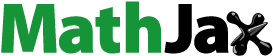
ABSTRACT
The circumpolar arctic tundra, located at Earth’s highest latitudes, is extremely sensitive to climate warming. Studies on arctic greening, based on satellite data and field measurements, show discrepancies due to differences in spatial resolution across datasets (e.g., Landsat 30-m, MODIS 250-m, and AVHRR GIMMS 8 km). Research on scale effects has been limited, mostly focusing on small areas rather than the entire 7.11 million km² arctic tundra. Our study addresses this by mapping scale effects across the entire tundra using Normalized Difference Vegetation Index (NDVI) measurements. Findings reveal: (1) Landsat data provides detailed spatial trends, identifying 18.7% of the area as significantly greening, whereas GIMMS data detects more browning due to spectral mixing; (2) GIMMS underestimates the greening to browning ratio at 2.2:1, compared to Landsat and MODIS ratios of 14.1:1 and 15.1:1, respectively; (3) Over 93% agreement exists between Landsat and MODIS or GIMMS trends, with discrepancies in limited areas. This highlights the importance of high-resolution data and field studies for accurately understanding vegetation trends across the arctic tundra.
KEYWORDS:
1. Introduction
The Arctic has warmed approximately four times more quickly than the rest of the world since 1979 (Rantanen et al. Citation2022). Arctic warming influences how the tundra ecosystem functions due to its interactions with flora, wildlife, and populated areas. Arctic warming has the potential to boost ecosystem respiration and increase greenhouse gas emissions into the atmosphere due to degradation of permafrost. In the meantime, rising temperatures encourage plant growth and boost carbon uptake (Li et al. Citation2021). Researchers have shown that the Arctic was once lush and green, according to a study of plant DNA found in lake sediments, and if the area continues to warm due to climate change, it may someday revert to this state (Crump et al. Citation2021).
Satellite observations over tundra ecosystems have fully documented increases in vegetation productivity (Berner et al. Citation2020; Ju and Masek Citation2016; Liu, Huang, and Sun Citation2021). Some of present changes have been connected to shrub expansion and rapid yearly growth at locations throughout the Arctic (Berner et al. Citation2020; Berteaux et al. Citation2017; Forbes, Macias Fauria, and Zetterberg Citation2010; Loranty et al. Citation2018; Wheeler, Høye, and Svenning Citation2018). However, the Arctic shrub expansion is spatially unequal. Studies have discovered that willow shrubs are growing more frequently on outcrops of higher salinized sea rocks (Ukraintseva et al. Citation2014). A considerable contribution to the greening of the tundra can also be made by the emergence of highly productive herbaceous plant communities in the basins of drained thermokarst lakes (Chen, Liu, and Cheng Citation2021; Loiko et al. Citation2020; Veremeeva et al. Citation2021).
At present, we still don't fully comprehend this prevalent and significant ‘Arctic greening’ process. This is caused, in part, by the fact that going green is not a consistent, all-encompassing reaction, but rather is heterogeneous. Throughout the Arctic, the rate of ‘greening’ varies both spatially and temporally, while some areas show ‘browning’. Accurate predictions of future carbon, nitrogen, and water cycling in the Arctic are significantly hampered by these complexity and substantial uncertainty on future vegetation change in the region (Berner et al. Citation2020; Phoenix and Treharne Citation2022).
To assess the Arctic vegetation response to climate change or anthropogenic influences, it is common practice to assess greening by examining maps showing statistically significant greening or browning trends (every grid cell with a p-value below the nominal value is statistically significant) (Chen et al. Citation2019; Ju and Masek Citation2016; Liu, Huang, and Sun Citation2021). Satellite-derived vegetation indices have been used to assess these changes at high northern latitudes over the past four decades (Berner et al. Citation2020; Elsakov Citation2017; Frost et al. Citation2020; Ju and Masek Citation2016). The most used vegetation indicator for measuring the greenness of aboveground vegetation is the normalized difference vegetation index (NDVI), which has a strong relationship with vegetation health and productivity which can demonstrate various alterations in the features of the land surface properties. Although the NDVI is not sensitive to changes in land surface attribute at high values (Pastick et al. Citation2019), most of the maximum NDVI values over circumpolar tundra are in low value ranges that are less than 0.6 (Walker et al. Citation2002). Therefore, NDVI was selected for the calculation of greenness trend in this study. The three most often used datasets come from the USGS Landsat (1972- present), the Moderate Resolution Imaging Spectroradiometer (MODIS; 2001- present), and the Advanced Very High-Resolution Radiometer (AVHRR; 1982–2015). The NASA Goddard Space Flight Centre’s Global Inventory Modelling and Mapping Studies (GIMMS) NDVI was discovered to be the best for AVHRR vegetation monitoring. Index-3rd generation is the most recent iteration of the GIMMS dataset. Although AVHRR is well designed for circumpolar-scale trend detection, the coarse spatial resolution of AVHRR NDVI data (typically 8 km) fails to satisfy the requirements for straight linking sensor signal with ecological change in heterogeneous tundra landscapes, which further restricts the ability to conduct an attribution analysis on greenness trends, which includes permafrost thaw (Verdonen et al. Citation2020), wildfires (Jenkins et al. Citation2014; Zhou et al. Citation2019), and human disturbances (Berner et al. Citation2020; Liu, Huang, and Sun Citation2021). Furthermore, it is challenging to correlate trends with field measurements due to the coarse spatial resolution (Berner et al. Citation2020).
Satellites with higher resolution, like Landsat, can lessen but not always eliminate the spectrum mixing. The Landsat satellites have been utilized to assess regional or even local trends in tundra greenness with vegetation index like NDVI or EVI. The prior greenness trend studies over the arctic tundra with Landsat satellites mainly focused on reginal sites in Alaska, Canada, and the Russian tundra for two or three decades (Ju and Masek Citation2016; Liu, Huang, and Sun Citation2021; Miles and Esau Citation2016; Pastick et al. Citation2019). Even though some researchers (Berner et al. Citation2020) extracted summer Landsat data for 50,000 randomly selected sampling sites (each with a radius of 50 m) in the circumpolar tundra and discovered that greening (tundra greenness) rose in about 37.3% of sampling sites and browning (tundra browning) increased in about 4.7% of sampling sites from 1985 to 2016, they did not show those changed magnitude pixel by pixel and it is still unclear how they are distributed geographically. In order to improve our ability to predict how the tundra will change in the future, it is imperative that we understand how the tundra as a whole is changing over time and space, as well as how these changes may impact the processes that shape the landscape.
Although it is difficult to quantify how much the scale of measurement affects the sometimes-contradictory greening and browning signals reported in various spectrum datasets (Berner et al. Citation2020), the variations in Arctic greenness trends can be examined using various data resolutions. In this work, we reanalyse circumpolar arctic tundra greening trends pixel by pixel based on Landsat NDVI datasets between 2000 and 2020. By doing this, we demonstrate the ability of the Landsat archive to identify vegetation trends throughout the whole circumpolar arctic tundra and offer the highest resolution spatially complete trend maps of the region to date. We used the same trend analysis method with rigorous significance testing to compare Landsat-based trends with MODIS-based and AVHRR-based trends in order to better understand the regional variability of the reported greening/browning trend. Our goals are to (1) generate a spatially explicit map of vegetation dynamics across the circumpolar arctic tundra at 30 m resolution between 2000 and 2020, (2) compare the fine and coarse data-driven results to find out whether satellite data at coarse resolution misleading the vegetation greening due to the ignorance of spatial heterogeneous at a fine scale, and (3) what are the differences in arctic greening and browning across bioclimatic zone and country level?
2. Data materials and method
2.1. Study areas
The study region was defined in the terrestrial Arctic. The terrestrial Arctic is defined as the northernmost part of the Earth characterized by tundra vegetation, an arctic climate and arctic flora, with the tree line and continental coastlines jointly determining the extent of borders (). Spatially, the present study covers an area of approximately 7.11 million km2, overlapping with parts of six countries including Canada (CA), Denmark (Greenland, GR), Iceland (IC), Norway (NO), Russia (RU), and the United States (Alaska, AK). The tundra regions of these nations have all experienced different levels of anthropogenic disturbance and Russian Arctic has the most densely populated clusters of impervious surfaces (Liu, Huang, and Sun Citation2021; Liu et al. Citation2022a; Liu et al. Citation2022b; Xu et al. Citation2022).
Based on the lowest to highest mean July temperatures, the Circumpolar Arctic bioclimate is classified into five subzones, A to E (Walker et al. Citation2002) (). The coldest subzone is A, where mean July temperatures range from 0 to 3°C, while the hottest subzone is E, where mean July temperatures range from 9 to 12°C. The mean July temperature at the most southern boundary of subzone B, C and D are about 5°C, 7°C and 9°C, respectively. Sub-area A is mostly composed of bare rock, has short vegetation heights, 40% lichen and moss cover, and has a total biomass of less than 3 t/ha; sub-area B has a two-layered vertical structure and has a herbaceous layer up to 10 cm tall, 60% cryptogamous plant cover, and a total biomass of 5–20 t/ha; The vascular plant cover is 5–50% and the height of the creeping and semi-prostrate dwarf shrubs in sub-area C can reach 15 cm, forming an open patchy vegetation zone. In sub-area D, the herbaceous and dwarf shrub layer grows to a height of 40 cm, and vascular plants cover 50–80 percent of the subarea, creating an intermittent and closed canopy zone. The vegetation in the E zone is more densely populated and has two to three layers of vertical structure. The shrub layer can occasionally reach a height of 80 cm. Elevation has a significant impact on the patterns of tundra plant communities and soil moisture. Most of the study area are low-elevation plains less than 100 metres above sea level except some mountains and hills around 1000 metres above sea level located in Alaska and eastern Siberian arctic tundra. Erect shrublands make up around 26% of the vegetated area, followed by peaty graminoid tundras (18%), mountain complexes (13%), barrens (12%), mineral graminoid tundras (11%), prostrate-shrub tundras (11%), and marshes (7%). Russia has the greatest area in the Low Arctic, largely low-shrub tundra, whereas Canada has by far the biggest terrain in the High Arctic, mostly linked with plentiful barren kinds and prostrate dwarf-shrub tundra (Walker et al. Citation2002).
2.2. Landsatbased NDVI datasets
This study utilizes Landsat 5 TM, Landsat 7 ETM+, and Landsat 8 OLI orthorectified top-of-atmosphere (TOA) reflectance data (L1 T) Tier 1. The TOA data were utilized instead of surface reflectance data because they are compatible with previous research due to known errors associated with Landsat SR data over high latitudes (above 65°N) and the lack of MODIS data required to constrain atmospheric correction models for a section of the time series (1984–2000) (Arndt et al. Citation2019; Ju and Masek Citation2016; Pastick et al. Citation2019). All the employed Landsat pictures have a 30 m spatial resolution and were collected between 2000 and 2020 during the melt season (from 1 July to 30 August). displays the number of Landsat observations made in the Arctic between 1984 and 2020 which shows Landsat archives had numerous (more than 20%) data gaps during the 1980s and 1990s, but could cover the entire region after the 2000s. The cloud cover in the TOA reflectance images was assessed using the SimpleCloudScore technique implemented in the Google Earth Engine (GEE). According to our visual interpretation, the threshold score at 20 was set to remove pixels with high cloud. For each land pixel after masking cloud, shadow, and snow with band ‘'BQA'’ in the TOA dataset, the NDVI time series data were calculated. Compared to the previous Landsat-7 Enhanced Thematic Mapper (ETM +), the Landsat-8 Operational Land Imager (OLI) offers better calibration, signal-to-noise characteristics, a greater 12-bit radiometric resolution, and narrower spectral bands, so cross calibration between the two sensors are required before time series analysis. The data from Landsat 7, and 8 were cross-calibrated using the equations from already existing studies (Roy et al. Citation2016) in which statistical functions have been given using ordinary least squares (OLS).
2.3. MODIS based NDVI datasets
The MOD13Q1 product of the Moderate Resolution Imaging Spectroradiometer (MODIS) provides global vegetation index observations at a spatial resolution of 250 m every 16 days. The version number of MOD13Q1 vegetation index product used in this study is 006. The product's V006 iteration delivers more spatial and temporal consistency and lessens systematic inaccuracy caused by sensor degradation. The MOD13Q1 package includes 12 bands, including red, green, blue, and mid-infrared reflectance, as well as quality control (QA) and observation azimuth bands, in addition to the two commonly used vegetation indices, NDVI and EVI. Only high-quality pixel observations were chosen for further investigation. Each image pixel had its unique QA band utilized to measure the observation quality level. In order to obtain the highest NDVI sequence during the growing season, the best inter-annual synthesis was performed based on this information using all valid observation sequences during melt season from 2000 to 2020.
2.4. AVHRR based NDVI datasets
AVHRR GIMMS NDVI3 g v3.1 time series (hereafter GIMMS) (Pinzon and Tucker Citation2014) was also used and it was download from NCAR (https://climatedataguide.ucar.edu/climate-data/ndvi-normalized-difference-vegetation-index-3rd-generation-nasagfsc-gimms) to compare geographic and trend differences. The GIMMS data are two estimates per month with a spatial resolution of 1/12 degrees (∼8 km at the equator). A maximum NDVI composite in every year (2000-2015) in July and August were derived and then used for trend calculation.
2.5. NDVI trend analysis
Every land pixel, with the exception of non-water and non-snow places, was used with the NDVI time series data during melt seasons. Valid time series were considered as having at least two observations within the research period. A Theil-Sen slope estimator was used to determine linear trends for the NDVI time series. Theil-Sen slope estimator performed better than a conventional least squares regression analysis since it is insensitive to outliers with a breakdown point of roughly 29% by using median of slope estimates [16]. The trend analysis of Landsat NDVI time series in Alaska [11], Landsat Tasseled Cap (TC) time series in the Canadian North-West (Fraser et al. Citation2014), and the Arctic Lena Delta in Russia [17–19] have been effectively conducted using Theil-Sen slope estimator because accurate slope estimates can be produced by Theil-Sen slope estimator in the face of arbitrarily extreme observations. The slope between each data pair was obtained at every single pixel, and the median value was then computed as follows.
(1)
(1) Where
is the Theil-Sen slope,
and
are the NDVI values in the time of
and
.
The annual NDVI trend is set as the Theil-Sen slope. When there are more valid data, the trend prediction accuracy might be elevated. A greening trend has a considerable positive slope, while a browning trend has a significant but negative slope. The remaining pixels other than greening and browning are considered to be stable regions where the greenness remains constant.
The Mann-Kendall significance test can be used to validate the significance of Theil-Sen slope estimator. The primary benefit is that it does not require the data to follow a normal distribution and is a widely used for trend test. The following is the relevant calculation formula:
(2)
(2)
(3)
(3)
(4)
(4) Where
is the Mann-Kendall test statistics,
and
are the NDVI values at the time of
and
.
is the time length of the NDVI values. The threshold of significance level is
(95% significance test). Only the altered pixels were included in the final results because trends with p < 0.05 were deemed to be statistically significant in this study. shows the workflow of this study.
2.6. Trend comparison
The comparison of three spatial resolution datasets (Landsat, MODIS, and GIMMS NDVI3g) involved assessing spatial consistency, where we summarize the agreement percentages between Landsat and MODIS or Landsat and GIMMS. Before comparison, bilinear interpolation was applied to resample Landsat results to match the spatial resolution of the other datasets. Additionally, we calculated and compared the green-brown ratio among the datasets. The comparison can help to facilitate a detailed examination of vegetation trends in the circumpolar arctic tundra, highlighting spatial agreement, resolution matching, and overall trends among the datasets.
3. Results
3.1. Landsat-based NDVI trends over the past two decades
Over the past two decades, arctic tundra has witnessed widespread greening (). 18.7% of the region experience greening trend at 95% significance level. Only 1.3% of the region were found has vegetation browning. When we take a close look at the spatial pattern, the most notable greening was detected over the northern and southern parts of Russia's Yamal Peninsula and the central Gydan Peninsula, the northeastern Chukchi Peninsula, northern Quebec tundra, and the North Slope of Alaska. Although browning was much less common than greening, it was more likely to occur at higher latitudes in Canada and lowlands in central and eastern Russia. The average greening trend value was 0.003 NDVI units per year.
Figure 4. Greenness trend (at p < 0.05) over circumpolar tundra acquired with Landsat images from 2000 to 2020.

The results show that the area with changes in vegetation greenness is 1.04 million km2, accounting for 20% of the total area of the study area. Greening, or growing greenness, makes up 95% of the altered regions, while browning, or declining greenness, makes up 5%.
Greening statistics can be summarized on the basis of national scale and bioclimatic subzone scale as shown in . On a national scale, Canada has the biggest amount of greening areas, accounting for 29% of its total area, compared to 9% in the US and 11% in Russia. The difference in browning regions was not statistically significant (1%). The yearly rate of change of NDVI in greening regions was mostly centred in the 0.002–0.004 value range, with the US and RU having a small proportion (5%) of fast greening regions (annual rate of change of NDVI >0.008). At the bioclimatic subzones scale, areas of rapid greening (annual rate of change of NDVI more than 0.008) existed in subzones A and B, accounting for 17% and 9% of the total, respectively. Subzones C、D and E had nearly the same percentage of the rate of change in the 0.002–0.004 interval, which equated to around 55%. As latitude declined, the amplitude of greening rose.
Figure 5. Landsat-based NDVI trend statistics in country and bioclimatic subzone scale. (a). Spatial distribution of greenness trend. (b). statistics of changed and unchanged area percentage over three main countries. (c). greening magnitude over three main countries. (d). greening magnitude over 5 bioclimatic subzones.

3.2. Landsat greening trends compared to MODIS and GIMMS
The trend findings of MODIS and GIMMS are given in , and the results show that the browning areas in the GIMMS results are quite big, cantered in the western and central sections of Russia, whereas the extent of the greening areas in MODIS is similar to that in Landsat results. The higher resolution trend results from MODIS and GIMMS can be found in Figure S4 and S5.
Figure 6. Greenness trend (at p < 0.05) over circumpolar tundra acquired with MODIS and GIMMS NDVI time series. (a). MODIS (b). GIMMS.

and Figure S6 provide a thorough summary of the three data sources used to detect greenness trends over the circumpolar arctic tundra. Landsat NDVI demonstrates a stability rate of 79.98%, indicating that almost 80% of the circumpolar arctic tundra exhibit consistent greenness over the study period. MODIS NDVI follows closely with a stability rate of 79.76%, while GIMMS NDVI3 g shows a slightly higher stability rate of 83.05%. These stability percentage provide valuable insights that the vegetation productivity remains unchanged. Landsat NDVI indicates a greening rate of 18.7%, suggesting that approximately 18.7% of the area exhibit a positive trend of vegetation growth. In contrast, MODIS NDVI shows a slightly higher greening rate of 19%, while GIMMS NDVI3 g exhibits a lower greening rate of 11.6%. Similarly, Landsat NDVI indicates a browning rate of 1.3%, implying a decreasing trend in vegetation growth in 1.3% of the areas. MODIS NDVI and GIMMS NDVI3 g exbibit lower browning rates of 1.3% and 5.3%, respectively.
Table 1. Comparison in the percentage of vegetation greening trend over the circumpolar tundra across three spatial resolution data.
summarizes the stable, greening, and browning areas that were obtained from the three NDVI datasets with regard to the greening tendency in each bioclimate zone. Overall, the areas of greenness change grow as latitude decreases. In Subzones A and B, Landsat and GIMMS both found small areas of greening in addition to patches of browning, while MODIS record few browning spots. The results of the three datasets are more dissimilar in Subzones C, D, and E. For Subzones C, D, and E, the findings from Landsat and MODIS were mostly similar, with greening areas ranged from 9 to 27 times greater than browning areas. However, the GIMMS data showed a less pronounced size difference between areas that are greening and those that are browning.
There is a significant variation between the three datasets regarding the area of change in greenness (). The biggest green-brown ratio, 15.1:1, was discovered by MODIS data, while 14.1:1 and 2.2:1 were computed by Landsat and GIMMS, respectively. The name of the bioclimate zones with the highest percentage of greening is different when it comes to the bioclimate zones of greening. Subzone D (41.251%) has the highest greening value according to Landsat data; however, subzone E has the highest greening value according to MODIS and GIMMS results. The name of the bioclimate zones with the highest level of browning in the browning zones is a distinct one. With percentages of 37.558% and 34.50%, respectively, subzone C has the largest browning proportion in the data from Landsat and GIMMS, whereas subzone E has the highest browning proportion in the results from MODIS (with a percentage of 45.54%). Overall, NDVI data at various resolutions demonstrate disparities in the bioclimate locations where changes in vegetation greenness occur.
Figure 8. Variation between the three datasets regarding the area of change in greenness over bioclimatic subzones. a. Landsat b. MODIS c.GIMMS.

The trend consistency between the Landsat, MODIS, and GIMMS products is distributed spatially in . The results from Landsat and MODIS and GIMMS agree well spatially, with a 95.32% and 93.95% agreement, respectively. The disparity, however, is greater in limited locations. Before the three datasets were compared, bilinear interpolation was used to resample the Landsat result to 250 m and 8 km, respectively. The consistency of Landsat's greening pixels with GIMMS and MODIS is 15.8% and 34.61%, respectively. The reason for the low agreement is that, particularly in the northern Canada and eastern Russia regions where the divergence is greater, the probability that GIMMS and MODIS deem them to be stable pixels exceeds 80% and 65%, respectively. In pixels where Landsat is stable, GIMMS and MODIS exhibit high agreement, with 97.67% and 98.06%, respectively. This may explain why the coarse resolution data overestimated vegetation greenness, with areas of greater disagreement with GIMMS occurring in the Alaska region, portions of the southern Yamal and Gydan peninsulas of Russia, and central Russia and areas of greater disagreement with MODIS occurring in the northern Alaskan, southern Canadian, central, and eastern Russian tundra. All these regions are all considered to be greening by both GIMMS and MODIS. Additionally, MODIS observes some browning in western Russia. Despite the modest browning area in the tundra region, the three datasets are noticeably more different in terms of Landsat browning pixels, with inconsistency rates of 82.9% (GIMMS) and 88.31% (MODIS), respectively.
Figure 9. Comparison of Landsat trend’s consistency with MODIS and GIMMS trend. The pixel frequency distribution (%) of the agreement and disagreement is displayed as a bar plot in each panel. When both two trends are constant, the agreement is displayed in blue; if not, it is displayed in red or green. A circle denotes no change, a + indicates greening, and a - indicates browning.

4. Discussion
4.1. Advantages of greenness trend derived from Landsat NDVI
In order to provide a pixel-by-pixel analysis of the greenness trend in the circumpolar Arctic tundra with fine spatial resolution, Landsat NDVI data collected between 2000 and 2020 were used. Apart from a few locations (less than 1%), where data gaps existed, more than 100 valid observations per pixel were used for trend analysis from 2000 to 2020 (). Landsat data on greenness trends indicated that approximately 18.69% and 1.33% of the Arctic were greening and browning, respectively, at a significance level of 0.05 when water bodies were eliminated in the first stage. The Yamal Peninsula, the Gydan Peninsula, and the northeastern Chukchi Peninsula in Russian tundra, southeast Canada where shrubs and grasses are growing thicker (Ju and Masek Citation2016), and northwestern Alaska all experienced significant greening, which is consistent with earlier studies that used sampled Landsat time series (Berner et al. Citation2020). The values of the NDVI/year greening trend are consistent with those obtained in other arctic regions (Cheţan et al. Citation2020; Fraser et al. Citation2014; Ju and Masek Citation2016; Nitze and Grosse Citation2016; Verdonen et al. Citation2020).
Furthermore, a number of examples of locations that are clearly getting greener were provided through a review of the literature and analysis of very high-resolution remote sensing images (Arndt et al. Citation2019; Davis et al. Citation2021; Edwards and Treitz Citation2017; Myers-Smith et al. Citation2019; Verdonen et al. Citation2020) as shown in . These locations have all undergone a totally greening process except a small portion of the southern areas in Cape Bounty Arctic Watershed Observatory (CBAWO) in (b). Although CBAWO showed a clear greening trend overall, especially in the dry and mesic vegetation types (Edwards and Treitz Citation2017), our Landsat trend demonstrates browning in the polar semi-desert types and GIMMS also shows a magnitude of −0.002 browning over the entire region. In other locations, Landsat and MODIS trend results show relatively high-level spatial consistency. However, GIMMS has no findings in Mordy Yakha in Russia and Barrow in Alaska because of coarse resolution as shown in (d) and 10(e). These case studies allow us to conclude that Landsat's trend maps are more trustworthy than coarser dataset with finer resolution spatial details.
Figure 10. Greening cases retrieved from Landsat, MODIS and GIMMS. These greening cases are validated by very high-resolution data or field photos. These five spots are (a) Torngat Mountains National Park (TMNP) in Canada, centred at 63.544°W,58.781°N. (b) CBAWO in Canada, centred at 109.531°W,74.885°N. (c) Herschel Island in Canada, centred at 139.012°W, 69.591°N. (d) Mordy Yakha in Russia, centred at 68.481°E,70.167°N. (e) Barrow in Alaska, US centred at 156.613°W,71.295°N. Colour code of trend values are the same as those in and , respectively.

Disparities between the datasets may be seen in the browning and greening areas’ spatial distribution, especially in Subzones A, B, C, D, and E. In these subzones, Landsat and MODIS display greater greening areas than GIMMS. The disparities in trend results for the Cape Bounty Arctic Watershed Observatory (CBAWO) underscore the significance of dataset resolution, as Landsat offers more precise spatial information.
4.2. Possible reasons for the differences between the three datasets
Different stability rates are shown by Landsat, MODIS, and GIMMS in , suggesting that greenness has persisted throughout the research period. The smoothing effect and poor resolution of GIMMS could be the reason for its greater stability. Interestingly, MODIS's greening rate is higher than Landsat's, indicating that they may be more or less sensitive to changes (Berner et al. Citation2020). The agreement percentages show that there is generally good spatial agreement between Landsat, MODIS, and GIMMS. Nonetheless, the reduced agreement in some areas, especially in eastern Russia and northern Canada, raises the possibility of constraints, maybe related to the coarse resolution of GIMMS and MODIS. The necessity of resolution matching for precise comparison is emphasized by the interpolation of Landsat data.
Before findings at various resolutions can be compared, the spatial resolution needs to be harmonized. Resampling ensures spatial consistency, allowing for the integration of datasets with different native resolutions. By using bilinear interpolation, which can increase the spatial distribution of continuous values to the maximum extent possible, the original 30 m Landsat NDVI trend could be aggregated to 250 m and 8 km, respectively, to match the resolution of the GIMMS NDVI and MODIS NDVI trend results, which can provide broader perspective on vegetation trends across the entire circumpolar tundra and is useful for understanding landscape-level changes and regional patterns. However, the smoothed trends may mask localized variations in vegetation health or changes, potentially affecting the interpretation of ecological process. In order to investigate the reasons behind the contradicting data, the discrepancies between Landsat vs. MODIS and Landsat vs. GIMMS are summarized in . Although Landsat's results provide a better representation of spatial detail, there may be information loss during the downscaling process when using bilinear interpolation in resampling based on the weighted average distance between the centres of the four closest input pixels.
Figure 11. The discrepancies between Landsat vs. MODIS and Landsat vs. GIMMS due to resampling. Letter A denotes a typical greening area, and letter B denotes a typical browning area in the results of the three datasets for these two locations, which are listed in (c) and (d). Agreement means both has the same greenness trend (stable/greening/browning) and disagreement means neither of the two maps has the same greenness trend.

A potential reason for the significant browning regions observed in the GIMMS data could be the disparity in the time interval for acquiring data between MODIS and Landsat. Since the Landsat and MODIS dataset covers the years 2000–2020but the GIMMS dataset we used covers the years 2000–2015. During the year around 2014, browning in GIMMS result may be caused by a variety of factors. Several different types of unusual weather events were discovered that induced browning in the record-low productivity for north-west Scandinavia reported in 2012 (Bjerke et al. Citation2014). Although they happened further south than our research area, higher latitudes may act as an early warning system. While this might indicate that the Arctic is at risk of browning, vegetation also shows hints of rapid recovery from such episodes. Several studies (Jenkins et al. Citation2014; Zhou et al. Citation2019) have shown that plants can recover from tundra fire within 2–5 years as well as indicated in our study shown in . Those fire events are considered as browning factors and occur at different times. Allman Lake fire started in 2009 ((a)) and Anaktuvuk River fire ((b)) started in 2007 (Zhou et al. Citation2019) and both of the vegetation is fully restored in 2020 and the vegetation is greener than it was before the fires in our Landsat and MODIS results. Nevertheless, Gechia Lake fire scar is browning in our trend results because it was a recent fire occurred in 2015 and has not recovered yet. The annual NDVI maps retrieved by Landsat data are listed in Figure S1–S3 and can confirm our trend results.
Figure 12. NDVI trend after browning event caused by tundra fires in Alaska. (a)-(c) are the trend results from Landsat, MODIS and GIMMS around Allman Lake fire scar. The black polygon is the maximum boundary of Allman Lake fire scar. (d)-(f) are the trend results from Landsat, MODIS and GIMMS around Anaktuvuk River fire scar. The black polygon is the maximum boundary of Anaktuvuk River fire scar. (g)-(i) are the trend results from Landsat, MODIS and GIMMS around Gechia Lake fire scar. The black polygon is the maximum boundary of Gechia Lake fire scar.

Another explanation for the disparity in the results of the three resolutions could be that small-scale biological changes in vegetation are overlooked by coarse resolution analysis since several of the areas that GIMMS perceive an area to be static when Landsat or MODIS deems it to be changing (greening or browning) shown in . provides additional examples of how tundra fire has changed localized vegetation to highlight the issue. GIMMS missed the greening trend in Allman Lake fire and the browning trend in Gechia Lake fire scar. Ecological contributions to spectral greening signals may coexist with browning signals from disturbances like retrogressive thaw slumps or vegetation trampling by herbivores, for example, within this aggregated pixel (Berner et al. Citation2020). Large-scale patterns of spatial variability in pixel-level browning and greening are influenced by spatial resolution. Higher resolution satellites like Landsat can lessen but not entirely stop this spectrum mixing. The significant discrepancies observed in Landsat browning pixels, particularly when compared to GIMMS and MODIS, imply difficulties in precisely identifying areas experiencing vegetation browning (Frost et al. Citation2021; Phoenix and Treharne Citation2022). This aspect holds crucial importance in comprehending the dynamics of Arctic tundra vegetation, and the elevated rates of inconsistency may signal constraints in the ability of coarser datasets to capture subtle changes effectively.
4.3. Limitations of the Landsat greenness trend over the circumpolar Arctic
The limited field measurements that were made could not confirm the general trend in greenness that was observed throughout the entire circumpolar tundra due to the research area's inaccessibility. On the other hand, a 14.1:1 ratio of greening to browning was obtained from 2000 to 2020 using Landsat NDVI time series. These ratios are partially consistent with the ratios found in existing study (Berner et al. Citation2020), who examined 50,000 sampling sites (50-metre circle buffer) across the whole Arctic. To further support the trends found in the Landsat NDVI, a number of hotspots that have been identified by other researchers using high-resolution data or field measurements were found. (see and 12). There is a high demand for higher spatial resolution observations in upcoming research (Berner et al. Citation2020), as well as for gathering field measurement data over focal scales and even the tundra biome scale (Cunliffe et al. Citation2020; Myers-Smith et al. Citation2019; Shabanov et al. Citation2021). These observations will aid in improving our understanding of vegetation greening in circumpolar areas in various vegetation types and geographic gradients.
The piecewise trend analysis of vegetation greenness is highly needed for Arctic tundra. Time series analysis techniques, such as Breaks For Additive Seasonal and Trend (BFAST) (Liu et al. Citation2019; Verbesselt et al. Citation2010a; Verbesselt et al. Citation2010b), CCDC (Zhu and Woodcock Citation2014) or LandTrendr (Kennedy, Yang, and Cohen Citation2010), which have been integrated into the platform of Google Earth Engine (Arévalo et al. Citation2020; Hamunyela et al. Citation2020; Kennedy et al. Citation2018), can be used to identify the breakpoints in the time series data. In addition, widespread active layer detachments and retrogressive thaw slumps are very common in the low arctic tundra zone (Verdonen et al. Citation2020). After a landslide, vegetation cannot fully regrow and the regeneration to original levels of composition, cover, and productivity could take several more decades. Those piecewise time series analysis algorithms are required for this regeneration study.
5. Conclusion
In conclusion, our comprehensive evaluation of pan-Arctic tundra greenness over the past two decades, using high-resolution Landsat satellite observations alongside coarser datasets, provides key insights into the dynamics of plant productivity and biomass. While a general increase in greenness was observed across 18.7% of the Arctic territory, our findings highlight nuanced patterns and challenges:
Disparities in the spatial distribution of browning and greening areas, notably in Subzones A, B, C, D, and E, underscore the pivotal role of dataset resolution. Landsat's finer resolution emerges as crucial for capturing detailed trends, as evidenced in comparisons with MODIS and GIMMS. The finer granularity of Landsat data allows for a more precise understanding of vegetation dynamics, particularly in heterogeneous Arctic landscapes.
Divergent stability rates among Landsat, MODIS, and GIMMS reveal variations in their ability to capture greenness persistence. GIMMS has a higher stability rate attributed to its smoothing effect, while MODIS displays a higher greening rate, suggesting differences in sensitivity to changes. These nuances in stability rates emphasize the importance of considering dataset characteristics when interpreting long-term trends in Arctic vegetation.
Notable inconsistencies in Landsat browning pixels, especially when compared to GIMMS and MODIS, highlight challenges in precisely identifying areas experiencing vegetation browning. These discrepancies underscore potential limitations in coarser datasets, emphasizing the necessity of finer resolution datasets to accurately capture subtle changes in Arctic tundra vegetation.
Challenges in precisely identifying vegetation browning areas, particularly in GIMMS data, include disparities in acquisition time intervals between datasets, spectrum mixing at coarser resolutions affecting detection accuracy, and the complex interplay of ecological contributions to spectral signals. These challenges emphasize the need for a nuanced approach to browning detection and the importance of incorporating additional contextual information for accurate interpretation.
In summary, our study quantifies the changing greenness patterns in the Arctic tundra and highlights the impact of dataset resolution on the observed trends, providing valuable insights for future research in understanding the intricate dynamics of this vital ecosystem.
Acknowledgements
We acknowledge Compton J. Tucker and Jorge E. Pinzon of NASA GSFC for providing GIMMS NDVI3 g data, and NASA for providing MODIS data products. We are appreciative of the USGS and Google Earth Engine's (https://earthengine.google.com/, last access: Feb 2024) assistance with Landsat data. Grateful appreciation to the reviewers for invaluable feedback and contributions to the enhancement of this manuscript.
Supplemental Material
Download MS Word (1.3 MB)Disclosure statement
No potential conflict of interest was reported by the author(s).
Data availability statement
The data that support the findings of this study can be obtained from the corresponding author upon reasonable request.
Additional information
Funding
References
- Arévalo, Paulo, Eric L Bullock, Curtis E Woodcock, and Pontus Olofsson. 2020. “A Suite of Tools for Continuous Land Change Monitoring in Google Earth Engine.” Frontiers in Climate 2: 576740. https://doi.org/10.3389/fclim.2020.576740.
- Arndt, Kyle A, Maria J Santos, Susan Ustin, Scott J Davidson, Doug Stow, Walter C Oechel, Thao TP Tran, Brian Graybill, and Donatella Zona. 2019. “Arctic Greening Associated with Lengthening Growing Seasons in Northern Alaska.” Environmental Research Letters 14 (12): 125018. https://doi.org/10.1088/1748-9326/ab5e26.
- Berner, Logan T, Richard Massey, Patrick Jantz, Bruce C Forbes, Marc Macias-Fauria, Isla Myers-Smith, Timo Kumpula, Gilles Gauthier, Laia Andreu-Hayles, and Benjamin V Gaglioti. 2020. “U1 snRNP Regulates Cancer Cell Migration and Invasion in Vitro.” Nature Communications 11 (1): 1–12. https://doi.org/10.1038/s41467-019-13993-7.
- Berteaux, Dominique, Gilles Gauthier, Florent Domine, Rolf A Ims, Scott F Lamoureux, Esther Lévesque, and Nigel Yoccoz. 2017. “Effects of Changing Permafrost and Snow Conditions on Tundra Wildlife: Critical Places and Times.” Arctic Science 3 (2): 65–90. https://doi.org/10.1139/as-2016-0023.
- Bjerke, Jarle W, Stein Rune Karlsen, Kjell Arild Høgda, Eirik Malnes, Jane U Jepsen, Sarah Lovibond, Dagrun Vikhamar-Schuler, and Hans Tømmervik. 2014. “Record-low Primary Productivity and High Plant Damage in the Nordic Arctic Region in 2012 Caused by Multiple Weather Events and Pest Outbreaks.” Environmental Research Letters 9 (8): 0084006. https://doi.org/10.1088/1748-9326/9/8/084006.
- Chen, Y., A. Liu, and X. Cheng. 2021. “Vegetation grows more luxuriantly in Arctic permafrost drained lake basins.” Global Change Biology 27: 5865–5876. https://doi.org/10.1111/gcb.15853.
- Chen, Chi, Taejin Park, Xuhui Wang, Shilong Piao, Baodong Xu, Rajiv K Chaturvedi, Richard Fuchs, Victor Brovkin, Philippe Ciais, and Rasmus Fensholt. 2019. “China and India Lead in Greening of the World Through Land-use Management.” Nature Sustainability 2 (2): 122–129. https://doi.org/10.1038/s41893-019-0220-7.
- Cheţan, Marinela-Adriana, Andrei Dornik, Florina Ardelean, Goran Georgievski, Stefan Hagemann, Vladimir E Romanovsky, Alexandru Onaca, and Dmitry S Drozdov. 2020. “35 Years of Vegetation and Lake Dynamics in the Pechora Catchment, Russian European Arctic.” Remote Sensing 12 (11): 1863. https://doi.org/10.3390/rs12111863.
- Crump, Sarah E, Bianca Fréchette, Matthew Power, Sam Cutler, Gregory de Wet, Martha K Raynolds, Jonathan H Raberg, Jason P Briner, Elizabeth K Thomas, and Julio Sepúlveda. 2021. “Ancient Plant DNA Reveals High Arctic Greening During the Last Interglacial.” Proceedings of the National Academy of Sciences 118 (13): e2019069118. https://doi.org/10.1073/pnas.2019069118.
- Cunliffe, Andrew M, Jakob J Assmann, Gergana N Daskalova, Jeffrey T Kerby, and Isla H Myers-Smith. 2020. “Aboveground Biomass Corresponds Strongly with Drone-Derived Canopy Height but Weakly with Greenness (NDVI) in a Shrub Tundra Landscape.” Environmental Research Letters 15 (12): 125004. https://doi.org/10.1088/1748-9326/aba470.
- Davis, Emma L, Andrew J Trant, Robert G Way, Luise Hermanutz, and Darroch Whitaker. 2021. “Rapid Ecosystem Change at the Southern Limit of the Canadian Arctic, Torngat Mountains National Park.” Remote Sensing 13 (11): 2085. https://doi.org/10.3390/rs13112085.
- Edwards, Rebecca, and Paul Treitz. 2017. “Vegetation Greening Trends at two Sites in the Canadian Arctic: 1984–2015.” Arctic, Antarctic, and Alpine Research 49 (4): 601–619. https://doi.org/10.1657/AAAR0016-075.
- Elsakov, V. V. 2017. “Spatial and Interannual Heterogeneity of Changes in the Vegetation Cover of Eurasian Tundra: Analysis of 2000–2016 MODIS Data.” Current Problems in Remote Sensing of the Earth from Space 14 (6): 56–72.
- Forbes, Bruce C, Marc Macias Fauria, and Pentti Zetterberg. 2010. “Russian Arctic Warming and ‘Greening’ are Closely Tracked by Tundra Shrub Willows‘.” Global Change Biology 16 (5): 1542–1554. https://doi.org/10.1111/j.1365-2486.2009.02047.x.
- Fraser, Robert H, Ian Olthof, Steven V Kokelj, Trevor C Lantz, Denis Lacelle, Alexander Brooker, Stephen Wolfe, and Steve Schwarz. 2014. “Detecting Landscape Changes in High Latitude Environments Using Landsat Trend Analysis: 1. Visualization.” Remote Sensing 6 (11): 11533–11557. https://doi.org/10.3390/rs61111533.
- Frost, G. V., Uma S Bhatt, Howard E Epstein, Donald A Walker, Martha K Raynolds, L. T. Berner, J. W. Bjerke, Amy L Breen, Bruce C Forbes, and Scott J Goetz. 2020. Tundra greenness.” In.
- Frost, Gerald V, Uma S Bhatt, Matthew J Macander, Amy S Hendricks, and M. Torre Jorgenson. 2021. “Is Alaska’s Yukon–Kuskokwim Delta Greening or Browning? Resolving Mixed Signals of Tundra Vegetation Dynamics and Drivers in the Maritime Arctic.” Earth Interactions 25 (1): 76–93. https://doi.org/10.1175/EI-D-20-0025.1.
- Hamunyela, Eliakim, Sabina Rosca, Andrei Mirt, Eric Engle, Martin Herold, Fabian Gieseke, and Jan Verbesselt. 2020. “Implementation of BFASTmonitor Algorithm on Google Earth Engine to Support Large-Area and sub-Annual Change Monitoring Using Earth Observation Data.” Remote Sensing 12 (18): 2953. https://doi.org/10.3390/rs12182953.
- Jenkins, Liza, Laura Bourgeau-Chavez, Nancy French, Tatiana Loboda, and Brian Thelen. 2014. “Development of Methods for Detection and Monitoring of Fire Disturbance in the Alaskan Tundra Using a Two-Decade Long Record of Synthetic Aperture Radar Satellite Images.” Remote Sensing.
- Ju, Junchang, and Jeffrey G Masek. 2016. “The Vegetation Greenness Trend in Canada and US Alaska from 1984–2012 Landsat Data.” Remote Sensing of Environment 176: 1–16. https://doi.org/10.1016/j.rse.2016.01.001.
- Kennedy, Robert E, Zhiqiang Yang, and Warren B Cohen. 2010. “Detecting Trends in Forest Disturbance and Recovery Using Yearly Landsat Time Series: 1. LandTrendr — Temporal Segmentation Algorithms.” Remote Sensing of Environment 114 (12): 2897–2910. https://doi.org/10.1016/j.rse.2010.07.008.
- Kennedy, Robert, Zhiqiang Yang, Noel Gorelick, Justin Braaten, Lucas Cavalcante, Warren Cohen, and Sean Healey. 2018. “Implementation of the LandTrendr Algorithm on Google Earth Engine.” Remote Sensing 10 (5): 691. https://doi.org/10.3390/rs10050691.
- Li, Zhi-Long, Cuicui Mu, Xu Chen, Xing-Yu Wang, Wen-Wen Dong, Lin Jia, Mei Mu, et al. 2021. “Changes in net ecosystem exchange of CO2 in Arctic and their relationships with climate change during 2002–2017.” Advances in Climate Change Research.
- Liu, Caixia, Huabing Huang, and Fangdi Sun. 2021. “A Pixel-Based Vegetation Greenness Trend Analysis Over the Russian Tundra with All Available Landsat Data from 1984 to 2018.” Remote Sensing 13 (23): 4933. https://doi.org/10.3390/rs13234933.
- Liu, Chong, Huabing Huang, Qi Zhang, Xuanzhu Chen, Xiaoqing Xu, Hanzeyu Xu, and Xiao Cheng. 2022a. “Arctic’s man-Made Impervious Surfaces Expanded by Over two-Thirds in the 21st Century.” Science Bulletin 67: 1425–1429. https://doi.org/10.1016/j.scib.2022.06.001.
- Liu, Caixia, John Melack, Ye Tian, Huabing Huang, Jinxiong Jiang, Xiao Fu, and Zhouai Zhang. 2019. “Detecting Land Degradation in Eastern China Grasslands with Time Series Segmentation and Residual Trend Analysis (TSS-RESTREND) and GIMMS NDVI3g Data.” Remote Sensing 11 (9): 1014. https://doi.org/10.3390/rs11091014.
- Liu, Chong, Xiaoqing Xu, Xuejie Feng, Xiao Cheng, Caixia Liu, and Huabing Huang. 2022b. “CALC-2020: A new Baseline Land Cover map at 10 m Resolution for the Circumpolar Arctic.” Earth Syst. Sci. Data 2022: 1–28.
- Loiko, Sergey, Nina Klimova, Darya Kuzmina, and Oleg Pokrovsky. 2020. “Lake Drainage in Permafrost Regions Produces Variable Plant Communities of High Biomass and Productivity.” Plants 9 (7): 867. https://doi.org/10.3390/plants9070867.
- Loranty, Michael M, Benjamin W Abbott, Daan Blok, Thomas A Douglas, Howard E Epstein, Bruce C Forbes, Benjamin M Jones, Alexander L Kholodov, Heather Kropp, and Avni Malhotra. 2018. “Reviews and Syntheses: Changing Ecosystem Influences on Soil Thermal Regimes in Northern High-Latitude Permafrost Regions.” Biogeosciences (online) 15 (17): 5287–5313. https://doi.org/10.5194/bg-15-5287-2018.
- Miles, Victoria V, and Igor Esau. 2016. “Spatial Heterogeneity of Greening and Browning Between and Within Bioclimatic Zones in Northern West Siberia.” Environmental Research Letters 11 (11): 115002. https://doi.org/10.1088/1748-9326/11/11/115002.
- Myers-Smith, Isla H, Meagan M Grabowski, Haydn JD Thomas, Sandra Angers-Blondin, Gergana N Daskalova, Anne D Bjorkman, Andrew M Cunliffe, Jakob J Assmann, Joseph S Boyle, and Edward McLeod. 2019. “Eighteen Years of Ecological Monitoring Reveals Multiple Lines of Evidence for Tundra Vegetation Change.” Ecological Monographs 89 (2): e01351.
- Myers-Smith, Isla H, Jeffrey T Kerby, Gareth K Phoenix, Jarle W Bjerke, Howard E Epstein, Jakob J Assmann, Christian John, Laia Andreu-Hayles, Sandra Angers-Blondin, and Pieter SA Beck. 2020. “Complexity Revealed in the Greening of the Arctic.” Nature Climate Change 10 (2): 106–117. https://doi.org/10.1038/s41558-019-0688-1.
- Nitze, Ingmar, and Guido Grosse. 2016. “Detection of Landscape Dynamics in the Arctic Lena Delta with Temporally Dense Landsat Time-Series Stacks.” Remote Sensing of Environment 181: 27–41. https://doi.org/10.1016/j.rse.2016.03.038.
- Pastick, Neal J, M. Torre Jorgenson, Scott J Goetz, Benjamin M Jones, Bruce K Wylie, Burke J Minsley, Hélène Genet, Joseph F Knight, David K Swanson, and Janet C Jorgenson. 2019. “Spatiotemporal Remote Sensing of Ecosystem Change and Causation Across Alaska.” Global Change Biology 25 (3): 1171–1189. https://doi.org/10.1111/gcb.14279.
- Phoenix, Gareth K, and Rachael Treharne. 2022. Arctic Greening and Browning: Challenges and a Cascade of Complexities, 3481–3483. Wiley Online Library.
- Pinzon, Jorge E, and Compton J Tucker. 2014. “A Non-Stationary 1981–2012 AVHRR NDVI3g Time Series.” Remote Sensing 6 (8): 6929–6960. https://doi.org/10.3390/rs6086929.
- Rantanen, Mika, Alexey Yu Karpechko, Antti Lipponen, Kalle Nordling, Otto Hyvärinen, Kimmo Ruosteenoja, Timo Vihma, and Ari Laaksonen. 2022. “The Arctic has Warmed Nearly Four Times Faster Than the Globe Since 1979.” Communications Earth & Environment 3 (1): 168. https://doi.org/10.1038/s43247-022-00498-3.
- Roy, David P, V. Kovalskyy, H. K. Zhang, Eric F Vermote, L. Yan, S. S. Kumar, and A. Egorov. 2016. “Characterization of Landsat-7 to Landsat-8 Reflective Wavelength and Normalized Difference Vegetation Index Continuity.” Remote Sensing of Environment 185: 57–70. https://doi.org/10.1016/j.rse.2015.12.024.
- Shabanov, N. V., G. J. Marshall, W. G. Rees, S. A. Bartalev, O. V. Tutubalina, and E. I. Golubeva. 2021. “Climate-driven Phenological Changes in the Russian Arctic Derived from MODIS LAI Time Series 2000-2019.” Environmental Research Letters 16: 084009. https://doi.org/10.1088/1748-9326/ac0be2.
- Ukraintseva, N., M. Leibman, I. Streletskaya, and T. Mikhaylova. 2014. “Geochemistry of Plant-Soil-Permafrost System on Landslide-Affected Slopes, Yamal, Russia as an Indicator of Landslide age.” In Landslides in Cold Regions in the Context of Climate Change, edited by W. Shan, Y. Guo, F. Wang, H. Marui, and A. Strom, 107–131. Cham: Springer International Publishing. https://doi.org/10.1007/978-3-319-00867-7_9.
- Verbesselt, Jan, Rob Hyndman, Glenn Newnham, and Darius Culvenor. 2010a. “Detecting Trend and Seasonal Changes in Satellite Image Time Series.” Remote Sensing of Environment 114 (1): 106–115. https://doi.org/10.1016/j.rse.2009.08.014.
- Verbesselt, Jan, Rob Hyndman, Achim Zeileis, and Darius Culvenor. 2010b. “Phenological Change Detection While Accounting for Abrupt and Gradual Trends in Satellite Image Time Series.” Remote Sensing of Environment 114 (12): 2970–2980. https://doi.org/10.1016/j.rse.2010.08.003.
- Verdonen, Mariana, Logan T Berner, Bruce C Forbes, and Timo Kumpula. 2020. “Periglacial Vegetation Dynamics in Arctic Russia: Decadal Analysis of Tundra Regeneration on Landslides with Time Series Satellite Imagery.” Environmental Research Letters 15 (10): 105020. https://doi.org/10.1088/1748-9326/abb500.
- Veremeeva, Alexandra, Ingmar Nitze, Frank Günther, Guido Grosse, and Elizaveta Rivkina. 2021. “Geomorphological and Climatic Drivers of Thermokarst Lake Area Increase Trend (1999–2018) in the Kolyma Lowland Yedoma Region, North-Eastern Siberia.” Remote Sensing 13 (2): 178. https://doi.org/10.3390/rs13020178.
- Walker, D. A., W. A. Gould, H. A. Maier, and M. K. Raynolds. 2002. “The Circumpolar Arctic Vegetation Map: AVHRR-Derived Base Maps, Environmental Controls, and Integrated Mapping Procedures.” International Journal of Remote Sensing 23 (21): 4551–4570. https://doi.org/10.1080/01431160110113854.
- Wheeler, Helen C, Toke T Høye, and Jens-Christian Svenning. 2018. “Wildlife Species Benefitting from a Greener Arctic are Most Sensitive to Shrub Cover at Leading Range Edges.” Global Change Biology 24 (1): 212–223. https://doi.org/10.1111/gcb.13837.
- Xu, Xiaoqing, Chong Liu, Caixia Liu, Fengming Hui, Xiao Cheng, and Huabing Huang. 2022. “Fine-resolution Mapping of the Circumpolar Arctic Man-Made Impervious Areas (CAMI) Using Sentinels, OpenStreetMap and ArcticDEM.” Big Earth Data 6 (2): 196–218. https://doi.org/10.1080/20964471.2022.2025663.
- Zhou, Zhiwei, Lin Liu, Liming Jiang, Wanpeng Feng, and Sergey V. Samsonov. 2019. “Using Long-Term SAR Backscatter Data to Monitor Post-Fire Vegetation Recovery in Tundra Environment.” Remote Sensing 11 (19): 2230. https://doi.org/10.3390/rs11192230.
- Zhu, Zhe, and Curtis E Woodcock. 2014. “Continuous Change Detection and Classification of Land Cover Using all Available Landsat Data.” Remote Sensing of Environment 144: 152–171. https://doi.org/10.1016/j.rse.2014.01.011.