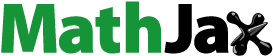
ABSTRACT
Tidal wetlands provide a variety of ecosystem services to coastal communities but suffer severe losses due to anthropogenic activities in the Yangtze River Estuary (YRE). However, the detailed dynamics of tidal wetlands have not been well studied with sufficient spatiotemporal resolution. Here, we proposed a rapid classification method that integrates the COntinuous monitoring of Land Disturbance (COLD) algorithm and Median Composite (MC) based on the dense Landsat time series to track the dynamic processes of tidal wetlands in the YRE from 1990 to 2020. The results showed that the COLD-MC demonstrated remarkable effectiveness in detecting the change of tidal wetlands and excellent overall accuracy and kappa coefficient ranging from 90% to 96% and 0.89–0.95, respectively. The overall accuracy of change detection was 97% with an absolute error of 0.4 years. We found that the total area of tidal wetlands experienced a net loss of 59.75 km2 in the YRE, but the gain and loss of the study period were 1556.07 and 1615.82 km2, respectively. Land reclamation, sediment reduction, and Spartina alterniflora invasion pose significant threats to tidal wetlands. Sustainable management could be implemented through the establishment of nature reserves and ecological sediment enhancement engineering projects.
1. Introduction
Tidal wetlands, thriving at the interface of terrestrial and marine ecosystems, primarily consist of mangroves, salt marshes, and mudflats (McLeod et al. Citation2011). They rank among the most productive ecosystems on the planet (Barbier et al. Citation2011; Davidson et al. Citation2019). Additionally, tidal wetlands play a crucial role in delivering a diverse range of irreplaceable ecosystem services, such as habitat provision for fauna and flora, biodiversity maintenance, coastal protection, erosion control, water purification, carbon sequestration, as well as raw materials and food provision, contributing to a staggering economic value of 20.4 trillion USD per year (Barbier et al. Citation2011; Castagno et al. Citation2021; Li et al. Citation2018; McLeod et al. Citation2011). Despite the vital economic and ecological importance of tidal wetlands, they are facing unprecedented pressures from climate change and anthropogenic activities (Murray et al. Citation2022). Over the period from 1999 to 2019, tidal wetlands experienced a net loss of 4,000 km2 globally (Murray et al. Citation2022), resulting in a substantial decline in the ecological functions that they provide (Li et al. Citation2018). Anthropogenic activities are considered the primary factor contributing to the loss of intertidal wetlands, particularly in developing nations with rapid economic development. For example, just from 1975 to 2015, a substantial decline of 2,953 km² was observed in the tidal wetlands along coastal regions of China due to extensive land reclamation (Song et al. Citation2020). Except for direct occupation by anthropogenic influences, tidal wetlands are subject to the impacts of various other factors such as dam construction, invasion of alien species, and sea level rise (Liu et al. Citation2018; Ma et al. Citation2014; Wang, Li, and Xiang Citation2022; Yang et al. Citation2006). Under the combined influence of various factors (Newton et al. Citation2020), tidal wetlands have become highly vulnerable ecosystems, casting a veil of uncertainty over their future fate. Therefore, comprehending the causes, processes, and future of tidal wetland changes is of great significance for the conservation, management, and restoration of tidal wetlands.
Due to the capability of satellites to make long-distance and large-scale earth observations with short-term revisits, mapping tidal wetlands using remote sensing images has become the primary method to study the dynamic changes of tidal wetlands (Huang et al. Citation2022; Sun, Liu, et al. Citation2021; Zhu Citation2017). With more than half a century of archived earth observation data, dense Landsat time-series data (DLTS) provides the longest temporal records of earth observation data with considerable temporal and spatial resolution, showing great potential in improving our knowledge of the dynamics of tidal wetlands under global change from vast coverage and long-term scale (Wu, Zhi, et al. Citation2022; Wulder et al. Citation2022). Moreover, the emergence of cloud computing platforms, such as Google Earth Engine (GEE), has created an environment for the invocation and computation of DLTS (Amani et al. Citation2020). Tidal wetland mapping categories that incorporate statistical methods to reduce tidal inundation, vegetation phonology, and cloud cover are widely developed. For example, Jia et al. calculated the maximum values of different spectral indices to extract the range of tidal wetlands and separated tidal flats using the Otsu algorithm (Jia et al. Citation2021). Wang and Wu et al. counted the annual frequency of marine water and tidal vegetation based on all available Landsat images and generated the annual maps of tidal wetlands (Wang et al. Citation2020; Wu, Zhi, et al. Citation2022). These studies account for spectral information over a certain period, typically 1–3 years, to eliminate the influence of short-term tidal dynamics, resulting in accurate maps of tidal wetlands. However, converting the temporal information of pixels into statistical data ignores the temporal continuity and correlation of pixels, which may lead to misclassification of tidal wetland categories under the influence of tidal inundation and vegetation phenology (Di Vittorio and Georgakakos Citation2018; Sagar et al. Citation2018). Furthermore, the thematic mapping of tidal wetlands still faces the challenge of not fully harnessing the potential of remote sensing data which record the entire developmental trajectory of tidal wetlands (Yang et al. Citation2022).
In recent years, several change detection algorithms have been developed to detect land use and land Cover (LULC) changes to make the most comprehensive use of all available remote sensing data. Such algorithms, including Landsat-based detection of Trends in Disturbance and Recovery (Landtrendr) (Kennedy, Yang, and Cohen Citation2010), Breaks For Additive Seasonal and Trend (BFAST) (Verbesselt et al. Citation2010), Continuous Change Detection and Classification (Zhu and Woodcock Citation2014), and COntinuous monitoring of Land Disturbance (COLD) (Zhu et al. Citation2020), detect changes by creating time-series models to track the development trajectories of each pixel (Zhu Citation2017). Based on the single band time series data, the Landtrendr and BFAST can detect external disturbances on a pixel scale (Rhif et al. Citation2022; Wu, Zhi, et al. Citation2022). Originally designed to monitor disturbances in terrestrial forests, Landtrendr and BFAST have found expanded utility in the monitoring of changes within intertidal wetlands (Chen et al. Citation2022; Wu, Zhi, et al. Citation2022). In comparison to Landtrendr and BFAST, CCDC and COLD, which calibrated based on various disturbance events including wetlands, combine multiple spectral bands to capture more LULC changes with higher accuracy (Zhu et al. Citation2020; Zhu and Woodcock Citation2014). The divergence lies in the fact that the COLD algorithm has updated the model initialization and change detection rules within the framework of the CCDC algorithm, leading to a notable enhancement in the precision of change detection (Zhu et al. Citation2020). In recent years, CCDC and COLD algorithm have been widely applied in the research of dynamic changes in tidal wetlands ecosystems, demonstrating substantial promise in unraveling the intricate dynamics of tidal wetland transformations (Awty-Carroll et al. Citation2019; Citation2021; Yang et al. Citation2022). However, condition change introduces the possibility of spurious changes being detected by the COLD algorithm, especially in active estuarine coastal regions, thereby potentially deviating the classification results from the ground truth (Yang et al. Citation2022). Moreover, the COLD algorithm has only been applied to a single category of mangrove or salt marsh vegetation, and it is less involved in mapping tidal wetlands of complicated classes. This issue underscores a critical challenge necessitating resolution within the scope of this study.
The Yangtze River Estuary (YRE) represents a pivotal ecological region with extensive tidal wetlands that serve as crucial supporters of multiple ecosystem services (Wu et al. Citation2003), and it stands as a prominent center of economic development in China (Sun, Zhu, and Cheng Citation2018). However, the rapid expansion of YRE's economy has attracted substantial human settlement and engendered profound anthropogenic stressors on tidal wetland habitats (Chen et al. Citation2018). Additionally, the Yangtze River Estuary is one of the areas that are most severely affected by the invasion of Spartina alterniflora in China (Liu et al. Citation2020). The integrated influences of human activities and climate changes have precipitated rapid transformations within the tidal wetlands of YRE (Tian et al. Citation2015). Consequently, delving into the dynamic fluctuations in the tidal wetlands of YRE serves as a representative marker, mirroring the broader panorama of tidal wetland changes not just within China but also on a global scale. However, due to the highly dynamic nature and high turbidity of the YRE, conventional mapping methods face challenges in generating a fine-scale tidal wetland spatial distribution dataset with high spatiotemporal resolution.
To address the aforementioned issues, we propose a rapid and robust classification method that can be applied to tidal wetlands with highly dynamic change and high turbidity based on statistical methods and change detection algorithm and generate a high spatiotemporal resolution spatial distribution dataset of tidal wetlands in the YRE covering the period from 1990 to 2020 using all available DLTS. From the results with higher spatiotemporal resolution derived from DLTS data, we aim to answer the following two questions:
How applicable is the classification method in highly dynamic estuarine coastal regions?
What are the distribution, dynamic changes, and drivers of the tidal wetlands in the YRE?
2. Data and methods
2.1. Study area
YRE is situated between Shanghai and Jiangsu Province, with geographical coordinates ranging from 30°33'38″ to 32°10'23″ N and 120°56'25″ to 122°16'15″ E. The Yangtze River carries abundant sediment into the estuary, with an annual average sediment discharge of 122 million tons in the last decade (Ministry of Water Resources of China Citation2022). YRE falls under the classification of a subtropical monsoon climate, characterized by an annual mean temperature of 17.3°C and precipitation of 1409.1 mm (Shanghai Statistical Bureau Citation2020). Ample sediment and hydrological conditions have contributed to the formation of diverse tidal wetland ecosystems, including Nanhui Dongtan, Jiuduansha, Chongming Dongtan, and Chongming Beitan. As habitats and wintering grounds for many migratory birds, Jiuduansha and Chongming Dongtang were listed as national nature reserves in 2005. The Yangtze River Delta is one of the fastest-growing economic regions in China. In recent years, as urbanization continues to accelerate, many large-scale reclamation activities and water conservancy projects have taken place along the coast, seriously squeezing the habitats of tidal wetlands. In this study, the land boundary was defined by the coastline in 1990, while the marine boundary was defined by the 15-km-wide buffer zone from the coastline in 2020. The area between the land boundary and the marine boundary is determined as the study area ().
2.2. Data and processing
2.2.1. Pre-processing of the DTLS
In this study, all available Landsat collections from 1 January 1990 to 31 December 2020, including Landsat 5, Landsat 7, and Landsat 8 images with a total number of 3227 were used to explore the dynamics of tidal wetlands in the YRE. We obtained these data from the United States Geological Survey official website (https://glovis.usgs.gov/). All images were geo-registered and inter-calibrated high-quality data across the Landsat instruments, making it well-suited for time series analysis (Awty-Carroll et al. Citation2019). Blue, Green, Red, Near Infrared (NIR), Short-wave Infrared 1 (SWIR1) and Short-wave Infrared 2 (SWIR2) bands were used in this study.
2.2.2. Sample dataset
Firstly, proportionable samples were generated according to the area of different LULC types in the YRE based on the GEE (Peng et al. Citation2023; Stehman and Xing Citation2022). Secondly, a multi-source incorporating high-resolution images from Google Earth, Sentinel-2 images and relevant reference, was employed for visual interpretation of these samples. In the absence of high-resolution data from historical periods, monthly Landsat images were synthesized using GEE. The interpretation of samples was conducted by considering various band combinations, taking into account spectral features, phenological characteristics, and geographical information. A total of 14,309 sample were obtained, including 1921 Phragmites australis samples, 1339 Scirpus mariqueter samples, 1454 Spartina alterniflora samples, 1684 tidal flats samples, 2129 marine water samples, 781 impervious surface samples, 1085 aquaculture ponds samples, 1211 land water samples, 1295 farmland samples and 1410 other LULC samples. The spatial distribution of the sample points in 2020 is shown in . Finally, we utilized the stratified sampling method to select validation and training samples (Jia et al. Citation2021).
2.2.3. Auxiliary data
The shoreline data for the YRE from 1990 to 2020 were obtained by visually interpreting Landsat images taken during high tide periods, with a temporal resolution of 2 years (Wu, Gao, et al. Citation2022). The social-economic data, such as Gross Domestic Product (GDP) and the resident population during the same period were requested from the Shanghai Bureau of Statistics (https://tjj.sh.gov.cn/index.html). We also collected the sediment data for the Yangtze River during the years 2000–2020 were sourced from the Information Center of the Ministry of Water Resources in China (http://xxzx.mwr.gov.cn/xxgk/gbjb/zghlnsgb/).
2.3. Methods for extracting tidal wetlands
The detailed workflow is presented in . First, time series models and synthesized annual spectral features were developed using the COLD algorithm and median composite method, respectively. Second, a random forest algorithm was employed to integrate the established time series models and synthesized annual spectral features as input features for the classifier, generating thematic maps of tidal wetlands from 1990 to 2020 in the YRE. Third, an analysis of the tidal wetland map was conducted based on the land use transition matrix and centroid migration model. Finally, the dynamic changes in the tidal wetlands at the YRE over the past three decades were delineated.
2.3.1. Time-series models construction
In this study, the COLD algorithm, which was developed for continuous monitoring of land disturbance and long-term LULC classification for large areas (Awty-Carroll et al. Citation2021; Zhu et al. Citation2020), was used for constructing time-series models of tidal wetlands in the YRE over the period from 1990 to 2020. The COLD algorithm employs the Fourier model to create time series models for various bands on a pixel-by-pixel basis, and then compares new observations to predicted values to detect LULC changes. Each time series model (Equation (1)) consists of a trend term and three harmonic components, where the trend term could capture the inter-annual variation characteristics of the time series, and the harmonic components are used to estimate the intra-annual variation characteristics of the time series (Xie et al. Citation2022).
(1)
(1) where
is the predicted value of surface reflectance for the ith Landsat band at the xth Julian day;
is the overall value of surface reflectance for the ith Landsat band (i.e. intercept);
indicates the inter-annual change of the ith band (i.e. slope);
represents the time frequency of harmonic components (
= 1, 2, and 3);
and
denote the intra-annual change of the ith Landsat band;
means the number of days per year, which is 365.25.
The operational procedure of the COLD algorithm is delineated into three principal steps. In the first step, stability testing is conducted during the model initialization phase because the changes occurring within this stage can impact the subsequent predictions and change detection of the model. Changes may occur at the beginning, middle, and end stages of model initialization, thereby causing alterations in the slope of the model. Model stability is evaluated based on Equation (2), which combines the absolute value of the slop of the time series model () and the larger absolute values between the difference in prediction value and clear Landsat observations at the start and end (
and
). The optimal outcome is attained when the change probability is set to 0.99 (Zhu et al. Citation2020). If the model is unstable, COLD automatically incorporates next clear observation and removes the earliest clear observation until the model stabilizes.
(2)
(2) In the second step, following stability testing, COLD incorporates new clear Landsat observation to update the existing time series model and detect changes by comparing the differences between clear observation and predicted value. The distinction between clear observation and predicted value is determined in COLD based on Equation (3). COLD assumes that the sum of the squares of model deviations follows a chi-square distribution (
), where the degrees of freedom are equal to the number of spectral bands used for change detection. Subsequently, a change probability of 0.99 is established based on the probability derived from the chi-square distribution (Equation (3)). Once six consecutive clear observations exceed the change probability threshold, COLD will flag it as a potential change. Given the approximate alignment of changes in direction, the COLD algorithm further confirms whether a real change has occurred by calculating the angle between each pair of neighboring change vectors for these six anomaly observations. The COLD algorithm specifies that a change event can be confirmed when the average angle of change vectors is less than 45° (Equation (4)).
(3)
(3) where
and
is the actual predicted value of surface reflectance for the ith Landsat band at the xth Julian day;
is the number of bands used for change detection.
(4)
(4) where
is the ith anomaly observation;
means the number of consecutive anomaly observations.
represents the neighboring change vectors between the ith and i + 1th anomaly observation.
Finally, once a change is detected, the time series model undergoes disruption, resulting in a breakpoint representing the occurrence of the change event. Afterward, a new time series model is established to search for the next breakpoint, and this process continues until clear Landsat observations are exhausted. All the spatial–temporal information of time series models on a pixel-by-pixel basis will be recorded in a specific data format and processed into raster files ().
Table 1. Output of the COLD algorithm.
An instance of change detection utilizing the COLD algorithm for a pixel (31.7553°N,121.59°E) which undergone LULC change once over the past three decades in . The COLD algorithm has constructed two segments of the time series model spanning the periods 1997–2018 and 2018–2020 for this pixel across multiple spectral bands, capturing the LULC change that occurred in 2018 ((a)). By examining historical high-resolution images from Google Earth Pro, it is revealed that the LULC type of the pixel was identified as marine water from 1990 to 2017. However, in 2018, it underwent reclamation and transformed into land ((b)). describes the output variables of the COLD algorithm.
2.3.2. Synthetic annual images
We noticed the presence of errors within the constructed time series model (). These deviations, arising from dynamic tidal inundation and non-LULC type alterations, introduced condition changes (Yang et al. Citation2022). The accumulation of these errors will lead to a decline in the accuracy of thematic maps. Therefore, we attempted to reflect the genuine ground conditions of each year by synthesizing annual images and integrating them into the classifier to mitigate such errors. Currently, the mainstream approach for the annual composite of remote sensing images in tidal wetlands is the maximum value composite (MVC) method, known for effectively delineating the boundaries of tidal wetlands (Jia et al. Citation2021). However, in estuaries with high turbidity like the YRE, the use of MVC amplifies the spectral information of turbidity ((a)), potentially leading to an over-identification of tidal flats in classification (Jia et al. Citation2021). Conversely, the median synthesis method weakens the influence of turbidity by considering the annual median value, reducing the risk of misidentification ((b)). Moreover, we found that MVC-derived images frequently exhibit noise and blank regions and images synthesized using the median composite (MC) method exhibit higher quality with minimal noise and blank areas (). The MC sets each pixel in the composite based on which pixel in the time series Landsat image collection has the median value of spectral bands. In this study, the synthesized annual images consist of six bands, including SWIR1, SWIR2, NIR, RED, GREEN, and BLUE, which almost maintain consistency with the bands used for constructing the time-series model and encompass most of the spectral information from the ground (Zhu et al. Citation2020).
2.3.3. Classification
We utilized the Random Forest (RF) algorithm to classify each time series model into 10 LULC types from 1990 to 2020 using Google Earth Engine (GEE) which is a cloud platform that enables rapid classification and efficient processing of large volumes of data (Amani et al. Citation2020). RF is considered one of the most effective machine-learning classifiers for LULC classification due to its ability to adapt to diverse datasets and account for correlations and interactions among features (Chen and Ishwaran Citation2012). The number of decision trees in the random forest is set to 100, with mtry defaulting to the square root of the variable count. The LULC types were categorized into tidal wetlands (Phragmites australis, Scirpus mariqueter, Spartina alterniflora, and tidal flats) and none-tidal wetlands (impervious surface, aquaculture ponds, land water, farmland, marine water, and others (unused land and terrestrial vegetation)) (). The input features for the classifier consist of all coefficients and root mean square error (RMSE) of the time series model () and annual images synthesized in section 2.3.2. The LULC of the tidal zone was classified from 1990 to 2020 with an interval of two years. Since the model was initialized in the initial year, the accuracy of classification may be affected. Due to the minimal changes observed between the satellite images of 1991 and 1990, we used the image of 1991 to represent the classification of 1990.
Table 2. Tidal wetland categories in the YRE.
2.4. Accuracy assessment
The accuracy assessment strategy for change maps and LULC maps is based on the stratified random sampling approach (Olofsson et al. Citation2014). For the change map, a total number of 600 random sampling points were generated, consisting of 387 stable points and 213 changed points. Additionally, 4209 random sampling points were generated for LULC maps. Then, all sampling points were interpreted with very high-resolution (VHR) imageries accessible in Google Earth Pro. Based on stratified reference samples, confusion matrixes are calculated including the user’s accuracy (UA), producer’s accuracy (PA), kappa coefficient (Kappa), and overall accuracy (OA) for change map and LULC maps (Olofsson et al. Citation2014). We also calculated the F1 score for the change map and the average of PA and UA (APA and AUA) for LULC maps based on confusion matrixes. Furthermore, a comparative analysis was conducted between the detected change years and the interpreted change years, aiming to provide a comprehensive evaluation of the accuracy of the change results.
2.5. Dynamic analysis method for tidal wetlands
2.5.1. Land use transition matrix
The land use transition matrix reveals both the direction and quantity of transitions between different LULC types, offering valuable insights into the spatiotemporal dynamics inherent in land use patterns (Eq (5)). In this study, the land use transition matrix is employed to investigate the spatial distribution of gains and losses in tidal wetlands of the YRE, quantifying the transitions into and out of tidal wetlands during different periods.
(5)
(5) where
represents the area of land use; n represents the number of land use types;
and
represent the land-use types before and after the transition;
represents the area of land use type
transitioning to land use type
during the study period.
2.5.2. Centroid migration model
The Centroid migration model can evaluate the spatiotemporal dynamics of tidal wetlands by computing centroids across different years and quantifying inter-centroid distances (Eq (6)&(7)). The centroid serves as a representation of the spatial distribution of tidal wetlands, while the migration distance of centroids serves as a quantitative measure of the extent of change within tidal wetlands. Based on the mean center tool in ArcGIS 10.7, we compute the centroid migration of tidal wetlands in the YRE from 1990 to 2020.
(6)
(6)
(7)
(7) where
and
represent the longitude and latitude coordinates of the centroid of tidal wetland area distribution in year
, respectively; n denotes the number of landscape patches of tidal wetland.
represents the area of the ith patch in year
;
and
represent the longitude and latitude coordinates of the ith patch in year
, respectively.
3. Results
3.1. Accuracy assessment
3.1.1. Performance of the COLD algorithm in tidal wetlands
All pixels were categorized into stable and changed pixels, and the results of change detection were validated based on a confusion matrix (). The Overall accuracy of the change map is 97%, with a kappa coefficient of 0.94. The PA and UA for stable and changed pixels are all above 94%. The F1 scores reached 0.96 and 0.98. Results also showed that the detected change years exhibited a high degree of consistency with the interpreted change years, suggesting the robust capability of the COLD algorithm in accurately capturing the occurrence time of majority change events (). The majority of deviations from the actual occurrence of change events fall within the range of 1–3 years, and the mean average error in validating the change detection results is 0.4 years. The application of the COLD algorithm for change detection demonstrated remarkable outcomes.
Table 3. Confusion matrix of change detection results.
3.1.2. Accuracy assessment for LULC maps
shows the accuracy assessment of LULC maps from 1990 to 2020. The OA of LULC maps ranged from 90% to 96% and the Kappa ranged from 0.89 to 0.95. The APA and AUA of classification maps also achieved satisfactory results with high values of around 90%. With the exception of specific years, the consistency in precision evaluation metrics across different years remains relatively stable on the LULC maps. The results demonstrated that the LULC map is highly consistent with real ground conditions, which can be used for subsequent analyses.
3.2. Status and historical change of tidal wetlands in YRE
Tidal wetlands exhibit extensive distribution across YRE, encompassing vast expanses of wetland habitats in prominent regions including Chongming Beitan ((a)), Chongming Dongtan ((b)), Jiuduansha ((c)), and Nanhui Dongtan ((d)). The total extent of tidal wetlands is 667.5 km2 in 2020, including 95.06 km2 of Phragmites australis, 80.9 km2 of Scirpus mariqueter, 151.1 km2 of Spartina alterniflora, and 340.44 km2 of tidal flats, respectively. Both Scirpus mariqueter and tidal flats are distributed throughout the YRE. Phragmites australis populations predominantly inhabit the southern expanse of Chongming Dongtan and Shansha of Jiuduansha, while Spartina alterniflora is mainly distributed in Chongming Beitan, and Zhongsha and Xiasha of Jiuduansha, as well as Nanhui Dongtan.
Figure 8. Type and spatial distribution of tidal wetland habitats in YRE in 2020. (a) Chongming Beitan. (b) Chongming Dongtan. (c) Jiuduansha. (d) Nanhui Dongtan. (e) Proportions of different LULC categories in YRE. The colors in e correspond to the legend.

The total area of tidal wetlands in YRE decreased slightly from 727.25 km2 to 667.5 km2 from 1990 to 2020. However, it exhibited distinct patterns over the past three decades, which can be divided into three stages: (1) gradual expansion from 1990 to 1996, (2) rapid decline from 1996 to 2010, and (3) a period of accelerated growth from 2010 to 2020 (). During the initial stage, the tidal wetlands showed a trend of increasing by 10.64 km2/a. In the subsequent stage, the wetlands experienced a remarkable decline with a slope twice as steep as the previous stage (), resulting in a loss of 383.06 km2 of tidal wetlands with an average rate of 23.55 km2/a. Notably, this phase was characterized by the emergence of the invasive species of tidal wetland vegetation, Spartina alterniflora, encompassing an area of 54.17 km2. The final stage witnessed a rebound of tidal wetlands, with tidal wetlands expanding at a rate of 18.67 km2/a. The overall vegetation area of tidal wetlands increased from 292.92 km2 to 327.09 km2 over the period from 1990 to 2020. However, the invasive species, Spartina alterniflora, gradually became the dominant wetland vegetation with an area of 151.1 km2 in 2020, almost approaching the total extent of Phragmites australis and Scirpus mariqueter with an area of 175.96 km2. Although there have been modest recoveries in the areas of Phragmites australis, Scirpus mariqueter, and tidal flats, they have yet to reach their original extents. Particularly noteworthy is the diminished presence of Scirpus mariqueter, which now occupies less than half of its extent in 1990.
The tidal wetlands also exhibited seaward movement. Notably, the largest movement occurred in Nanhui Dongtan, and the seaward movement of the centroid was 8.54 km from 1990 to 2020 ((d)), while Jiuduansha is the smallest with a distance of 1.66 km ((c)). Moreover, Chongming Beitan ((a)) and Chongming Dongtan ((b)) also showed seaward movement with the distance of 2.57 and 3.43 km, respectively. Consequently, over the last three decades, there has been a remarkable reduction in marine water, reaching an extensive area of 853.16 km2.
3.3. Gains and losses of tidal wetlands in YRE
The gains and losses of coastal wetlands fluctuated in the YRE (). Over the period spanning 1990–2020, an extensive area of 1556.07 km2 was gained, while a substantial extent of 1615.82 km2 was concurrently lost, ultimately resulting in a net loss of 59.75 km2. The total ratio of wetland gains to losses is 0.96, highlighting a quasi-equilibrium that existed in the dynamics of tidal wetlands in the YRE over the study period. The tidal wetlands in YRE suffered the most severe losses between 1998 and 2000 and experienced the greatest gains from 2010 to 2012 with a total area of 158.81 and 170.01 km2, respectively.
Figure 11. (a) Gains and losses of tidal wetlands from 1990 to 2020. (b) Gain/loss ratio of tidal wetlands from 1990 to 2020.

The gains and losses of tidal wetlands exhibit a spatial variation ( and ). The gains of tidal wetlands due to the natural deposition were characterized by the gradual expansive belt towards the sea, such as Chongming Island and Jiuduan Island (). However, the gains of coastal wetlands were little in the position where along the main channel of Yangtze Estuary, such as the Southen Chongming Dongtan Wetland near to the North Channel, and Southern Jiuduansha along the South Passage ((b and c)). The significant gains of coastal wetlands from 2016 to 2020 in Nanhui Dongtan Wetland ((d)) resulted from the sedimentation promotion project. Almost 104.35 km2 of tidal wetlands were formed rapidly.
Figure 12. Gain of tidal wetlands in YRE during 1990–2020. (a) Chongming Beitan. (b) Chongming Dongtan. (c) Jiuduansha. (d) Nanhui Dongtan.

Figure 13. Loss of tidal wetlands in YRE during 1990–2020. (a) Chongming Beitan. (b) Chongming Dongtan. (c) Jiuduansha. (d) Nanhui Dongtan.

The losses of tidal wetlands are affected by extensive land reclamation and natural erosion. A net area of 587.42 km2 of coastal wetlands in the YRE was reclaimed from 1990 to 2020, which most showed regular shapes in space (). Moreover, a substantial decline in tidal wetland area was observed in spatial, with a notable emphasis on Chongming Dongtan ((b)) and Nanhui Dongtan ((d)). The prevailing trend of area loss was predominantly directed from land to sea, primarily occurring before 2010. Additionally, a continuous loss of area from sea to land due to erosion was also identified in the southeastern region of Xiasha of Jiuduan Sha ((c)).
The inflow and outflow of tidal wetlands have undergone various changes in the past 30 years (). During the periods spanning from 1990 to 2000 and from 2000 to 2010, a declining trend in tidal wetlands was observed. Interestingly, a contrasting trend of wetland expansion emerged during the period from 2010 to 2020. During the period from 1990 to 2010, a considerable number of tidal wetlands were reclaimed, with respective areas of 274.66 and 205.41 km2. A noticeable decline in reclamation activities occurred after 2010, leading to a reduced extent of tidal wetland reclamation, encompassing an area of 107.35 km2. Among all the reclaimed tidal wetlands, ‘Others’ (unused land and terrestrial vegetation) represents the main type of wetland conversion. Over the period spanning 1990–2010, this category witnessed large conversion extents of 127.99 and 105.49 km2, which declined to 47.12 km2 during 2010–2020. Notably, the conversion to ‘Others’ accounted for approximately 50% of the total reclaimed area in each period. The remaining reclaimed areas consist of impervious surfaces, aquaculture ponds, land water, and farmland. Marine water is also one of the major types of tidal wetlands conversion, with areas of 85.33 and 92.94 km2 being converted during the periods of 1990–2010. After 2010, the conversion of marine water decreased significantly to 24.17 km2. Moreover, marine water is the primary type contributing to the influx of tidal wetlands. Over the three periods, it replenished tidal wetlands with areas of 209.55, 139.36, and 374.73 km2, respectively. The contribution of other LULC types to the influx of tidal wetlands can be deemed inconsequential.
Figure 14. LULC transition in the tidal zone from 1990 to 2020. Tidal wetlands: Phragmites australis, Scirpus mariqueter, Spartina alterniflora, and tidal flats. MW: marine water; IS: impervious surface; AP: aquaculture ponds; LW: land water; FL: farmland; OT: others (unused land and terrestrial vegetation). The features marked with black dashed rectangular boxes are tidal wetlands. The thickness of the lines represents the area transferred in and out.

4. Discussion
4.1. Adaptability of the method
4.1.1. Reliability of tidal wetland mapping
In this investigation, we presented a method named COLD-MC for mapping the tidal wetlands in YRE based on all available Landsat images with highly accurate. The successful implementation of this investigation can be attributed to open data sources, robust algorithms, and the powerful computational capabilities of the GEE platform. Firstly, the availability of open-source Landsat time-series data has provided a wealth of clear observational data, offering the potential for continuous monitoring of highly dynamic tidal wetlands. Secondly, the COLD algorithm maximizes the use of all available clear pixels by continuously tracking the developmental changes of each pixel, provides temporal information about tidal wetlands, and effectively mitigates the short-term impacts caused by dynamic tidal inundation. Concurrently, owing to the elevated sediment concentration in estuaries, marine water reflectance experiences an increase in specific bands at certain times within a specific year. The MC algorithm weakens the influence of high turbidity in estuaries and avoids the occurrence of extreme values by selecting median values compared to MVC ( & (a)), generating high-quality images representing the annual ground conditions and significantly reducing classification errors attributed to the time-series models constructed by the COLD algorithm ( & (b)). For example, under the same conditions, MC provides a more comprehensive extraction of the marginal tidal flats ((a)), achieving a more thorough separation between turbid marine water and tidal flats (). The integration of continuous change detection algorithms and statistical methods effectively mitigated the uncertainty arising from environmental factors ( COLD_MC). Lastly, the GEE platform offers an environment for extensive remote sensing image access, processing, and computation (Amani et al. Citation2020). The approach demonstrated remarkable success within the challenging zones of the YRE which are characterized by high turbidity and dynamic environmental shifts, paving the way for potential applications in broader regions in the future.
Figure 15. Classification results of different strategies in Jiuduansha. The distinctions in classification are denoted within the confines of the black rectangular frame. The reference Landsat 8 image was acquired on 2020/05/12. R:G:B = 5:4:3.
Note: The results here have not undergone any post-processing.

Table 4. Different strategies for classification accuracy.
4.1.2. Comparison with other datasets
We compared the tidal wetland vegetation and tidal flats in our tidal wetland map (TWM) with two other publicly available datasets, respectively (). One dataset was obtained from Wang et al., who calculated the annual frequency of marine water and tidal wetland based on all available Landsat images and applied a decision tree to generate the annual map of tidal wetlands in YRE in 2020 at 30-m spatial resolution (FUDAN/OU) (Wang et al. Citation2023). Another dataset was obtained from Jia et al., who extracted a 10-meter resolution map of tidal flats of China in 2020 using the maximum composite method and OUST algorithm (CTF) based on Sentinel-2 images between 1 January 2019 and 30 June 2020 (Jia et al. Citation2021).
Figure 16. Comparison of tidal wetland areas in the YRE between our tidal wetland map (TWM) in 2020 and the FUDAN/OU map in 2020 (Wang et al. Citation2023) and the CTF map in 2020 (Jia et al. Citation2021). (a) Comparison of vegetation area in tidal wetlands. (b) Comparison of tidal flats area in tidal wetlands

Figure 17. Subset views of tidal wetland vegetation from our tidal wetland map (TWM) and the FUDAN/OU map in 2020 (Wang et al. Citation2023) in Chongming Beitan (A), Jiuduansha (B) and Nanhui Dongtan (C). The background Landsat 8 image was acquired on 2020/08/16. R:G:B = 5:4:3.

Figure 18. Subset views of tidal flats from our tidal wetland map (TWM), the FUDAN/OU map in 2020 (Wang et al. Citation2023), and the CTF map (Jia et al. Citation2021) in Chongming Beitan (A), Jiuduansha (B) and Nanhui Dongtan (C). The background Landsat 8 image was acquired on 2020/05/12. R:G:B = 5:4:3.

In comparison to the FUDAN/OU map, both datasets exhibited a remarkably high consistency in tidal wetland vegetation ((a)), as FUDAN/OU reported the area to be 350 km2, and the area we extracted was 327.06 km2. Additionally, their spatial distributions exhibited a striking congruence (). The slight differences in tidal wetland vegetation areas between the TWM and FUDAN/OU datasets may be attributed to mapping methods. The tidal wetland vegetation in FUDAN/OU was separated through a decision tree. In the YRE, Scirpus mariqueter and tidal flats, which are located on low tidal zones, tend to intermingle. Consequently, utilizing a decision tree often leads to misclassifying these mixed pixels (Wang et al. Citation2020). However, the FUDAN/OU dataset (200.39 km2) detected fewer tidal flats than our study (340.44 km2) ((b)). The primary factor accounting for this disparity is attributed to mapping methods. Due to the influence of clouds and fog in coastal areas, clear Landsat pixels are already scarce (Zhi, Wu, and Su Citation2022). Moreover, the impact of tidal inundation may result in a higher frequency of pixels being classified as water rather than tidal flats ( B_FUDAN/OU, C_FUDAN/OU). Therefore, under the same data source conditions, eliminating short-term tidal inundation effects necessitates considering the long-term developmental trajectory of pixels ().
The TWM dataset had a much smaller tidal flats area than the CTF dataset, with 340.44 and 437.73 km2, respectively ((b)). The differences between the TWM and CTF datasets can be attributed to the data sources and mapping methods. Despite the higher spatial and temporal resolution of Sentinel-2, the practice of compressing short-term data into a group of maximum values data may result in the loss of essential temporal information in tidal wetlands (Yang et al. Citation2022), such as the phenological information of vegetation. A considerable portion of tidal wetland vegetation in the CTF dataset had been categorized as tidal flats, leading to an overestimation of the areas ().
4.1.3. Uncertainties in tidal wetland mapping
The uncertainties of tidal wetland maps were primarily caused by image data, mapping algorithms and inappropriate training data. Firstly, despite utilizing the QA band to eliminate low-quality pixels, it is impossible to attain entirely high-quality pixels, which may introduce uncertainty into the resultant maps (Wang et al. Citation2020). Additionally, the quantity of clear observations for different pixels ranges from 114 to 705 (), with an inconsistent spatial distribution that may impact the accuracy of change detection algorithms. Multiple data sources will be considered to enhance clear observations in future research, such as dense Sentinel-2 images. Secondly, the median composite method may lead to an underestimation of tidal flats, which could contribute to the smaller area of tidal flats in the TWM compared to the CTF ((b)). Thirdly, given the analogous features of Phragmites australis and Spartina alterniflora in remote sensing images, visual interpretation of sample points is solely reliant on phenological characteristics. In regions characterized by mixed vegetation, the potential for misclassification may contribute to a decline in overall classification accuracy (Liu et al. Citation2022). Moreover, pixel-based classification is susceptible to pronounced salt-and-pepper artifacts. A label similarity probability filter may be used for post-classification to improve accuracy (Sun, Yang, et al. Citation2021). Finally, despite utilizing all available time-series remote sensing images to accurately extract intertidal flats, the duration of local tidal flats covered by marine water is significantly higher than their exposed duration, resulting in incomplete extraction of some local tidal flats (Jia et al. Citation2021; Peng et al. Citation2024; Zhang, Xu, et al. Citation2022).
4.2. Challenges faced by tidal wetlands under rapid dynamic changes
Intensive land reclamation has been conducted due to accelerated urbanization in the YRE, which resulted in substantial losses of tidal wetlands (Cheng et al. Citation2023; Sun, Zhu, and Cheng Citation2018; Tian et al. Citation2016). A significant area of 587.42 km2 of tidal wetlands has been reclaimed for urban expansion ((I)), agricultural cultivation ((II)), aquaculture ((III)), and the construction of reservoirs ((IV)), which accounts for the decrease of intertidal wetlands in the second phase of . In addition, reclamation-induced loss of intertidal wetlands is nearly irreversible, with only 5.39 km2 of reclaimed land reverting to tidal wetlands () (Liu et al. Citation2020; Tian et al. Citation2015; Wu et al. Citation2018). Consequently, following extensive land reclamation, the majority of tidal wetlands undergo significant displacement from their original positions ().
Figure 19. Typical land reclamation of the tidal zone in YRE. (I) Pudong Airpot; (II) Qianshao farm; (III) aquaculture pond; (IV) Qingcaosha Reservoir.

Declining sediment supply caused by large-scale dam construction along the Yangtze River is also one of the reasons for changes in intertidal wetlands, particularly following the operation of the Three Gorges Dam (TGD) (Yang et al. Citation2021). During the period from the initial impoundment of the TGD in 2003 to its peak water level in 2010 (Wang et al. Citation2013), the sediment supply experienced a pronounced decline (), slowing down the expansion rate of tidal wetlands and even resulting in loss of subaqueous delta in the YRE (Yang et al. Citation2015). After the TGD reached its maximum water level, the sediment supply to the YRE peaked in 2012, possibly contributing to an increase in the area of tidal flats that year (). During the period of reduced sediment, the vegetation within tidal wetlands has exhibited a sustained and relatively stable rate of accretion, particularly with an elevation rise in the vertical dimension (Shi et al. Citation2022; Yang et al. Citation2008; Zhang, Zhang, et al. Citation2022). In contrast, tidal flats suffer from a rapid decline in sediment rates, with localized areas transitioning from accretion to erosion due to the sediment rate decreases to a level lower than the rate of sea-level rise ((c)) (Crosby et al. Citation2016; Shi et al. Citation2022). This transition is particularly pronounced in the tidal wetland zones between −5-meter and 0-meter isobath, where a discernible reduction in the area has been observed (Shi et al. Citation2022). Therefore, the surface of tidal wetland was progressively becoming steeper and narrower, impeding outward expansion through sediment deposition.
The invasion of Spartina alterniflora is also recognized as one of the key factors driving dynamic changes in tidal wetlands (Liu et al. Citation2018). In 1997, the Shanghai municipal government introduced a limited area of Spartina alterniflora to Jiuduansha through ecological engineering, and it was not until 2000 that the presence of Spartina alterniflora was observed from Landsat images. Spartina alterniflora expands from 3.86 km2 in 2000–151.1 km2 in 2020 at a rate of 7.36 km2/a, and constitutes 46.2% of the tidal wetland vegetation in the YRE, making it the dominant vegetation in the tidal zone ( and ). Therefore, Spartina alterniflora plays a crucial role in maintaining the dynamic equilibrium of tidal wetland areas. However, the rapid expansion of Spartina alterniflora comes at the expense of displacing native vegetation habitats, especially Scirpus mariqueter (Shi et al. Citation2022). The Scirpus mariqueter community provides critical habitats and food for diverse waterbird species (Ma et al. Citation2017). As the habitat of Scirpus mariqueter communities diminishes, there has been a discernible reduction in the abundance and diversity of waterbird species (Ge et al. Citation2009; Ma et al. Citation2009; Zou et al. Citation2016). In addition, the prominent silt-promoting capacity of Spartina alterniflora leads to a rapid increase in elevation, while tidal creeks within the community exhibit increased density and reduced connectivity (Hou et al. Citation2013; Zong et al. Citation2023). Furthermore, the biogenic barriers formed by Spartina alterniflora communities restrict the inward extension of tidal creeks, hindering the entry of tidal water into Spartina alterniflora salt marshes or higher tidal flats (Hou et al. Citation2013; Shen, Zhang, and Wang Citation2003; Zong et al. Citation2023). Consequently, a cross-shore circulation pattern emerges on the outer side, triggering sediment erosion at the marsh edge (Shen, Zhang, and Wang Citation2003).
4.3. Protection and restoration of tidal wetlands
Despite the sustained loss of tidal wetlands throughout the past three decades, the net losses of tidal wetlands in the YRE amount to a mere 59.75 km2, accounting for less than one-tenth of the extent in 1990. This is attributed to the efforts of the local government in the protection and restoration of tidal wetlands, such as establishing nature reserves and implementing ecological sediment promotion engineering projects (ESPEP) (Yuan et al. Citation2022). Jiuduansha Wetland National Nature Reserve and ESPEP in Nanhui Dongtan wetland serve as typical representatives. In 1997, the government introduced Spartina alterniflora and Phragmites australis in Jiuduansha. Subsequently, the establishment of the Jiuduansha Wetland National Nature Reserve has provided favorable conditions for the natural expansion of wetlands in Jiuduansha while effectively minimizing anthropogenic disturbances. During the past three decades, Jiuduansha wetland has experienced notable natural expansion, witnessing a substantial increase in its extent from 38.96 km2 in 1990–155.33 km2 in 2020 at a rate of 3.88 km2/a (). Contrasting the protracted and gradual natural progression of wetland development, ESPEP has expedited the process of wetland formation. The construction of groins and breakwaters in ESPEP has effectively weakened hydrodynamic forces (Wu, Yang, et al. Citation2022), facilitating sediment accumulation and accelerating the formation of tidal wetlands within the project area (Yuan et al. Citation2022). Nanhui Dongtan wetland, characterized as one of the most severely reclaimed areas in YRE, has formed rapidly after the conduction of the ESPEP (2013–2018), with a growth rate of 13.38 km2/a, almost elevenfold compared to the area observed in 2012 (). Notably, Jiuduansha and Nanhui Dongtan wetlands alone contribute to 40% of the total tidal wetland area in the YRE. However, a considerable abundance of Spartina alterniflora was observed in both Jiuduansha Wetland National Nature Reserve and Nanhui Dongtan wetland. Therefore, the proposition of Spartina alterniflora removal within the YRE and the implementation of the native wetland vegetation restoration project are under consideration.
Figure 21. The dynamic changes of Jiuduansha Wetland National Nature Reserve from 1990 to 2020. (a) Landsat image of Jiuduansha wetland. (b) Bar chart illustrating the changes in the area of Jiuduansha wetland. Subfigures (c)∼(f) represent the spatial changes in the area of Jiuduansha wetland in 1990, 2000, 2010, and 2020, respectively.

Figure 22. The dynamic changes of Nanhui Dongtan wetland from 2012 to 2020 (a) The breakwaters, groins, and shoreline of Nanhui Dongtan wetland. (b) Bar chart illustrating the changes in the area of Nanhui Dongtan wetland. Subfigures(c)∼(f) represent the spatial changes in the area of Jiuduansha wetland in 2012, 2014, 2018, and 2020, respectively.

5. Conclusion
In this study, we developed a rapid classification method for tidal wetlands, integrating COLD algorithms with MC, and utilized all available Landsat remote sensing images to track the spatiotemporal changes and explore driving factors for the tidal wetlands of the YRE over the past 30 years. The results showed that our method achieved satisfactory classification results in the tidal wetlands with an accuracy range of 90% to 96%. 667.5 km2 of tidal wetlands were present in the tidal zone of YRE in 2020, a decrease of 59.75 km2 compared to 1990. Anthropogenic activities were the primary drivers, which resulted in the migration of the tidal wetlands habitat towards the sea. The refinement of relevant legal and policy frameworks has led to a reduction in the intensity of land reclamation activities. The diminished sediment supply and the invasion of Spartina alterniflora have also exerted substantial influences on the genesis and ecological structure of tidal wetlands, respectively. Concurrently, the construction of natural reserves and the implementation of ESPEP have resulted in the formation of new tidal wetland habitats, mitigating a significant portion of the previously incurred losses. The discoveries in this study provide crucial insights for the government and stakeholders, enabling them to tackle the multifaceted challenges associated with the management, restoration, conservation, and sustainable development of the tidal wetlands in the YRE.
Acknowledgment
This research was funded by the National Natural Science Foundation of China (Grant No. 42301540), the Open Research Fund of State Key Laboratory of Estuarine and Coastal Research (Grant number SKLEC-KF202307), the Natural Science Foundation of Fujian Province (Grant No. 2022J05024), the Education Department of Fujian Province (Grant No. JAT210027), and the Natural Science Foundation for Distinguished Young Scholars of Fujian Province, China (Grant No. 2021J06014).
Data availability statement
The data presented in this study are available on request from the corresponding author.
Disclosure statement
No potential conflict of interest was reported by the author(s).
Additional information
Funding
References
- Amani, M., A. Ghorbanian, S. A. Ahmadi, M. Kakooei, A. Moghimi, S. M. Mirmazloumi, S. H. A. Moghaddam, et al. 2020. “Google Earth Engine Cloud Computing Platform for Remote Sensing Big Data Applications: A Comprehensive Review.” IEEE Journal of Selected Topics in Applied Earth Observations and Remote Sensing 13:5326–5350. https://doi.org/10.1109/JSTARS.2020.3021052.
- Awty-Carroll, K., P. Bunting, A. Hardy, and G. Bell. 2019. “Using Continuous Change Detection and Classification of Landsat Data to Investigate Long-term Mangrove Dynamics in the Sundarbans Region.” Remote Sensing 11 (23): 2833. https://doi.org/10.3390/rs11232833.
- Awty-Carroll, K., P. Bunting, A. Hardy, and G. Bell. 2021. “Evaluation of the Continuous Monitoring of Land Disturbance Algorithm for Large-Scale Mangrove Classification.” Remote Sensing 13 (19): 3978. https://doi.org/10.3390/rs13193978.
- Barbier, E. B., S. D. Hacker, C. Kennedy, E. W. Koch, A. C. Stier, and B. R. Silliman. 2011. “The Value of Estuarine and Coastal Ecosystem Services.” Ecological Monographs 81 (2): 169–193. https://doi.org/10.1890/10-1510.1.
- Castagno, K. A., T. Tomiczek, C. C. Shepard, M. W. Beck, A. A. Bowden, K. O’Donnell, and S. B. Scyphers. 2021. “Resistance, Resilience, and Recovery of Salt Marshes in the Florida Panhandle following Hurricane Michael.” Scientific Reports 11 (1): 20381. https://doi.org/10.1038/s41598-021-99779-8.
- Chen, X., and H. Ishwaran. 2012. “Random Forests for Genomic Data Analysis.” Genomics 99 (6): 323–329. https://doi.org/10.1016/j.ygeno.2012.04.003.
- Chen, L., C. Ren, B. Zhang, L. Li, Z. Wang, and K. Song. 2018. “Spatiotemporal Dynamics of Coastal Wetlands and Reclamation in the Yangtze Estuary During Past 50 Years (1960s–2015).” Chinese Geographical Science 28 (3): 386–399. https://doi.org/10.1007/s11769-017-0925-3.
- Chen, G., C. Zhong, M. Li, Z. Yu, X. Liu, and M. Jia. 2022. “Disturbance of Mangrove Forests in Guangxi Beilun Estuary during 1990–2020.” Journal of Remote Sensing 26 (6): 1112–1120. https://doi.org/10.11834/jrs.20221579.
- Cheng, S., X. Zeng, Z. Wang, C. Zeng, and L. Cao. 2023. “Spatiotemporal Variations of Tidal Flat Landscape Patterns and Driving Forces in the Yangtze River Delta, China.” 9. https://doi.org/10.3389/fmars.2022.1086775.
- Crosby, S. C., D. F. Sax, M. E. Palmer, H. S. Booth, L. A. Deegan, M. D. Bertness, and H. M. Leslie. 2016. “Salt Marsh Persistence is Threatened by Predicted Sea-level Rise.” Estuarine, Coastal and Shelf Science 181:93–99. https://doi.org/10.1016/j.ecss.2016.08.018.
- Davidson, N. C., A. A. van Dam, C. M. Finlayson, and R. J. McInnes. 2019. “Worth of Wetlands: Revised Global Monetary Values of Coastal and Inland Wetland Ecosystem Services.” Marine and Freshwater Research 70 (8): 1189–1194. https://doi.org/10.1071/MF18391.
- Di Vittorio, C. A., and A. P. Georgakakos. 2018. “Land Cover Classification and Wetland Inundation Mapping Using MODIS.” Remote Sensing of Environment 204:1–17. https://doi.org/10.1016/j.rse.2017.11.001.
- Ge, Z. M., X. Zhou, T. H. Wang, K. Y. Wang, E. Pei, and X. Yuan. 2009. “Effects of Vegetative Cover Changes on the Carrying Capacity of Migratory Shorebirds in a Newly Formed Wetland, Yangtze River Estuary, China.” Zoological Studies 48 (6): 769–779.
- Hou, M., H. Liu, H. Zhang, C. Wang, and Q. Tan. 2013. “Influences of Topographic Features on the Distribution and Evolution of Landscape in the Coastal Wetland of Yancheng.” Acta Ecologica Sinica 33 (12): 3765–3773. https://doi.org/10.5846/stxb201211121591.
- Huang, Y., J. Peng, W. Sun, N. Chen, Q. Du, Y. Ning, and H. Su. 2022. “Two-branch Attention Adversarial Domain Adaptation Network for Hyperspectral Image Classification.” IEEE Transactions on Geoscience and Remote Sensing 60:1–13. https://doi.org/10.1109/TGRS.2022.3215677.
- Jia, M., Z. Wang, D. Mao, C. Ren, C. Wang, and Y. Wang. 2021. “Rapid, Robust, and Automated Mapping of Tidal Flats in China Using Time Series Sentinel-2 Images and Google Earth Engine.” Remote Sensing of Environment 255:112285. https://doi.org/10.1016/j.rse.2021.112285.
- Kennedy, R. E., Z. Yang, and W. B. Cohen. 2010. “Detecting Trends in Forest Disturbance and Recovery Using Yearly Landsat Time Series: 1. LandTrendr – Temporal Segmentation Algorithms.” Remote Sensing of Environment 114 (12): 2897–2910. https://doi.org/10.1016/j.rse.2010.07.008.
- Li, X., R. Bellerby, C. Craft, and S. E. Widney. 2018. “Coastal Wetland Loss, Consequences, and Challenges for Restoration.” Anthropocene Coasts 1 (1): 1–15. https://doi.org/10.1139/anc-2017-0001.
- Liu, Y.-F., J. Ma, X.-X. Wang, Q.-Y. Zhong, J.-M. Zong, W.-B. Wu, Q. Wang, and B. Zhao. 2020. “Joint Effect of Spartina alterniflora Invasion and Reclamation on the Spatial and Temporal Dynamics of Tidal Flats in Yangtze River Estuary.” Remote Sensing 12 (11): 1725. https://doi.org/10.3390/rs12111725.
- Liu, M., D. Mao, Z. Wang, L. Li, W. Man, M. Jia, C. Ren, and Y. Zhang. 2018. “Rapid Invasion of Spartina Alterniflora in the Coastal Zone of Mainland China: New Observations from Landsat OLI Images.” Remote Sensing 10 (12): 1933. https://doi.org/10.3390/rs10121933.
- Liu, K., W. Sun, Y. Shao, W. Liu, G. Yang, X. Meng, J. Peng, D. Mao, and K. Ren. 2022. “Mapping Coastal Wetlands Using Transformer in Transformer Deep Network on China ZY1-02D Hyperspectral Satellite Images.” IEEE Journal of Selected Topics in Applied Earth Observations and Remote Sensing 15:3891–3903. https://doi.org/10.1109/JSTARS.2022.3173349.
- Ma, Z., D. S. Melville, J. Liu, Y. Chen, H. Yang, W. Ren, Z. Zhang, T. Piersma, and B. Li. 2014. “Rethinking China's New Great Wall.” Science 346 (6212): 912–914. https://doi.org/10.1126/science.1257258.
- Ma, Z., Y. Wang, X. Gan, B. Li, Y. Cai, and J. Chen. 2009. “Waterbird Population Changes in the Wetlands at Chongming Dongtan in the Yangtze River Estuary, China.” Environmental Management 43 (6): 1187–1200. https://doi.org/10.1007/s00267-008-9247-7.
- Ma, Q., W. Wu, C. Tang, D. Niu, J. Wu, and Z. Ma. 2017. “Effects of Habitat Restoration on the Diversity of Bird and Marcobenthos in the Chongming Dongtan wetland.” Journal of Nanjing Forestry University. Natural Sciences Edition 41 (1): 9–14. https://doi.org/10.3969/j.issn.1000-2006.2017.01.002.
- McLeod, E., G. L. Chmura, S. Bouillon, R. Salm, M. Björk, C. M. Duarte, C. E. Lovelock, W. H. Schlesinger, and B. R. Silliman. 2011. “A Blueprint for Blue Carbon: Toward an Improved Understanding of the Role of Vegetated Coastal Habitats in Sequestering CO2.” Frontiers in Ecology and the Environment 9 (10): 552–560. https://doi.org/10.1890/110004.
- Ministry of Water Resources of China. 2022. China River Water and Sediment Bulletin (2020). Beijing: China Water Resources and Hydropower Press.
- Murray, N. J., T. A. Worthington, P. Bunting, S. Duce, V. Hagger, C. E. Lovelock, R. Lucas, et al. 2022. “High-resolution Mapping of Losses and Gains of Earth’s Tidal Wetlands.” Science 376 (6594): 744–749. https://doi.org/10.1126/science.abm9583.
- Newton, A., J. Icely, S. Cristina, G. M. E. Perillo, R. E. Turner, D. Ashan, S. Cragg, et al. 2020. “Anthropogenic, Direct Pressures on Coastal Wetlands.” Frontiers in Ecology and Evolution 8. https://doi.org/10.3389/fevo.2020.00144.
- Olofsson, P., G. M. Foody, M. Herold, S. V. Stehman, C. E. Woodcock, and M. A. Wulder. 2014. “Good Practices for Estimating Area and Assessing Accuracy of Land Change.” Remote Sensing of Environment 148:42–57. https://doi.org/10.1016/j.rse.2014.02.015.
- Peng, K., W. Jiang, P. Hou, Z. Wu, and T. Cui. 2024. “Detailed wetland-type classification using Landsat-8 time-series images: A pixel- and object-based algorithm with knowledge (POK).” GIScience & Remote Sensing 61 (1): 2293525. https://doi.org/10.1080/15481603.2023.2293525.
- Peng, K., W. Jiang, P. Hou, Z. Wu, Z. Ling, X. Wang, Z. Niu, and D. Mao. 2023. “Continental-scale wetland mapping: A novel algorithm for detailed wetland types classification based on time series Sentinel-1/2 images.” Ecological Indicators 148:110113. https://doi.org/10.1016/j.ecolind.2023.110113.
- Rhif, M., A. B. Abbes, B. Martinez, R. de Jong, Y. Sang, and I. R. Farah. 2022. “Detection of Trend and Seasonal Changes in Non-stationary Remote Sensing Data: Case Study of Tunisia Vegetation Dynamics.” Ecological Informatics 69:101596. https://doi.org/10.1016/j.ecoinf.2022.101596.
- Sagar, S., C. Phillips, B. Bala, D. Roberts, and L. Lymburner. 2018. “Generating Continental Scale Pixel-Based Surface Reflectance Composites in Coastal Regions with the Use of a Multi-Resolution Tidal Model.” Remote Sensing 10 (3): 480. https://doi.org/10.3390/rs10030480.
- Shanghai Statistical Bureau. 2020. Overview of Shanghai. Shanghai: Shanghai People's Publishing House.
- Shen, Y., R. Zhang, and Y. Wang. 2003. “The Tidal Creek Character in Salt Marsh of Spartina Alterni Flora Loisel on Strong Tide Coast.” Geographical Research 22 (4): 520–527.
- Shi, Y., Y. Li, Y. Meng, Z. Zhao, T. Zhang, D. Wang, and L. Yuan. 2022. “Pattern Evolution and Impact Factor of Jiuduansha Wetland at the Yangtze Estuary during 1989–2020.” Chinese Journal of Applied Ecology 33 (8): 2229–2236. https://doi.org/10.13287/j.1001-9332.202208.022.
- Song, S., Z. Wu, Y. Wang, Z. Cao, Z. He, and Y. Su. 2020. “Mapping the Rapid Decline of the Intertidal Wetlands of China Over the Past Half Century Based on Remote Sensing.” Frontiers in Earth Science 8. https://doi.org/10.3389/feart.2020.00016.
- Stehman, S. V., and D. Xing. 2022. “Confidence Intervals for Proportion of Area Estimated from a Stratified Random Sample.” Remote Sensing of Environment 280:113193. https://doi.org/10.1016/j.rse.2022.113193.
- Sun, W., K. Liu, G. Ren, W. Liu, G. Yang, X. Meng, and J. Peng. 2021. “A simple and Effective Spectral-spatial Method for Mapping Large-scale Coastal Wetlands Using China ZY1-02D Satellite Hyperspectral Images.” International Journal of Applied Earth Observation and Geoinformation 104:102572. https://doi.org/10.1016/j.jag.2021.102572.
- Sun, W., G. Yang, K. Ren, J. Peng, C. Ge, X. Meng, and Q. Du. 2021. “A Label Similarity Probability Filter for Hyperspectral Image Postclassification.” IEEE Journal of Selected Topics in Applied Earth Observations and Remote Sensing 14:6897–6905. https://doi.org/10.1109/JSTARS.2021.3094197.
- Sun, N., W. Zhu, and Q. Cheng. 2018. “GF-1 and Landsat Observed a 40-year Wetland Spatiotemporal Variation and its Coupled Environmental Factors in Yangtze River Estuary.” Estuarine, Coastal and Shelf Science 207:30–39. https://doi.org/10.1016/j.ecss.2018.03.022.
- Tian, B., W. Wu, Z. Yang, and Y. Zhou. 2016. “Drivers, Trends, and Potential Impacts of Long-term Coastal Reclamation in China from 1985 to 2010.” Estuarine, Coastal and Shelf Science 170:83–90. https://doi.org/10.1016/j.ecss.2016.01.006.
- Tian, B., Y.-X. Zhou, R. M. Thom, H. L. Diefenderfer, and Q. Yuan. 2015. “Detecting Wetland Changes in Shanghai, China Using FORMOSAT and Landsat TM Imagery.” Journal of Hydrology 529:1–10. https://doi.org/10.1016/j.jhydrol.2015.07.007.
- Verbesselt, J., R. Hyndman, A. Zeileis, and D. Culvenor. 2010. “Phenological Change Detection While Accounting for Abrupt and Gradual Trends in Satellite Image Time Series.” Remote Sensing of Environment 114 (12): 2970–2980. https://doi.org/10.1016/j.rse.2010.08.003.
- Wang, H., W. Li, and W. Xiang. 2022. “Sea Level Rise along China Coast in the Last 60 Years.” Acta Oceanologica Sinica 41 (12): 18–26. https://doi.org/10.1007/s13131-022-2066-5.
- Wang, J., Y. Sheng, C. J. Gleason, and Y. Wada. 2013. “Downstream Yangtze River Levels Impacted by Three Gorges Dam.” Environmental Research Letters 8 (4): 044012. https://doi.org/10.1088/1748-9326/8/4/044012.
- Wang, X., X. Xiao, X. Zhang, J. Wu, and B. Li. 2023. “Rapid and Large Changes in Coastal Wetland Structure in China's Four Major River Deltas.” Global Change Biology 29 (8): 2286–2300. https://doi.org/10.1111/gcb.16583.
- Wang, X., X. Xiao, Z. Zou, L. Hou, Y. Qin, J. Dong, R. B. Doughty, et al. 2020. “Mapping Coastal Wetlands of China Using Time Series Landsat Images in 2018 and Google Earth Engine.” ISPRS Journal of Photogrammetry and Remote Sensing 163:312–326. https://doi.org/10.1016/j.isprsjprs.2020.03.014.
- Wu, W., Y. Gao, C. Chen, Y. Sun, and H. Su. 2022. “A Framework for Assessing the Dynamic Coastlines Induced by Urbanization Using Remote Sensing Data: A Case Study in Fujian, China.” Remote Sensing 14 (12): 2911. https://doi.org/10.3390/rs14122911.
- Wu, L., J. Lu, C. Tong, and C. Liu. 2003. “Valuation of Wetland Ecosystem Services in the Yangtze River Estuary.” Resources and Environment in the Yangtze Basin 12 (5): 411–416.
- Wu, W., Z. Yang, C. Chen, and B. Tian. 2022. “Tracking the Environmental Impacts of Ecological Engineering on Coastal Wetlands with Numerical Modeling and Remote Sensing.” Journal of Environmental Management 302:113957. https://doi.org/10.1016/j.jenvman.2021.113957.
- Wu, W., Z. Yang, B. Tian, Y. Huang, Y. Zhou, and T. Zhang. 2018. “Impacts of Coastal Reclamation on Wetlands: Loss, Resilience, and Sustainable Management.” Estuarine, Coastal and Shelf Science 210:153–161. https://doi.org/10.1016/j.ecss.2018.06.013.
- Wu, W., C. Zhi, C. Chen, B. Tian, Z. Chen, and H. Su. 2022. “Detecting Annual Anthropogenic Encroachment on Intertidal Vegetation Using Full Landsat Time-series in Fujian, China.” GIScience & Remote Sensing 59 (1): 2266–2282. https://doi.org/10.1080/15481603.2022.2158521.
- Wulder, M. A., D. P. Roy, V. C. Radeloff, T. R. Loveland, M. C. Anderson, D. M. Johnson, S. Healey, et al. 2022. “Fifty Years of Landsat Science and Impacts.” Remote Sensing of Environment 280:113195. https://doi.org/10.1016/j.rse.2022.113195.
- Xie, S., L. Liu, X. Zhang, and J. Yang. 2022. “Mapping the Annual Dynamics of Land Cover in Beijing from 2001 to 2020 Using Landsat Dense Time Series Stack.” ISPRS Journal of Photogrammetry and Remote Sensing 185:201–218. https://doi.org/10.1016/j.isprsjprs.2022.01.014.
- Yang, S. L., M. Li, S. B. Dai, Z. Liu, J. Zhang, and P. X. Ding. 2006. “Drastic Decrease in Sediment Supply from the Yangtze River and its Challenge to Coastal Wetland Management.” Geophysical Research Letters 33 (6). https://doi.org/10.1029/2005GL025507.
- Yang, S. L., H. Li, T. Ysebaert, T. J. Bouma, W. X. Zhang, Y. Y. Wang, P. Li, M. Li, and P. X. Ding. 2008. “Spatial and Temporal Variations in Sediment Grain Size in Tidal Wetlands, Yangtze Delta: On the Role of Physical and Biotic Controls.” Estuarine, Coastal and Shelf Science 77 (4): 657–671. https://doi.org/10.1016/j.ecss.2007.10.024.
- Yang, S. L., K. H. Xu, J. D. Milliman, H. F. Yang, and C. S. Wu. 2015. “Decline of Yangtze River Water and Sediment Discharge: Impact from Natural and Anthropogenic Changes.” Scientific Reports 5 (1): 12581. https://doi.org/10.1038/srep12581.
- Yang, H. F., S. L. Yang, B. C. Li, Y. P. Wang, J. Z. Wang, Z. L. Zhang, K. H. Xu, Y. G. Huang, B. W. Shi, and W. X. Zhang. 2021. “Different Fates of the Yangtze and Mississippi Deltaic Wetlands under Similar Riverine Sediment Decline and Sea-level Rise.” Geomorphology 381:107646. https://doi.org/10.1016/j.geomorph.2021.107646.
- Yang, X., Z. Zhu, S. Qiu, K. D. Kroeger, Z. Zhu, and S. Covington. 2022. “Detection and Characterization of Coastal Tidal Wetland Change in the Northeastern US Using Landsat Time Series.” Remote Sensing of Environment 276:113047. https://doi.org/10.1016/j.rse.2022.113047.
- Yuan, L., D. Liu, B. Tian, X. Yuan, S. Bo, Q. Ma, W. Wu, Z. Zhao, L. Zhang, and J. K. Keesing. 2022. “A Solution for Restoration of Critical Wetlands and Waterbird Habitats in Coastal Deltaic Systems.” Journal of Environmental Management 302:113996. https://doi.org/10.1016/j.jenvman.2021.113996.
- Zhang, Z., N. Xu, Y. Li, and Y. Li. 2022. “Sub-continental-scale Mapping of Tidal Wetland Composition for East Asia: A Novel Algorithm Integrating Satellite Tide-level and Phenological Features.” Remote Sensing of Environment 269:112799. https://doi.org/10.1016/j.rse.2021.112799.
- Zhang, Y., W. Zhang, B. Shi, and Y. Wang. 2022. “Study on Sediment Stability between Vegetation and Bare Flats in a Muddy Intertidal Flat: A Case Study for Chongming Dongtan in the Yangtze River Estuary.” Journal of East China Normal University. Natural Science (6): 169–177. https://doi.org/10.3969/j.issn.1000-5641.2023.03.017.
- Zhi, C., W. Wu, and H. Su. 2022. “Mapping the Intertidal Wetlands of Fujian Province Based on Tidal Dynamics and Vegetational Phonology.” Journal of Remote Sensing 26 (2): 373–385. https://doi.org/10.11834/jrs.20210586.
- Zhu, Z. 2017. “Change Detection Using Landsat Time Series: A Review of Frequencies, Preprocessing, Algorithms, and Applications.” ISPRS Journal of Photogrammetry and Remote Sensing 130:370–384. https://doi.org/10.1016/j.isprsjprs.2017.06.013.
- Zhu, Z., and C. E. Woodcock. 2014. “Continuous Change Detection and Classification of Land Cover Using All Available Landsat Data.” Remote Sensing of Environment 144:152–171. https://doi.org/10.1016/j.rse.2014.01.011.
- Zhu, Z., J. Zhang, Z. Yang, A. H. Aljaddani, W. B. Cohen, S. Qiu, and C. Zhou. 2020. “Continuous Monitoring of Land Disturbance Based on Landsat Time Series.” Remote Sensing of Environment 238:111116. https://doi.org/10.1016/j.rse.2019.03.009.
- Zong, Y., J. Yang, H. Liu, Y. Li, Y. Zhang, and Y. Wu. 2023. “Study on the Impact of Spartina Alterniflora Invasion on Tidal Creek System in the Coastal Wetland of Yancheng.” Advances in Marine Science 41 (1): 109–122. https://doi.org/10.12362/j.issn.1671-6647.20210822001.
- Zou, Y.-A., C.-D. Tang, J.-Y. Niu, T.-H. Wang, Y.-H. Xie, and H. Guo. 2016. “Migratory Waterbirds Response to Coastal Habitat Changes: Conservation Implications from Long-term Detection in the Chongming Dongtan Wetlands, China.” Estuaries and Coasts 39 (1): 273–286. https://doi.org/10.1007/s12237-015-9991-x.