and conduct long-term field surveys for dynamic parameters like net primary productivity and soil erosion.
5. Conclusions
In this study, our aim was to comprehensively assess the impacts of LULC changes on ESV in Ibb City, Yemen, over a period of three decades (1990–2020) and project these trends into the future (2035 and 2050). The results of the analysis indicated a substantial shift in land cover, characterized by an expansion of built-up areas and barren land, and a corresponding decline in cultivated land and grassland. These changes have led to a notable decrease in the total ESV, from US$ 68.5 million in 1990 to US$ 65.2 million in 2020, with predictions of further decline to US$ 61.2 million by 2050. The sensitivity analysis conducted reinforces the robustness of our ESV estimations and highlights the significant role of specific land cover types, particularly water bodies, in influencing the overall ecosystem service provision. The continuous urban expansion and agricultural land conversion in Ibb City, driven by population pressure and socio-economic activities, pose a critical challenge to the sustainability of regional ecosystems. Our study emphasizes the need for integrated land management strategies that prioritize the protection of high-cover croplands and grasslands, which are crucial for maintaining high ESV and are currently facing rapid degradation. The results underline the importance of incorporating ESV considerations into urban planning and policy-making to balance development needs with ecological preservation. This study contributes valuable insights into the spatial relationship between LULC changes and ESV in a rapidly urbanizing context. It provides a basis for future studies and policy interventions aimed at promoting sustainable land use practices and safeguarding the regional eco-environment in Yemen. The approach and findings of this study are not only relevant to Ibb City but also offer a framework that can be applied to similar data-scarce, rapidly changing urban environments.
Consent to publish
All authors of a manuscript have read and agreed to the published version before it is submitted for publication.
Disclosure statement
No potential conflict of interest was reported by the author(s).
Data availability statement
The data presented in this study are available from the corresponding author (B.Q.D.) upon request.
Additional information
Funding
References
- Al-Darwish, Yazid, Hany Ayad, Dina Taha, and Dina Saadallah. 2018. “Predicting the Future Urban Growth and It’s Impacts on the Surrounding Environment Using Urban Simulation Models: Case Study of Ibb City – Yemen.” Alexandria Engineering Journal 57 (4) Faculty of Engineering, Alexandria University: 2887–2895. https://doi.org/10.1016/j.aej.2017.10.009.
- Al Kafy, Abdulla, Abdullah Al Faisal, Abdullah Al Rakib, Sumita Roy, Jannatul Ferdousi, Vinay Raikwar, Marium Akter Kona, and S. M. Abdullah Al Fatin. 2021a. “Predicting Changes in Land Use/Land Cover and Seasonal Land Surface Temperature Using Multi-temporal Landsat Images in the Northwest Region of Bangladesh.” Heliyon 7 (7). https://doi.org/10.1016/j.heliyon.2021.e07623.
- Al Kafy, Abdulla, Md Nazmul Huda Naim, Gangaraju Subramanyam, Abdullah Al Faisal, Nessar Uddin Ahmed, Abdullah Al Rakib, Marium Akter Kona, and Golam Sabbir Sattar. 2021b. “Cellular Automata Approach in Dynamic Modelling of Land Cover Changes Using RapidEye Images in Dhaka, Bangladesh.” Environmental Challenges 4 (January) Elsevier B.V.: 100084. https://doi.org/10.1016/j.envc.2021.100084.
- Al Kafy, Abdulla, Milan Saha, Abdullah Al Faisal, Zullyadini A. Rahaman, Muhammad Tauhidur Rahman, Desheng Liu, Md Abdul Fattah, et al. 2022. “Predicting the Impacts of Land Use/Land Cover Changes on Seasonal Urban Thermal Characteristics Using Machine Learning Algorithms.” Building and Environment 217 (March) Elsevier Ltd: 109066. https://doi.org/10.1016/j.buildenv.2022.109066.
- Al Sabahi, Esmail, Abdul S. Rahim, Wan W.Y. Zuhairi, Fares Alshaebi, and Fadhl Al Nozaily. 2009. “Assessment of Groundwater and Surface Water Pollution at Mitm Area, Ibb City, Yemen.” American Journal of Applied Sciences 6 (4): 772–783. https://doi.org/10.3844/ajassp.2009.772.783.
- Aneseyee, Abreham Berta, Teshome Soromessa, Eyasu Elias, Tomasz Noszczyk, Józef Hernik, and Natei Ermais Benti. 2022. “Expressing Carbon Storage in Economic Terms: The Case of the Upper Omo Gibe Basin in Ethiopia.” Science of The Total Environment 808 (Elsevier): 152166.
- Basu, Tirthankar, Arijit Das, Ketan Das, and Paulo Pereira. 2023. “Urban Expansion Induced Loss of Natural Vegetation Cover and Ecosystem Service Values: A Scenario-based Study in the Siliguri Municipal Corporation (Gateway of North-East India).” Land Use Policy 132 (March 2021) Elsevier Ltd: 106838. https://doi.org/10.1016/j.landusepol.2023.106838.
- Belay, Tatek, Tadele Melese, and Abebe Senamaw. 2022. “Impacts of Land Use and Land Cover Change on Ecosystem Service Values in the Afroalpine Area of Guna Mountain, Northwest Ethiopia.” Heliyon 8 (12) The Author(s): e12246. https://doi.org/10.1016/j.heliyon.2022.e12246.
- Berghof foundation. 2020. https://berghof-foundation.org/library/mapping-of-local-governance-in-yemeni-governorates.
- Berihun, Mulatu Liyew, Atsushi Tsunekawa, Nigussie Haregeweyn, Mitsuru Tsubo, and Ayele Almaw Fenta. 2021. “Changes in Ecosystem Service Values Strongly Influenced by Human Activities in Contrasting Agro-Ecological Environments.” Ecological Processes 10 (1) Ecological Processes: 1–18. https://doi.org/10.1186/s13717-020-00255-4.
- Bindajam, Ahmed Ali, Javed Mallick, Swapan Talukdar, Abu Reza Md Towfiqul Islam, and Saeed Alqadhi. 2021. “Integration of Artificial Intelligence–Based LULC Mapping and Prediction for Estimating Ecosystem Services for Urban Sustainability: Past to Future Perspective.” Arabian Journal of Geosciences 14 (18). https://doi.org/10.1007/s12517-021-08251-4.
- CEPF. 2012. “Eastern Afromontane Biodiversity Hotspot.” (January): 1–268.
- Chatterjee, Soumen, Shyamal Dutta, Ipsita Dutta, and Arijit Das. 2022. “Ecosystem Services Change in Response to Land Use Land Cover Dynamics in Paschim Bardhaman District of West Bengal, India.” Remote Sensing Applications: Society and Environment 27 (March 2021) Elsevier B.V.: 100793. https://doi.org/10.1016/j.rsase.2022.100793.
- Chen, Bingming, Xin Jing, Shensi Liu, Jiang Jiang, and Yugang Wang. 2022. “Intermediate Human Activities Maximize Dryland Ecosystem Services in the Long-term Land-use Change: Evidence from the Sangong River Watershed, Northwest China.” Journal of Environmental Management 319 (Elsevier): 115708.
- Chopra, Bhuvan, Y. S. C. Khuman, and Shalini Dhyani. 2022. “Advances in Ecosystem Services Valuation Studies in India: Learnings from a Systematic Review.” Anthropocene Science 1 (3) Springer Nature Singapore: 342–357. https://doi.org/10.1007/s44177-022-00034-0.
- Chu-Agor, M. L., R. Muñoz-Carpena, G. Kiker, A. Emanuelsson, and I. Linkov. 2011. “Exploring Vulnerability of Coastal Habitats to Sea Level Rise through Global Sensitivity and Uncertainty Analyses.” Environmental Modelling and Software 26 (5) Elsevier Ltd: 593–604. https://doi.org/10.1016/j.envsoft.2010.12.003.
- Costanza, Robert, Ralph d’Arge, Rudolf De Groot, Stephen Farber, Monica Grasso, Bruce Hannon, Karin Limburg, Shahid Naeem, Robert V O’neill, and Jose Paruelo. 1997. “The Value of the World’s Ecosystem Services and Natural Capital.” Nature 387 (6630) Nature publishing group: 253–260. https://doi.org/10.1038/387253a0.
- Costanza, Robert, Rudolf De Groot, Paul Sutton, Sander Van der Ploeg, Sharolyn J Anderson, Ida Kubiszewski, Stephen Farber, and R. Kerry Turner. 2014. “Changes in the Global Value of Ecosystem Services.” Global Environmental Change 26 (Elsevier): 152–158. https://doi.org/10.1016/j.gloenvcha.2014.04.002.
- Dammag, Abdulkarem Qasem, Jian Dai, Shan Cao, Akram Ahmed Alabsi, Basema Qasim Derhem, and Hafiza Zara Latif. 2023a. “Sustainable Planning Strategies for Rural Land Use Using a Hybridized Technique and GIS: Application to An Nijād Village in Ibb City, Yemen.” Journal of Urban Planning and Development 149:1. https://doi.org/10.1061/(asce)up.1943-5444.0000893.
- Dammag, Abdulkarem Qasem, Jian Dai, Shan Cao, Akram Ahmed Alabsi, Basema Qasim Derhem, and Hafiza Zara Latif. 2023b. “Sustainable Planning Strategies for Rural Land Use Using a Hybridized Technique and GIS: Application to An Nijād Village in Ibb City, Yemen.” Journal of Urban Planning and Development 149 (1) American Society of Civil Engineers: 5022052.
- Dammag, Abdulkarem Qasem, Dai Jian, Guo Cong, Basema Qasim Derhem, and Hafiza Zara Latif. 2023. “Predicting Spatio-Temporal Land Use/Land Cover Changes and Their Drivers Forces Based on a Cellular Automated Markov Model in Ibb City, Yemen.” Geocarto International 38 (1) Taylor & Francis. https://doi.org/10.1080/10106049.2023.2268059.
- Darem, Abdulbasit A., Asma A. Alhashmi, Aloyoun M. Almadani, Ali K. Alanazi, and Geraldine A. Sutantra. 2023. “Development of a Map for Land Use and Land Cover Classification of the Northern Border Region Using Remote Sensing and GIS.” Egyptian Journal of Remote Sensing and Space Science 26 (2) National Authority of Remote Sensing & Space Science: 341–350. https://doi.org/10.1016/j.ejrs.2023.04.005.
- Das, Arijit, and Tirthankar Basu. 2020. “Assessment of Peri-Urban Wetland Ecological Degradation through Importance-performance Analysis (IPA): A Study on Chatra Wetland, India.” Ecological Indicators 114 (March) Elsevier: 106274. https://doi.org/10.1016/j.ecolind.2020.106274.
- Das, Manob, and Arijit Das. 2019. “Dynamics of Urbanization and Its Impact on Urban Ecosystem Services (UESs): A Study of a Medium Size Town of West Bengal, Eastern India.” Journal of Urban Management 8 (3) Elsevier B.V.: 420–434. https://doi.org/10.1016/j.jum.2019.03.002.
- Das, Manob, Arijit Das, and Rajiv Pandey. 2022. “Importance-performance Analysis of Ecosystem Services in Tribal Communities of the Barind Region, Eastern India.” Ecosystem Services 55 (January 2021) Elsevier B.V.: 101431. https://doi.org/10.1016/j.ecoser.2022.101431.
- Dey, Nataraj Narayan, Abdullah Al Rakib, Abdulla Al Kafy, and Vinay Raikwar. 2021. “Geospatial Modelling of Changes in Land Use/Land Cover Dynamics Using Multi-Layer Perception Markov Chain Model in Rajshahi City, Bangladesh.” Environmental Challenges 4 (May) Elsevier B.V.: 100148. https://doi.org/10.1016/j.ecoser.2022.101431.
- Gao, Lin, Chuanming Ma, Qixin Wang, and Aiguo Zhou. 2019. “Sustainable Use Zoning of Land Resources Considering Ecological and Geological Problems in Pearl River Delta Economic Zone, China.” Scientific Reports 9 (1) Springer US: 1–14. https://doi.org/10.1038/s41598-018-37186-2.
- Gao, Xing, Jie Wang, Chenxi Li, Weining Shen, Zhaoying Song, Chengjing Nie, and Xueru Zhang. 2021. “Land Use Change Simulation and Spatial Analysis of Ecosystem Service Value in Shijiazhuang under Multi-Scenarios.” Environmental Science and Pollution Research 28 (24) Springer: 31043–31058.
- Gashaw, Temesgen, Taffa Tulu, Mekuria Argaw, Abeyou W. Worqlul, Terefe Tolessa, and Mengistie Kindu. 2018. “Estimating the Impacts of Land Use/Land Cover Changes on Ecosystem Service Values: The Case of the Andassa Watershed in the Upper Blue Nile Basin of Ethiopia.” Ecosystem Services 31(Elsevier B.V.): 219–228. https://doi.org/10.1016/j.ecoser.2018.05.001.
- Grammatikopoulou, Ioanna, and Davina Vačkářová. 2021. “The Value of Forest Ecosystem Services: A Meta-Analysis at the European Scale and Application to National Ecosystem Accounting.” Ecosystem Services 48. https://doi.org/10.1016/j.ecoser.2021.101262.
- Haque, Md Nazmul, Mahdi Mansur Mahi, Md Shahriar Sharif, Rhyme Rubayet Rudra, and Ayyoob Sharifi. 2023. “Changes in the Economic Value of Ecosystem Services in Rapidly Growing Urban Areas: The Case of Dhaka, Bangladesh.” Environmental Science and Pollution Research 30 (18) Springer Berlin Heidelberg: 52321–52339. https://doi.org/10.1007/s11356-022-23840-w.
- Hasan, Shaikh Shamim, Lin Zhen, Md Giashuddin Miah, Tofayel Ahamed, and Abdus Samie. 2020. “Impact of Land Use Change on Ecosystem Services: A Review.” Environmental Development 34:Elsevier B.V. https://doi.org/10.1016/j.envdev.2020.100527.
- Hoque, Muhammad Ziaul, Minhaz Ahmed, Imranul Islam, Shenghui Cui, Lilai Xu, Foyez Ahmed Prodhan, Sharif Ahmed, Md Atikur Rahman, and Jahid Hasan. 2022. “Monitoring Changes in Land Use Land Cover and Ecosystem Service Values of Dynamic Saltwater and Freshwater Systems in Coastal Bangladesh by Geospatial Techniques.” Water (Switzerland) 14 (15): 1–21. https://doi.org/10.3390/w14152293.
- Hou, Jun, Tianlin Qin, Shanshan Liu, Jianwei Wang, Biqiong Dong, Sheng Yan, and Hanjiang Nie. 2021. “Analysis and Prediction of Ecosystem Service Values Based on Land Use/Cover Change in the Yiluo River Basin.” Sustainability (Switzerland) 13 (11): 1–14. https://doi.org/10.3390/su13116432.
- Hu, Sai, Longqian Chen, Long Li, Ting Zhang, Lina Yuan, Liang Cheng, Jia Wang, and Mingxin Wen. 2020. “Simulation of Land Use Change and Ecosystem Service Value Dynamics under Ecological Constraints in Anhui Province, China.” International Journal of Environmental Research and Public Health 17 (12): 1–21. https://doi.org/10.3390/ijerph17124228.
- Jamal, Juhaida, Nurul Ain Mohd Zaki, Noorfatekah Talib, Nurhafiza Md Saad, Ernieza Suhana Mokhtar, Hamdan Omar, Zulkiflee Abd Latif, and Mohd Nazip Suratman. 2022. “Dominant Tree Species Classification Using Remote Sensing Data and Object -Based Image Analysis.” IOP Conference Series: Earth and Environmental Science 1019 (1). https://doi.org/10.1088/1755-1315/1019/1/012018.
- Jiang, Wei, Bojie Fu, and Yihe Lü. 2020. “Assessing Impacts of Land Use/Land Cover Conversion on Changes in Ecosystem Services Value on the Loess Plateau, China.” Sustainability (Switzerland) 12 (17), https://doi.org/10.3390/su12177128.
- Jodder, Pankaj Kanti, Rabeya Sultana Leya, Khan Rubayet Rahaman, Abdulla Al Kafy, Zullyadini A. Rahaman, and Abdulaziz I. Almulhim. 2023. “Modeling the Impacts of Residential, Commercial, and Industrial Land Use on Carbon Footprints Using Kernel Density Function in an Urban Setting.” Earth Systems and Environment 7 (1) Springer International Publishing: 237–254. https://doi.org/10.1007/s41748-022-00332-y.
- Kornatowska, Bozena, and Jadwiga Sienkiewicz. 2018. “Forest Ecosystem Services-assessment Methods.” Folia Forestalia Polonica, Series A 60 (4): 248–260. https://doi.org/10.2478/ffp-2018-0026.
- Li, Jiangyue, Hongxing Chen, Chi Zhang, and Tao Pan. 2019. “Variations in Ecosystem Service Value in Response to Land Use/Land Cover Changes in Central Asia from 1995-2035.” PeerJ 2019 (9): 1–22. https://doi.org/10.7717/peerj.7665.
- Li, Linming, Haining Tang, Jinrui Lei, and Xiqiang Song. 2022. “Spatial Autocorrelation in Land Use Type and Ecosystem Service Value in Hainan Tropical Rain Forest National Park.” Ecological Indicators 137 (March). https://doi.org/10.1016/j.ecolind.2022.108727.
- Li, Ning, Jinye Wang, Haoyu Wang, Bolin Fu, Jianjun Chen, and Wen He. 2021. “Impacts of Land Use Change on Ecosystem Service Value in Lijiang River Basin, China.” Environmental Science and Pollution Research 28 (34) Environmental Science and Pollution Research: 46100–46115. https://doi.org/10.1007/s11356-020-12190-0.
- Li, Chen, Yingmei Wu, Binpin Gao, Kejun Zheng, Yan Wu, and Chan Li. 2021. “Multi-scenario Simulation of Ecosystem Service Value for Optimization of Land Use in the Sichuan-Yunnan Ecological Barrier, China.” Ecological Indicators 132(Elsevier): 108328.
- Liu, Wei, Jinyan Zhan, Fen Zhao, Haiming Yan, Fan Zhang, and Xiaoqing Wei. 2019. “Impacts of Urbanization-Induced Land-use Changes on Ecosystem Services: A Case Study of the Pearl River Delta Metropolitan Region, China.” Ecological Indicators 98 (November 2018) Elsevier: 228–238. https://doi.org/10.1016/j.ecolind.2018.10.054.
- Luo, Rui, Shiliang Yang, Zhiying Wang, Tianming Zhang, and Pengqun Gao. 2022. “Impact and Trade off Analysis of Land Use Change on Spatial Pattern of Ecosystem Services in Chishui River Basin.” Environmental Science and Pollution Research 29 (14): 20234–20248. https://doi.org/10.1007/s11356-021-17188-w.
- Masike, Sennye. 2014. “Key Ecosystems Economic Valuation and Climate Change Key Ecosystems Economic Valuation and Climate Change.”
- Morshed, Syed Riad, Md Abdul Fattah, Md Nazmul Haque, and Syed Yad Morshed. 2022. “Future Ecosystem Service Value Modeling with Land Cover Dynamics by Using Machine Learning Based Artificial Neural Network Model for Jashore City, Bangladesh.” Physics and Chemistry of the Earth 126 (April 2021) Elsevier Ltd: 103021. https://doi.org/10.1016/j.pce.2021.103021.
- Msofe, Nangware Kajia, Lianxi Sheng, Zhenxin Li, and James Lyimo. 2020. “Impact of Land Use/Cover Change on Ecosystem Service Values in the Kilombero Valley Floodplain, Southeastern Tanzania.” Forests 11 (1): 1–17. https://doi.org/10.3390/f11010109.
- Msofe, Nangware Kajia, Lianxi Sheng, and James Lyimo. 2019. “Land Use Change Trends and their Driving Forces in the Kilombero Valley Floodplain, Southeastern Tanzania.” Sustainability (Switzerland) 11 (2): 1–25. https://doi.org/10.3390/su11020505.
- National Information Center (NIC). 2015. 2015. Sana’a, Yemen, http://www.yemen-nic.net/.
- Niu, Haipeng, Ran An, Dongyang Xiao, Mengmeng Liu, and Xiaoming Zhao. 2022. “Estimation of Ecosystem Services Value at a Basin Scale Based on Modified Equivalent Coefficient: A Case Study of the Yellow River Basin (Henan Section), China.” International Journal of Environmental Research and Public Health 19:24. https://doi.org/10.3390/ijerph192416648.
- Olén, Niklas Boke, Fabian Roger, Mark V Brady, Cecilia Larsson, Georg K S Andersson, Johan Ekroos, Paul Caplat, Henrik G Smith, Juliana Dänhardt, and Yann Clough. 2021. “Effects of Farm Type on Food Production, Landscape Openness, Grassland Biodiversity, and Greenhouse Gas Emissions in Mixed Agricultural-Forestry Regions.” Agricultural Systems 189(Elsevier): 103071.
- Pham, Kien T., and Tang Huang Lin. 2023. “Effects of Urbanisation on Ecosystem Service Values: A Case Study of Nha Trang, Vietnam.” Land Use Policy 128 (August 2021) Elsevier Ltd: 106599. https://doi.org/10.1016/j.landusepol.2023.106599.
- Rahaman, Zullyadini A., Abdulla Al Kafy, Abdullah Al Faisal, Abdullah Al Rakib, Dewan Md Amir Jahir, Md Abdul Fattah, S. Kalaivani, R. Rathi, Saumik Mallik, and Muhammad Tauhidur Rahman. 2022. “Predicting Microscale Land Use/Land Cover Changes Using Cellular Automata Algorithm on the Northwest Coast of Peninsular Malaysia.” Earth Systems and Environment 6 (4) Springer International Publishing: 817–835. https://doi.org/10.1007/s41748-022-00318-w.
- Rahaman, Zullyadini A., Abdulla Al Kafy, Milan Saha, Asyirah Abdul Rahim, Abdulaziz I. Almulhim, Sk Nafiz Rahaman, Md Abdul Fattah, et al. 2022. “Assessing the Impacts of Vegetation Cover Loss on Surface Temperature, Urban Heat Island and Carbon Emission in Penang City, Malaysia.” Building and Environment 222 (July) Elsevier Ltd: 109335. https://doi.org/10.1016/j.buildenv.2022.109335.
- Rahman, Md Mostafizur, and György Szabó. 2021. “Impact of Land Use and Land Cover Changes on Urban Ecosystem Service Value in Dhaka, Bangladesh.” Land 10:8. https://doi.org/10.3390/land10080793.
- Servadio, Joseph L., and Matteo Convertino. 2018. “Optimal Information Networks: Application for Data-Driven Integrated Health in Populations.” Science Advances 4 (2): 1–12. https://doi.org/10.1126/sciadv.1701088.
- Shi, Feifei, Bingrong Zhou, Huakun Zhou, Hao Zhang, Hongda Li, Runxiang Li, Zhuanzhuan Guo, and Xiaohong Gao. 2022. “Spatial Autocorrelation Analysis of Land Use and Ecosystem Service Value in the Huangshui River Basin at the Grid Scale.” Plants 11 (17): 1–20. https://doi.org/10.3390/plants11172294.
- Shrestha, Manish, and Suwash Chandra Acharya. 2021. “Assessment of Historical and Future Land-Use–Land-Cover Changes and Their Impact on Valuation of Ecosystem Services in Kathmandu Valley, Nepal.” Land Degradation and Development 32 (13): 3731–3742. https://doi.org/10.1002/ldr.3837.
- Shrestha, Bhaskar, Qinghua Ye, and Nitesh Khadka. 2019. “Assessment of Ecosystemservices Value Based on Land Use and Land Cover Changes in the Transboundary Karnali River Basin, Central Himalayas.” Sustainability (Switzerland) 11 (11). https://doi.org/10.3390/su11113183.
- Su, Kai, Dao zhi Wei, and Wen xiong Lin. 2020. “Evaluation of Ecosystem Services Value and Its Implications for Policy Making in China – A Case Study of Fujian Province.” Ecological Indicators 108 (May 2019) Elsevier: 105752. https://doi.org/10.1016/j.ecolind.2019.105752.
- Taiwo, Balogun Emmanuel, Abdulla Al Kafy, Ajeyomi Adedoyin Samuel, Zullyadini A. Rahaman, Ologun Emmanuel Ayowole, Mahir Shahrier, Bushra Monowar Duti, Muhammad Tauhidur Rahman, Olarewaju Timilehin Peter, and Olamiju Olayinka Abosede. 2023. “Monitoring and Predicting the Influences of Land Use/Land Cover Change on Cropland Characteristics and Drought Severity Using Remote Sensing Techniques.” Environmental and Sustainability Indicators 18 (March) Elsevier Inc.: 100248. https://doi.org/10.1016/j.indic.2023.100248.
- Tolessa, Terefe, Feyera Senbeta, and Moges Kidane. 2017. “The Impact of Land Use/Land Cover Change on Ecosystem Services in the Central Highlands of Ethiopia.” Ecosystem Services 23 (June 2016) Elsevier: 47–54. https://doi.org/10.1016/j.ecoser.2016.11.010.
- Varisco, Daniel Martin. 2018. “Agriculture in the Northern Highlands of Yemen: From Subsistence to Cash Cropping.” Journal of Arabian Studies 8 (2) Taylor & Francis: 171–192. https://doi.org/10.1080/21534764.2018.1551470.
- Wang, Ao, Maomao Zhang, Abdulla Al Kafy, Bin Tong, Daoqing Hao, and Yanfei Feng. 2023. “Predicting the Impacts of Urban Land Change on LST and Carbon Storage Using InVEST, CA-ANN and WOA-LSTM Models in Guangzhou, China.” Earth Science Informatics 16 (1) Springer Berlin Heidelberg: 437–454. https://doi.org/10.1007/s12145-022-00875-8.
- Ward, Christopher. 2014. The Water Crisis in Yemen: Managing Extreme Water Scarcity in the Middle East. New York: Bloomsbury Publishing.
- Woldeyohannes, Ashebir, Marc Cotter, Wubneshe Dessalegn Biru, and Girma Kelboro. 2020. “Assessing Changes in Ecosystem Service Values over 1985–2050 in Response to Land Use and Land Cover Dynamics in Abaya-Chamo Basin, Southern Ethiopia.” Land 9 (2) MDPI: 37.
- Wu, Jiansheng. 2021. “What’s the Relationship between Urbanization and Ecosystem Services from Urban to Rural Area.”
- Yang, Yuejuan, Di Liu Kun Wang, Xinquan Zhao, and Jiangwen Fan. 2020. “Effects of Land-use Conversions on the Ecosystem Services in the Agro-Pastoral Ecotone of Northern China.” Journal of Cleaner Production 249(Elsevier Ltd): 119360. https://doi.org/10.1016/j.jclepro.2019.119360.
- Yuan, Shaofeng, Congmou Zhu, Lixia Yang, and Fenghua Xie. 2019. “Responses of Ecosystem Services to Urbanization-Induced Land Use Changes in Ecologically Sensitive Suburban Areas in Hangzhou, China.” International Journal of Environmental Research and Public Health 16 (7): 1–14. https://doi.org/10.3390/ijerph16071124.
- Zhang, Yan, Xia Chang, Yanfang Liu, Yanchi Lu, Yiheng Wang, and Yaolin Liu. 2021. “Urban Expansion Simulation under Constraint of Multiple Ecosystem Services (MESs) Based on Cellular Automata (CA)-Markov Model: Scenario Analysis and Policy Implications.” Land Use Policy 108 (April) Elsevier Ltd: 105667. https://doi.org/10.1016/j.landusepol.2021.105667.
- Zhang, Fei, Ayinuer Yushanjiang, and Yunqing Jing. 2019. “Assessing and Predicting Changes of the Ecosystem Service Values Based on Land Use/Cover Change in Ebinur Lake Wetland National Nature Reserve, Xinjiang, China.” Science of the Total Environment 656(Elsevier B.V.): 1133–1144. https://doi.org/10.1016/j.scitotenv.2018.11.444.