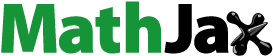
ABSTRACT
Due to the difficulty of obtaining survey data, nighttime light (NTL) imagery has emerged as valuable alternative data for asset wealth estimation. However, the nighttime light values do not differentiate between levels of asset wealth in various unlit areas, as the nighttime light values in unlit areas are all 0. Here, NTL data and World Settlement Footprint (WSF) data were combined to extract multidimensional luminous features that are also differential in unlit areas to estimate asset wealth in nighttime light-poor areas at 500 m × 500 m spatial units. A random forest model was used to estimate asset wealth, based on the shortest distance of settlements to three categories of lighted areas, along with the brightness values derived from the nearest lighted area and the settlements themselves. This model achieved an explanation of 71% for the variation in settlement asset wealth and demonstrated effectiveness in estimating the asset wealth of unlit areas. The MAE and RMSE of asset wealth estimation in the unlit clusters were 4.03 and 5.28, respectively. Asset wealth is generally low across most African settlements, with clear two-tier differentiation in Africa. In summary, the proposed method can extensively explore the luminous information in unlit areas.
1. Introduction
Poverty has long been a significant social issue impeding the sustainable development of humanity (Leal Filho et al. Citation2021). As such, the first Sustainable Development Goal (SDG) established by the United Nations Sustainable Development Summit aims to eradicate all forms of poverty worldwide (Halisçelik and Soytas Citation2019; Schmidt-Traub et al. Citation2017). Measuring regional asset wealth can offer crucial location-based insights into the distribution of poverty, empowering governments and non-governmental organizations to promote the proper allocation of public resources and international aid. Traditionally, household asset surveys and economic statistics have been the primary methods for measuring asset wealth. However, numerous developing countries, particularly in Africa, lack sufficient statistical data, hindering efforts to identify the geographical distribution of impoverished populations (Diaz-Sarachaga, Jato-Espino, and Castro-Fresno Citation2018; Elvidge et al. Citation2009). Billions of dollars of international aid are trying to flow to areas of extreme poverty to address the problem of poverty every year, but researches indicate that much of this aid ends up in wealthier areas (Briggs Citation2018, Citation2021). Given the data limitations associated with traditional measures of human asset wealth, exploring alternative data sources, such as nighttime light (NTL) remote sensing data, has proven effective and feasible (Levin et al. Citation2020; Miller et al. Citation2012).
NTL remote sensing satellites can capture information on artificial light emissions stemming from human social activities during the night. Early studies have demonstrated a strong correlation between NTL and regional economic indicators, which can be used as proxy data for monitoring regional socio-economic development (Bennett and Smith Citation2017; Chen et al. Citation2022; Chen and Nordhaus Citation2011; Gibson, Olivia, and Boe-Gibson Citation2020; Mellander et al. Citation2015). Consequently, NTL data has been extensively employed in studies on measuring regional asset wealth and poverty identification. Some studies have explored the relationship between NTL and regional wealth levels, using machine learning models to estimate economic well-being and poverty at the grid level (Lin et al. Citation2022; McCallum et al. Citation2022) or different administrative levels (Jean et al. Citation2016; Xu et al. Citation2021). Furthermore, some studies have integrated multi-source data to compensate for the lack of luminous information in unlit areas (Lee and Braithwaite Citation2022; Niu, Chen, and Yuan Citation2020; Shao and Li Citation2023; Yeh et al. Citation2020). For instance, Yeh et al. (Citation2020) used daytime imagery to supplement the information with differences between unlit areas, while Lee and Braithwaite (Citation2022) incorporated OpenStreetMap (OSM) data to improve regional asset wealth estimation. Points of interest (POI) data contain a large amount of information on the location and attributes of geographic entities involved in human activities, from which information on the spatial distribution of regional facilities and services can be obtained, and have been widely used in poverty estimation (Hu et al. Citation2022; Niu, Chen, and Yuan Citation2020; Shi et al. Citation2020). Niu, Chen, and Yuan (Citation2020) combined nighttime light images with social media data, such as POI and housing rents, to measure urban poverty. Despite these advancements, existing studies have yet to fully extract the differential luminous information of unlit areas to estimate the asset wealth of unlit areas. However, recent research suggests the feasibility of fully utilizing multidimensional luminous information from unlit areas to estimate the asset wealth of unlit areas (McCallum et al. Citation2022).
In numerous African countries where nighttime light is scarce, using NTL imagery to estimate regional asset wealth may not yield satisfactory results, as the asset wealth of unlit areas cannot be effectively estimated (McCallum et al. Citation2022; Smith and Wills Citation2018). NTL imagery not only provides information about lighted areas, but luminous information derived from unlit areas can also serve as remote sensing information reflecting asset wealth. By utilizing the luminous information from unlit areas, it becomes feasible to better estimate the asset wealth of regions lacking adequate lighting, thereby achieving greater precision in assessing regional asset wealth in fine spatial resolution. However, currently, the information disparity within unlit areas has not been fully explored, as only the information that unlit areas have an NTL brightness value of 0 has been utilized, resulting in a significant homogenization of luminous information in unlit areas and hindering the ability to estimate asset wealth within these areas. Consequently, this study aims to extract multidimensional luminous features that are also differential in unlit areas, thus enhancing the accuracy of using nighttime light data to estimate asset wealth in nighttime light scarce areas at 500 m × 500 m spatial units.
In this study, the multidimensional luminous information was utilized to achieve a fine-grained estimate of regional asset wealth. We combined the 2015 World Settlement Footprint (WSF) dataset with NTL imagery to spatially determine whether settlements have nighttime lighting. Guided by the principle that settlements in proximity to lighted areas and exhibiting higher NTL brightness values are indicative of higher asset wealth, we extracted the shortest distance of settlements to three categories of lighted areas, along with the brightness values derived from the nearest lighted area and the settlements themselves. These pieces of information combined with household wealth data, we estimated and generated asset wealth maps for African countries at a spatial resolution of 500 m.
2. Data
2.1. Wealth Index data
We collected the Wealth Index (WI) data from the Demographic and Health Surveys (DHS) available through the DHS Program (https://dhsprogram.com/data). These DHS WI data reflect the household asset wealth. We obtained DHS WI data from 19,467 clusters, representing a total of 450,927 households, spread across 33 African countries (). The data collection period spanned from 2011 to 2019. Each cluster's DHS WI value represents the average WI of all households within that cluster. Alongside WI data, we collected geographical coordinates for each cluster. Calculated based on observable and collectible household assets, the DHS WI is divided into five quintiles (1–5). Household assets include the number of rooms occupied in the household, whether the house has electricity, the quality of the flooring in the house, the water supply and toilets, as well as the ownership of telephones, radios, televisions, automobiles, and motorcycles (Rutstein and Johnson Citation2004; Yeh et al. Citation2020). Asset-based wealth indices are considered to be indicators that can measure long-term economic well-being while minimizing interference (Benton and Keister Citation2017; Filmer and Scott Citation2012; Haveman and Wolff Citation2004; Sahn and Stifel Citation2003). Given the provision of specific geographical coordinates for survey clusters, the DHS WI has been extensively utilized in research requiring precise location data of survey subjects. However, to safeguard respondent privacy, the geographical coordinates provided by the DHS WI undergo random shifts. Urban areas experience a maximum shift of 2 kilometers, while rural areas exhibit a maximum shift of 5 kilometers, with 1% of rural areas subjected to a maximum shift of 10 kilometers. The shifting of geographic coordinates introduces uncertainty into prediction models.
2.2. International Wealth Index data
The DHS WI serves as a survey indicator evaluating the relative economic conditions within a specific region and is not directly comparable across different regions and periods. To construct a comparable asset wealth index, Smits and Steendijk (Citation2015) developed the International Wealth Index (IWI) based on household assets, including 7 durable goods (TV, refrigerator, telephone, bicycle, car, inexpensive utensils, and expensive utensils), 2 public services (water and electricity), and 3 housing characteristic assets (number of bedrooms, quality of floor material, and quality of toilet facilities) (Smits and Steendijk Citation2015). We obtained country-level IWI data for African countries from Global Data Lab (https://globaldatalab.org/). By utilizing the IWI, we can obtain a more uniform and consistent assessment of asset wealth across diverse geographical regions and timeframes.
2.3. Nighttime light image
We downloaded the annual nighttime light (NTL) image of Africa for the year 2015 from the Visible Infrared Imaging Radiometer Suite Day/Night Band (VIIRS DNB) V2.1 dataset from Earth Observation Group (https://eogdata.mines.edu/products/vnl/). We use a cloudless and moonlight-free average radiance brightness product that filters out the moonlight and cloudy pixels and discards biomass-burning pixels using outlier removal methods (Elvidge et al. Citation2017, Citation2021). The annual NTL image boasts a spatial resolution of 15 arc-seconds (approximately 500 m at the equator). The NTL image underwent projection and resampling processes to achieve a spatial resolution of 500 m.
2.4. World Settlement Footprint data
We obtained the 2015 African World Settlement Footprint (WSF) data from Figshare (https://figshare.com/articles/dataset/World_Settlement_Footprint_WSF_2015_-_Percent_Settlement_Area_-_500m/10048520). This dataset provides detailed information about the distribution of human settlements across Africa. WSF2015 is a high-accuracy product generated using Landsat-8 multispectral imagery and Sentinel-1 radar imagery, which has been validated and assessed, demonstrating an average accuracy of 86.37% (Marconcini et al. Citation2020; McCallum et al. Citation2022). It stands out as the most reliable and precise product among comparable offerings presently available (Marconcini et al. Citation2020; McCallum et al. Citation2022). The WSF2015 data we downloaded has a spatial resolution of approximately 500 m. We projected and resampled the data to a 500 m spatial resolution. Within the WSF2015 dataset, the pixel values represent the proportion of the pixel area occupied by human settlements.
3. Methods
To address the problem of not being able to differentiate between levels of asset wealth in various unlit areas because nighttime light values in unlit areas are all 0, this study combines nighttime light (NTL) image and World Settlement Footprint (WSF) data to extract multidimensional luminous features that are also differential in unlit areas to estimate asset wealth at 500 m × 500 m spatial units. Firstly, WI data underwent processing to generate the International Wealth Index (IWI) that is comparable over time and across countries. Subsequently, multidimensional luminous features, especially spatial neighborhood information of settlements and lighted areas, such as the shortest distance to the lighted areas and the brightness of that nearest lighted area, were extracted. Lastly, a random forest prediction model for asset wealth was built, generating an asset wealth distribution map at a spatial resolution of 500 m in Africa. The specific process is shown in .
3.1. Construction of comparable WI
The DHS WI is a regional survey indicator that measures the relative economic level of households within specific periods and countries or regions. It can only be used within specific periods and countries or regions. Therefore, the DHS WI data needs further processing to render it comparable across different periods and regions. To address this limitation, we have used the IWI as a comparable wealth index for this study because it is based on household surveys conducted in low and middle-income countries and can be used across all low and middle-income countries with comparability across different periods and countries.
Rutstein and Staveteig (Citation2014) proposed the Comparative Wealth Index (CWI), which showed a strong correlation with the logarithm of per capita Gross National Income adjusted for purchasing power parity [ln (per capita GNI)] (Rutstein and Staveteig Citation2014). Upon comparing the IWI with ln (per capita GNI), we found a high correlation between the two variables, with an R2 of 0.74 (). This indicates that the IWI has similar economic characteristics to the CWI, further demonstrating the validity and reliability of the IWI in reflecting asset wealth.
Figure 3. Correlation of country-level IWI and the logarithm of per capita Gross National Income (GNI).

To transfer the comparability of IWI to survey clusters, the comparability adjustment was applied to cluster WI using the African country-specific IWI. The calculation formula is shown in EquationEquation (1(1)
(1) ).
(1)
(1) where
represents the IWI of the jth survey cluster in the ith country, WIij represents the WI of the jth survey cluster in the ith country, IWIi represents the IWI of the ith country, and WIi·ave represents the average WI of survey clusters in the ith country.
We have normalized by scaling its values to a range of [0, 100]. The calculation formula is shown in EquationEquation (2
(2)
(2) ).
(2)
(2) where IWIij represents the normalized IWI of the jth survey cluster in the ith country after the normalization process.
3.2. Multidimensional luminous feature extraction
Early research has demonstrated a strong correlation between NTL and regional economic indicators (Levin et al. Citation2020). Multidimensional luminous features are extracted based on the principle that the lighted area has a positive impact on the asset wealth of the surrounding area. Raster pixels with NTL and WSF values greater than 0 were converted into vector points, which were luminous points and settlement points, respectively. The nearest neighbor analysis is subsequently used to calculate the shortest distance between each settlement point and its corresponding luminous points. Considering that weak NTL may have a limited impact on neighborhood asset wealth. The regional median and mean nighttime light values are utilized to distinguish between relatively high NTL regions and relatively low NTL regions in terms of the number and value of luminous points. Furthermore, the median (0.78 nW/cm2/sr) and mean (3.70 nW/cm2/sr) luminous point values for Africa are calculated. Luminous points with NTL values greater than or equal to the median and mean nighttime light values were extracted as median luminous points and mean luminous points, respectively. The shortest distance between each settlement point and the median and mean luminous points are then calculated. The clusters were divided into 10 IWI grades with intervals of 10 and plotted box plots for the three distance values for each grade (). In the five grades of IWI ranging from 0 to 50, all three box plots showed a decreasing trend with increasing IWI, underscoring a discernible correlation between the shortest distance and asset wealth.
Figure 4. Box plots depicting the distribution patterns of distances between cluster settlement points in ten IWI grades and three levels of lighted areas. In (a), it represents the shortest distance from areas with NTL greater than 0. In (b), it represents the shortest distance from areas with NTL greater than or equal to 0.78. In (c), it represents the shortest distance from areas with NTL greater than or equal to 3.70. The median is represented by the central line and triangle shape. The lower and upper limits of the box represent the first (Q1) and third (Q3) quartiles, and the lower and upper whiskers depict the minimum and maximum values, respectively, excluding outliers. Outliers are defined as values greater than Q3 + 1.5 * (Q3-Q1) or smaller than Q1 – 1.5 * (Q3-Q1).

However, this relationship disappeared in grades with an IWI greater than 50. This phenomenon arises because the majority of settlement points in the 50–100 grades already exhibit NTL, resulting in shortest distance values clustering around 0 within these grades. To address this, efforts were made to enhance differentiation within the 50–100 IWI grade by incorporating NTL brightness features. Considering the varying impact of different NTL brightness values on surrounding asset wealth, brightness values from the nearest luminous point, nearest median luminous point, and nearest mean luminous point were extracted and assigned to the corresponding settlement points. Additionally, to account for the direct reflection of the settlement point's own NTL brightness on its asset wealth, the NTL brightness value of the settlement point itself was also extracted.
From this, the shortest distances from each settlement point to the luminous points, median luminous points, and mean luminous points were obtained, as well as the NTL brightness value of the corresponding nearest luminous point, and the NTL brightness value of the settlement point itself. Consequently, each settlement point includes three distance values and four brightness values (). Due to the spatial bias of clusters, the study established 2 and 5 km buffer zones for clusters in urban and rural areas, respectively. The average shortest distance values and brightness values of the settlement points within the buffer zone were assigned to the corresponding cluster, as the cluster's distance values and brightness values.
Table 1. Multidimensional luminous feature.
3.3. Random forest model
To estimate asset wealth in Africa by integrating the cluster's IWI with the cluster's distance values and brightness values, we chose to use a random forest model. The random forest is an ensemble learning model based on a bagging algorithm (Breiman Citation1996, Citation2001). It creates multiple decision trees, referred to as ensembles or a forest, for prediction. Each tree generates its prediction, contributing to the final prediction through a voting mechanism. The final prediction is not based on any individual tree but on an entire forest, helping to avoid overfitting the model (Gu et al. Citation2022; Matsuki, Kuperman, and Van Dyke Citation2016). The random forest model can handle multidimensional data and address multicollinearity, rendering it well-suited for processing our multidimensional luminous features (Niu, Chen, and Yuan Citation2020). Due to these advantages, random forest models can accurately estimate asset wealth or poverty based on datasets like nighttime light data (Hu et al. Citation2022; Niu, Chen, and Yuan Citation2020; Shao and Li Citation2023). In addition, the ability of the random forest model to analyze feature importance can help us understand the importance of multidimensional nightlight features for the predictive model.
We utilized the cluster's IWI as the predicted variable and employed the cluster's distance values and brightness values as the explanatory variables to construct the random forest prediction model. Furthermore, we employed the distance values and brightness values of the settlement points as the explanatory variables for prediction, resulting in the model outputting the estimated IWI values for settlement points in Africa. The performance of the training model was evaluated according to a mean square error of the out-of-bag error of the model and explained the percentage of the change. Out-of-bag error represents the model's error calculated using unselected samples from the training data. The hyperparameters of the random forest model were determined based on the performance of the training model (). Additionally, ten-fold cross-validation was conducted to validate the model's performance. This involved randomly dividing the training data into ten subsets, with nine subsets used for training and the remaining subset used for evaluating the model's predictive performance.
Table 2. Random forest model hyperparameters.
4. Results
4.1. The shortest distance between the settlement point and the lighted area
The shortest distances of African settlement points to luminous points, median luminous points, and mean luminous points were calculated as shown in . Satellites did not detect nighttime light in 34.98% of the African settlement areas. This result indicates that a significant portion of the building facilities in Africa lacks satellite-detectable nighttime light. Therefore, utilizing unlit area information for asset wealth estimations has evident advantages by providing supplementary information. The average shortest distances between African luminous points and luminous points, median luminous points, and mean luminous points were 11.49, 15.20, and 29.39 km, respectively. Regions with larger distance values are primarily located in the Democratic Republic of the Congo, Central Africa, Chad, Mali, Somalia, and the island country of Madagascar.
Figure 5. Shortest distance between settlement points and lighted areas. (a) The shortest distance between settlement points at a resolution of 500 m and lighted areas with NTL greater than 0. (b) The shortest distance between settlement points at a resolution of 500 m and lighted areas with NTL greater than or equal to 0.78. (c) The shortest distance between settlement points at a resolution of 500 m and lighted areas with NTL greater than or equal to 3.70. (d) The average shortest distance of settlement points in 27 countries or areas where the three distances add up to the smaller distance to the three lighted level areas. (e) The average shortest distance of settlement points in 27 countries or areas where the three distances add up to the greater distance to the three lighted level areas.

4.2. Model accuracy validation
Based on the cluster International Wealth Index (IWI) and 7 predictor variables, the random forest asset wealth prediction model was developed for African settlement points, capable of generating asset wealth estimation maps at a resolution of 500 m. The mean of estimated IWI values within the cluster buffer zone was considered as the estimated IWI for the cluster buffer zone. The coefficient of determination (R2) between the observed and estimated IWI for the cluster reached 0.71 (), indicating that our prediction model can explain 71% of the variation in the observed IWI for the clusters. The Mean Absolute Error (MAE) and Root Mean Square Error (RMSE) of asset wealth estimation in the lighted clusters were recorded at 8.85 and 11.73 respectively. The MAE and RMSE of asset wealth estimation in the unlit clusters were lower at 4.03 and 5.28, respectively. This suggests that our prediction model possesses good predictive performance, especially an ability to estimate asset wealth in unlit clusters with high accuracy. Previous studies have primarily relied on the nighttime light brightness value of settlements themselves to estimate asset wealth (Weidmann and Schutte Citation2017). As can be seen from (b), compared with the prediction model that only uses the NTL brightness value of settlements themselves, our model significantly improves the accuracy estimates for unlit areas. Specifically, the MAE and RMSE for unlit areas were reduced by 31.35% and 31.43%, respectively. There is also a degree of error reduction in the lighted area.
Figure 6. Comparison of data between estimated IWI and observed IWI based on survey data. (a) and (c) represent the validation results for clusters and sub-national levels, respectively. (b) Represents the validation results using only the brightness values of the NTL of the settlements.

Furthermore, the observed and estimated IWI values were both aggregated at the sub-national levels and computed the R2 between observed and estimated IWI. The R2 value between observed and estimated IWI at the sub-national levels was found to be 0.80. The MAE and RMSE of the estimated IWI at the sub-national levels were determined to be 4.42 and 5.92, respectively. These accuracy validation results show that aggregating the estimated IWI at higher geographic levels further enhances the model's performance.
To further validate the predictive performance of the prediction model in the case where all survey clusters were unlit areas, only unlit clusters were selected as the model sample to construct the prediction model to estimate asset wealth in unlit areas. The results show that the MAE and RMSE of asset wealth estimation in unlit clusters are 4.03 and 5.29, respectively. These findings indicate that the model performs well in estimating asset wealth in unlit areas, even when there are only samples of unlit clusters ().
4.3. Impact of luminous feature selection on model performance
A comparison was made between the errors of estimating asset wealth using different features for lighted and unlit areas. The effect of selecting different features on the model's performance was analyzed (). The results show that incorporating the distance feature of settlements from the lighted area, along with the brightness feature of this lighted area can improve the model's performance, and in particular, it can significantly reduce the estimation error in the unlit area. The accuracy of estimating unlit areas was further improved by using multidimensional luminous features.
Table 3. Error analysis with different features.
The improvement in model performance is visualized by plotting the estimated International Wealth Index (IWI) for Nigeria, the most populous country in Africa. From (b and d), it becomes apparent that if only the NTL brightness values of the settlements themselves are used, lacking differential luminous information in the unlit areas makes their estimated IWI all the same. Differences in estimated IWI for unlit areas emerged after the inclusion of multidimensional luminous features [(a)]. Combined with the IWI obtained from the field survey [(c)], the two models estimated IWI for the surveyed clusters were extracted for error comparison. From (e) and (f), it can be seen that the errors of estimated IWI obtained from the multidimensional luminous features are significantly reduced, with most of the unlit areas experiencing reduced errors and only a small portion of the unlit areas experiencing a low increase in error. The overall error in the cluster IWI in the lighted areas is also reduced.
Figure 8. Asset wealth prediction model error analysis. (a) and (b) represent the Nigerian IWI estimated using multidimensional luminous features and using only the NTL brightness values of the settlements themselves, respectively. (c) Represent the cluster IWI obtained from the field survey. (d) Represent the map of NTL in Nigeria. (e) and (f) are the error reductions in unlit and lighted areas, respectively, with the use of multidimensional luminous features, relative to the use of only the NTL brightness values of the settlements themselves.

Furthermore, the model's performance was assessed with different explanatory variables (). When the shortest distance to the luminous points was utilized as a sole explanatory variable, two high-density peaks were observed in the estimated IWI density clusters, which differed greatly from the density distribution shape of the observed IWI clusters. This inability to provide sufficient information to distinguish regional asset wealth differences resulted in overly concentrated estimation results, particularly for clusters with IWI within the 50–100 range. The limitation of using the shortest distance to the luminous points as a sole explanatory variable was also identified, as analyzed in . The inclusion of NTL brightness values of the nearest luminous point as explanatory variables significantly altered the shape of the estimated IWI density distribution, making it more closely resemble the shape of the observed IWI density distribution. This alleviated the issue of high-density peaks and enabled effective estimation of cluster IWI within the 50–100 range. By multidimensional luminous features as explanatory variables in the model, the shape of the estimated IWI density distribution further approached that of the observed IWI, effectively improving the model's performance.
Figure 9. Cluster density and average IWI values for ten IWI categories. The areas represent cluster density and the dots represent the average values. Grey represents observed IWI, red represents estimated IWI with only the shortest distance to luminous points as the explanatory variable, blue represents estimated IWI with the shortest distance to the luminous point and the nearest luminous point brightness value as explanatory variables, and green represents estimated IWI with multidimensional luminous features as explanatory variables.

From the average values of the 10 IWI categories (with an interval of 10), it is evident that the estimated IWI obtained by using multidimensional luminous features as explanatory variables is closer to the observed cluster IWI. However, there is an issue of overestimation or underestimation in the model's estimation results, which is most apparent in the 0–10 and 50–100 cluster IWI ranges. Within the 0–30 cluster IWI range, the model tends to overestimate cluster asset wealth, with the overestimation being particularly pronounced in the 0–10 IWI category. Conversely, within the 30–100 cluster IWI range, the model shifts towards underestimating cluster asset wealth. Overall, incorporating multidimensional luminous features as explanatory variables can effectively improve the model's predictive performance.
The random forest model was used to obtain the importance values of seven features and test the necessity of the features based on their contribution to the asset wealth prediction model (). The results show that all seven features are significant contributors to the model, with the NTL brightness value of the settlements themselves being the most important. Conversely, the shortest distance to the luminous points and the brightness value of the nearest mean luminous point are identified as the least important features in the model.
4.4. Spatial distribution analysis of asset wealth in Africa
The asset wealth of 3,192,638 settlements was estimated in Africa based on the asset wealth prediction model (). The International Wealth Index (IWI) of the settlements is excessively concentrated within the range of 10–20, with 56.46% of settlements falling within this range. The high-asset wealth areas are mainly located in Egypt, Libya, Algeria, Morocco, and Tunisia, as well as in South Africa. On the other hand, low asset wealth areas are predominantly found in Congo (DRC), Central African Republic, and Madagascar. The average and median IWI values for all settlements in Africa are 21.4 and 17.1, respectively. For settlements in lighted areas, the average and median IWI values are notably higher at 34.0 and 32.3, while for unlit areas, they are 14.9 and 14.0, respectively. In terms of human settlement area, the average and median IWI values for all settlements in Africa are 31.2 and 30.3, for lighted areas they are 39.6 and 36.0, and for unlit areas, they are 15.5 and 14.4, respectively.
Figure 11. Estimated asset wealth results and distribution patterns of settlements in Africa. (a) Spatial distribution of estimated asset wealth for settlements in Africa at a resolution of 500 m. (b) Distribution patterns of the number and area of settlements in lighted areas and unlit areas based on twenty estimated IWI categories (with an interval of 5).

The distribution shape of asset wealth in unlit areas, based on both the number and area of settlements, is similar, and the average and median values are relatively close [(b)]. However, the distribution shapes of asset wealth based on settlement area show significant variation in lighted areas, with a noticeable peak around the range of 30–40 [(b)]. This is because settlements in lighted areas have a larger proportion of human settlement areas, especially for settlements with higher asset wealth values, which tend to have larger human settlement areas. As a result, the average and median values of IWI based on settlement area in lighted areas are higher than those based on the number of settlements. This also leads to a significant increase in the average and median values of IWI for the entire Africa when considering the settlement area.
The spatial distribution of asset wealth at the sub-national level was mapped by aggregating asset wealth from settlements at 500 m resolution [(a)]. The areas of high asset wealth values are mainly located in northern and southern Africa. To further analyze the distribution characteristics of the asset wealth of the settlements, a quantile classification method was employed (Goswami and Chakrabarti Citation2012). The numbers of settlements with IWI values located at 0–33%, 33–66%, and 66–100% of the IWI at the subnational level were counted, respectively. The 33% and 66% quantiles of IWI values for African settlements were 14.04 and 22.20, respectively. A ternary diagram was plotted using the proportions of settlements in these three IWI ranges as dimensions [(b)]. Each point on the ternary diagram corresponds to the proportion of settlements in the three dimensions. A point draws an extension line counterclockwise along the direction of the three sides of the equilateral triangle, and the position where the extension line intersects the axis is the ratio of this dimension.
Figure 12. Spatial distribution and internal distribution of asset wealth at the sub-national level. (a) Spatial distribution results of sub-national asset wealth. (b) Ternary plot of sub-national level. The proportions of settlements with IWI in the ranges of 0–33%, 33–66%, and 66–100% are represented as three dimensions. The color of the sub-national level points corresponds to the average IWI value of the settlements.

The results show that the majority of points are distributed near the left and right sides of the ternary diagram. Points distributed near the left side signify regions where the number of settlements with IWI values falling within the 0–33% range is minimal, while those falling within the 66–100% range are substantial, indicating higher average asset wealth in these areas. Conversely, points located near the right side exhibit a lower proportion of settlements with IWI values in the 66–100% range and a higher proportion in the 0–33% range, suggesting lower average asset wealth in these regions. Hence, it can be inferred that there is a significant two-level differentiation in asset wealth in the sub-national of Africa, along with an existing problem of asset wealth inequality within regions.
5. Discussion
We extracted luminous information from settlements, especially unlit areas, and used them as inputs for the random forest prediction model to generate a fine-grained map of asset wealth in Africa with a spatial resolution of 500 m. Our prediction method may help developing countries with limited statistical survey data understand the precise distribution of asset wealth within their countries. Particularly in areas with a lack of lighting, our prediction model compensates for the lack of differential information in unlit areas. Overall, we provide a low-cost, accurate, easy-to-implement, and scalable approach to predicting asset wealth.
Based on the model accuracy validation results, our estimated International Wealth Index (IWI) has a strong correlation with the observed IWI, with an R2 value of 0.71. The performance of our model is comparable to or even surpasses previous relevant studies. For example, a study utilizing high-resolution satellite imagery and a machine learning model based on ‘transfer learning’ estimated asset wealth with an R2 value of 0.56 (Jean et al. Citation2016). Another study combined nighttime and daytime imagery and used a deep learning model to predict asset wealth in Africa, achieving an R2 value of 0.70. However, the interpretability challenges of deep learning hindered the translation of research findings into policy (Yeh et al. Citation2020). Furthermore, our model provides a finer spatial resolution for the spatial distribution of asset wealth.
Current research that combines nighttime light data and multi-source data, such as POI data and OSM data, to estimate asset wealth or poverty has achieved high accuracy (Hu et al. Citation2022; Lee and Braithwaite Citation2022). However, current studies have not fully utilized the differential luminous information in unlit areas, making nighttime light data difficult to effectively estimate asset wealth or poverty in areas with relatively scarce nighttime light (McCallum et al. Citation2022). The results of this paper demonstrate the feasibility and validity of using multidimensional luminous features to estimate asset wealth in nighttime light-poor areas at 500 m × 500 m spatial units. However, our prediction model has some limitations. For example, the estimated results for areas with very low asset wealth values are not ideal because the input luminous information in these areas lacks sufficient differentiation. Consequently, the estimated results for areas with very low asset wealth values tend to be closer to areas with higher asset wealth values. Combining other sources of data may improve the model's performance, particularly data that provide significant differentiation in areas with low asset wealth values. Additionally, the positional offset of DHS WI data is a major source of uncertainty in the model. Assuming that WSF within the cluster buffer area represents households in that cluster may lead to discrepancies between the actual surveyed households and those within the buffer zones. In the future, if more accurate positional information becomes available, it may further enhance the model's performance.
The current overpass time of Suomi NPP is often late, which may lead to missing a significant portion of the light generated from production and living activities (Stokes et al. Citation2021). Additionally, faint light in rural areas may be difficult to capture by satellites (Kyba et al. Citation2017), which could result in an overestimation of the distance between settlements and lighted areas, and reduce the distinction of information in regions with lower asset wealth. Additionally, WSF data may not detect some extreme poverty dwellings (huts, shacks, tents), and as these housing structures are unlikely to have lights, it would underestimate the distance between settlements and lighted areas. We hope that future advancements in NTL satellite technology and improvements in settlement products can address these issues as much as possible and enhance the performance of our model.
6. Conclusions
In this paper, the asset wealth prediction method has been proposed based on NTL satellite images, which explored the luminous information, especially in unlit areas, to generate an asset wealth map at a spatial resolution of 500 m for Africa. It is difficult to use nighttime light data to estimate asset wealth in unlit areas because the current research does not adequately extract differential luminous information in unlit areas (McCallum et al. Citation2022). In contrast, our method is advantageous in capturing differentiated information from NTL images in areas with limited light, making it suitable for estimating asset wealth in night-light-poor regions of Africa. Through model validation, our model effectively estimates the spatial distribution of asset wealth in Africa, explaining 71% of the variation in asset wealth across settlements. The MAE and RMSE of asset wealth estimation in unlit areas were only 4.03 and 5.28, respectively. The MAE and RMSE for estimated asset wealth in unlit areas were reduced by 31.35% and 31.43%, respectively, compared to using only the NTL brightness values from the settlements themselves.
A large proportion of settlements in Africa lack satellite-detectable nighttime light. Utilizing multidimensional luminous features, asset wealth can be effectively estimated in both unlit and lighted areas. In Africa, the majority of settlements have relatively low levels of asset wealth. There is a clear two-tier differentiation in asset wealth at the sub-national level within African countries, such as the Democratic Republic of Congo, Angola, Somalia, and Chad.
Asset wealth maps have been crucial data products for monitoring and promoting sustainable development goals such as poverty eradication in regions. Our model may provide low-cost asset wealth mapping for developing countries rapidly and efficiently, allowing for continuous data updates to align with regional development needs.
Disclosure statement
No potential conflict of interest was reported by the author(s).
Data availability statement
The data used in this study are available by contacting the corresponding author.
Additional information
Funding
References
- Bennett, M. M., and L. C. Smith. 2017. “Advances in Using Multitemporal Night-Time Lights Satellite Imagery to Detect, Estimate, and Monitor Socioeconomic Dynamics.” Remote Sensing of Environment 192: 176–197. https://doi.org/10.1016/j.rse.2017.01.005.
- Benton, R. A., and L. A. Keister. 2017. “The Lasting Effect of Intergenerational Wealth Transfers: Human Capital, Family Formation, and Wealth.” Social Science Research 68: 1–14. https://doi.org/10.1016/j.ssresearch.2017.09.006.
- Breiman, L. 1996. “Bagging Predictors.” Machine Learning 24: 123–140. https://doi.org/10.1007/BF00058655.
- Breiman, L. 2001. “Random Forests.” Machine Learning 45: 5–32. https://doi.org/10.1023/A:1010933404324.
- Briggs, R. C. 2018. “Poor Targeting: A Gridded Spatial Analysis of the Degree to Which Aid Reaches the Poor in Africa.” World Development 103: 133–148. https://doi.org/10.1016/j.worlddev.2017.10.020.
- Briggs, R. C. 2021. “Why Does Aid Not Target the Poorest?” International Studies Quarterly 65 (3): 739–752. https://doi.org/10.1093/isq/sqab035.
- Chen, X., and W. D. Nordhaus. 2011. “Using Luminosity Data as a Proxy for Economic Statistics.” Proceedings of the National Academy of Sciences 108 (21): 8589–8594. https://doi.org/10.1073/pnas.1017031108.
- Chen, Z. Q., Y. Wei, K. F. Shi, Z. Y. Zhao, C. X. Wang, B. Wu, B. W. Qiu, and B. L. Yu. 2022. “The Potential of Nighttime Light Remote Sensing Data to Evaluate the Development of Digital Economy: A Case Study of China at the City Level.” Computers, Environment and Urban Systems 92: 101749. https://doi.org/10.1016/j.compenvurbsys.2021.101749.
- Diaz-Sarachaga, J. M., D. Jato-Espino, and D. Castro-Fresno. 2018. “Is the Sustainable Development Goals (SDG) Index an Adequate Framework to Measure the Progress of the 2030 Agenda?” Sustainable Development 26 (6): 663–671. https://doi.org/10.1002/sd.1735.
- Elvidge, C. D., K. Baugh, M. Zhizhin, F. C. Hsu, and T. Ghosh. 2017. “VIIRS Night-Time Lights.” International Journal of Remote Sensing 38 (21): 5860–5879. https://doi.org/10.1080/01431161.2017.1342050.
- Elvidge, C. D., P. C. Sutton, T. Ghosh, B. T. Tuttle, K. E. Baugh, B. Bhaduri, and E. Bright. 2009. “A Global Poverty Map Derived from Satellite Data.” Computers & Geosciences 35 (8): 1652–1660. https://doi.org/10.1016/j.cageo.2009.01.009.
- Elvidge, C. D., M. Zhizhin, T. Ghosh, F. C. Hsu, and J. Taneja. 2021. “Annual Time Series of Global VIIRS Nighttime Lights Derived from Monthly Averages: 2012 to 2019.” Remote Sensing 13 (5): 922. https://doi.org/10.3390/rs13050922.
- Filho, W. L., V. O. Lovren, M. Will, A. L. Salvia, and F. Frankenberger. 2021. “Poverty: A Central Barrier to the Implementation of the UN Sustainable Development Goals.” Environmental Science & Policy 125: 96–104. https://doi.org/10.1016/j.envsci.2021.08.020.
- Filmer, D., and K. Scott. 2012. “Assessing Asset Indices.” Demography 49 (1): 359–392. https://doi.org/10.1007/s13524-011-0077-5.
- Gibson, J., S. Olivia, and G. Boe-Gibson. 2020. “Night Lights in Economics: Sources and Uses.” Journal of Economic Surveys 34 (5): 955–980. https://doi.org/10.1111/joes.12387.
- Goswami, S., and A. Chakrabarti. 2012. “Quartile Clustering: A Quartile Based Technique for Generating Meaningful Clusters.” arXiv preprint arXiv:1203.4157.
- Gu, Q. H., J. N. Tian, X. X. Li, and S. Jiang. 2022. “A Novel Random Forest Integrated Model for Imbalanced Data Classification Problem.” Knowledge-Based Systems 250: 109050. https://doi.org/10.1016/j.knosys.2022.109050.
- Halisçelik, E., and M. A. Soytas. 2019. “Sustainable Development from Millennium 2015 to Sustainable Development Goals 2030.” Sustainable Development 27 (4): 545–572. https://doi.org/10.1002/sd.1921.
- Haveman, R., and E. N. Wolff. 2004. “The Concept and Measurement of Asset Poverty: Levels, Trends and Composition for the U.S.,1983–2001.” The Journal of Economic Inequality 2: 145–169. https://doi.org/10.1007/s10888-004-4387-3.
- Hu, S., Y. Ge, M. X. Liu, Z. P. Ren, and X. N. Zhang. 2022. “Village-Level Poverty Identification Using Machine Learning, High-Resolution Images, and Geospatial Data.” International Journal of Applied Earth Observation and Geoinformation 107: 102694. https://doi.org/10.1016/j.jag.2022.102694.
- Jean, N., M. Burke, M. Xie, W. M. Davis, D. B. Lobell, and S. Ermon. 2016. “Combining Satellite Imagery and Machine Learning to Predict Poverty.” Science 353: 790–794. https://doi.org/10.1126/science.aaf7894.
- Kyba, C. C. M., T. Kuester, A. S. D. Miguel, K. Baugh, A. Jechow, F. Hölker, J. Bennie, C. D. Elvidge, K. J. Gaston, and L. Guanter. 2017. “Artificially Lit Surface of Earth at Night Increasing in Radiance and Extent.” Science Advances 3: e1701528. https://doi.org/10.1126/sciadv.1701528.
- Lee, K., and J. Braithwaite. 2022. “High-resolution Poverty Maps in Sub-Saharan Africa.” World Development 159: 106028. https://doi.org/10.1016/j.worlddev.2022.106028.
- Levin, N., C. C. M. Kyba, Q. L. Zhang, A. S. d. Miguel, M. O. Román, X. Li, B. A. Portnov, et al. 2020. “Remote Sensing of Night Lights: A Review and an Outlook for the Future.” Remote Sensing of Environment 237: 111443. https://doi.org/10.1016/j.rse.2019.111443.
- Lin, Y., T. H. Zhang, X. Q. Liu, J. Yu, J. Li, and K. Gao. 2022. “Dynamic Monitoring and Modeling of the Growth-Poverty-Inequality Trilemma in the Nile River Basin with Consistent Night-Time Data (2000–2020).” International Journal of Applied Earth Observation and Geoinformation 112: 102903. https://doi.org/10.1016/j.jag.2022.102903.
- Marconcini, M., A. Metz-Marconcini, S. Ureyen, D. Palacios-Lopez, W. Hanke, F. Bachofer, J. Zeidler, et al. 2020. “Outlining Where Humans Live, the World Settlement Footprint 2015.” Scientific Data 7 (1): 242. https://doi.org/10.1038/s41597-020-00580-5.
- Matsuki, K., V. Kuperman, and J. A. Van Dyke. 2016. “The Random Forests Statistical Technique: An Examination of Its Value for the Study of Reading.” Scientific Studies of Reading 20 (1): 20–33. https://doi.org/10.1080/10888438.2015.1107073.
- McCallum, I., C. C. M. Kyba, J. C. L. Bayas, E. Moltchanova, M. Cooper, J. C. Cuaresma, S. Pachauri, et al. 2022. “Estimating Global Economic Well-Being with Unlit Settlements.” Nature Communications 13 (1): 2459. https://doi.org/10.1038/s41467-022-30099-9.
- Mellander, C., J. Lobo, K. Stolarick, and Z. Matheson. 2015. “Night-Time Light Data: A Good Proxy Measure for Economic Activity?” PLoS One 10 (10): e0139779. https://doi.org/10.1371/journal.pone.0139779.
- Miller, S. D., S. P. Mills, C. D. Elvidge, D. T. Lindsey, T. F. Lee, and J. D. Hawkins. 2012. “Suomi Satellite Brings to Light a Unique Frontier of Nighttime Environmental Sensing Capabilities.” Proceedings of the National Academy of Sciences 109 (39): 15706–15711. https://doi.org/10.1073/pnas.1207034109.
- Niu, T., Y. M. Chen, and Y. Yuan. 2020. “Measuring Urban Poverty Using Multi-Source Data and a Random Forest Algorithm: A Case Study in Guangzhou.” Sustainable Cities and Society 54: 102014. https://doi.org/10.1016/j.scs.2020.102014.
- Rutstein, S. O., and K. Johnson. 2004. The DHS Wealth Index. DHS Comparative Reports NO. 6. Calverton, USA: ORC Macro. https://dhsprogram.com/pubs/pdf/CR6/CR6.pdf.
- Rutstein, S. O., and S. Staveteig. 2014. Making the Demographic and Health Surveys Wealth Index Comparable. DHS Methodological Reports NO. 9. Rockville, USA: ICF International. https://iussp.org/sites/default/files/event_call_for_papers/ComparativeWealth-DRAFT-IUSSP.pdf.
- Sahn, D. E., and D. Stifel. 2003. “Exploring Alternative Measures of Welfare in the Absence of Expenditure Data.” Review of Income and Wealth 49 (4): 463–489. https://doi.org/10.1111/j.0034-6586.2003.00100.x.
- Schmidt-Traub, G., C. Kroll, K. Teksoz, D. Durand-Delacre, and J. D. Sachs. 2017. “National Baselines for the Sustainable Development Goals Assessed in the SDG Index and Dashboards.” Nature Geoscience 10 (8): 547–555. https://doi.org/10.1038/ngeo2985.
- Shao, Z. X., and X. Li. 2023. “Multi-scale Estimation of Poverty Rate Using Night-Time Light Imagery.” International Journal of Applied Earth Observation and Geoinformation 121: 103375. https://doi.org/10.1016/j.jag.2023.103375.
- Shi, K. F., Z. J. Chang, Z. Q. Chen, J. P. Wu, and B. L. Yu. 2020. “Identifying and Evaluating Poverty Using Multisource Remote Sensing and Point of Interest (POI) Data: A Case Study of Chongqing, China.” Journal of Cleaner Production 255: 120245. https://doi.org/10.1016/j.jclepro.2020.120245.
- Smith, B., and S. Wills. 2018. “Left in the Dark? Oil and Rural Poverty.” Journal of the Association of Environmental and Resource Economists 5 (4): 865–904. https://doi.org/10.1086/698512.
- Smits, J., and R. Steendijk. 2015. “The International Wealth Index (IWI).” Social Indicators Research 122 (1): 65–85. https://doi.org/10.1007/s11205-014-0683-x.
- Stokes, E. C., M. O. Romn, Z. S. Wang, C. C. M. Kyba, S. D. Miller, T. Storch, and K. R. Gurney. 2021. “Retired Satellites: A Chance to Shed Light.” Science 373: 1451–1452. https://doi.org/10.1126/science.abl9965.
- Weidmann, N. B., and S. Schutte. 2017. “Using Night Light Emissions for the Prediction of Local Wealth.” Journal of Peace Research 54 (2): 125–140. https://doi.org/10.1177/0022343316630359.
- Xu, J. B., J. Song, B. C. Li, D. Liu, and X. S. Cao. 2021. “Combining Night Time Lights in Prediction of Poverty Incidence at the County Level.” Applied Geography 135: 102552. https://doi.org/10.1016/j.apgeog.2021.102552.
- Yeh, C., A. Perez, A. Driscoll, G. Azzari, Z. Tang, D. Lobell, S. Ermon, and M. Burke. 2020. “Using Publicly Available Satellite Imagery and Deep Learning to Understand Economic Well-Being in Africa.” Nature Communications 11 (1): 2583. https://doi.org/10.1038/s41467-020-16185-w.