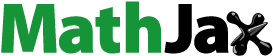
ABSTRACT
Ground surface deformation must be monitored to understand and prevent geological hazards. Global navigation satellite system (GNSS) technology has been widely used in deformation monitoring; however, current GNSS station layout strategies are often subjective and lack theoretical guidance. Therefore, in this study, a GNSS deformation observation network optimization method was developed based on interferometric synthetic aperture radar (InSAR) prior to deformation assistance. This method considers the deformation distribution, cost, accuracy, topography, and actual monitoring requirements and uses kriging interpolation and multiscale iterative optimization to obtain the reference number, spatial distribution, and deformation capture accuracy of GNSS stations in areas experiencing deformation. Simulation experiments showed that the proposed method strengthened the spatial deformation monitoring ability of GNSS by 46%–68% compared with the traditional station layout methods, which ignore the distribution of the deformation. Then, based on the small baseline subset (SBAS) InSAR technology and applying the new method, we evaluated the number and location of the most suitable GNSS stations for a mining area and found that 89.6% of the error was less than 1.5 cm, which further illustrates the practicability and reliability of the proposed method. This method provides an effective supplement to station layouts for monitoring GNSS deformation.
1. Introduction
The Global Navigation Satellite System (GNSS), as one of the most mature precision positioning technologies, provides all-weather, automated, long-term, and continuous observations, so it has been widely used in engineering surveys and for natural disaster monitoring (Petropoulos and Srivastava Citation2021). However, the layout of stations for deformation monitoring using GNSS technology is mainly determined with empirical guidance; moreover, the spatial density of GNSS stations is limited by the cost of the ground equipment (Zulkifli, Din, and Som Citation2018), so the results of GNSS deformation monitoring do not accurately reflect the spatial distribution of deformation in an area. Interferometric synthetic aperture radar (InSAR) (Massonnet and Feigl Citation1998; Zhu, Li, and Hu Citation2017) technology, with its high spatial resolution, high precision, and large-scale rapid monitoring, after decades of development has made considerable breakthroughs in various fields such as the monitoring of volcanoes, earthquakes, landslides, mining areas, glaciers, and urban infrastructure, and for atmospheric correction (Soldato et al. Citation2021; Xue et al. Citation2020). InSAR is one of the effective methods used for monitoring geological disasters. However, the temporal resolution of InSAR, when used for monitoring deformation, is limited by the revisit period of the sensors, which is 6–24 days for most satellites at present (Macchiarulo et al. Citation2022). When monitoring deformation in mining areas (Yang et al. Citation2020), for landslides (Rosi et al. Citation2018; Zheng et al. Citation2023), and groundwater (Motagh et al. Citation2017), which have nonlinear characteristics and large deformation gradients, fully reflecting the temporal characteristics of their deformation is not yet impossible. Therefore, InSAR deformation results cannot be easily used for timely and accurate judgments in some disaster emergency monitoring and engineering applications.
Given these problems, many researchers have focused on the integration of InSAR and GNSS technologies by, for example, using GNSS to correct the atmospheric delay of the InSAR troposphere (Houlie, Funning, and Bürgmann Citation2016; Li et al. Citation2006; Reuveni et al. Citation2015), combining GNSS and InSAR to retrieve three-dimensional surface deformation (Gudmundsson, Sigmundsson, and Carstensen Citation2002; Samsonov et al. Citation2007), and fusing InSAR and GNSS deformation results to obtain high spatial and temporal resolutions (Ge, Han, and Rizos Citation2000; Liu et al. Citation2019; Yan et al. Citation2022). These technologies and their applications have made substantial progress. In these studies, the results of spatial monitoring obtained with GNSS were used, but few studies have considered the required layout of GNSS observation stations to optimize InSAR and GNSS fusion or how to balance cost and monitoring accuracy. The accuracy of deformation monitoring increases with the number of GNSS points. However, increasing the number of GNSS points raises the costs. Therefore, the amount of GNSS equipment used and the accuracy of the deformation monitoring must be balanced. Relevant research in this area remains relatively scarce. Zou et al. (Citation2019) designed a layout strategy for GNSS stations for strike–slip faults based on physical models and parameters such as site spacing and minimum effective distance, and they discussed the influence of the GNSS station distribution on the slip rate and locking depth. Sarsito et al. (Citation2019) designed an ideal GNSS network by measuring the three-dimensional deformation of a volcanic surface using physical equations, such as the Mogi and Okada models. These model-driven methods require prior knowledge of the physical characteristics or equations of the monitored object that cannot be obtained through general monitoring, hindering their generalization and applicability.
The layout of traditional GNSS observation station for deformation monitoring has relied mostly on experience, lacking a theoretical basis. This GNSS observation station layout strategy is not a sufficient scheme: it cannot reflect the spatial distribution characteristics of the deformation. Although adding additional equipment can reduce the estimation error of the parameters, it increases the overall system cost. Some individual methods rely on physical parameters, which are difficult to obtain and thus these methods lack generalizability. This is primarily because of the past lack of technical methods for understanding and evaluating large-scale deformations, resulting in the selection of GNSS station locations by mainly relying on experience and data surveys. In recent years, the launching of various synthetic aperture radar (SAR) satellites (Xue et al. Citation2020) and the provision of free data (Torres et al. Citation2012) have increased the efficiency and precision of InSAR technology while decreasing its cost when determining surface deformation, so the GNSS deformation monitoring network can now be deployed through a data-driven method with the assistance of prior InSAR deformation information. However, to the best of our knowledge, no such study has yet been conducted. Therefore, a system is needed for determining the number and spatial distribution of GNSS deformation monitoring stations with the support of an a priori InSAR high-spatial-resolution deformation field.
In this study, we developed a GNSS observation network Layout optimization method based on InSAR Deformation Assistance (IDAL). Based on prior InSAR deformation results, and considering the actual monitoring requirements, cost, and spatial deformation recovery accuracy, this method accurately obtains the reference number, spatial distribution, and the corresponding spatial deformation of the GNSS station layout in areas experiencing deformation through multiscale iterative optimization. The remainder of this paper is organized as follows: Section 2 introduces the InSAR deformation retrieval method and the GNSS deformation observation network optimization method, which uses prior InSAR deformation information. Section 3 presents a verification of the reliability and practicability of the proposed method, which was achieved through simulations and real data experiments. Section 4 describes the evaluation of the influence of certain factors on the proposed method. Finally, Section 5 concludes the paper.
2. Methods
2.1. Prior deformation retrieval based on SBAS–InSAR technology
Through decades of development, InSAR technology has gradually matured. Various advanced InSAR techniques (Xue et al. Citation2020) can be used to determine the surface deformation rate and time-series deformation. In this study, the SBAS–InSAR (Berardino et al. Citation2002; Lanari et al. Citation2004) method was used to obtain deformation, as only interferograms with short spatiotemporal baselines are required for calculation, which can suppress the influence of spatiotemporal decorrelation to a certain extent.
Assuming SAR images,
interferograms are generated according to the spatiotemporal baseline threshold, with only one small baseline set.
(1)
(1) The solution of EquationEquation (1
(1)
(1) ) can be obtained using the least-squares method, which is expressed as:
(2)
(2) where
is the final deformation result after the adjustment.
is the coefficient matrix,
is the observation matrix, and
is the weight matrix, which can be determined from the coherence of the interferogram.
After obtaining the InSAR deformation results, the GNSS station layout was determined based on the mean InSAR deformation rate because the mean deformation rate comprehensively reflects the deformation over a time period, which shows not only the spatial distribution of deformation but also the temporal accumulation of deformation.
InSAR technology can only be used to obtain one-dimensional line-of-sight (LOS) deformation. According to the SAR data coverage, multitrack InSAR deformation results can be obtained to perform a three-dimensional surface deformation reconstruction.
2.2. Establishment of optimization objective function using prior InSAR deformation
The purpose in this study was to enable GNSS monitoring to more accurately reflect the spatial characteristics of deformation. Therefore, InSAR deformation monitoring results were used as a reference; the deformation at a station monitored with GNSS was interpolated and compared with the InSAR deformation results, and the minimum root mean square error (RMSE) was taken as the optimization goal.
Assuming that set indicates that
GNSS station candidate points (CPs) were obtained in study area D, the selection of
points from
for the layout of GNSS stations is required. The following symbols are defined:
represents the set of the distribution of these
GNSS stations;
denote the position and deformation of the
th point in the current point set, where
, which can be simplified as
;
represents a set of
with the same number of GNSS stations but different distributions, where
denotes
sets
in the set, then
, and
, which all represent the
th station distribution set.
Now all in
are interpolated to recover the spatial deformation field. As Kriging interpolation (Shahbeik et al. Citation2014) can be used to perform an unbiased optimal estimation of the regionalized variables, all
sets in
are subjected to Kriging interpolation (the process of recovering the spatial deformation field through GNSS stations via Kriging interpolation is referred to as the deformation recovered by GNSS stations in this paper). Prior to this, the degree of variation in the spatial deformation field must be estimated.
(3)
(3) where
is the distance between the InSAR points,
represents the deformation value at
;
is the number of pairs of all observation points, with
being the interval; and
is the semivariance of the interval
, which increases with the increase in
within a certain range.
An appropriate variogram model (Knospe and Jonsson Citation2010) was used to interpolate the deformation field. The recovered deformation field was then compared with the InSAR deformation field, and the RMSE of each GNSS distribution set was calculated as follows:
(4)
(4) where
represents the deformation monitored using InSAR,
represents the recovered deformation from the GNSS stations,
denotes the number of InSAR points in monitoring area D, and
represents the RMSE of the
th point set in
.
The objective function is a function of the position of each station. Therefore, using the RMSE calculated using EquationEquation (4(4)
(4) ) as an indicator, the problem of solving the position of the GNSS stations can be converted into the problem of minimizing the RMSE:
(5)
(5) where
represents the optimal solution of the station position,
is the objective function with the coordinates of each GNSS point as the parameters to be solved,
is the weight or scale factor, and
represents the RMSE between the deformation recovered from each potential GNSS station distribution and the InSAR deformation.
2.3. Determination of GNSS CP set
Before solving the model in EquationEquation (5(5)
(5) ), CPs set of the GNSS stations must be determined. As the computational burden of using all InSAR deformation points as CPs would be huge, quadtree downsampling is required (Decriem et al. Citation2010) for the InSAR deformation points, which enables the selection of GNSS station CPs that reflect the spatial characteristics of deformation and reasonably reduces the number and density of InSAR points.
Specifically, the InSAR deformation field is continuously subdivided into squares according to the quadtree recursive algorithm, and the variance in the InSAR deformation in each square is calculated as follows:
(6)
(6) where
is the variance of the InSAR points in the square,
is the number of InSAR points in the square,
denotes the deformation of the
th point in the square, and
is the mean value of
InSAR deformation points in the square.
Set the segmentation threshold δ. If the variance is greater than δ, continue to divide until the variance of the deformation in each square is less than or equal to δ. The points after quadtree downsampling are taken as the CPs.
Not every point is suitable for a GNSS station. Simultaneously, contemplating future equipment maintenance, the available geological structure, topography, and other external data must be integrated. Subsequently, areas with excessive terrain undulation and overly lush vegetation were masked.
2.4. Multiscale iterative solution
Even with the use of quadtree downsampling to reduce the number and density of candidate GNSS points, attempting to directly generate different distributions of GNSS stations through permutation and combination and subsequently applying Kriging interpolation to recover deformations for solving the models in EquationEquation (5(5)
(5) ) would become challenging. As the number of solutions exponentially increases, directly traversing all possible combinations become impractical. Some heuristic searches, such as the greedy algorithm (DeVore and Temlyakov Citation1996) and simulated annealing (Bertsimas and Tsitsiklis Citation1993) fall into local solutions, and the solution is unstable. Therefore, considering the efficiency and accuracy of the solution, a multiscale iterative optimization method was developed to solve the proposed model.
The initial station distribution must be determined before solving the model. This is recorded as , where
represents the
th point at the initial station. There are two types of stations in
, one of which must be monitored according to actual requirements, and these stations will not change in the future; the other includes stations that require iterative optimization, and the initial positions of these stations can be roughly selected according to the InSAR deformation.
Next, a multiscale iterative optimization method is applied to solve the objective function model according to the InSAR deformation, , CPs, and variogram. First, the initial station points are iteratively solved on a large scale (i.e. with a small number of CPs). One method of obtaining large-scale CPs is to increase the variance threshold of the quadtree downsampling, as described in Section 2.3, to reduce the number of CPs. Another method involves downsampling the InSAR deformation field by reducing the resolution to reduce the number of CPs, as shown in .
Figure 1. CPs downscaling using (a) different quadtree downsampling thresholds and (b) different downsampling resolutions.

Assume that the large-scale CP set is obtained through the first method and that its density and number are much smaller than those of
. Now, the optimal solution
of
for large-scale
can be iteratively solved.
First, the RMSE between the spatial deformation recovered from the and the InSAR deformations is calculated and denoted as
. Second, for each point in
, excluding the fixed point, a search is conducted in
for points with a distance less than
, forming a new point set
, where
indicates
points in
. The current station in
is replaced by each point in
in turn, and the RMSE
between the spatial deformation recovered from the formed
station distributions and the InSAR deformation is calculated. Finally, we obtain
(7)
(7) The minimum value
in
and its corresponding station distribution
,
are recorded, indicating that the minimum
corresponds to the
th station distribution in
.
(8)
(8) As shown in EquationEquation (8
(8)
(8) ), when
<0, the station distribution
is more appropriate than the current station distribution. Thus,
is used to replace the current station distribution; otherwise, the current station remains unchanged. This process is repeated for all nonfixed stations until the distance between the corresponding positions of each point in the two iteration stations is less than a given threshold. The final solution can be expressed as
(9)
(9) The final station distribution
is the optimal solution for the initial station
for large-scale
.
is still a rough solution relative to the final station, but its approximate position is correct. Therefore, only a smaller search threshold needs to be used to iterate further on a small-scale point with denser CPs. Similar to the process of solving for station
, the initial station
needs to be replaced with
, where the large-scale CPs set
is replaced with the small-scale candidate point set
, and the larger distance threshold
is replaced with the smaller distance threshold
. Then, the optimization of large-scale stations is repeated to obtain the final GNSS station optimization result
.
(10)
(10) Then, the IDAL method is used to calculate the RMSE of the optimal station distribution corresponding to different numbers of stations and to determine the corresponding relationship between the number of stations and the RMSE. The inflection point of the curve represents the optimal number of GNSS stations in the area experiencing deformation. presents a flowchart of the IDAL method.
3. Results
3.1. Simulation experiments
A simulation was conducted to verify the effectiveness of the IDAL method. shows a simulated deformation field (200 300 pixels; resolution 10
10 m). To make the deformation more realistic, local subsidence and Gaussian white noise were added.
3.1.1. Optimal distribution of GNSS stations based on IDAL method
First, the stable area was masked according to the InSAR deformation field to reduce unnecessary calculations. Second, employing the IDAL method, the station layout results of the deformation area were obtained. Finally, taking the deployment of nine stations in this area as an example, traditional methods, such as the empirical layout (EL) and uniform layout (UL) methods, were compared with the IDAL method (as shown in ). The EL method is an empirical GNSS station layout method that initially considers the deformation distribution. The UL method evenly lays out stations in areas with deformation, and their number and spacing are determined using human experience.
Figure 4. Accuracy comparison of different station layout methods (black triangles: fixed stations; red triangles: final optimized stations). (a–c) Deformation results recovered using EL, UL, and IDAL methods; (d–f) the between (a–c) and the simulated deformation; (g–i) error histograms.

(a–i) show the deformation fields and their error distribution results recovered using the three methods based on the corresponding nine stations. The deformation obtained using these three methods was similar to the simulated deformation, indicating that they could recover the general characteristics of the deformation. However, their error distributions and histograms significantly differed. The IDAL method fully considers the distribution of deformation; therefore, it accurately captured the spatial characteristics of deformation in the entire region using only nine stations, and the error was small and almost obeyed a normal distribution. The results of the EL method were primarily arranged near the two central axes, which was reasonable. However, compared with the IDAL method, the accuracy on the axis was not significantly improved, and the deformation error of some areas exceeded 4 cm. Similarly, the local error produced with the UL method was also large. In addition, the errors of the EL and UL methods were more dispersed.
lists the mean absolute error (MAE), standard deviation (STD), and RMSE of the three methods. Overall, the IDAL method exhibits the smallest errors. Compared with the EL method, its MAE, STD and RMSE increased by 54.96%, 46.32 and 55.28% respectively; compared with the UL method, its performance in MAE, STD and RMSE increased by 66.67%, 61.17 and 68.42% respectively. These results demonstrated the rationality and reliability of the proposed method.
Table 1. Statistical error analysis of EL, UL and IDAL methods from (g–i).
3.1.2. Optimal number of GNSS stations
To evaluate the most appropriate number of stations for an area experiencing deformation, the variation in the RMSE for different numbers of stations was obtained using the IDAL method. As shown in , the shape of the curve approximates that of an L curve (Hansen and O’Leary Citation1993). With an increase in the number of stations, the increase in deformation accuracy was strong at the beginning but eventually tended to flatten.
Figure 5. RMSE corresponding to different number of stations (blue dotted line: RMSE with 9 stations; green ellipse: reference range of the number of stations in deformation area).

Because a large number of stations cannot be laid, regardless of cost, a compromise is required between the number of stations and accuracy. The green ellipse in presents the reference range of the number of stations for the simulated deformation given by the new method. Within this range, a quality standard for deformation monitoring can be designed, and the number of stations can be reasonably determined according to the actual monitoring requirements and budget. Generally, when the deformation accuracy was higher than 1 cm, most of the monitoring requirements were achieved; therefore, only nine stations were deployed in this simulation experiment ( g).
The above simulation experiments and analyses demonstrate that the traditional EL and UL methods, which ignore the deformation distribution, are unreasonable. The proposed IDAL method fully considers the distribution of the deformation field, which maximizes the ability of GNSS stations to capture spatial deformation.
3.2. Real test case: mining deformation monitoring in Datong
3.2.1. SBAS–InSAR ground surface deformation retrieval and analysis
Datong is the northernmost city in Shanxi province, China. To promote urban energy conservation, emission reductions, and local economic development, Datong has built many photovoltaic facilities, including photovoltaic panels, booster stations, and transmission towers, for power generation, and has achieved good results. However, owing to the ongoing coal mining activities in Datong, ground subsidence has occurred in many places (Jia, Ma, and Zhao Citation2008; Zhao et al. Citation2014), which seriously threatens the safety of these photovoltaic facilities. Numerous researchers (Palamà et al. Citation2022; Pawluszek-Filipiak and Borkowski Citation2020; Pawłuszek-Filipiak et al. Citation2023) have employed InSAR technology to monitor the ground deformation in mining areas. These studies have considerably contributed to reducing temporal and spatial decoherence, eliminating atmospheric interference, restoring complete deformation information, and improving the overall accuracy of deformation monitoring in mining areas. However, the revisit period of InSAR data is limited. Therefore, in order to grasp the safety and deformation of photovoltaic equipment in the region in a timelier manner, GNSS stations must be deployed in key locations to enable collaborative monitoring on the basis of InSAR deformation monitoring.
(a) shows an optical image of the mining area in Datong, where several photovoltaic devices were located. DT05 was located at a booster station; therefore, a GNSS station was deployed to monitor the local deformation. DT03 is another GNSS station located far from DT05, in an area with factories, which monitored the local deformation.
Figure 6. (a) Optical image of the study area (red box: coverage of ALOS2 image; yellow polygon: factory area with installed solar photovoltaic panels; white stars: transmission towers; black triangles: GNSS stations that were deployed; white dotted line: boundary of mining area), and (b) annual mean deformation rate obtained with InSAR (black box: selected deformation region).

In the study area, 11 ALOS2 ascending SAR images from June 19, 2020 to April 23, 2021 and the Shuttle Radar Topography Mission (SRTM) 30 m DEM data were obtained. After registration, a baseline set was formed, with a temporal baseline threshold of 126 days and a spatial perpendicular baseline threshold of 270.8 meters. Then, a multi-look of 1:2 in the range and azimuth directions was used for differential interference, and the obtained differential interferograms were filtered using the Goldstein method (Goldstein and Werner Citation1998). In general, the phase quality in the center of a mining area is relatively low, which causes difficulties in phase unwrapping. Therefore, the hierarchical networking and constrained adjustment (HNCA) phase unwrapping method (Mao et al. Citation2021) was used to recover the complete phase with low coherence at the center of the mining area. The orbital error was removed using polynomial fitting (Ferretti et al. Citation2007). The least-squares method was used to obtain the final mean deformation rate. For the atmosphere in nonlinear deformation, spatiotemporal filtering (Hooper et al. Citation2012; Osmanoğlu et al. Citation2016) was used to separate the atmosphere; finally, the complete time series deformation was obtained. (b) shows the annual mean deformation rate in the LOS direction obtained using SBAS–InSAR technology.
Subsequently, to compare the InSAR and GNSS results, the GNSS deformation was projected in the LOS direction using EquationEquation (11(11)
(11) ) (Hanssen Citation2001); DT06, a station far from the deformation area, was employed as a reference station to correct the InSAR deformation results.
(11)
(11) where
represents the deformation obtained with the GNSS transformed into the LOS direction;
,
and
represent the east–west, north–south, and vertical deformation of the GNSS points, respectively;
represents the incident radar angle of the satellite; and
represents the radar azimuth angle.
In , the InSAR time-series deformation is compared with that of the two GNSS stations in the study area, and the corresponding RMSE was calculated. The RMSE of DT03 and DT05 were found to be 0.97 and 0.54 cm, respectively, which demonstrate the reliability of the deformation results obtained with InSAR.
Figure 7. Comparison of the time-series deformation accuracy of the InSAR and GNSS results at DT03 and DT05.

(b) shows a large subsidence basin in the study area, with the maximum subsidence rate exceeding –15 cm/year. Simultaneously, some transmission towers were at the edge of the subsiding basin, which may cause disasters such as ground fissures. shows that on June 25, 2021, a wall that cracked and sank by 15 cm was observed near DT05, indicating that the subsidence was serious. The ALOS2 data have a spatial resolution of 6 m, but their temporal resolution is 14 days. As such, the satellite sometimes fails to capture data, resulting in only one SAR scene per month. Although Sentinel-1 data can be obtained for free, their spatial resolution is too low (approximately 20 m) to meet monitoring requirements. Therefore, to more accurately identify the subsidence in the area and ensure the safety of the photovoltaic power generation equipment, more GNSS stations are required for joint monitoring.
3.2.2. GNSS observation station optimization using IDAL method
Because the spatial distribution of the deformation was ignored when the GNSS stations were laid out, some of the GNSS stations indicated in (a) are not reasonable, so they could not effectively monitor the deformation. To enable the new GNSS stations to more accurately capture the spatial characteristics of the deformation, the IDAL method was used for evaluating the number and locations of the most suitable GNSS stations in this area. (a) shows the clipped deformation area (black rectangular area in (b)); the stable area without deformation and the area away from the plots (purple polygon region) are masked out. Because DT05 was located near an important facility booster station at the center of the local subsidence, a GNSS station is needed here. DTM1 and DTM2 are two local subsidence centers, where the annual mean deformation rate also exceeds –16 cm/year, so GNSS stations must also be deployed at these two locations.
Figure 9. (a) Deformation region of interest, and (b) RMSE corresponding to the number of different GNSS stations (blue dotted line: deformation recovery accuracy with 12 stations; green ellipse: recommended range for the number of stations in this area).

Considering the above three stations as constraints, the IDAL method was used to determine the change in the RMSE of the deformation recovery accuracy with the number of stations in the area. As shown in (b), the result is similar to that of the simulation experiment. Compared with the numbers found in the simulation, more stations are required to achieve the same accuracy in this deformation area because of the three local settlement centers in this area, and the deformation is more complicated. However, this reflects the universality of the IDAL method: it can handle complex scenes. The green ellipse in (b) represents the reference range for the number of stations in the region based on the curve. Considering the high cost of GNSS ground equipment and the substantial mining deformation, an RMSE of less than 1 cm can satisfy the deformation monitoring requirements. Therefore, 12 GNSS stations are required in this area, which would produce an RMSE of 0.95 cm at this time.
As the InSAR-based deformation results were previously verified, these results for these stations were used to recover the deformation field. (a) shows the deformation field recovered using these 12 GNSS stations (three black triangles and nine red triangles). The black triangles denote the constrained stations, while the red triangles represent station positions optimized by the IDAL method. The deformation field retrieved from the GNSS stations aligns with the deformation characteristics observed in the InSAR deformation field depicted in (a). (b) and (c) show that the error obeys an approximately normal distribution, which indicates that the spatial distribution of these stations is reasonable. The statistical results of the absolute error percentage in show that 89.6% of the point errors were less than 1.5 cm, which indicates that the deformation recovered using the 12 stations is reliable and can be used to determine the deformation in the entire study area. Of the point errors, 4.8% were greater than 2 cm because the deformation in this area was more complicated. However, given the few photovoltaic devices in these areas, this error is acceptable.
Figure 10. (a) The station-recovered deformation (red triangles: stations optimized using IDAL method), (b) the difference between the deformation recovered using the GNSS stations and the InSAR deformation, and (c) distribution histogram of the error in b.

Table 2. Statistics of the absolute error from (b).
(a) also shows that although DT03 was not selected as a necessary station, it almost coincides with the optimized station DTB2, which indicates that the position of station DT03 is reasonable. Five stations, DTB1, DTB2, DTB6, DTB7, and DTB8, are located inside or near the equipment; therefore, they can be used for the high-precision monitoring not only of the overall deformation but also of the deformation of the equipment. Four stations, DTB3, DTB4, DTB5, and DTB9, were located outside the plot and were used to control the spatial characteristics of the entire deformation area. In the future, these stations could be used to invert the deformation of the entire region without using InSAR data.
Generally, the IDAL method can be used to determine the number and spatial distribution of GNSS stations in a mining area experiencing deformation, considering accuracy, cost, and specific facilities. These GNSS stations captured the spatial characteristics of the deformation to the greatest extent possible, and the proposed method was used to improve the locations of GNSS stations and to provide guidance for station layout in GNSS deformation monitoring networks.
4. Discussion
4.1. Effect of initial GNSS station distribution on deformation capture ability
To explore the effect of the initial GNSS station distribution on the ability of the IDAL method to capture deformation, the simulated data and the layout of nine stations were considered as an example. The random layout (RL), uniform layout (UL), and empirical layout (EL) methods were used for the initial stations, which was followed by the application of the IDAL method to obtain a solution. The processes for solving the locations of these three different initial stations using on the IDAL method are named RL-IDAL, UL-IDAL, and EL-IDAL, respectively.
(a–c) show the deformation recovered using the RL-IDAL, UL-IDAL, and EL-IDAL methods, respectively: the distribution of the initial stations widely varies among the methods. However, these three completely different initial station layouts can recover the spatial characteristics of the deformation after being optimized using the IDAL method. The distribution of the red triangle stations shows that the positions of the optimized final stations are not considerably different, which preliminarily shows that the IDAL method is less dependent on the initial locations of the stations. (d–f) present the differences between (a–c) and the simulated deformation, respectively. After the initial stations of these three methods were optimized using the IDAL method, the error distributions of the recovered deformation were similar, and the magnitudes were relatively small. The error distribution histogram in (g–i), the error of the RL-IDAL method was slightly higher, the error of the EL-IDAL method was the smallest, and the errors of these three methods were close to a normal distribution.
Figure 11. The effect of different initial station locations on the results produced with the IDAL method (black triangles: fixed stations; white triangles: initial stations to be optimized; red triangles: stations optimized using IDAL method).

lists the MAE, STD, and RMSE values for the three methods. The EL-IDAL method was superior to the RL-IDAL and UL-IDAL methods for all indices. This is because the EL-IDAL method considers deformation, which helps guide the reasonable distribution of initial stations; thus, the optimized deformation accuracy obtained using this method was the highest.
Table 3. Statistical error analysis of RL-IDAL, UL-IDAL and EL-IDAL methods in (g–i).
The results of the above analysis show that the different initial station distributions weakly impact the final optimization results, showing that the IDAL method is relatively robust. However, from subtle differences, it can still be observed that the more reasonable the distribution of the initial stations, the higher the final optimization accuracy. This means that before determining station layout, a somewhat reliable initial station distribution can be provided according to the actual requirements and InSAR deformation results, which can help to further strengthen the spatial deformation monitoring capability of GNSS.
4.2. Effect of search thresholds on the efficiency of the IDAL method
shows the variation in the RMSE of the deformation on two different scales for different numbers of iterations during the optimization process. The RMSE relatively quickly changes when the initial station is optimized on large-scale CPs, and the rough solution quickly converges. When the rough solution is optimized on small-scale CPs, the change in the RMSE is small; however, it also quickly converges to obtain a refined solution. This confirms the validity of the theoretical method developed in this study.
Figure 12. When the number of stations (Ns) is 9, the RMSE of the stations obtained with the IDAL method varies with the number of iterations.

However, the distance threshold must be set for the iterative solution because the distance threshold at different scales affects the efficiency of the solution. Therefore, to study the rule for selecting the distance threshold at different scales, the simulated data and the layout of nine stations were again taken as examples to design an experiment of the influence of the combination of different distance thresholds on the accuracy and efficiency of the solution.
As shown in (a) and (b), as long as and
are sufficiently large, the optimization accuracy is not affected; however, the solution efficiency is seriously impacted. Moreover,
and
cannot be too small; otherwise, the CPs in some regions cannot be searched, resulting in low accuracy.
Figure 13. Effect of distance search threshold on different scales on IDAL (a) efficiency and (b) accuracy.

The results of the above experiment demonstrate that selecting the appropriate parameters can increase the efficiency by more than two times without affecting the accuracy, and the improvement is more noticeable when the study area is larger. Here, the selection of can be determined based on the initial stations. If the initial stations are reliable (such as those obtained using the EL method), a smaller
value could be used.
can be smaller because, after large-scale optimization, the positions of the stations are relatively accurate. To be safe, a value of 200–400 m can be chosen.
5. Conclusions
In this study, a GNSS observation network layout and an optimization method based on InSAR prior deformation information were developed. For an area experience ground deformation, the user only needs to provide a quality standard for deformation monitoring to rapidly obtain the number, spatial distribution, and corresponding deformation recovery accuracy of the GNSS station layout. The main findings are as follows:
The IDAL method fully uses of the advantages provided by InSAR technology in deformation monitoring, such as its large scale, high precision, and high spatial resolution. Considering factors such as spatial deformation monitoring accuracy, cost, topography, and the actual monitoring requirements of GNSS stations, according to the deformation spatial correlation, a multiscale iterative optimization method was adopted to take advantage of the ability of GNSS stations to capture the spatial characteristics of regional group deformation and increase the availability of GNSS deformation monitoring results.
Simulation experiments demonstrated that, compared with the traditional station layout methods that scarcely considered deformation distribution, the new method improved GNSS spatial deformation monitoring ability by 46%–68%. The algorithm exhibited high stability, efficient performance, and strong applicability. Utilizing ALOS2 data and the SBAS–InSAR method, the deformation of a mining area in Datong City, Shanxi Province, China, was assessed. The findings revealed that deployed GNSS stations could effectively capture the deformation of the mining area with local subsidence. In comparison with the InSAR deformation results, 89.6% of the deformation recovered by the new method exhibited a point error of less than 1.5 cm. In situations where InSAR data is unavailable, this method can be used as a substitute to promptly understand the safety and deformation status of photovoltaic equipment in the region. The proposed method provides guidance and a reliable basis for determining the station layout of GNSS deformation monitoring networks and provides a suitable supplement for the current GNSS station layout, which is conducive to the monitoring and understanding of geological disasters given the frequency of geological disasters.
In the future, this method will need to be extended to different ground deformation scenarios, such as landslides, earthquakes, and groundwater as InSAR technology matures and given the widespread availability of free SAR data, which reduces the difficulty of acquiring deformation information. However, due to the high dependence on the InSAR deformation results, the method will fail in some completely decoherent regions. Additionally, in this study, only InSAR LOS deformation data were considered. However, surface deformation occurs in the three-dimensional direction. In the future, attention will be paid to incorporating three-dimensional deformation results into the IDAL model.
Acknowledgements
The authors wish to thank the Japan Aerospace Exploration Agency (JAXA) for providing the ALOS-2/PALSAR-2 data.
Disclosure statement
No potential conflict of interest was reported by the author(s).
Data availability statement
The ALOS-2/PALSAR-2 data used in this paper can be applied for on the https://auig2.jaxa.jp/ips/home. Other data in this paper can be obtained by contacting the authors.
Additional information
Funding
References
- Berardino, P., G. Fornaro, R. Lanari, and E. Sansosti. 2002. “A New Algorithm for Surface Deformation Monitoring Based on Small Baseline Differential SAR Interferograms.” IEEE Transactions on Geoscience and Remote Sensing 40 (11): 2375–2383. https://doi.org/10.1109/TGRS.2002.803792.
- Bertsimas, Dimitris, and John Tsitsiklis. 1993. “Simulated Annealing.” Statistical Science 8 (1): 10–15. https://doi.org/10.1214/ss/1177011077.
- Decriem, J., Th Árnadóttir, A. Hooper, Halldór Geirsson, Freysteinn Sigmundsson, Marie Keiding, B. G. Ófeigsson, Sigrún Hreinsdóttir, Páll Einarsson, and P. LaFemina. 2010. “The 2008 May 29 Earthquake Doublet in SW Iceland.” Geophysical Journal International 181 (2): 1128–1146. https://doi.org/10.1111/j.1365-246X.2010.04565.x.
- DeVore, Ronald A., and Vladimir N. Temlyakov. 1996. “Some Remarks on Greedy Algorithms.” Advances in Computational Mathematics 5 (1): 173–187. https://doi.org/10.1007/BF02124742.
- Ferretti, Alessandro, Andrea Monti-Guarnieri, Claudio Prati, Fabio Rocca, and Didier Massonet. 2007. InSAR Principles-Guidelines for SAR Interferometry Processing and Interpretation. Vol. 19. https://ui.adsabs.harvard.edu/abs/2007ESATM..19 … ..F/abstract.
- Ge, Linlin, Shaowei Han, and Chris Rizos. 2000. “Interpolation of GPS Results Incorporating Geophysical and InSAR Information.” Earth, Planets and Space 52 (11): 999–1002. https://doi.org/10.1186/BF03352320.
- Goldstein, Richard M., and Charles L. Werner. 1998. “Radar Interferogram Filtering for Geophysical Applications.” Geophysical Research Letters 25 (21): 4035–4038. https://doi.org/10.1029/1998GL900033.
- Gudmundsson, Sverrir, Freysteinn Sigmundsson, and Jens Michael Carstensen. 2002. “Three-Dimensional Surface Motion Maps Estimated from Combined Interferometric Synthetic Aperture Radar and GPS Data.” Journal of Geophysical Research: Solid Earth 107 (B10): ETG-13. https://doi.org/10.1029/2001JB000283.
- Hansen, Per Christian, and Dianne Prost O’Leary. 1993. “The Use of the L-Curve in the Regularization of Discrete Ill-Posed Problems.” SIAM Journal on Scientific Computing 14 (6): 1487–1503. https://doi.org/10.1137/0914086.
- Hanssen, Ramon F. 2001. Radar Interferometry: Data Interpretation and Error Analysis. Vol. 2. Springer Science & Business Media. https://books.google.com/books?hl = zh-CN&lr = &id = bqNkJUk4wtMC&oi = fnd&pg = PA4&dq = RADAR+INTERFEROMETRY&ots = 8QbtmGD2cR&sig = UG5XACFCLTN5g92Zs6dj_sFhFeM.
- Hooper, Andrew, David Bekaert, Karsten Spaans, and Mahmut Arıkan. 2012. “Recent Advances in SAR Interferometry Time Series Analysis for Measuring Crustal Deformation.” Tectonophysics 514–517 (January): 1–13. https://doi.org/10.1016/j.tecto.2011.10.013.
- Houlie, Nicolas, Gareth J. Funning, and Roland Bürgmann. 2016. “Use of a GPS-Derived Troposphere Model to Improve InSAR Deformation Estimates in the San Gabriel Valley, California.” IEEE Transactions on Geoscience and Remote Sensing 54 (9): 5365–5374. https://doi.org/10.1109/TGRS.2016.2561971.
- Jia, Xiuming, Chao Ma, and Anyuan Zhao. 2008. “Environmental Investigation and Evaluation of Land Subsidence in the Datong Coalfield Based on InSAR Technology.” Acta Geologica Sinica-English Edition 82 (5): 1035–1044. https://doi.org/10.1111/j.1755-6724.2008.tb00660.x.
- Knospe, S., and S. Jonsson. 2010. “Covariance Estimation for dInSAR Surface Deformation Measurements in the Presence of Anisotropic Atmospheric Noise.” IEEE Transactions on Geoscience and Remote Sensing 48 (4): 2057–2065. https://doi.org/10.1109/TGRS.2009.2033937.
- Lanari, R., O. Mora, M. Manunta, J. J. Mallorqui, P. Berardino, and E. Sansosti. 2004. “A Small-Baseline Approach for Investigating Deformations on Full-Resolution Differential SAR Interferograms.” IEEE Transactions on Geoscience and Remote Sensing 42 (7): 1377–1386. https://doi.org/10.1109/TGRS.2004.828196.
- Li, Zhiwei, Xiaoli Ding, Chen Huang, G. Wadge, and D. W. Zheng. 2006. “Modeling of Atmospheric Effects on InSAR Measurements by Incorporating Terrain Elevation Information.” Journal of Atmospheric and Solar-Terrestrial Physics 68 (11): 1189–1194. https://doi.org/10.1016/j.jastp.2006.03.002.
- Liu, Ning, Wujiao Dai, Rock Santerre, Jun Hu, Qiang Shi, and Changjiang Yang. 2019. “High Spatio-Temporal Resolution Deformation Time Series With the Fusion of InSAR and GNSS Data Using Spatio-Temporal Random Effect Model.” IEEE Transactions on Geoscience and Remote Sensing 57 (1): 364–380. https://doi.org/10.1109/TGRS.2018.2854736.
- Macchiarulo, Valentina, Pietro Milillo, Chris Blenkinsopp, Cormac Reale, and Giorgia Giardina. 2022. “Multi-Temporal InSAR for Transport Infrastructure Monitoring: Recent Trends and Challenges.” In Proceedings of the Institution of Civil Engineers-Bridge Engineering, 1–26. Thomas Telford Ltd. https://doi.org/10.1680/jbren.21.00039.
- Mao, Wenxiang, Sai Wang, Bing Xu, Zhiwei Li, and Yan Zhu. 2021. “An Improved Phase Unwrapping Method Based on Hierarchical Networking and Constrained Adjustment.” Remote Sensing 13 (21): 4193. https://doi.org/10.3390/rs13214193.
- Massonnet, Didier, and Kurt L. Feigl. 1998. “Radar Interferometry and Its Application to Changes in the Earth’s Surface.” Reviews of Geophysics 36 (4): 441–500. https://doi.org/10.1029/97RG03139.
- Motagh, Mahdi, Roghayeh Shamshiri, Mahmud Haghshenas Haghighi, Hans-Ulrich Wetzel, Bahman Akbari, Hossein Nahavandchi, Sigrid Roessner, and Siavash Arabi. 2017. “Quantifying Groundwater Exploitation Induced Subsidence in the Rafsanjan Plain, Southeastern Iran, Using InSAR Time-Series and in Situ Measurements.” Engineering Geology 218: 134–151. https://doi.org/10.1016/j.enggeo.2017.01.011.
- Osmanoğlu, Batuhan, Filiz Sunar, Shimon Wdowinski, and Enrique Cabral-Cano. 2016. “Time Series Analysis of InSAR Data: Methods and Trends.” ISPRS Journal of Photogrammetry and Remote Sensing 115 (May): 90–102. https://doi.org/10.1016/j.isprsjprs.2015.10.003.
- Palamà, Riccardo, Michele Crosetto, Jacek Rapinski, Anna Barra, María Cuevas-González, Oriol Monserrat, Bruno Crippa, Natalia Kotulak, Marek Mróz, and Magdalena Mleczko. 2022. “A Multi-Temporal Small Baseline Interferometry Procedure Applied to Mining-Induced Deformation Monitoring.” Remote Sensing 14 (9): 2182. https://doi.org/10.3390/rs14092182.
- Pawluszek-Filipiak, Kamila, and Andrzej Borkowski. 2020. “Integration of DInSAR and SBAS Techniques to Determine Mining-Related Deformations Using Sentinel-1 Data: The Case Study of Rydułtowy Mine in Poland.” Remote Sensing 12 (2): 242. https://doi.org/10.3390/rs12020242.
- Pawłuszek-Filipiak, Kamila, Natalia Wielgocka, Damian Tondaś, and Andrzej Borkowski. 2023. “Monitoring Nonlinear and Fast Deformation Caused by Underground Mining Exploitation Using Multi-Temporal Sentinel-1 Radar Interferometry and Corner Reflectors: Application, Validation and Processing Obstacles.” International Journal of Digital Earth 16 (1): 251–271. https://doi.org/10.1080/17538947.2023.2166607.
- Petropoulos, George P., and Prashant K. Srivastava. 2021. GPS and GNSS Technology in Geosciences. Amsterdam: Elsevier.
- Reuveni, Yuval, Yehuda Bock, Xiaopeng Tong, and Angelyn W. Moore. 2015. “Calibrating Interferometric Synthetic Aperture Radar (InSAR) Images with Regional GPS Network Atmosphere Models.” Geophysical Journal International 202 (3): 2106–2119. https://doi.org/10.1093/gji/ggv253.
- Rosi, A., V. Tofani, L. Tanteri, C. Tacconi Stefanelli, A. Agostini, F. Catani, and N. Casagli. 2018. “The New Landslide Inventory of Tuscany (Italy) Updated with PS-InSAR: Geomorphological Features and Landslide Distribution.” Landslides 15 (1): 5–19. https://doi.org/10.1007/s10346-017-0861-4.
- Samsonov, Sergey, Kristy Tiampo, John Rundle, and Zhenhong Li. 2007. “Application of DInSAR-GPS Optimization for Derivation of Fine-Scale Surface Motion Maps of Southern California.” IEEE Transactions on Geoscience and Remote Sensing 45 (2): 512–521. https://doi.org/10.1109/TGRS.2006.887166.
- Sarsito, D. A., E. Kriswati, I. Meilano, H. Andreas, and D. Pradipta. 2019. “Volcano Deformation Monitoring Using Geodetic Method: Optimal Network Design.” IOP Conference Series: Earth and Environmental Science 389 (1): 012039. https://doi.org/10.1088/1755-1315/389/1/012039.
- Shahbeik, Shahab, Peyman Afzal, Parviz Moarefvand, and Mania Qumarsy. 2014. “Comparison between Ordinary Kriging (OK) and Inverse Distance Weighted (IDW) Based on Estimation Error. Case Study: Dardevey Iron Ore Deposit, NE Iran.” Arabian Journal of Geosciences 7 (9): 3693–3704. https://doi.org/10.1007/s12517-013-0978-2.
- Soldato, Matteo Del, Pierluigi Confuorto, Silvia Bianchini, Paolo Sbarra, and Nicola Casagli. 2021. “Review of Works Combining GNSS and InSAR in Europe.” Remote Sensing 13 (9): 1684. https://doi.org/10.3390/rs13091684.
- Torres, Ramon, Paul Snoeij, Dirk Geudtner, David Bibby, Malcolm Davidson, Evert Attema, Pierre Potin, BjÖrn Rommen, Nicolas Floury, and Mike Brown. 2012. “GMES Sentinel-1 Mission.” Remote Sensing of Environment 120: 9–24. https://doi.org/10.1016/j.rse.2011.05.028.
- Xue, Feiyang, Xiaolei Lv, Fangjia Dou, and Ye Yun. 2020. “A Review of Time-Series Interferometric SAR Techniques: A Tutorial for Surface Deformation Analysis.” IEEE Geoscience and Remote Sensing Magazine 8 (1): 22–42. https://doi.org/10.1109/MGRS.2019.2956165.
- Yan, Huineng, Wujiao Dai, Lei Xie, and Wenbin Xu. 2022. “Fusion of GNSS and InSAR Time Series Using the Improved STRE Model: Applications to the San Francisco Bay Area and Southern California.” Journal of Geodesy 96 (7): 47. https://doi.org/10.1007/s00190-022-01636-7.
- Yang, Zefa, Zhiwei Li, Jianjun Zhu, Yuedong Wang, and Lixin Wu. 2020. “Use of SAR/InSAR in Mining Deformation Monitoring, Parameter Inversion, and Forward Predictions: A Review.” IEEE Geoscience and Remote Sensing Magazine 8 (1): 71–90. https://doi.org/10.1109/MGRS.2019.2954824.
- Zhao, Chaoying, Zhong Lu, Qin Zhang, Chengsheng Yang, and Wu Zhu. 2014. “Mining Collapse Monitoring with SAR Imagery Data: A Case Study of Datong Mine, China.” Journal of Applied Remote Sensing 8 (1): 083574–083574. https://doi.org/10.1117/1.JRS.8.083574.
- Zheng, Wanji, Jun Hu, Zhong Lu, Xie Hu, Qian Sun, Jihong Liu, Jianjun Zhu, and Zhiwei Li. 2023. “Enhanced Kinematic Inversion of 3-D Displacements, Geometry, and Hydraulic Properties of a North-South Slow-Moving Landslide in Three Gorges Reservoir.” Journal of Geophysical Research: Solid Earth 128 (6): e2022JB026232. https://doi.org/10.1029/2022JB026232.
- Zhu, Jianjun, Zhiwei Li, and Jun Hu. 2017. “Research Progress and Methods of InSAR for Deformation Monitoring.” Acta Geodaetica et Cartographica Sinica 46 (10): 1717. https://doi.org/10.11947/j.AGCS.2017.20170350.
- Zou, Zhenyu, Zaisen Jiang, Yueju Cui, Long Zhang, Peng Wang, and Tai Liu. 2019. “Study on the Layout of GNSS Sites for Strike-Slip Faults.” Geophysical Journal International 219 (2): 1131–1137. https://doi.org/10.1093/gji/ggz353.
- Zulkifli, Nur Adilla, Ami Hassan Md Din, and Zainal Abidin Md Som. 2018. “Vertical Land Motion Quantification Using Space-Based Geodetic Methods: A Review.” IOP Conference Series: Earth and Environmental Science 169 (July): 012024. https://doi.org/10.1088/1755-1315/169/1/012024.