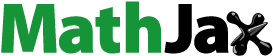
ABSTRACT
Due to the Sustainable Development Goals (SDGs) being designed at both national and globally applicable level, it is challenging to adequately reflect the local context and characteristics in different urban regions without fully utilizing big earth data. To effectively address this issue, this study localized 73 urban indicators for 13 SDGs and both assessed and forecasted urban sustainability across 18 cities in Hainan (2010-2030) using big earth data. Our analysis specifically focused on indicator score, goal score, SDG index, SDG spatial spillover effect, and trade-offs and synergies. The results indicated an overall upward trend in sustainable development in Hainan province, predicting achievement of the SDGs by 2030. The SDG index and spatial spillover showed a pattern of ‘high in the north and south, low in the middle’. While synergies outweighed trade-offs, trade-offs increased at a faster pace. More specifically, the average SDG index increased from 29.85 in 2010–60.09 in 2018, with a projected score of 89.76 by 2030. During 2010–2018, the synergy-to-trade-off ratio declined from 3.91–1.84, driven by a trade-off growth rate 2.03 times higher than synergy. Our work provides a valuable localized case method, and data support for monitoring sustainable development at the global urban level.
1. Introduction
Currently, global urbanization constantly continues to progress, with the United Nations (UN) predicting that global urbanization will increase from 56% in 2021–68% in 2050 (UN Citation2022). Urbanization is intertwined with numerous critical global sustainability challenges experienced over the past 50 years, including climate change, rising inequality, urban flooding, traffic congestion, slums, inadequate infrastructure, resource scarcity, environmental pollution, and others (Guo et al. Citation2021; Guo et al. Citation2022). To monitor the global sustainable development issues, the UN unveiled the 17 Sustainable Development Goals (SDGs) in 2015 across the social, economic, and environmental dimensions of sustainable development (UN Citation2023). Notably, SDG 11 serves as the foremost goal for monitoring urban sustainable development, aiming to ‘Make cities and human settlements inclusive, safe, resilient, and sustainable’. Nonetheless, all other SDGs will also need to be met in the world’s cities (Sustainable Development Solutions Network Citation2016). Additionally, SDG 11 is intricately interconnected with a minimum of 11 other goals, including SDG1, SDG3, SDG4, SDG6, SDG7, SDG8, SDG9, SDG12, SDG13, SDG16, SDG17 (Sustainable Development Solutions Network Citation2015). Nearly one-third of the 244 indicators associated with the SDGs can be evaluated on an urban scale (United Nations Citation2015; Citation2018). Subsequently, the UN has introduced more comprehensive monitoring frameworks exclusively for cities, known as the Global Urban Monitoring Framework (UMF) and New Urban Agenda (NUA), based on the SDGs.
In 2016, the UN endorsed NUA, offering a comprehensive urban framework that encourages cities to incorporate all facets of sustainable development (Satterthwaite Citation2016). In 2022, the UN introduced UMF, featuring 78 indicators spanning across social, economic, environmental, cultural, governance, and implementation dimensions. This framework aims to expedite the realization of SDGs and NUA goals at the urban level by evaluating the existing urban monitoring indicator frameworks (Guzmán, Pereira Roders, and Colenbrander Citation2017). However, the above-mentioned urban monitoring frameworks are designed at national and international levels and may not adequately reflect the local context and characteristics in different regions. Additionally, the achievement of SDGs will depend on the ability to realize SDGs in cities and regions (UN-Habitat Citation2014). Hence, it is essential to establish a localized urban SDG framework and enhance comprehensive localized monitoring at the city level.
Localization refers to the process of adapting, implementing, and monitoring the SDGs at a local level (UN-Habitat Citation2014). SDG localization can be initiated by a range of different actors, including national government, local government, civil society and academia (Sustainable Development Solutions Network Citation2016). In 2017, half of the municipalities in the Netherlands participated in the Global Goals Municipal Campaign to promote the alignment of their policies with SDGs (Global Taskforce of Local and Regional Governments Citation2016). The South African National Ministry has initiated a comprehensive review of the regulatory framework for human settlements in accordance with SDG 11 (Global Taskforce of Local and Regional Governments Citation2016). The National Confederation of Municipalities of Brazil is engaging in collaboration to reinforce the role of municipal authorities in the localization of SDGs (Global Taskforce of Local and Regional Governments Citation2016). Although many cities globally have a solid foundation for implementing SDGs, various challenges such as poor data availability continue to hinder the promotion of localized monitoring for urban sustainable development (Sean Citation2023).
Presently, big earth data offers a robust solution for enabling global cross-domain and interdisciplinary collaboration, effectively mitigating challenges such as disparities in statistical data resolution, data decomposition complexities, inconsistent statistical methodologies, and incomplete statistical information (Guo Citation2021; Guo et al. Citation2017; Guo et al. Citation2020). Organizations such as the Committee on Earth Observation Satellites and the Group on Earth Observations have extensively harnessed big earth data technologies and methods to bolster support for the SDGs (Anderson et al. Citation2017; Kavvada et al. Citation2020; Paganini et al. Citation2018). Currently, big earth data widely employed in SDGs research primarily encompass satellite remote sensing data, sensor network data, trajectory data, socio-economic statistical data, opinions and behavior data, transaction data, and survey data, among other sources (Ferreira, Iten, and Silva Citation2020). The SDG Global Database from the UN Statistics Division provides data on 171 out of 232 indicators for a total of 247 countries and areas worldwide between the years from 1990 to 2019 (United Nations Citationn.d.). Based on big earth data, numerous works have been conducted from different perspectives around the SDGs.
In recent years, an increasing number of works in the literature have undertaken assessments on urban SDGs including the single-goal evaluation (Ferguson and Roofe Citation2020; Gulseven and Ahmed Citation2022; McDonald, Marois, and Spronk Citation2021; Sadoff, Borgomeo, and Uhlenbrook Citation2020; Song et al. Citation2023) and multi-goals evaluation (Allen et al. Citation2020; Sinha, Sengupta, and Alvarado Citation2020; Xiao et al. Citation2022; Xiao et al. Citation2023; Zhou et al. Citation2022). For single-goal assessments, such as SDG 11.1, machine learning algorithms and the use of remote sensing big data have enabled the estimation of poverty in urban areas (Jean et al. Citation2016). One of the crucial indicators for measuring urban sustainable development is the proportion of slums/informal settlements to the total population (Simon et al. Citation2016). For SDG 11.3.1, a global land use efficiency trend assessment has been conducted by calculating the ratio between the land consumption rate (LCR) and population growth rate (PGR) for global megacities (Jiang et al. Citation2021). To comprehensively conduct urban monitoring of multi-goals, the Sustainable Development Solution Network carried out a thorough assessment of urban sustainability for 45 European capital cities and 105 major U.S. cities (Lafortune et al. Citation2019; Lynch, LoPresti, and Fox Citation2019). Using causal diagnosis and network analysis methods, the comprehensive analysis of 1302 networks of SDGs across 31 provinces in China from 2000 to 2020 was performed (Cao et al. Citation2023). Based on a comprehensive assessment of SDGs revealing global imbalances, a deep understanding of the interrelationships between the goals is currently a research hotspot.
The focus of the inter-goals correlation analysis primarily centers on trade-offs and synergies(Bandari et al. Citation2022; Bisaga et al. Citation2021; Gao et al. Citation2021; Mainali et al. Citation2018; Pradhan et al. Citation2017). For example, network analysis was employed to model the interconnections between SDGs (Swain and Ranganathan Citation2021). Global time series data of SDGs indicators from countries with various income levels were used to conduct SDGs interaction networks (Lusseau and Mancini Citation2019). The interactions among SDGs were then scrutinized based on population, location, income, and regional groupings (Warchold, Pradhan, and Kropp Citation2021). Non-linear relationships between SDGs and climate change were explored using World Bank data for 181 countries from 2000 to 2019, categorized by region, development, and income levels (Laumann et al. Citation2022). Additionally, a data-driven classification of SDGs was conducted for 166 countries globally, investigating the interactions among the goals (Wu et al. Citation2023).
Nonetheless, the majority of the aforementioned assessments predominantly utilize national-level data for conducting evaluations at a national level, with fewer localized assessments at the municipal scale. This is primarily attributed to the challenges of gathering urban-related statistical data at smaller scales, which limits the spatial and temporal resolutions, and raises difficulties in disaggregating data to align with specific spatial dimensions. Along these lines, in this work, the localized urban SDGs for the period 2010–2018 across 18 cities in Hainan province were monitored and comprehensively assessed. First, a localized urban SDGs assessment framework is established. Second, the urban sustainable development status of these 18 cities is quantitatively evaluated using big earth data. The analysis covers five key aspects: monitoring of urban sustainable development types and stages; evaluating the three-dimensional sustainability status encompassing social, economic, and environmental dimensions; monitoring of SDG index scores and 2030 forecasts; analysing the impact of sustainable development spillover; quantitative analysing the goal trade-offs and synergies. Our work delivers valauble localized monitoring frameworks, data, and methodologies to support the monitoring and assessment of global urban SDGs.
2. Study area and dataset
2.1. Study area
Hainan province is situated at the southernmost geographical extremity of China, encompassing a total land area of 35,400 km2, along with an extensive sea territory spanning approximately 2 million km2. Being the southernmost province in China, Hainan possesses a highly favorable ecological environment and a wealth of natural resources, encompassing a comprehensive spectrum of factors that are pivotal for the pursuit of sustainable development, both on terrestrial and marine fronts (Ding et al. Citation2024). This research focused on the region encompassing 18 cities in Hainan province, with the except for Sansha city. provides an illustrative overview map of Hainan province.
The establishment of the Hainan Free Trade Port in 2018 has created both opportunities and challenges to the sustainable development in Hainan province. While the free trade port has stimulated continuous economic growth, it has concurrently imposed environmental burdens. The latter effect is particularly evident in the thriving tourism industry causing issues like overdevelopment, land use conflicts, and waste management problems. Addressing these challenges necessitates stringent protection of the marine ecosystem and the establishment of a comprehensive land-sea integrated ecosystem protection and restoration mechanism. Furthermore, the evolving demands accompanying the free trade port's development pose challenges in ensuring energy supply and promoting the utilization of renewable energy sources. Hence, there is a pressing demand for the localized monitoring and evaluation of sustainable development in Hainan province (People's Government of Hainan province Citation2020).
2.2. Dataset
In this work, a variety of big earth data sources covering the period from 2010 to 2018 were utilized for 18 cities in Hainan province. These encompassed 73 different datasets, including remote sensing data, statistical records, and ground-level observations. A total of 73 localized urban indicators related to sustainable development were covered across the social, economic, and environmental dimensions. Specific details can be found in Appendix A.
3. Methods
3.1. Construction of localized urban SDGs
In this work, a localized urban SDGs framework was constructed based on SDGs. Overall, the 10 fundamental principles of global monitoring indicators by the Sustainable Development Solutions Network were utilized (SDSN Citation2015). More specifically, limited in number and globally harmonized, simple single-variable indicators with straightforward policy implications were used, allowing for high-frequency monitoring, consensus-based in line with international standards and system-based information. These indicators were constructed from well-established data sources, disaggregated, universal, mainly outcome-focused, science-based, and forward-looking, serving as a proxy for broader issues or conditions. The process of indicator selection and localization was carried out in two steps. In the initial phase of indicator selection, emphasis was placed on urban areas, selecting comprehensive indicators that encompass social, economic, and environmental dimensions. Specific to Hainan province, indicators incongruent with the local context were excluded. Subsequently, the process involves localizing indicators based on the accessibility of data and methodologies, excluding indicators characterized by inadequate data availability and methodological limitations.
In this work, localization was accomplished for the 13 SDGs, namely SDG1, SDG2, SDG3, SDG4, SDG6, SDG7, SDG8, SDG9, SDG11, SDG13, SDG14, SDG15, and SDG17. From these, a total of 73 indicators were derived, comprising 35 in the social dimension, 13 in the economic dimension, and 25 in the environmental dimension. The assessment methods for each indicator are detailed in Appendix A. Owing to inadequate timeliness and accessibility of data, impeding the support for effective analysis, as well as concerns regarding indicator redundancy, SDG5, SDG10, SDG12, and SDG16 were excluded from the selection.
In this work, all indicators underwent threshold selection and normalization based on the upper and lower bounds decision tree proposed in the United Nations Sustainable Development Report 2023. Different principles were applied in the scoring process to determine the bounds and normalization for various indicators, including positive, negative, neutral, textual indicators, among others. The decision tree comprises specific decision-making methods such as absolute quantitative thresholds, the average of the top five performers, progress percentage, and others (UN Citation2023).
Additionally, weights for each indicator were established. To ensure a balanced assessment across the social, economic, and environmental dimensions, equal weights were assigned to these three dimensions. The weight for indicator is detailed in formula (1).
(1)
(1) where, n is the number of indicators within a specific dimension,
denotes the weight of indicator i within that dimension.
3.2. Assessment methodology of localized urban SDGs
Normalization of Indicator Scores
In this work, the score range for indicators was established as 0–100, where 0 symbolizes the lowest level of sustainable development, and 100 signifies the highest attainable level. When a higher score signifies a greater degree of sustainability, the normalization is carried out using Equationequation (2(2)
(2) ). Conversely, if a lower score indicates a higher level of sustainability, normalization is performed using Equationequation (3
(3)
(3) ) (UN Citation2023).
(2)
(2)
(3)
(3) where,
is the observation score of indicator j under goal i, with outliers removed.
represent the minimum and maximum values of the variable x, respectively.
or
is the normalized scores of the indicator j.
(2) | Calculation of Goal Scores, Goal Status Index, Growth Rates, and Quantification of Trade-offs and Synergies |
Goals Scores can be formulated as follows:
(4)
(4) where
is the score of goal k,
stands for the number of indicators under the goal k,
and
are the score and weight of indicator i under the goal k, respectively.
The Goal Status Index, defined as the ratio of the SDG Goal score to the maximum SDG Goal score, can be formulated as follows:
(5)
(5) Growth Rates can be formulated as follows:
(6)
(6) where,
refers to the growth rates index of the indicator/goal over N years, while
and S represent the indicator/goal scores N years ago and in the current year, respectively.
Quantification of Trade-offs and Synergies:
Based on the goal scores, the Pearson correlation coefficient matrices for the 13 Goals in 18 cities from 2010 to 2018 were obtained. This allowed for an analysis of the trade-offs and synergies between the SDGs. The Pearson correlation coefficient, which falls between −1 and 1, with values closer to 0 indicating weaker correlations, is interpreted as [0,1] denoting synergies and [−1,0] representing trade-offs (Chicco Citation2021).
Global synergies and trade-offs:
Categorized by synergies and trade-offs, the sum of the absolute values of the Pearson correlation coefficient obtained for the 13 Goals provides a measure of Hainan province's global synergies and trade-offs.
Spatial Autocorrelation:
Spatial autocorrelation and its spatial distribution can be assessed using the Global Moran's I and local Moran's I indices (Bivand, Müller, and Reder Citation2009; Tiefelsdorf and Boots Citation1997). Global Moran's I evaluates overall spatial patterns for significance through metrics like the Moran's I index, z-score, and p-value, encompassing assessments of clustering, dispersion, and randomness. Local Moran's I evaluates four spatial autocorrelation patterns at specific local positions: High-High Cluster (where high values are clustered together), Low-Low Cluster (where low values cluster together), High-Low Outlier (where high values are surrounded by low values), and Low-High Outlier (where low values are surrounded by high values).
3.3. Calculation of SDG index
For various geographical or administrative units, the SDG index can be computed using a spatial aggregation index among indicators score:
(7)
(7) where
represents the SDG index for geographical unit
, while
denotes the number of goals for geographical unit
.
states the number of indicators under goal
. Additionally,
and
respectively stand for the value and weight of indicator k under goal
.
3.4. Prediction of the SDG index
The forecasting of the SDG Index is facilitated by the Autoregressive Integrated Moving Average model ARIMA(p, d, q), which encompasses autoregressive terms of order p, moving average terms of order q, and differencing of order d (Shumway et al. Citation2017). An ARIMA(p, d, q) model can be represented as follows:
(8)
(8) where,
is the observed value of the time series,
signifies the constant term,
are autoregressive coefficient representing the impact of past p observations on the current one,
denotes the white noise error term,
are moving average coefficients representing the influence of past
white noise terms on the current time, and d is the order of differencing.
To eliminate the trend in the original time series, first-order differencing was performed and d was set to 1. The selection of model parameters, specifically p = 1 and q = 0, was adjudicated based on the Bayesian Information Criterion (Neath and Cavanaugh Citation2012).
4. Results and analysis
4.1. Analysis of indicators scores
4.1.1. Analysis of urban sustainable development types and development phases
The indicator scores for the 18 cities in Hainan province were obtained and normalized over the period from 2010 to 2018. The findings suggest an overall improvement in the sustainable development status of these cities during this timeframe. The blue-low score areas have progressively diminished, while the red-high score areas have experienced a gradual increase (). In the years 2010-2011, there were widespread blue-low score regions spanning indicators 2.1.1.1–6.5.1.1 across all 18 cities. Except for Haikou, other cities showed blue-low score regions for indicators 8.10.1.1–11.2.1.1 and indicators 14.1.1.1–15.1.1.1 during the period from 2010 to 2018. Of all the indicator scores from 2010 to 2018, Haikou City exhibited a more evenly distributed and notably prominent yellow-orange high score area, indicating its superior sustainable development status among all the cities.
Figure 2. Heatmap of Indicator Scores for 18 Cities in Hainan province from 2010 to 2018, where each heatmap consists of 73 circles. From inner to outer, each colored circle represents the score of indicator1, … , indicator n, up to indicator 73.

For the quantitative assessment of Hainan province's SDG indicator development status, the indicators scores were classified into five levels: Level 1 [100, 85], Level 2 (85, 60], Level 3 (60, 30], Level 4 (30, 0], and Level 5 for no data (). The results revealed an overall upward trend in indicator scores in Hainan province from 2010 to 2018. Notably, the proportion of indicators scoring above 85 points witnessed a significant increase, rising from 16.97% in 2010–51.67% in 2018. Concurrently, the percentage of no data indicators decreased from 58.30% in 2010–6.39% in 2018.
Figure 3. Spatial Distribution Map depicting the Percentage Distribution of Indicators at Different Levels among the 18 Cities in Hainan province during the period from 2010 to 2018.

During the period spanning 2010–2014, none of the 18 cities exhibited a prevalence of Level 1 indicators exceeding 50%. However, 2015 marked a pivotal juncture as two cities, namely, Sanya (53.42%) and Haikou (52.05%), surpassed the 50% threshold for Level 1 indicators. By 2018, Sanya had broken the 60% threshold with a percentage of 61.64%, and 13 cities had more than 50% of Level 1 indicators. After nine years of development, in 2018, over 72.22% of cities within Hainan province had achieved excellence in more than half of their SDG indicators.
To mitigate the impact of low scores and no data, an analysis of the urban sustainable development types and phases for the 18 cities in Hainan province was conducted based on the year-on-year growth rates of Level 1 indicators’ percentage from 2011 to 2018. The growth rates falling within the range of [0.45,1] were classified as high-speed (H), those within [0.25,0.45) as medium-speed (M), and those within [0.20,0.25) as low-speed (L). The 18 cities demonstrated three sustainable development types: High-Medium-Low-speed (HML), referred to as high-speed, Low-High-Low-speed (LHL), referred to as low-speed, and Medium-High-Low-speed (MHL), referred to as medium-speed (). For cities of the HML type, 2010–2011 is defined as H, 2012–2015 as M, and 2015–2018 as L. For cities of the MHL type, 2010 is defined as M, 2011 as H, and 2012–2018 as L. For cities of the LHL type, 2010 is defined as L, 2012 as H, and 2012–2018 as L.
Figure 4. Proportional Growth Rates of Level1 Indicators and Spatial Distribution of Urban Sustainable Development Types for 18 Cities in Hainan province from 2011 to 2018.

The findings indicate that Hainan province is predominantly characterized by high-speed cities, constituting 44.44% of the total, while low-speed and medium-speed cities each make up 27.78%. High-speed cities went through a phase of rapid development from 2010 to 2011, followed by a deceleration period from 2011 to 2013. Conversely, low-speed and medium-speed cities experienced rapid development from 2010 to 2012, followed by a deceleration phase from 2012 to 2013. Subsequently, all three city types entered a stable phase from 2013 to 2018.
High-speed cities are primarily located in both the northern and southern regions of Hainan province, whereas low-speed cities are concentrated in the northern part, and medium-speed cities are distributed in the eastern and western regions. High-speed cities include Haikou, Sanya, Wuzhishan, Wanning, Tunchang, Chengmai, Ledong, and Lingshui. Medium-speed cities consist of Qionghai, Anding, Dongfang, Qiongzhong, and Baoting. Low-speed cities encompass Wenchang, Lingao, Danzhou, Baisha, and Changjiang.
4.1.2. A graded assessment of sustainable development across three dimensions: socioeconomic, economic, and environmental
To monitor and evaluate the sustainable development within the three dimensions of socioeconomic, economic, and environmental in Hainan province from 2010 to 2018, a statistical analysis was carried out to examine the changes in the percentage of indicators falling into levels 1–5 within these dimensions ().
Figure 5. Grading of Three-Dimensional Indicators Scores in the Socioeconomic, Economic, and Environmental Dimensions for Hainan province in the Years 2010 and 2018. L1-L5 represent the different levels of indicators within these three dimensions, ranging from level 1 to level 5.

Among the indicators that achieved a score of 85 or higher, in 2010, the proportion of the environmental dimension was 3.72 times greater than that of the economic dimension and 6.24 times greater than that of the social dimension. However, in 2018, this relationship shifted, with the social dimension having a proportion 1.18 times greater than that of the environmental dimension and 5.12 times greater than that of the economic dimension. It's important to notice that the social dimension exhibited an imbalance, as it had the highest proportion of indicators with the lowest scores and missing data.
Particularly, within the social dimension, Level 1 indicators increased from 3.97% (2010) to 52.86% (2018), reflecting a growth rate of 44.75%. In contrast, Level 5 indicators decreased from 75.24% (2010) to 6.03% (2018), with a decline rate of 30.27%. Within the environmental dimension, Level 1 indicators rose from 34.67% (2010) to 62.67% (2018), representing a growth rate of 8.83%. Conversely, Level 5 indicators decreased from 52.00% (2010) to 4.22% (2018), indicating a decline rate of 30.15%. In the economic dimension, Level 1 indicators increased from 17.95% (2010) to 27.78% (2018), with a growth rate of 6.44%, while Level 5 indicators decreased from 24.79% (2010) to 13.68% (2018), resulting in a decline rate of 8.14%. Overall, over the 9-year period, the social dimension exhibited the most significant sustainable development status, with a growth rate 5.07 times that of the environmental dimension and 6.95 times that of the economic dimension. Notably, the economic dimension showed the slowest decrease in indicators with no data, at a rate of 0.27 times that of the social and environmental dimensions.
4.2. Analysis of goals scores
4.2.1. Sustainable development status of goals
The goals-weighted scores for the period of 2010–2018 were calculated through the summation of weighted indicators scores. This allowed for an analysis of the status of sustainable development goals among the 18 cities in 2018. Additionally, an evaluation of the distance to achieving the ‘targets of the 2030 Agenda for Sustainable Development’ was further assessed based on the goal status index ().
Figure 6. Overview of Goals Status for 18 Cities in Hainan province in 2018. A: Haikou, B: Sanya, C: Wuzhishan, D: Wenchang, E: Qionghai, F: Wanning, G: Anding, H: Tunchang, I: Chengmai, J: Lingao, K: Danzhou, L: Dongfang, M: Ledong, N: Qiongzhong, O: Baoting, P: Lingshui, Q: Baisha, R: Changjiang.

Regarding the sustainable development status in 2018, on average, each of the 18 cities had achieved ‘ fully achieved + basic achievement’ in 4.89 goals. Based on the number of goals achieved in this state, these cities can be categorized into four tiers: Tier1 includes Sanya, which has achieved 7 goals; Tier2 comprises Haikou, Qionghai, Ledong, Lingshui, and Baisha, all of which has achieved 6 goals; Tier3 consists of Wuzhishan, Wenchang, Anding, Lingao, Danzhou, Baoting, and Changjiang, each of which had achieved 5 goals; Tier4 encompasses Wanning, Chengmai, Dongfang, and Qiongzhong, all of which had achieved 4 goals.
From the perspective of goals development status, in 2018, SDG7 demonstrated the most outstanding performance. Conversely, SDG9 and SDG17 exhibited the weakest performance. Notably, SDG14, SDG15, and SDG17 encountered serious issues related to data availability.
4.2.2. Sustainable development potential areas of goals
Additionally, for the monitoring of spatial development potential zones for each Goal, the spatial autocorrelation of the goal growth rate for each city was extracted using the Global Moran's I index (). By examining the values of Moran's Index, z-score, and p-value, it can be inferred that the highest positive spatial autocorrelation was demonstrated in SDG1, SDG6, and SDG13 among the 13 Goals. This suggests their considerable development potential in spatial terms. The spatial patterns of other goals do not exhibit a significant difference compared to random patterns.
Table 1. Global Moran’s I Index for Sustainable Development Goals.
Furthermore, spatial autocorrelation mapping for SDG1, SDG6, and SDG13 were conducted based on Anselin Local Moran’s I, and the spatial clustering patterns within each goal were analysed (). During the period spanning from 2010 to 2018, various SDGs displayed distinct spatial autocorrelation patterns. Notably, SDG1 in Baoting and Sanya, as well as SDG13 in Wuzhishan, Baoting, and Lingshui exhibited significant High-High Clusters, indicating these regions had a high development potential. Conversely, SDG6 in Wenchang revealed a noteworthy Low-Low Cluster, suggesting a lower development potential in this specific region. Moreover, SDG6 in Ledong displayed notable High-Low Outliers, suggesting that these regions had siphon effects.
4.3. Quantitative analysis of trade-offs and synergies between the SDGs
To analyse the dynamic changes in the synergies and trade-offs of sustainable development Goals in Hainan province, Pearson correlation coefficients were calculated between the scores of the 13 Goals for the 18 cities from 2010 to 2018, and significance tests were conducted ().
The results indicate that in 2018, compared to 2010, both synergies and trade-offs became more significant. More specifically, in 2018, the average synergy of goals increased by 1.99%, while the average trade-off of goals grew by 1.44%. In 2010, there were 20 pairs of synergy goals with an average synergy correlation coefficient of 0.27, as well as 8 pairs of trade-off goals with an average trade-off correlation coefficient of −0.19. In contrast, by 2018, the number of synergy goals pairs had risen to 44, exhibiting an average synergy correlation coefficient of 0.31, while the number of trade-off indicator pairs reached 33, with an average trade-off correlation coefficient of −0.21.
Subsequently, based on the global trade-offs and synergies of the goals, a quantitative analysis of the overall correlation changes in Hainan province's SDGs was conducted (). In 2010, there were 8 goals showing trade-offs and synergies, including SDG1, 3, 7, 8, 9, 11, 13, and 17. By 2018, all 13 indicators exhibited trade-offs and synergies. In 2018, the goals that experienced an increase in global synergies and a decrease in trade-offs were SDG1 and SDG7, while SDG11 and SDG13 showed a decrease in global synergies and an increase in trade-offs. For the remaining goals, both global synergies and trade-offs increased. The top three goals in terms of global synergies in 2018 were SDG3 (3.23), SDG8 (3.12), and SDG1 (2.89), while the top three goals in terms of global trade-offs were SDG6 (2.13), SDG5 (1.78), SDG8 (1.42).
Based on the cumulative values of global synergy and balance across all goals, it can be observed that the SDG in Hainan province displayed a global synergy index of 11.45 in 2010, alongside a global trade-off index of 2.93. However, by 2018, the global synergy index had significantly increased to 26.09, accompanied by a rise in the global trade-off index to 14.22. Over the period from 2010 to 2018, the growth rate of global synergy for Hainan province's SDGs amounted to 12.49%, while the growth rate of global trade-off reached 25.30%. In summary, during the 2010–2018 timeframe, the SDG in Hainan province displayed a notable prevalence of synergy over trade-off. More specifically, in 2010, synergy was 3.91 times greater than the trade-off, and by 2018, synergy remained dominant, although at 1.84 times the trade-off. However, trade-off grew at a rate 2.03 times that of synergy during this period.
4.4. Spatial spillover effect of SDGs
The spatial clustering patterns of SDG growth rates can lead to the emergence of spatial spillover effects in sustainable development. In 2018, most of the 18 cities in Hainan province were in an accelerated development state for SDG3, a rapid development state for SDG4, a stagnation state for SDG7, and a decline state for SDG8, SDG9, SDG15, and SDG17. The remaining goals exhibited a moderate development status ().
Figure 10. Growth rates of goals Scores for 18 cities in Hainan province from 2010 to 2018, green represents growth, while red indicates regression. Growth rates exceeding 0.5 signify an accelerated development status, growth rates ranging from 0.2–0.5 represent a rapid development status, growth rates between 0 and 0.2 indicate a moderate development status, a growth rate of 0 indicates stagnation, and growth rates below 0 denote a declining status. A: Haikou, B: Sanya, C: Wuzhishan, D: Wenchang, E: Qionghai, F: Wanning, G: Anding, H: Tunchang, I: Chengmai, J: Lingao, K: Danzhou, L: Dongfang, M: Ledong, N: Qiongzhong, O: Baoting, P: Lingshui, Q: Baisha, R: Changjiang.

Furthermore, the Pearson correlation coefficient matrix for the growth rates of the 13 goals was extracted, and a spatial network of SDGs growth rate correlations was constructed to analysethe spatial spillover effects of sustainable development status among the 18 cities (). The edges in the spatial network represent the connectivity between cities, and the Pearson correlation coefficients of goals scores growth rates between cities are extracted as the edge weights. The color and thickness of the edges indicate the magnitude of these weights, with darker and thicker edges representing higher weights. The network nodes represent the 18 cities, and the weighted degree centrality of the nodes characterizes spatial spillover effects (Li et al. Citation2023; Zhang and Luo Citation2017). Nodes with higher weighted degree centrality have darker color and larger sizes, signifying their greater importance in the network and more significant spatial spillover effects on other cities.
The results indicate a spatial spillover effect in the growth rates of SDGs within Hainan province, characterized by a ‘high in the north and south, low in the middle’ pattern. Explicitly, among the 18 cities, the top five cities with the highest weighted degree of centrality are Lingao (16.19), Danzhou (16.03), Sanya (16.02), Wenchang (15.98), and Baoting (15.82). It's noteworthy that Sanya and Baoting are located in the southern region, while the others belong to the northern part of the province. On the other hand, the five cities with the lowest weighted degree centrality rankings are Tunchang (15.16), Wuzhishan (14.93), Changjiang (13.65), Qiongzhong (13.60), and Baisha (13.44), all situated in the central region.
4.5. Analysis and projection of SDG index
Through weighted composite evaluation, the SDGs index scores for 18 cities in Hainan province from 2010 to 2018 were calculated (). The results indicate that in 2010, the overall scores of the 18 cities were relatively low, ranging from 24 to 38, with an average score of 29.85. In 2011, the scores of the 18 cities ranged from 28 to 41, with an average of 32.48. The range for 2012 was 39–55, with an average of 44.93. In 2013, the range was 40–56, with an average of 44.57. For 2014, the range was 42–56, with an average of 48.97. In 2015, the 18 cities had scores ranging from 48 to 63, with an average of 54.15. Notably, in 2015, several cities achieved scores exceeding 60, with Haikou scoring 62.64. In 2016, the range was 47–64, with an average of 54.46. In 2017, the range was 51–64, with an average of 56.84, and in 2018, the range extended from 53 to 69, with an average of 60.09.
Over nine years, Hainan province's SDG index score followed a trend of ‘higher in the south and north, lower in the central region’, with sustainable development progressing more rapidly in the southern and northern areas compared to the central region.
In summary, Haikou, Sanya, Chengmai, Tunchang, and Changjiang exhibited the highest SDG index scores in the SDGs. Haikou City consistently performed the best in SDG completion, ranking first for eight out of the nine years. Sanya followed, ranking first for one year, second for five years, and third for one year. Chengmai came next, with three years of third-place rankings. Tunchang ranked second for one year and third for one year. Changjiang achieved third place for two years, and Ledong secured third place for one year.
Based on the year-on-year growth rates of the SDG index for the 18 cities in Hainan province from 2010 to 2018 (), it can be observed that over the 9-year period, the SDG index for all 18 cities showed an overall positive trend. The year 2012 exhibited the highest year-on-year growth rate, indicating that 2012 witnessed the most rapid progress in SDGs advancement among the 18 cities.
Conversely, negative growth was observed in the years 2010, 2013, 2014, 2016, and 2017. The most notable decline occurred in 2013, with 12 cities experiencing a decrease. These cities included Sanya, Wuzhishan, Wenchang, Qionghai, Wanning, Anding, Lingao, Dongfang, Qiongzhong, Baoting, Lingshui, and Baisha. Cities that faced negative growth on two occasions were Lingao, Chengmai, Wenchang, Danzhou, Dongfang, Wanning, and Baoting, while Baisha experienced three instances of negative growth.
Additionally, an ARIM model was employed to forecast SDGs index scores for the 18 cities from 2019 to 2030 based on their performance between 2010 and 2018 (). Overall, Hainan province's urban sustainable development exhibited a consistent upward trend from 2010 to 2030. The average scores for each city surged from 29.85 in 2010 to 60.09 in 2018, indicating a projected score of 89.76 by 2030. This trajectory implies that by 2030, Hainan province is poised to fulfill the objectives outlined in the 2030 Agenda for Sustainable Development and exhibit outstanding performance. Notably, a score of 85 or above is deemed excellent, while a score of 60 is considered satisfactory. The overall pattern of predicted SDG index for the 18 cities in Hainan province in 2030 reveals a trend of ‘higher scores along the coast, lower scores inland, higher scores in the southern and northern regions, and lower scores in the central region’. More specifically, Sanya is anticipated to achieve the highest score in the south, while Haikou is expected to lead in the north. In the central region, Baisha is forecasted to attain the lowest score.
The predicted average scores for high-speed, medium-speed, and low-speed cities were 88.71, 83.04, and 76.55, respectively. This suggests that high-speed cities exhibit greater potential and more notable achievements in the SDG process, followed by medium-speed cities, and finally, low-speed cities ( and ).
5. Discussion
Sustainable development requires a balance between the economic, social, and environmental dimensions (Doyle and Perez-Alaniz Citation2017; Wu et al. Citation2023; Zhong et al. Citation2021). of Hainan, with the highest proportion of indicators falling into the lowest score and no data categories. Additionally, improvements in monitoring and assessment methods led to a substantial reduction in no data for all indicators, from over half in 2010–6.39% in 2018, indicating success in addressing data issues. The latter effect took notably in social aspects like SDG1 (No Poverty), SDG2 (Zero Hunger), SDG3 (Good Health and Well-being), and SDG4 (Quality Education). In 2012 and 2014, many indicators with no data in these goals significantly improved. This change is linked to Hainan province's 12th Five-Years Plan (2011–2015), which prioritized people's livelihoods and social development. This led to synchronized income growth, better labourcompensation, and improved education and healthcare. Additionally, housing and medical insurance issues were addressed, resulting in enhanced social well-being (The People's Government of Hainan province Citation2011).
In 2018, on average, each of the 18 cities had 40% of their goals reaching the SDGs. SDG7 (Affordable and Clean Energy) demonstrated the most outstanding performance. Conversely, SDG9 (Industry, Innovation and Infrastructure) and SDG17 (Partnerships for the Goals) exhibited the weakest performance. Notably, SDG14 (Life Below Water), SDG15 (Life on Land), and SDG17 (Partnerships for the Goals) encountered serious issues related to data availability.
Hainan province's SDG index and 2030 projections showed a regional pattern: higher scores along the coast, and lower scores inland, with the north and south scoring higher and the middle region lower. Sanya in the south and Haikou in the north are expected to have the highest scores, while Baisha in the central area is projected to have the lowest. This pattern is affected by various factors, such as topography and industrial distribution. Hainan's land is relatively elevated in the middle and flat in the north and south. The province's land use planning emphasizes regional development, with the north focusing on high-tech and modern services, the south on tourism, and the central region primarily on agriculture and ecological conservation. Hainan province's 12th Five-Years Plan (2011–2015) and 13th Five-Years Plan (2016–2020) aimed to optimize the industrial structure, resulting in a shift in the composition of the three major industries. The proportion of the three industries changed from 26.1:27.7:46.2 in 2010–23.1:23.6:53.3 in 2015 (The People's Government of Hainan province Citation2016).
Over the period from 2010 to 2018, the ratio of synergy to trade-off decreased from 3.91 in 2010–1.84 in 2018. This was influenced by the fact that the growth rate of trade-off during this timeframe was 2.03 times that of synergy. This unveils dynamic shifts in the internal relationships of SDGs, underscoring the imperative for SDG transformations aligned with these changes. To achieve the optimal state of sustainable development, the government needs to formulate policies based on the specific correlations between the goals. It should provide more supportive policies for indicators with lower synergies, such as SDG3 (Good Health and Well-being), SDG8 (Decent Work and Economic Growth), and more balanced policies for indicators with higher trade-offs, such as SDG6 (Clean Water and Sanitation), SDG5 (Gender Equality). The combined consideration of trade-offs and synergies can facilitate a robust dynamic equilibrium, ultimately steering towards the optimal state of sustainable development.
Based on the SDG-driven developmental imbalances, governmental policies should foster essential transformations across sectors for sustainable development. Hainan province should specifically bolster policy support, investment, and implementation for underdeveloped SDG9 (Industry, Innovation, and Infrastructure) and SDG17 (Partnerships for the Goals). This entails promoting innovation and industry upgrades, enhancing infrastructure, fostering public-private partnerships, and establishing collaborative frameworks. Simultaneously, improving data accessibility for SDG14 (Life Below Water), SDG15 (Life on Land), and SDG17 (Partnerships for the Goals). It is crucial to reduce imbalances and provide enhanced policy support for the central region. Within the context of the central region, marked by lower SDG index scores and limited spatial spillover effects, it is imperative to place significant emphasis on bolstering the development of its central urban core. Furthermore, the ongoing endeavors to expedite the sequential transfer of industries should be sustained, thereby, fostering seamless interconnectivity in sustainable development across the northern, central, and southern regions, ultimately culminating in the establishment of an integrated and comprehensive developmental framework.
This study diverges from the previously reported works in the literature by focusing specifically on localized assessments at the urban level. In terms of indicator localization, a more comprehensive set of indicators was employed. From a data perspective, a larger and more comprehensive dataset was obtained by fully harnessing earth observation data, facilitating a more extensive evaluation. Before this study, surveillance and evaluation of sustainable development in Hainan province from diverse perspectives had been conducted by numerous works. Zhang C (Zhang et al. Citation2021) focused on the individual assessment of SDG11, while Liang (Liang et al. Citation2023) selected 11 SDGs with 23 indicators, and Zhang X (Zhang et al. Citation2023) chose 10 SDGs with 43 indicators for a comprehensive localized assessment. Liang and Zhang X primarily analysed SDG scores, with Zhang C incorporating spatial spillover analysis. This study localized 13 SDGs with a total of 73 indicators, conducting evaluations that encompassed not only SDG scores but also incorporated predictions for the year 2030. Additionally, a deeper analysis of spatial spillover effects was undertaken, with changes in trade-offs and synergies being quantified. The research findings of the three scholars align with the conclusions of this study, indicating an overall upward trend in sustainable development in Hainan. This trend reveals an imbalanced pattern characterized by ‘higher scores in the north and south, and lower scores in the central region’.
Owing to data constraints, the pool of indicators amenable to localization is restricted. In future investigations, our objective is to integrate a more diverse array of big earth data sources, encompassing surveys, census data, administrative records, geospatial imagery, and other forms of open data. This expansion aims to bolster the spatial resolution of data, enabling more nuanced spatial analyses across various scales. Moreover, the inclusion of additional indicators related to urban contexts is anticipated, further enhancing the capacity for localized assessments of urban Sustainable Development Goals across different scales.
6. Conclusions
This study localized 73 urban indicators across 13 SDGs, using big earth data to assess and predict urban sustainable development in 18 cities of Hainan province from 2010 to 2030. The analysis included scores for indicators, goals, and SDG index, along with the examination of spatial spillover effects, trade-offs, and synergies. The findings reveal a positive trajectory in the overall sustainable development of Hainan province, projecting SDGs achievement by 2030. The spatial distribution of the SDG index score and spillover effect exhibits a distinct pattern characterized by elevated levels in the northern and southern regions and lower levels in the central region.
More specifically, first, from 2010 to 2018, Haikou and Sanya achieved the highest SDG index scores. In 2018, SDG7 (Affordable and Clean Energy) exhibited exceptional performance in Hainan province, with SDG9 (Industry, Innovation and Infrastructure) and SDG17 (Partnerships for the Goals) demonstrating comparatively weaker performance. Second, the average SDG index of each city increased from 29.85 in 2010–60.09 in 2018, with a projected score of 89.76 by 2030, which indicates that Hainan will basically achieve the SDGs in 2030. Third, the growth rate of social dimension sustainability was 5.07 times for the environmental dimension and 6.95 times for the economic dimension. Fourth, due to the growth rate of d was 2.03 times for synergies from 2010 to 2018, the synergies in Hainan province were 3.91 times for trade-offs in 2010, which decreased to 1.84 times in 2018.
Our findings offer insightful localized monitoring frameworks, datasets, and methodologies that can be employed to facilitate the monitoring and evaluation of global urban SDGs.
Author contributions
Conceptualization Z.S.; methodology, S.L. and Z.S.; formal analysis, S.L.; writing – original draft preparation, S.L.; writing – review and editing, Z.S., Z.L., H.J., H.L. and X.O.; supervision, H.G.; funding acquisition, H.G. and Z.S. All authors have read and agreed to the published version of the manuscript.
Supplemental Material
Download MS Excel (25.8 KB)Acknowledgments
We appreciate the critical and constructive comments and suggestions from the reviewers that helped improve the quality of this manuscript.
Disclosure statement
No potential conflict of interest was reported by the author(s).
Data availability statement
The data presented in this study are available on request from the corresponding author.
Additional information
Funding
References
- Allen, Cameron, Michael Reid, John Thwaites, Rod Glover, and Tahl Kestin. 2020. “Assessing National Progress and Priorities for the Sustainable Development Goals (SDGs): Experience from Australia.” Sustainability Science 15: 521–538. https://doi.org/10.1007/s11625-019-00711-x.
- Anderson, Katherine, Barbara Ryan, William Sonntag, Argyro Kavvada, and Lawrence Friedl. 2017. “Earth Observation in Service of the 2030 Agenda for Sustainable Development.” Geo-spatial Information Science 20 (2): 77–96. https://doi.org/10.1080/10095020.2017.1333230.
- Bandari, Reihaneh, Enayat A Moallemi, Rebecca E Lester, David Downie, and Brett A Bryan. 2022. “Prioritising Sustainable Development Goals, Characterising Interactions, and Identifying Solutions for Local Sustainability.” Environmental Science & Policy 127: 325–336. https://doi.org/10.1016/j.envsci.2021.09.016.
- Bisaga, Iwona, Priti Parikh, Julia Tomei, and Long Seng To. 2021. “Mapping Synergies and Trade-Offs Between Energy and the Sustainable Development Goals: A Case Study of off-Grid Solar Energy in Rwanda.” Energy Policy 149: 112028. https://doi.org/10.1016/j.enpol.2020.112028.
- Bivand, Roger, Werner G Müller, and Markus Reder. 2009. “Power Calculations for Global and Local Moran’s.” Computational Statistics & Data Analysis 53 (8): 2859–2872. https://doi.org/10.1016/j.csda.2008.07.021.
- Cao, Min, Min Chen, Junze Zhang, Prajal Pradhan, Huadong Guo, Bojie Fu, Yue Li, Yuying Bai, Lijiao Chang, and Yu Chen. 2023. “One Country with two Systems: The Characteristics and Development of Higher Education in the Guangdong–Hong Kong–Macau Greater Bay Area.” Humanities and Social Sciences Communications 10 (1): 1–9. https://doi.org/10.1057/s41599-022-01483-z.
- Chicco, Davide. 2021. “Siamese Neural Networks: An Overview.” Artificial Neural Networks, 73–94. https://doi.org/10.1007/978-1-0716-0826-5_3.
- Ding, Z., Z. Mou, Y. Li, J. Wang, D. Wu, C. Liang, D. Hui, et al. 2024. “Cross-scale Spatial Variability and Associations of Carbon Pools Provide Insight Into Regulating Carbon Sequestration in Tropical Montane Rainforests.” Journal of Environmental Management 353: 120288. https://doi.org/10.1016/j.jenvman.2024.120288.
- Doyle, Eleanor, and Mauricio Perez-Alaniz. 2017. “From the Concept to the Measurement of Sustainable Competitiveness: Social and Environmental Aspects.” Entrepreneurial Business and Economics Review 5 (4): 35–59. https://doi.org/10.15678/EBER.2017.050402.
- Ferguson, Therese, and Carmel Geneva Roofe. 2020. “SDG 4 in Higher Education: Challenges and Opportunities.” International Journal of Sustainability in Higher Education 21 (5): 959–975. https://doi.org/10.1108/IJSHE-12-2019-0353.
- Ferreira, Bruno, Muriel Iten, and Rui G Silva. 2020. “Relationship Between Agricultural Pesticides and the Diet of Riparian Spiders in the Field.” Environmental Sciences Europe 32 (1): 1–17. https://doi.org/10.1186/s12302-019-0282-1.
- Gao, Xiaodong, Xining Zhao, Pute Wu, Min Yang, Miaotai Ye, Lei Tian, Yufeng Zou, Yong Wu, Fusuo Zhang, and Kadambot HM Siddique. 2021. “The Economic–Environmental Trade-off of Growing Apple Trees in the Drylands of China: A Conceptual Framework for Sustainable Intensification.” Journal of Cleaner Production 296: 126497. https://doi.org/10.1016/j.jclepro.2021.126497.
- Global Taskforce of Local and Regional Governments. 2016. Roadmap for Localizing the SDGs: Implementation and Monitoring at Subnational Level. Accessed on September 28, 2023. https://www.uclg.org/sites/default/files/roadmap_for_localizing_the_sdgs_0.pdf.
- Gulseven, Osman, and Gouher Ahmed. 2022. “The State of Life on Land (SDG 15) in the United Arab Emirates.” International Journal of Social Ecology and Sustainable Development 13 (1): 1–15. https://doi.org/10.4018/IJSESD.306264.
- Guo, Huadong. 2021. Big Earth Data in Support of the Sustainable Development Goals (2020). Beijing: EDP Sciences.
- Guo, Huadong, Fang Chen, Zhongchang Sun, Jie Liu, and Dong Liang. 2021. “Big Earth Data: A Practice of Sustainability Science to Achieve the Sustainable Development Goals.” Science Bulletin 66 (11): 1050. https://doi.org/10.1016/j.scib.2021.01.012.
- Guo, Huadong, Dong Liang, Zhongchang Sun, Fang Chen, Xinyuan Wang, Junsheng Li, Li Zhu, Jinhu Bian, Yanqiang Wei, and Lei Huang. 2022. “Measuring and Evaluating SDG Indicators with Big Earth Data.” Science Bulletin 67 (17): 1792–1801. https://doi.org/10.1016/j.scib.2022.07.015.
- Guo, Huadong, Zhen Liu, Hao Jiang, Changlin Wang, Jie Liu, and Dong Liang. 2017. “Big Earth Data: A new Challenge and Opportunity for Digital Earth’s Development.” International Journal of Digital Earth 10 (1): 1–12. https://doi.org/10.1080/17538947.2016.1264490.
- Guo, Huadong, Stefano Nativi, Dong Liang, Max Craglia, Lizhe Wang, Sven Schade, Christina Corban, Guojin He, Martino Pesaresi, and Jianhui Li. 2020. “Big Earth Data Science: An Information Framework for a Sustainable Planet.” International Journal of Digital Earth 13 (7): 743–767. https://doi.org/10.1080/17538947.2020.1743785.
- Guzmán, P. C., A. R. Pereira Roders, and B. J. F. Colenbrander. 2017. “Measuring Links Between Cultural Heritage Management and Sustainable Urban Development: An Overview of Global Monitoring Tools.” Cities 60: 192–201. https://doi.org/10.1016/j.cities.2016.09.005.
- Jean, Neal, Marshall Burke, Michael Xie, W. Matthew Davis, David B Lobell, and Stefano Ermon. 2016. “Combining Satellite Imagery and Machine Learning to Predict Poverty.” Science 353 (6301): 790–794. https://doi.org/10.1126/science.aaf7894.
- Jiang, Huiping, Zhongchang Sun, Huadong Guo, Qihao Weng, Wenjie Du, Qiang Xing, and Guoyin Cai. 2021. “An Assessment of Urbanization Sustainability in China Between 1990 and 2015 Using Land use Efficiency Indicators.” Npj Urban Sustainability 1 (1): 34. https://doi.org/10.1038/s42949-021-00032-y.
- Kavvada, Argyro, Graciela Metternicht, Flora Kerblat, Naledzani Mudau, Marie Haldorson, Sharthi Laldaparsad, Lawrence Friedl, Alex Held, and Emilio Chuvieco. 2020. “Towards Delivering on the Sustainable Development Goals Using Earth Observations.” Remote Sensing of Environment 247: 111930. https://doi.org/10.1016/j.rse.2020.111930.
- Lafortune, G., K. Zoeteman, G. Fuller, R. Mulder, J. Dagevos, and G. Schmidt-Traub. 2019. The 2019 SDG Index and Dashboards Report for European Cities (Prototype Version). Tilburg, Netherlands: Sustainable development solutions network (SDSN) and the brabant center for sustainable development (Telos).
- Laumann, Felix, Julius von Kügelgen, Thiago Hector Kanashiro Uehara, and Mauricio Barahona. 2022. “Complex Interlinkages, Key Objectives, and Nexuses among the Sustainable Development Goals and Climate Change: A Network Analysis.” The Lancet Planetary Health 6 (5): e422–e430. https://doi.org/10.1016/S2542-5196(22)00070-5.
- Li, S., H. Guo, Z. Sun, Z. Liu, H. Jiang, and H. Zhang. 2023. “Patterns of Typical Chinese Urban Agglomerations Based on Complex Spatial Network Analysis.” Remote Sensing 15 (4): 920. https://doi.org/10.3390/rs15040920.
- Liang, Anning, Dongmei Yan, Jun Yan, Yayang Lu, Xiaowei Wang, and Wanrong Wu. 2023. “A Comprehensive Assessment of Sustainable Development of Urbanization in Hainan Island Using Remote Sensing Products and Statistical Data.” Sustainability 15 (2): 979. https://doi.org/10.3390/su15020979.
- Lusseau, David, and Francesca Mancini. 2019. “Income-based Variation in Sustainable Development Goal Interaction Networks.” Nature Sustainability 2 (3): 242–247. https://doi.org/10.1038/s41893-019-0231-4.
- Lynch, A., A. LoPresti, and C. Fox. 2019. The 2019 US Cities Sustainable Development Report. New York: Sustainable Development Solutions Network (SDSN).
- Mainali, Brijesh, Jyrki Luukkanen, Semida Silveira, and Jari Kaivo-oja. 2018. “Evaluating Synergies and Trade-Offs among Sustainable Development Goals (SDGs): Explorative Analyses of Development Paths in South Asia and Sub-Saharan Africa.” Sustainability 10 (3): 815. https://doi.org/10.3390/su10030815.
- McDonald, David A, Thomas Marois, and Susan Spronk. 2021. “Public Banks + Public Water = SDG 6?” Water Alternatives 14 (1): 117–134.
- Neath, Andrew A., and Joseph E. Cavanaugh. 2012. “The Bayesian Information Criterion: Background, Derivation, and Applications.” WIRES Computational Statistics 4 (2): 199–203. https://doi.org/10.1002/wics.199.
- Paganini, M., I. Petiteville, S. Ward, G. Dyke, M. Steventon, J. Harry, and Flora Kerblat. 2018. Satellite Earth Observations in Support of the Sustainable Development Goals. The CEOS Earth Observation Handbook. Paris, France: Committee on Earth Observation Satellites.
- The People’s Government of Hainan province. 2011. “Hainan Province 12th Five-Year Plan Outline (Draft Sum-mary).” The People’s Government of Hainan province. Accessed September 28, 2023. https://www.hainan.gov.cn/hainan/5309/201102/4855238c256544b1a4137f62044f2c81.shtml.
- The People’s Government of Hainan province. 2016. “Hainan province Outline of the 13th Five-Year Plan for National Economic and Social Development (Summary)” The People's Government of Hainan province. Accessed September 28, 2023. https://www.hainan.gov.cn/hainan/5334/201603/2630f296222447a890bce91d106f7e6c.shtml.
- People’s Government of Hainan province. 2020. Overall Plan for the Construction of Hainan Free Trade. Accessed on February 2, 2024. https://www.hainan.gov.cn/hainan/hnzmgztfa/hnzmgztfa.shtml.
- Pradhan, Prajal, Luís Costa, Diego Rybski, Wolfgang Lucht, and Jürgen P Kropp. 2017. “A Systematic Study of Sustainable Development Goal (SDG) Interactions.” Earth’s Future 5 (11): 1169–1179. https://doi.org/10.1002/2017EF000632.
- Sadoff, Claudia W, Edoardo Borgomeo, and Stefan Uhlenbrook. 2020. “Rethinking Water for SDG 6.” Nature Sustainability 3 (5): 346–347. https://doi.org/10.1038/s41893-020-0530-9.
- Satterthwaite, David. 2016. “A New Urban Agenda?” Environment and Urbanization 28 (1): 3–12. https://doi.org/10.1177/0956247816637501.
- Sean, Allan. 2023. “Localizing the SDGs in Cities: Reflections from an Action Research Project in Bristol, UK.” Urban Geography 44 (3): 517–537. https://doi.org/10.1080/02723638.2021.1953286.
- Shumway, R. H., D. S. Stoffer, R. H. Shumway, and D. S. Stoffer. 2017. Time Series Analysis and its Applications: With R Examples, 75–163
- Simon, David, Helen Arfvidsson, Geetika Anand, Amir Bazaz, Gill Fenna, Kevin Foster, Garima Jain, Stina Hansson, Louise Marix Evans, and Nishendra Moodley. 2016. “Developing and Testing the Urban Sustainable Development Goal’s Targets and Indicators – a Five-City Study.” Environment and Urbanization 28 (1): 49–63. https://doi.org/10.1177/0956247815619865.
- Sinha, Avik, Tuhin Sengupta, and Rafael Alvarado. 2020. “Interplay Between Technological Innovation and Environmental Quality: Formulating the SDG Policies for Next 11 Economies.” Journal of Cleaner Production 242: 118549. https://doi.org/10.1016/j.jclepro.2019.118549.
- Song, Wen, Shisong Cao, Mingyi Du, and Linlin Lu. 2023. “Distinctive Roles of Land-use Efficiency in Sustainable Development Goals: An Investigation of Trade-Offs and Synergies in China.” Journal of Cleaner Production 382: 134889. https://doi.org/10.1016/j.jclepro.2022.134889.
- Sustainable Development Solutions Network. 2015. Indicators and a Monitoring Frameworkfor the Sustainable Development Goals. Accessed on September 28, 2023. https://sustainabledevelopment.un.org/content/documents/2013150612-FINAL-SDSN-Indicator-Report1.pdf.
- Sustainable Development Solutions Network. 2016. Getting Started with the SDGs in Cities. Accessed on September 28, 2023. https://irp-cdn.multiscreensite.com/6f2c9f57/files/uploaded/Cities-SDG-Guide.pdf.
- Swain, Ranjula Bali, and Shyam Ranganathan. 2021. “Modeling Interlinkages Between Sustainable Development Goals Using Network Analysis.” World Development 138: 105136. https://doi.org/10.1016/j.worlddev.2020.105136.
- Tiefelsdorf, Michael, and Barry Boots. 1997. “A Note on the Extremities of Local Moran’s Iis and Their Impact on Global Moran’s I.” Geographical Analysis 29 (3): 248–257. https://doi.org/10.1111/j.1538-4632.1997.tb00960.x.
- UN-Habitat. 2014. Localizing the Post-2015 Development Agenda. Accessed on September 28, 2023. https://www.uclg.org/sites/default/files/dialogues_on_localizing_the_post-2015_development_agenda.pdf.
- United Nations. 2015. “Transforming our World: The 2030 Agenda for Sustainable Development,” Working Papers id: 7559, eSocialSciences.
- United Nations. 2018. The World’s Cities in 2018 – DataBooklet (ST/ESA/ SER.A/417).
- United Nations. 2022. World Cities Report 2022. Accessed on September 28, 2023. https://unhabitat.org/sites/default/files/2022/06/wcr_2022.pdf.
- United Nations. 2023. The Sustainable Development Goals Report 2023. Accessed on September 28, 2023. https://unstats.un.org/sdgs/report/2023/The-Sustainable-Development-Goals-Report-2023.pdf.
- United Nations. n.d. “SDG Global Database Gives you Access to Data on More Than 210 SDG Indicators for Countries Across the Globe.” United Nations. Accessed on September 28, 2023. https://unstats.un.org/sdgs/dataportal.
- Warchold, Anne, Prajal Pradhan, and Jürgen P Kropp. 2021. “Variations in Sustainable Development Goal Interactions: Population, Regional, and Income Disaggregation.” Sustainable Development 29 (2): 285–299. https://doi.org/10.1002/sd.2145.
- Wu, Xutong, Bojie Fu, Shuai Wang, Yanxu Liu, Ying Yao, Yingjie Li, Zhenci Xu, and Jianguo Liu. 2023. “Three Main Dimensions Reflected by National SDG Performance.” The Innovation 4 (6): 100507. https://doi.org/10.1016/j.xinn.2023.100507.
- Wu, Xutong, Bojie Fu, Shuai Wang, Shuang Song, David Lusseau, Yanxu Liu, Zhenci Xu, and Jianguo Liu. 2023. “Bleak Prospects and Targeted Actions for Achieving the Sustainable Development Goals.” Science Bulletin, https://doi.org/10.1016/j.scib.2023.09.010.
- Xiao, Huijuan, Sheng Bao, Jingzheng Ren, and Zhenci Xu. 2023. “Transboundary Impacts on SDG Progress Across Chinese Cities: A Spatial Econometric Analysis.” Sustainable Cities and Society 92: 104496. https://doi.org/10.1016/j.scs.2023.104496.
- Xiao, Huijuan, Zhenci Xu, Jingzheng Ren, Ya Zhou, Ruojue Lin, Sheng Bao, Long Zhang, Shengfang Lu, Carman KM Lee, and Jianguo Liu. 2022. “Navigating Chinese Cities to Achieve Sustainable Development Goals by 2030.” The Innovation 3 (5), https://doi.org/10.1016/j.xinn.2022.100288.
- Zhang, Junlong, and Yu Luo. 2017. “Degree Centrality, Betweenness Centrality, and Closeness Centrality in Social Network.” Paper Presented at the 2017 2nd International Conference on Modelling, Simulation and Applied Mathematics (MSAM2017), Bangkok, Thailand, March 2017.
- Zhang, Chi, Zhongchang Sun, Qiang Xing, Jialong Sun, Tianyu Xia, and Hao Yu. 2021. “Localizing Indicators of SDG11 for an Integrated Assessment of Urban Sustainability—A Case Study of Hainan Province.” Sustainability 13 (19): 11092. https://doi.org/10.3390/su131911092.
- Zhang, Xinyi, Li Zhang, Linyan Bai, Jingjuan Liao, Bowei Chen, and Min Yan. 2023. “Assessment of Localized Targets of Sustainable Development Goals and Future Development on Hainan Island.” Sustainability 15 (11): 8551. https://doi.org/10.3390/su15118551.
- Zhong, Rui, Fengsong Pei, Kuiqi Yang, Yan Xia, Huaili Wang, and Gaoxin Yan. 2021. “Coordinating Socio-Economic and Environmental Dimensions to Evaluate Regional Sustainability – Towards an Integrative Framework.” Ecological Indicators 130: 108085. https://doi.org/10.1016/j.ecolind.2021.108085.
- Zhou, Changchang, Mimi Gong, Zhenci Xu, and Shen Qu. 2022. “Urban Scaling Patterns for Sustainable Development Goals Related to Water, Energy, Infrastructure, and Society in China.” Resources, Conservation and Recycling 185: 106443. https://doi.org/10.1016/j.resconrec.2022.106443.