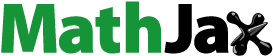
ABSTRACT
Validation of the TROPOspheric Monitoring Instrument (TROPOMI) SIF product is a necessity to evaluate its feasibility in various applications. A few validation works have been conducted through direct comparison with in-situ SIF retrievals or cross-comparison with similar satellite-based SIF or vegetation index (VI) products. Nevertheless, the influence of the geolocation mismatch between the validation pixel and reference data on validation results was never considered. This study, for the first time, quantifies the geolocation shift of the TROPOMI validation pixel based on a geometric location matching method and then the uncertainty caused by the geolocation mismatch. The results indicate that the geolocation shift of the TROPOMI pixel shows large temporal variations, with a standard deviation of 2.30 km and 1.91 km in the across- and along-track directions. The mean shifts are 0.17 km and 0.28 km in the across- and along-track directions respectively, indicating eastward shifts in the across-track direction and northward shifts in the along-track direction in general. More than half (42/54) of the time periods showed relative uncertainty larger than 5%, with the maximum even reaching 55.7%. Hence, the uncertainty caused by geolocation mismatch should be fully considered in the validation of satellite SIF products, especially over heterogeneous surfaces.
1. Introduction
Solar-induced chlorophyll fluorescence (SIF) was considered to be an ideal proxy for gross primary production (GPP), because it is directly related to vegetation photosynthesis (Frankenberg et al. Citation2011; Guanter et al. Citation2014; Gupana et al. Citation2021). The recent progress in remote sensing techniques and retrieval algorithms has enabled different kinds of satellite SIF products such as GOSAT (Joiner et al. Citation2011), OCO-2(Frankenberg et al. Citation2014), TanSat (Du et al. Citation2018), and TROPOMI (Guanter et al. Citation2021) SIF products, which provide an unprecedented opportunity for large-scale monitoring of SIF and diverse applications in climate, biogeochemical cycle, and earth system science (Porcar-Castell et al. Citation2021). Nevertheless, due to the errors in processing raw data to radiance units and the limitations of the SIF retrieval algorithm, satellite SIF products inevitably suffer from errors. Hence, it is a necessity to validate the accuracy of satellite SIF products to inform end-users of their feasibility in various applications (Wu et al. Citation2019).
In previous years, the accuracy of satellite SIF products was generally evaluated indirectly through the cross-comparison with similar satellite-based SIF or VI or eddy covariance (EC) GPP products (Du et al. Citation2021; Frankenberg et al. Citation2011; Joiner et al. Citation2016; Sun et al. Citation2018). However, this kind of validation can merely identify the consistency or disagreement between these products, which cannot reveal the true accuracy of satellite SIF products. Direct validation based on in-situ based SIF measurements is still a necessity to fully understand the performance of satellite SIF products. It has been well acknowledged that the direct validation of satellite SIF products based on in-situ SIF measurements is challenged by the uncertainties related to the in-situ measuring system, the spatial scale mismatch between in-situ and satellite-based SIF measurements, spectral difference, and retrieval algorithms. By addressing these challenges, Du et al. (Citation2023) conducted a direct validation of the TROPOMI SIF product based on a sufficient in-situ SIF database. However, the influence of the geolocation mismatch between the validation pixel and reference data on validation results has not been recognized.
Within the direct validation framework, the validation pixel generally refers to the satellite pixel containing in-situ measurements. The reference data for validation is generally obtained within the nominal spatial extent of the validation pixel from in-situ observations through either upscaling or spatial representativeness assessment (Cescatti et al. Citation2012; Wu et al. Citation2016). Nevertheless, the validation pixel may suffer from geometric errors and deviate from its nominal location. Then the signal of the validation pixel may come from a different area instead of its nominal location, resulting in inherent incompatibilities (Wu et al. Citation2021). The geolocation mismatch between the validation pixel and reference data will introduce large uncertainty to the validation results, especially for validation pixels covering heterogeneous areas. This kind of uncertainty obscures the true accuracy of satellite SIF products. Hence, it is necessary to quantify the uncertainty caused by the geolocation mismatch between the validation pixel and reference data.
This study makes the first attempt to quantitatively identify the geolocation shift of satellite SIF products and quantify the magnitude of uncertainty caused by geolocation mismatch between validation pixel and reference data. Among the various kinds of satellite-baesd SIF products, the TROPOMI SIF product has been identified as the one with the highest potential due to its relatively high spatial resolution (3.5 km × 7.5/5.5 km at the nadir) and wide swath (∼2600 km) (Guanter et al. Citation2021). Hence, it was selected to conduct the analysis. The findings in this study are expected to improve the strictness and reliability of validation results of satellite SIF products.
2. Methods
The purpose of this study is to quantify the uncertainty caused by the geolocation errors of the validation pixel. To achieve this goal, the geolocation shift of the validation pixel should be first identified. It is generally well known that satellite products have been geometrically corrected with errors less than 0.5 pixels (Wu et al. Citation2021). However, the geometric correction was generally carried out on the satellite image level instead of pixel level. When it comes to a specific pixel, we do not know its exact geolocation error and how it shifts. In response to this challenge, a pixel-based geometric location matching method (Tang et al. Citation2023) was introduced here to identify the geolocation shift of the TROPOMI SIF validation pixel. The basic idea of this method is to detect the shift in the along-track and across-track directions on the basis of a geo-corrected high-resolution map. The flowchart of the methodology of this study is shown in . The key steps to quantify the uncertainty caused by geolocation mismatch include: preprocessing of a high-resolution map, identification of geolocation shift of TROPOMI SIF validation pixel, and quantification of uncertainty caused by geolocation mismatch.
Figure 1. Workflow of quantifying the uncertainty caused by the geolocation errors of the TROPOMI pixel.

2.1. The preprocessing of a high-resolution map
The geo-corrected high-resolution map was extracted from level-2 Sentinel-2 Surface Reflectance (SR) data due to its high spatial resolution. Considering the surface reflectance anisotropy, the Sentinel-2 SR should be first normalized to the geometry of the TROPOMI SIF validation pixel. Here, we employed the MODIS MCD43A1 product to calculate the directional reflectance ratio (), which will be used to adjust Sentinel-2 SR (
) from original geometry (
) to the geometry of TROPOMI validation pixel (
) (Equations (1–3)) (Roy et al. Citation2016, 2017).
(1)
(1)
(2)
(2)
(3)
(3) where
is Sentinel-2 adjusted SR,
was estimated using the kernel coefficients (
,
,
) provided by MCD43A1.
and
are volumetric scattering and geometric-optical kernels (Roujean, Leroy, and Deschamps Citation1992; Schaaf et al. Citation2002), respectively.
Considering the inconsistency in spectral bands between TROPOMI and Sentinel-2, spectral conversion from Sentinel-2 SR (https://sentinels.copernicus.eu/web/sentinel/user-guides/sentinel-2-msi/document-library/-/asset_publisher/Wk0TKajiISaR/content/sentinel-2a-spectral-responses) to TROPOMI (https://sentinels.copernicus.eu/web/sentinel/technical-guides/sentinel-5p/products-algorithms/tropomi-response-functions) was conducted utilizing the ground spectral data and the spectral response function of the two sensors. The detailed information on the transformation process can be found in Lin et al. (Citation2022). Near-infrared reflectance of vegetation (NIRv) (NIRv = NDVI × ρNIR, where NDVI is the normalized difference VI based on NIR and red spectral bands and ρNIR is the NIR reflectance) has been identified as the prominent indicator of SIF (Turner et al. Citation2020; Zeng et al. Citation2019). Hence, we generated the high-resolution NIRv maps based on angular-corrected and band consistent Sentinel-2 SR data.
2.2. Geolocation shift identification
The geolocation shift of the TROPOMI SIF validation pixel was identified based on the Sentinel-2 NIRv data and the geolocation matching method. During the geolocation matching process, the nominal spatial extent of the validation pixels was extracted as the initial template. Then the template was shifted in the along- and across-track directions with a step of 10 m (i.e. the pixel size of the NIRv map). The maximum shift was set to 0.5 TROPOMI pixel, resulting in [(0.5 TROPOMI pixel /10) × 2 + 1]2 shift cases for each validation pixel. For each simulated shift case, the NIRv values on the TROPOMI pixel scale (denoted as ) was estimated using the aggregated 10 m Sentinel-2 SR within the template. Afterward, the difference between the simulated TROPOMI pixel scale NIRv (
) and the TROPOMI validation pixel NIRv (denoted as
) was calculated. The shift and the exact location (denoted as
) of the validation pixel correspond to the simulate case with the minimum absolute difference between
and
(EquationEquation (4
(4)
(4) )).
(4)
(4) where
denotes the minimal function, and abs represents the absolute difference function.
2.3. Quantification of uncertainty caused by geolocation mismatch
To quantify the uncertainty caused by the geolocation mismatch in validation, the TROPOMI pixel scale reference SIF in the nominal location (denoted as ) and exact location (denoted as
) should be calculated. Here, the TROPOMI pixel scale reference SIF was determined based on tower-based SIF measurements (denoted as
) and an upscaling function. The basic idea of the upscaling function is that the ratio of the NIRv with different spatial scales can be regarded as the upscaling coefficients of SIF for the two spatial scales (EquationEquation (5
(5)
(5) )) (Du et al. Citation2023).
(5)
(5)
(6)
(6) where
and
represented the Sentinel-2 NIRv on the TROPOMI pixel scale at the nominal and exact locations of validation pixel, which was calculated by aggregating Sentinel-2 SR within the footprint of TROPOMI pixel as recommended by Du et al. (Citation2023).
is the Sentinel-2 pixel NIRv containing the tower-based location.
The uncertainty caused by the geolocation mismatch (denoted as ) between validation pixel and reference data can be calculated using EquationEquation (7
(7)
(7) ).
(7)
(7)
3. Study area and materials
3.1. Tower-based measurements
The tower-based SIF validation datasets were collected from seven ChinaSpec (http://chinaspec.nju.edu.cn) network sites (i.e. XTS, DM, GC, HL, AR, PYH and YX) (Zhang et al. Citation2021). The main emphasis of ChinaSpec is to gather ground-based SIF measurements from the flux sites that are part of the ChinaFLUX network (http://www.chinaflux.org/) (Yu et al. Citation2006). The locations of these seven sites are graphically illustrated in .
Figure 2. The locations and land-cover types of these seven sites were used in this study. The base land-cover map was extracted from the 30m annual land-cover dataset (i.e. CLCD) of 2020 developed by Wuhan University (https://zenodo.org/record/8176941). The subplots indicate the surrounding land-cover types of each in-situ site (indicated by red flag symbols) with a range of 0.05°.

The seven ChinaSpec sites encompass three cropland sites, two grassland sites, and two wetland sites which are spread out throughout China. The main vegetation types of these cropland sites are different. At XTS and GC sites, a rotation system of maize and wheat crops was implemented. However, at the DM site, only maize was cultivated during the summer season each year. The HL site featured a combination of grass and several crabapple trees (Du et al. Citation2023). Except for the YX site, all sites were equipped with the SIFSpec instrument consisting of the QE 65Pro spectrometer. The operational wavelength range of the instrument is approximately 650 nm to 840 nm, with a spectral resolution of around 0.31 nm. At the YX site, the SIFSpec instrument installed is the QE-PRO (Zhu et al. Citation2021), which is an improved version of the QE65Pro. The QE-PRO features a high saturation threshold, with digital numbers (DN) reaching up to 200,000. Both types of spectrometers have similar spectral resolution and signal-to-noise ratio (SNR), making their SIF measurements comparable.
In order to obtain tower-based SIF, a series of processes have been carried out, including raw data preprocessing, quality control, atmospheric correction, and SIF retrieval. Detailed information on the processing can be referred to Du et al. (Citation2019) and Zhu et al. (Citation2021). The in-situ tower-based SIF observations over these sites are free to access at https://zenodo.org/record/7244183 and https://zenodo.org/records/454363, respectively. In order to minimize the uncertainties caused by temporal inconsistencies in SIF retrievals, the local time of the TROPOMI overpass was used to extract in-situ measurements. Furthermore, to reduce the effect of random errors associated with tower-based measurements, the in-situ observations within a 30-minute window of TROPOMI overpasses were employed, which were averaged to generate half-hourly SIF values to be matched with TROPOMI instantaneous SIF measurements (Du et al. Citation2023).
It is noteworthy that there are wavelength differences between TROPOMI SIF products (∼740 nm) and tower-based SIF retrievals (∼760 nm). To address this issue, a ratio of 1.48 was used to convert tower-based SIF760 to SIF740 as recommended by Du et al. (Citation2023). Considering the temporal variability of the geolocation error in TROPOMI SIF products, the experiments at XTS, DM, GC, HL, and AR sites spanned the entire year of 2020. However, the experiment at the YX site was conducted in 2018. The PYH site encompasses data from both 2020 and 2021. The data matching among tower-based measurements, Sentinel-2, MODIS MCD43A1, and TROPOMI SIF products was conducted rigorously, with full consideration of the data quality of all three data sources. As a result, a total of 64 data pairs were retained.
3.2. Satellite data
3.2.1. TROPOMI SIF products
TROPOMI is a step forward of SIF measurements on the global scale due to its high spatial resolution of 3.5 km × 7.5 km at the nadir (adjusted to 3.5 km × 5.5 km since August 2019) and high temporal resolution of nearly daily. This improved spatial and temporal resolution significantly increases the number of clear-sky measurements per day compared to previous missions. The TROPOMI SIF product employed in this study was established by Guanter et al. (Citation2021) (http://ftp.sron.nl/open-access-data-2/TROPOMI/tropomi/sif/v2.1/l2b/), which includes two far-red SIF datasets derived from the 743–758 nm window and the extended 735–758 nm window. The former was the baseline SIF product for the moment and recommended for use. Hence, only the 743–758 nm SIF product was adopted in this paper. This product was retrieved from TROPOMI TOA radiance in the far-red spectral region based on a data-driven forward model (Guanter et al. Citation2012). In addition to SIF data, this product also provides top-of-atmosphere (TOA) reflectance for the bands ranging from red-edge to NIR wavelengths, enabling the calculation of NIRv, which is considered to be a good proxy of SIF and approximately equal to the multiplication of NIR reflectance and NDVI. To avoid the uncertainties caused by large view zenith angle (SZA), the TROPOMI SIF records with SZA greater than 60° were excluded from the analysis.
3.2.2. Sentinel-2 SR products
The Level-2A Sentinel-2 SR products were obtained from the Google Earth Engine (GEE) platform (https://developers.google.com/earthengine/datasets/catalog/COPERNICUS_S2_S). The surface reflectance data along with the associated cloud probability product and default cloud masking parameter (https://developers.google.com/earthengine/tutorials/community/sentinel-2-s2cloudle) were extracted. The cloud masking parameter utilizes a machine-learning-based Sentinel-2 cloud detection algorithm. However, for the selected period of the YX site in 2018, Level-2A Sentinel-2 SR products covering this site were not available. Therefore, the Level-1C Sentinel-2 SR products obtained from the Copernicus Data Space Ecosystem (https://dataspace.copernicus.eu/) were used instead at the YX site. These Level-1C products were preprocessed using the Sen2Cor plugin (https://step.esa.int/main/snap-supported-plugins/sen2cor/) provided by the European Space Agency (ESA), resulting in the generation of Level-2A Sentinel-2 SR products. The Sentinel-2 offers multispectral optical images with 13 bands ranging from visible to shortwave infrared. Considering the inconsistency of viewing geometry between Sentinel-2 and TROPOMI, the viewing geometry for three visible bands (blue, green, and red), NIR, and two short wave infrared (SWIR) spectral bands were also extracted to carry out the angular normalization.
The spatial resolutions of the three visible bands (blue, green, and red), and the NIR band are 10 m, while that of the SWIR band is 20 m. To maintain consistency with other bands, the two SWIR bands were resampled to a spatial resolution of 10 m. Since the Sentinel-2 data have a repeat period of 10 days, the bottom-of-atmosphere reflectance from both Sentinel-2A and 2B were used to calculate high-resolution NIRv maps in order to get more synchronized observations. Meanwhile, to ensure the reliability of the analysis, a filtering process was conducted for the Sentinel-2 observations. The data records with a cloud, snow, or ice coverage exceeding 30% were discarded.
3.2.3. Land cover data
In this study, a land cover dataset, namely the 30m annual China Land Cover Dataset (CLCD), was used to explore the relation between the spatial heterogeneity around the validation pixel and the uncertainty caused by the geolocation errors of the validation pixel. This dataset was selected partly because of its high spatial resolution and partly because of its availability in China. It was generated from Landsat data on the GEE platform with the random forest (RF) classifier based on the training samples which were collected by combing stable samples provided by China’s land-use/cover datasets and visually interpreted samples from satellite time series data, Google Earth, and Google Maps. Two post-processing procedures including spatial–temporal filtering and logical reasoning were carried out to further improve the spatial–temporal consistency of CLCD. The overall accuracy of CLCD is reasonable, with a value of 79.31% (Yang and Huang Citation2021).
3.2.4. Spectral data samples
The spectral data samples consist of 815 samples from the Ground Object Background Spectral Library and Mapping (GOSPEL) (Zhong et al. Citation2020), 119 samples extracted from the book ‘Quantitative Remote Sensing for Land Surfaces’, 221 samples obtained from the Spectral Library of Typical Objects in China, 47 samples derived from the snow spectral datasets in Greenland (Qu et al. Citation2014), and 1192 samples acquired from the USGS Digital Spectral Library. These spectral data, combined with the spectral response functions of TROPOMI and Sentinel-2, were utilized to calculate the inter-satellite band conversion coefficients through linear regression. Finally, the inter-satellite band conversion coefficients were employed to convert Sentinel-2 surface reflectance to TROPOMI band-consistent surface reflectance.
4. Results and discussion
4.1. The variation of NIRv for all simulated shift cases
shows the spatial distribution of the throughout the shifted cases within the ±1 TROPOMI pixels range at a step change of 10 m. For the sake of brevity, only the results for the XTS site on November 10th, 2020 ((a)) and December 8th, 2020 ((b)) are displayed here.
Figure 3. Variation of for each shift combination (a–b) and their histogram distribution (c–d). The Y-axis and X-axis represent the along-track and across-track directions, respectively. +Y and + X indicate the shift to the north and east, respectively. The exact shift of the validation pixel is determined by the minimum absolute difference between
and
. The geolocation error can be converted to distance in meters by multiplying the grid location by 10 m.

Each grid in (a–b) represents a shifted case, which is represented by the distance from the center in the two directions. The center of each subfigure denotes the nominal location of the validation pixel on the reference scene. It can be seen that show differences among the different simulated cases. The NIRv corresponding to the exact location of the validation pixel may be either larger or smaller than the NIRv at its nominal location. The maximum and minimum show a difference of about 0.008 for the two dates ((c–d)). It is important to note that the magnitude of NIRv itself is small, with values less than 0.05. Hence, the influence of geometric mismatch cannot be ignored since it may obscure the true accuracy of TROPOMI SIF products. It is important to note that the spatial range of the TROPOMI pixel is irregular, and the location of one TROPOMI pixel changes with time. Hence, the number of simulated cases for each period is not equal ((c–d)).
4.2. The shifts of the validation pixels of TROPOMI
and show the geometric shift of the TROPOMI validation pixel in the across-track (X) and along-track (Y) directions, respectively. As shown in (a) and (a), the geolocation shift of the validation pixel is widely present over these seven sites throughout the experimental period in both across-track and along-track directions.
Figure 4. The geometric shift of the validation pixels in the across-track directions. (a) display the histogram distribution of the across-track directions shifts of the validation pixel during the experimental period. (b–h) present the day-by-day shift of the validation pixel at each site in the across-track directions.

Figure 5. The geometric shift of the validation pixels in the along-track directions. (a) display the histogram distribution of the along-track directions shifts of the validation pixel during the experimental period. (b–h) present the day-by-day shift of the validation pixel at each site in the along-track directions.

The shift in the across-track direction ranges from −3.92 km to 6.48 km, with a mean value of 0.17 km, indicating eastward shifts in the across-track direction in general. While in the along-track direction, the shift of the validation pixel shows directions are comparable, indicated by the similar mean and a range from −3.07 km to 2.80 km, with a mean value of 0.28 km, indicating a northward shift in the along-track direction in general. It is noteworthy that the temporal variations of shifts in the across- and along-track directions are both significant, with a standard deviation of 2.30 km and 1.91 km, respectively. The magnitude of the shift of the TROPOMI validation pixel is generally reasonable, with a mean value of 1.90 km and 1.68 km in two directions ((a) and (a)). Moreover, both the magnitude and the temporal variation degree of the shifts in two directions are comparable, indicated by the similar mean and standard deviation values.
(b–f) and (b–f) display the day-by-day variation of geometric shifts of the validation pixels at these seven sites in both the cross-track and along-track directions, respectively. It is obvious that the geometric shift of the validation pixels corresponding to the seven in-situ sites all show significant temporal variations. However, the magnitude of geolocation shift and its variation degree show a certain dependence on in-situ sites ( and ). In the cross-track direction (), the XTS site shows the largest range of geolocation shift, with a minimum of −3.92 km and a maximum of 6.48 km. Its mean geolocation error is 0.59 km, indicating an eastward shift on average. Similarly, the YX site also shows an eastward shift, but with the smallest geolocation shift deviation ranging from −0.12 km to 1.03 km. The mean geolocation error for YX site is 0.3 km. Likewise, both the PYH and AR sites exhibit an eastward shift, with average geolocation errors of 1.07 km and 1.57 km, respectively. Nevertheless, it is important to note that the number of data pairs is so small at AR site that the results may be not statistically significant. Contrary to the eastward shift observed in the aforementioned sites, the opposite phenomenon occurs at GC, DM, and HL sites, which in general show a westward shift, indicated by the mean shifts of −0.4 km, −0.49 km, and −0.25 km, respectively. Among these three in-situ sites, DM site shows the largest variation range as well as variation degree (i.e. 2.43 km). By contrast, GC site present the smallest variation range as well as variation degree (i.e. 2.14 km).
When it comes to along-track direction (), XTS, GC, AR, and PYH sites generally show a northward shift, indicated by the mean shift of 0.49 km, 0.29 km, 1.69 km, and 0.87km, respectively. The temporal variation range and variation degree of geolocation shift were the largest at the XTS site, with values ranging from −2.81 km to 2.80 km and a standard deviation of 2.04 km. This is followed by GC site with comparable variation ranges and variation degrees, indicated by the standard deviations of 2.16 km. The standard deviations of geolocation shift for PYH site and AR site are 1.33 km and 0.92 km, respectively. It is evident that AR site exhibits the smallest standard deviation, which may be partially attributed to the relatively low quantity of data available for this site. Compared to the northward shift of these four sites, a different phenomenon can be observed at the DM, HL, and YX sites, which show a southward shift in the along-track direction, indicated by the mean shift of −0.19 km, −0.25 km, and −0.02km, respectively. The temporal variation degree of geolocation shift is also significant at both sites, with a standard deviation of 1.85 km, 2.58km, and 2.05km, respectively.
To show the geolocation shift of the TROPOMI validation pixel corresponding to in-situ site more intuitively, we present the nominal location and the exact location of validation pixels in . To show the temporal variation characteristics of TROPOMI validation pixel geolocation shift at a specific area, the results at the XTS site on October 16, October 19, and October 21, 2020 were shown ((a–c)). To display the geolocation shift of validation pixels at different locations on the same day, the results at XTS, HL, and GC sites on October 4, 2020, were presented ((d–f)).
Figure 6. The nominal (black square) and exact (red square) location of the validation pixel corresponds to the in-situ site. (a), (b) and (c) represent the nominal and exact locations of the validation pixels at the XTS sites on October 16, 2020, October 19, 2020, and October 21, 2020, respectively. (c), (d) and (f) represent the nominal and exact locations of the validation pixels at the XTS, HL, and GC sites on October 4, 2020, respectively.

From , it can be seen that the nominal spatial extent of the TROPOMI validation pixel and its exact spatial extent are always not totally overlapped. At the XTS site, the TROPOMI validation pixel exhibits different degrees of geolocation shift in the southeast, northeast, and southwest directions at three different but adjacent time points. This indicates that even for the same site, the magnitude and direction of geolocation shift are inconsistent and even opposite within adjacent periods. As shown in (d–f), it can be found that the validation pixels at XTS, HL, and GC sites on October 4, 2020, exhibit different degrees of shifts in the northwest, southwest, and southeast directions, respectively. This indicates that even at the same time, the shift of TROPOMI validation pixels over different areas is not consistent and even the opposite.
Based on these results, it can be concluded that the geolocation shifts of TROPOMI vary irregularly with time and space. It is hard to correct such a kind of geolocation error through general methods such as the coastline crossing method, the land-sea fraction method, and the coregistration method, because the correction models were generally established on the image level. Instead, the geolocation shift of TROPOMI data should be identified pixel by pixel. It is important to point out that in general validation work, the reference value for validation is generally obtained within the nominal spatial extent of the validation pixel (i.e. black square), but the signal of the satellite validation pixel corresponds to the exact spatial extent (i.e. red square). This geolocation mismatch may artificially expand the difference between the reference value and satellite validation pixel. Therefore, the geolocation shift of TROPOMI SIF pixels should be taken seriously when validating TROPOMI SIF products.
4.3. The uncertainty caused by geometric errors of validation pixel
To illustrate the effect of geolocation mismatch on the validation of TROPOMI SIF products, (a–f) shows the and
for XTS, DM, and GC sites at each time point. It can be seen that
and
show similar temporal variation characteristics throughout the experimental period. Hence, the geolocation shift of the validation pixel is not a main influencing factor on the temporal change trend of SIF. However, it is obvious that the magnitude of
is not always equal to
in the time series. Therefore, the effect of the geolocation shift of the validation pixel cannot be ignored when the magnitude of SIF is focused. Because it will lead to different interpretations of TROPOMI SIF product errors.
Figure 7. The value of TROPOMI pixel scale reference SIF at the nominal ()(up) and exact (
) (down) locations, respectively. The first to third columns present the results for XTS, DM, and GC sites, respectively.

To show the influence of the geolocation shift of validation pixel on reference value more intuitively, we present the distribution of uncertainty and the relative uncertainty of the TROPOMI pixel scale SIF reference caused by geolocation shift in and , respectively. It is worth noting that due to the absence of TROPOMI pixels covering the PYH site, the result was not shown for this site. Regarding the YX site, the time period of in-situ data at this site was different from the other in-situ sites. Moreover, the data record frequency is too low to be precisely synchronized with TROPOMI observations. Hence, for the purpose of consistent comparison, only the uncertainty results from the remaining five sites were presented here. (a) shows the frequency histogram of the uncertainty of pixel scale reference values by combining all the results of these five sites throughout the experimental period. (b–f) shows the day-by-day variation of the uncertainty of pixel scale reference for each site.
Figure 8. The uncertainty is caused by the geolocation shift of the TROPOMI validation pixel. (a) display the histogram distribution of the uncertainty () caused by the geolocation shift of the validation pixels at these five sites throughout the experimental period. (b–f) present the day-by-day variation of the errors of reference values caused by the geolocation shift of the validation pixels for each site.

Figure 9. The relative uncertainty (%) caused by the geolocation shift of the TROPOMI validation pixel. (a) display the histogram distribution of relative uncertainty (%) caused by the geolocation shift of validation pixels at these five sites throughout the experimental period (b–f) present the day-by-day variation of the relative uncertainty (%) caused by the geolocation shift of validation pixels for each site.

From (a), it can be seen that the uncertainty caused by the geolocation shift of the TROPOMI validation pixel presents significant spatial and temporal variations, with values ranging from −0.38mWm-2sr-1nm-1 to 0.09mWm-2sr-1nm-1. Furthermore, negative values occur more frequently and have higher magnitudes compared to positive values, resulting in a mean uncertainty of −0.03mWm-2sr-1nm-1 overall. When it comes to each specific site ((b–f)), it can be seen that all these sites show significant temporal variations regarding the uncertainty of pixel scale reference. This is partly due to the fact that the geolocation shift varies with time, and partly due to the temporal variation of spatial heterogeneity around the validation pixel. For instance, if the land surface around the validation pixel is absolutely homogeneous, then the geolocation mismatch would not cause errors regardless of the shift magnitude of the validation pixel. Nevertheless, it is noteworthy that the distribution characteristics of the uncertainty of pixel scale reference with time are not consistent between these sites. The uncertainties at GC, DM, HL, and AR sites are basically negative. By contrast, positive and negative values occur with almost equal frequency at XTS site.
Considering that the magnitude of SIF values may have an influence on the uncertainty, we also calculated the relative uncertainty caused by the geolocation shift of the validation pixel (). Here, the absolute value was shown because only the magnitude of uncertainty was focused. It can be seen that most of the relative uncertainties are lower than 30%. But more than half (i.e. 42/54) of the time periods showed relative uncertainty larger than 5%. It is noteworthy that the maximum uncertainty caused by geolocation shift can be even larger than 50% ((a)).
The magnitude of the relative uncertainty caused by geolocation errors shows different temporal variation characteristics among these five sites. For instance, at the XTS site ((b)), the relative uncertainty is generally small in the growing season, with the values distributed around 5%, but becomes larger in the dormant season, with the values consistently larger than 10%. By contrast, at the DM site, the relative uncertainty is large in the growing season occasionally. In particular, the relative uncertainty in the growing season is even larger than that in the dormant season ((d)). It is noteworthy that although the relative uncertainty at HL site is generally distributed around 10%, there is a significantly large value of 55.7%. Hence, it is difficult to give a common conclusion about the magnitude of relative uncertainty caused by geolocation shifts. Instead, such a kind of uncertainty should be quantified pixel-by-pixel for each time period in the validation of satellite SIF products, especially over heterogeneous surfaces.
5. Conclusion
The validation of TROPOMI SIF is an essential process to judge whether its accuracy is adequate for the intended use. Although some validation studies have been conducted through direct comparison with in-situ SIF retrievals or cross-comparison with similar satellite-based SIF or VI products, the impact of geolocation mismatch between validation pixel and reference data on validation results has not been taken into account. In fact, the geolocation mismatch is a big contributor to the uncertainty of validation results. This is particularly true over heterogeneous surfaces where the objects at the nominal and exact locations may differ. Hence, it is necessary to quantify such a kind of uncertainty in order to determine whether the direct comparison between TROPOMI SIF and reference SIF is feasible and whether the validation results are rigorous and reliable.
This study, for the first time, quantifies the geolocation shift of the TROPOMI validation pixel and then the uncertainty caused by the geolocation mismatch between the TROPOMI SIF and reference SIF. It was found that the shift ranges from −3.92 km to 6.48 km and from −3.07 km to −2.80 km in the across- and along-track directions, respectively. The mean shifts are 0.17 km and 0.28 km in the across- and along-track directions respectively, indicating eastward shifts in the across-track direction in general and northward shifts in the along-track direction. The shift shows significant temporal variations with a standard deviation of 2.30 km and 1.91 km in the across- and along-track directions. In particular, the shifts are even opposite between adjacent times in two directions. When the magnitude of the shift is focused, the geolocation shift of the TROPOMI validation pixel are 1.90 km and 1.68 km in the across-track and along-track directions, respectively. However, it is important to note that the method in this study cannot be applied to homogeneous surfaces such as water and desert. Because the simulated values are almost the same in any simulated shift cases. Given that the in-situ sites are featured by different degrees of spatial heterogeneity as shown in , the shortcoming of this method is not a problem in this study.
Regarding the influence of the geolocation shift of the validation pixel, it is not a main influencing factor on the temporal change trend of SIF, but it cannot be ignored when the magnitude of SIF is focused. More than half (42/54) of the time periods showed relative uncertainty larger than 5%, with the maximum even reaching 55.7%. Hence, the uncertainty caused by the geolocation shift of the validation pixel cannot be ignored, because it will lead to different interpretations of TROPOMI SIF product errors. However, it is important to note that this study is only limited to validation pixels from a specific period at five in-situ sites. Hence, whether the findings of this study can be applied to other regions is still an open question. More analyses will be made when more data are available in the future. But anyway, this study is the first step towards a more rigorous and reliable validation of the TROPOMI SIF products.
Acknowledgments
We gratefully acknowledge the anonymous reviewers and editor of the paper for providing critical and constructive comments, which have helped us to significantly improve the manuscript. We also appreciate the assistance of the all authors of this paper.
Disclosure statement
No potential conflict of interest was reported by the author(s).
Data availability statement
The data that support the findings of this study are available from the website given in the manuscript.
Additional information
Funding
References
- Cescatti, A., B. Marcolla, S. K. Santhana Vannan, J. Y. Pan, M. O. Román, X. Yang, P. Ciais, et al. 2012. “Intercomparison of MODIS Albedo Retrievals and in Situ Measurements Across the Global Fluxnet Network.” Remote Sensing of Environment 121: 323–334. https://doi.org/10.1016/j.rse.2012.02.019.
- Du, S., X. Liu, J. Chen, W. Duan, and L. Liu. 2023. “Addressing Validation Challenges for TROPOMI Solar-Induced Chlorophyll Fluorescence Products Using Tower-Based Measurements and an NIRv-Scaled Approach.” Remote Sensing of Environment 290: 113547. https://doi.org/10.1016/j.rse.2023.113547.
- Du, S., L. Liu, X. Liu, and J. Chen. 2021. “First Investigation of the Relationship Between Solar-Induced Chlorophyll Fluorescence Observed by TanSat and Gross Primary Productivity.” IEEE Journal of Selected Topics in Applied Earth Observations and Remote Sensing 14: 11892–11902. https://doi.org/10.1109/JSTARS.2021.3128355.
- Du, S., L. Liu, X. Liu, J. Guo, J. Hu, S. Wang, and Y. Zhang. 2019. “SIFSpec: Measuring Solar-Induced Chlorophyll Fluorescence Observations for Remote Sensing of Photosynthesis.” Sensors 19 (13): 3009. https://doi.org/10.3390/s19133009.
- Du, S., L. Liu, X. Liu, X. Zhang, X. Zhang, Y. Bi, and L. Zhang. 2018. “Retrieval of Global Terrestrial Solar-Induced Chlorophyll Fluorescence from TanSat Satellite.” Science Bulletin 63 (22): 1502–1512. https://doi.org/10.1016/j.scib.2018.10.003.
- Frankenberg, C., J. B. Fisher, J. Worden, G. Badgley, S. S. Saatchi, J.-E. Lee, G. C. Toon, et al. 2011. “New Global Observations of the Terrestrial Carbon Cycle from GOSAT: Patterns of Plant Fluorescence with Gross Primary Productivity.” Geophysical Research Letters 38 (17): L17706. https://doi.org/10.1029/2011GL048738.
- Frankenberg, C., C. O’Dell, J. Berry, L. Guanter, J. Joiner, P. Köhler, R. Pollock, and T. E. Taylor. 2014. “Prospects for Chlorophyll Fluorescence Remote Sensing from the Orbiting Carbon Observatory-2.” Remote Sensing of Environment 147: 1–12. https://doi.org/10.1016/j.rse.2014.02.007.
- Guanter, L., C. Bacour, A. Schneider, I. Aben, T. A. van Kempen, F. Maignan, C. Retscher, et al. 2021. “The TROPOSIF Global sun-Induced Fluorescence Dataset from the Sentinel-5P TROPOMI Mission.” Earth System Science Data 13 (11): 5423–5440. https://doi.org/10.5194/essd-13-5423-2021.
- Guanter, L., C. Frankenberg, A. Dudhia, P. E. Lewis, J. Gómez-Dans, A. Kuze, H. Suto, and R. G. Grainger. 2012. “Retrieval and Global Assessment of Terrestrial Chlorophyll Fluorescence from GOSAT Space Measurements.” Remote Sensing of Environment 121: 236–251. https://doi.org/10.1016/j.rse.2012.02.006.
- Guanter, L., Y. Zhang, M. Jung, J. Joiner, M. Voigt, J. A. Berry, C. Frankenberg, et al. 2014. “Global and Time-Resolved Monitoring of Crop Photosynthesis with Chlorophyll Fluorescence.” Proceedings of the National Academy of Sciences 111 (14): E1327–E1333. https://doi.org/10.1073/pnas.1320008111.
- Gupana, R. S., D. Odermatt, I. Cesana, C. Giardino, L. Nedbal, and A. Damm. 2021. “Remote Sensing of sun-Induced Chlorophyll-a Fluorescence in Inland and Coastal Waters: Current State and Future Prospects.” Remote Sensing of Environment 262: 112482. https://doi.org/10.1016/j.rse.2021.112482.
- Joiner, J., Y. Yoshida, L. Guanter, and E. M. Middleton. 2016. “New Methods for the Retrieval of Chlorophyll red Fluorescence from Hyperspectral Satellite Instruments: Simulations Andapplication to GOME-2 and SCIAMACHY.” Atmospheric Measurement Techniques 9 (8): 3939–3967. https://doi.org/10.5194/amt-9-3939-2016.
- Joiner, J., Y. Yoshida, A. P. Vasilkov, Y. Yoshida, L. A. Corp, and E. M. Middleton. 2011. “First Observations of Global and Seasonal Terrestrial Chlorophyll Fluorescence from Space.” Biogeosciences (online) 8 (3): 637–651. https://doi.org/10.5194/bg-8-637-2011.
- Lin, X., S. Wu, B. Chen, Z. Lin, Z. Yan, X. Chen, G. Yin, et al. 2022. “Estimating 10-m Land Surface Albedo from Sentinel-2 Satellite Observations Using a Direct Estimation Approach with Google Earth Engine.” ISPRS Journal of Photogrammetry and Remote Sensing 194: 1–20. https://doi.org/10.1016/j.isprsjprs.2022.09.016.
- Porcar-Castell, A., Z. Malenovský, T. Magney, S. Van Wittenberghe, B. Fernández-Marín, F. Maignan, Y. Zhang, et al. 2021. “Chlorophyll a Fluorescence Illuminates a Path Connecting Plant Molecular Biology to Earth-System Science.” Nature Plants 7 (8): 998–1009. https://doi.org/10.1038/s41477-021-00980-4.
- Qu, Y., Q. Liu, S. Liang, L. Wang, N. Liu, and S. Liu. 2014. “Direct-Estimation Algorithm for Mapping Daily Land-Surface Broadband Albedo from MODIS Data.” IEEE Transactions on Geoscience and Remote Sensing 52 (2): 907–919. https://doi.org/10.1109/TGRS.2013.2245670.
- Roujean, J. L., M. Leroy, and P. Y. Deschamps. 1992. “A Bidirectional Reflectance Model of the Earth’s Surface for the Correction of Remote Sensing Data.” Journal of Geophysical Research: Atmospheres 97: 20455–20468. https://doi.org/10.1029/92JD01411.
- Roy, D. P., V. Kovalskyy, H. K. Zhang, E. F. Vermote, L. Yan, S. S. Kumar, and A. Egorov. 2016. “Characterization of Landsat-7 to Landsat-8 Reflective Wavelength and Normalized Difference Vegetation Index Continuity.” Remote Sensing of Environment 185: 57–70. https://doi.org/10.1016/j.rse.2015.12.024.
- Roy, D. P., J. Li, H. K. Zhang, L. Yan, H. Huang, and Z. Li. 2017. “Examination of Sentinel-2A Multi-Spectral Instrument (MSI) Reflectance Anisotropy and the Suitability of a General Method to Normalize MSI Reflectance to Nadir BRDF Adjusted Reflectance.” Remote Sensing of Environment 199: 25–38. https://doi.org/10.1016/j.rse.2017.06.019.
- Schaaf, C. B., F. Gao, A. H. Strahler, W. Lucht, X. Li, T. Tsang, N. C. Strugnell, et al. 2002. “First Operational BRDF, Albedo Nadir Reflectance Products from MODIS.” Remote Sensing of Environment 83: 135–148. https://doi.org/10.1016/S0034-4257(02)00091-3.
- Sun, Y., C. Frankenberg, M. Jung, J. Joiner, L. Guanter, P. Köhler, and T. Magney. 2018. “Overview of Solar-Induced Chlorophyll Fluorescence (SIF) from the Orbiting Carbon Observatory-2: Retrieval, Cross-Mission Comparison, and Global Monitoring for GPP.” Remote Sensing of Environment 209: 808–823. https://doi.org/10.1016/j.rse.2018.02.016.
- Tang, R., X. Wu, J. Wang, L. Zheng, Q. Zeng, J. Wen, and Q. Xiao. 2023. “A Geometric Location Matching Method for Validation of Satellite Products: A Case Study for Albedo.” IEEE Geoscience and Remote Sensing Letters 19: 3001405. https://doi.org/10.1109/LGRS.2023.3253550.
- Turner, A. J., P. Köhler, T. S. Magney, C. Frankenberg, I. Fung, and R. C. Cohen. 2020. “A Double Peak in the Seasonality of California’s Photosynthesis as Observed from Space.” Biogeosciences (online) 17 (2): 405–422. https://doi.org/10.5194/bg-17-405-2020.
- Wu, X., J. Wen, Q. Xiao, Y. Bao, D. You, J. Wang, D. Ma, X. Lin, and B. Gong. 2021. “Quantification of the Uncertainty Caused by Geometric Registration Errors in Multiscale Validation of Satellite Products.” IEEE Geoscience and Remote Sensing Letters 9: 1–5. https://doi.org/10.1109/LGRS.2021.3099833.
- Wu, X., J. Wen, Q. Xiao, Q. Liu, J. Peng, B. Dou, X. Li, D. You, Y. Tang, and Q. Liu. 2016. “Coarse Scale in Situ Albedo Observations Over Heterogeneous Snow-Free Land Surfaces and Validation Strategy: A Case of MODIS Albedo Products Preliminary Validation Over Northern China.” Remote Sensing of Environment 184: 25–39. https://doi.org/10.1016/j.rse.2016.06.013.
- Wu, X., Q. Xiao, J. Wen, D. You, and A. Hueni. 2019. “Advances in Quantitative Remote Sensing Product Validation: Overview and Current Status.” Earth-Science Reviews 196: 102875. https://doi.org/10.1016/j.earscirev.2019.102875.
- Yang, J., and X. Huang. 2021. “The 30 m Annual Land Cover Dataset and its Dynamics in China from 1990 to 2019.” Earth System Science Data 13 (8): 3907–3925. https://doi.org/10.5194/essd-13-3907-2021.
- Yu, G. R., X. F. Wen, X. M. Sun, B. D. Tanner, X. H. Lee, and J. Y. Chen. 2006. “Overview of ChinaFLUX and Evaluation of its Eddy Covariance Measurement.” Agricultural and Forest Meteorology 137: 125–137. https://doi.org/10.1016/j.agrformet.2006.02.011.
- Zeng, Y., G. Badgley, B. Dechant, Y. Ryu, M. Chen, and J. A. Berry. 2019. “A Practical Approach for Estimating the Escape Ratio of Near-Infrared Solar-Induced Chlorophyll Fluorescence.” Remote Sensing of Environment 232: 111209. https://doi.org/10.1016/j.rse.2019.05.028.
- Zhang, Y., Q. Zhang, L. Liu, Y. Zhang, S. Wang, W. Ju, G. Zhou, et al. 2021. “ChinaSpec: A Network for Long-Term Ground-Based Measurements of Solar-Induced Fluorescence in China.” Journal of Geophysical Research: Biogeosciences 126: e2020JG006042. https://doi.org/10.1029/2020JG006042.
- Zhong, Y., Q. Xiao, J. Wen, X. Zheng, M. Ma, Y. Qu, K. Zheng, et al. 2020. “Design and realization of ground object background spectral library for surveying and mapping.” Journal of Remote Sensing 24: 701–716. https://doi.org/10.11834/jrs20209482.
- Zhu, X., Y. Hou, Y. Zhang, X. Lu, Z. Liu, and Q. Weng. 2021. “Potential of Sun-Induced Chlorophyll Fluorescence for Indicating Mangrove Canopy Photosynthesis.” Journal of Geophysical Research: Biogeosciences 126 (4): e2020JG006159. https://doi.org/10.1029/2020JG006159.