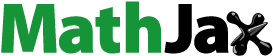
ABSTRACT
Since the initiation of the Grain for Green Project (GFGP) in 1999, dramatic change in vegetation status on the Loess Plateau. Spatially, geographical detector was employed to detect dominant variables influencing the spatial arrangement of normalized difference vegetation index (NDVI). Temporally, lagged or accumulated monthly precipitation, temperature and standardized precipitation evapotranspiration indices (SPEIs) sensitive to the monthly NDVI were first detected for every individual pixel, and the correlation between the NDVI and meteorological elements with time-lag effects was established a random forest model of unchanged land cover, followed by attributing impacts of climatic alterations and human interventions through residual examination across changed land cover. The findings indicate that (1) precipitation, slope, and soil dominantly influence the spatial arrangement of the NDVI. (2) Precipitation in current the month and cumulative temperatures of the previous 1–2 months steadily affect vegetation growth significantly, the optimal accumulation time interval for SPEI around 2000 are 8 months and 4 months, respectively. (3) Increases in the average NDVI within woodland and meadow vegetation on the Loess Plateau were primarily driven by climate change before 2000, accounting for 76.2%, whereas after 2000 it was dominantly driven by human activities, accounting for 64.16%.
1 Introduction
Plant life serves as an inherent connection linking factors such as earth, air, and H2O and assumes a notable function in aqua, substance, and vitality rotations (Deng et al. Citation2019). Moreover, plant life exhibits heightened responsiveness to alterations in the surroundings and is frequently viewed as a gauge of worldwide ecological and climatic alterations. As the supreme indicator of plant development and coverage, the normalized difference in the plant vitality index (NDVI) is a surface trait parameter of remote sensing data that mirrors the arrangement and alterations of plant life. This constitutes a fundamental dataset for plant investigations and has been extensively applied in examinations concerning alterations in plant cover and categorization of land cover, desertification of land, and primary productivity of vegetation (Wei et al. Citation2022).
Alterations in plant life resulting from the combined impact of climate transformations and human interventions (Qi et al. Citation2019). The surge in worldwide temperatures and shifts in rainfall induced by planetary warming notably influence configurations and alterations in plant life. Moreover, anthropogenic elements, comprising the execution of projects for ecological rehabilitation, strategies for land administration, pasturing, and urban development, propel alterations in plant life (Qiao, Jiang, and Zhang Citation2021). The Loess Plateau experiences intense soil depletion and stands as a locality with ecological fragility, constituting a pivotal zone for safeguarding soil and water in China. To enhance the declining setting on the Loess Plateau, China has initiated enforcements of transforming farmland into woodland and grassland, instating and applying the foundational concept that verdant mountains and ample water are priceless treasures, and adhering to the principles of ecological precedence and eco-friendly advancement. The growth and maturation of plant life constitute an intricate progression and are impacted by an array of natural elements and human interventions. Consequently, delving into the attributes of alterations in plant life on the Loess Plateau and scrutinizing the repercussions of climatic transformations and human interventions on vegetation has immense significance and becomes imperative.
Rainfall and temperature stand out as the two most common and comprehensive climate elements for studying changes in plant life (Su et al. Citation2016). Within the interplay, the temporal impact of climate and plant life is an essential phenomenon (Ma et al. Citation2022). If climate alterations surpass the ability of plant life to adjust, the reaction of vegetation to the expansion of climatic elements might be delayed (Zhao et al. Citation2020a). Specifically, the time-lag effects represent vegetation growth being signally affected by the climatic conditions of a certain previous month (Wu et al. Citation2015), while the time-accumulation effects represent vegetation growth being markedly affected by the cumulative climatic condition over the previous months as well as the current month (Vicente-Serrano et al. Citation2013). Certain earlier investigations have already scrutinized the time-lag consequences of conventional climate markers, encompassing precipitation and temperature, on alterations in plant life (Ding, Li, and Peng Citation2020; Wang, Chen et al. Citation2023). When analysing vegetation changes caused by climate change, an increasing number of studies are beginning to consider time-lag effects. Wu et al. (Citation2015) discovered that, with the incorporation of time-delay consequences, climatic elements can account for 64% of alterations in global plant life, marking an 11% elevation compared to neglecting time-lag effects.
Apart from climatic alterations, human interventions also serve as a principal impetus for variations in plant life. Due to population expansion and economic progress, human interventions exert a mounting influence on plant life (Liu et al. Citation2015). Zheng et al. (Citation2019) proposed that the Green for Grain Initiative executed on the Loess Plateau has contributed to some of the rejuvenation of plant life. Chu et al. (Citation2019) demonstrated through residual trend analysis that human interventions might result in the deterioration of plant life in agricultural zones within the Heilongjiang River Basin. Thus, gauging the individual contributions of meteorological shifts and human interventions to the dynamics of plant life holds substantial importance for formulating judicious strategies for ecological restoration (Tong et al. Citation2017).
In recent years, numerous investigations have been carried out on alterations in plant life on the Loess Plateau. The residual technique, which is grounded in linear regression, is frequently employed to measure the influences of climatic variations and human interventions on alterations in plant life. However, it does not account for the nonlinear responsive connections between vegetation and climate or the hysteresis effects of plant life on climatic factors. The principal aims of this research are as follows: (1) To scrutinize the spatiotemporal patterns of NDVI variations on the Loess Plateau from 1982 to 1999 and 2000 to 2020; (2) To establish the time-delay impact of climatic vegetation variables, considering the random forest regression analysis methods models; and (3) To measure the roles of climatic shifts and human efforts in the dynamics of flora within the woodlands and meadows of the Loess Plateau.
2 Study area and data
2.1 Study area
Situated in the northwestern inland region of China, in the middle reaches of the Yellow River, the Loess Plateau spans from 100°54′ to 114°33′E longitude and 33°43′ to 41°16′N latitude. With an estimated area of approximately 630,000 km2, the topography of the Loess Plateau experiences substantial variation, gradually diminishing from the northwest to the southeast ((a)). The predominant land cover types on the plateau comprise grassland and cultivated areas ((b)).
2.2 Data
2.2.1 NDVI products
The NDVI information employed in this investigation, covering the period from 1982 to 1999, was acquired from the Advanced Very High Resolution Radiometer (AVHRR) NDVI product of the National Oceanographic and Atmospheric Administration’s climate data record. The dataset possesses spatial and temporal resolutions of 5 kilometers and 16 days, respectively. As indicated by Sun et al. (Citation2021), NDVI information spanning from 2000 to 2020 was procured from the MODIS13Q1 product, which from NASA has a spatial resolution of 250 meters and a temporal resolution of 16 days. The method of maximum vegetation composite was employed for additional removal of disruptions arising from elements such as clouds and the atmosphere (Holben Citation1986; Stow et al. Citation2004) and to correct the two NDVI datasets.
2.2.2 Climatic data
The precipitation information was sourced from the National Centers for Environmental Prediction in the United States. The temperature and radiation data are from the ERA5 dataset of Atmospheric Reanalysis of Global Climate. We employed the standardized precipitation-evapotranspiration index (SPEI) to evaluate the intensity and duration of drought. The monthly SPEI dataset is sourced from the following website: https://spei.csic.es/spei_database/ (Vicente-Serrano, Beguería, and Lopez-Moreno Citation2010). The SPEI is multifaceted; for instance, the 1-month SPEI and the 12-month SPEI furnish insights into the drought circumstances for the present month and the complete year, respectively (Vicente-Serrano et al. Citation2013). The resolution of the climatic information was maintained in harmony with that of the NDVI.
2.2.3 Other data
The information regarding land classification products comes from three decades land cover dataset of the Loess Plateau in China spanning from 1990 to 2020 (Wang, Cheng et al. Citation2023). The elevation information, represented by the digital elevation model (DEM), was procured from Japan’s AW3D30 product, possessing a spatial resolution of 30 meters. The slope and aspect were computed utilizing the DEMs and were identified through the use of raster analysis tools in ArcGIS software. The Topographic Index is a comprehensive index that describes the morphology of surface features. The calculation of the terrain index utilizes elevation and slope data and applies the point by point scaling method to compare the elevation and slope data of each pixel with the average value of the entire region, in order to obtain the terrain index of each pixel. Soil types are divided into 12 types: leached soil, semi-leached soil, calcareous soil, arid soil, desert soil, primary soil, semi-hydraulic soil, hydraulic soil, saline alkali soil, anthropogenic soil, alpine soil, and others. Soil texture data include silt content, sand content, and clay content. The nighttime light dataset is sourced from the National Science and Technology Infrastructure Platform – National Earth System Science Data Center’s ‘NPP-VIIRS like’ nighttime light dataset. Other data can be found in .
Table 1. Data sources for the study area.
2.4.4 Data source
3 Methods
3.1 Overall methodology
The overall workflow of this study is illustrated in . uses geographic detectors to analyze the dominant factors affecting NDVI spatial differentiation based on the annual average of NDVI and the annual average of various factors. Based on monthly NDVI and monthly rainfall, temperature, and SPEI analysis, the time lag effect of NDVI on climate factors is analyzed. Based on this, a random forest regression model is established for NDVI and climate factors in areas with unchanged farmland, grassland, and bare land. It is then applied to areas where land use types change, and residual analysis is used to attribute the impact of climate change and human activities.
3.2 NDVI trend analysis
Theil-Sen Median trend analysis and the Mann-Kendall test were combined to investigate the trend characteristic of NDVI in the study period (Cao et al. Citation2014). Theil-Sen Median method is a robust trend calculation method of non-parametric statistics, which is also known as Sen-slope estimation (Ahmed Citation2014). The Sen-slope is defined as follows.
(1)
(1) where i and j denote the indices of years, i, j = 1, 2 … , n, with n being the total count of years in the time series. NDVIi and NDVIj represent the time series data for the NDVI.
Mann-Kendall (MK) is a non-parametric test method, which does not require samples to follow a certain distribution compared with other parameter test methods. Sen-slope value is calculated first, and then the MK method is used to determine the trend significance. The test statistic S is defined as follows.
(2)
(2)
(3)
(3)
The statistic S is approximately normally distributed with the variance as follows.
(4)
(4) The standardized test statistic Z is computed by
(5)
(5)
The statistic Z follows the standard normal distribution with a mean of zero and a variance of one. The critical value is 1.96 at the significance level a = 0.05, that is, if the trend has passed the significance test with confidence intervals of 95%.
3.3 Geographic detector
The geodetector stands as a statistical approach for identifying spatial heterogeneity and correlation patterns of driving factors (Wang et al. Citation2010). We employ this analytical tool to compute the explanatory capacity of the spatial variation factors that affect the NDVI. The explanatory power is computed as follows:
(6)
(6) where q is the explanatory capability of element Xh. When q = 1, spatial fluctuations in the NDVI are entirely governed by this singular element. Conversely, when q = 0, this factor is unrelated to the variation in the NDVI. n denotes the number of grids. nXh represents the number of subregions influenced by factor Xh. The geodetector can also determine whether there is an interaction between two factors by comparing the single factor q value and the q value after the two factors are superimposed.
Considering the effects of climate, topography, soil composition, and human interventions on vegetation on the Loess Plateau, a total of 12 factors-precipitation, temperature, aspect, slope, altitude, clay content, sand content, silt content, soil type, topographic index, population density, and nighttime light data-are chosen as the determinants impacting the NDVI. However, data on population density and nighttime light exposure prior to 2000 are lacking. We examined the factors shaping the spatial alterations in the NDVI throughout the periods of 1982–1999 and 2000–2020. In the execution of the geographical detector, 12 factors possessing continuous values (excluding soil type) are chosen and categorized into nine groups utilizing the natural fracture method. The soil types with discrete values (soil) are retained, resulting in the original 13 categories.
3.4 Time-lag impacts of meteorological factors on alterations in vegetation
For precipitation (pre) and temperature (temp), the delayed climatic factors are specified as follows:
(7)
(7) where PREt(m, n) signifies the precipitation in the tth month with an m-month lag and an aggregation over n months. The total precipitation in the present month and the antecedent n months were computed. Here, m denotes the lag duration, n signifies the accumulation duration, and PREt-m-i refers to the precipitation in the m + i months preceding the current month. Experience has shown that at the monthly scale, the temporal delay in the reaction of vegetation to climate typically falls below one-fourth (Chen et al. Citation2014). Hence, m and n chosen from the interval of 0–3 months are chosen for in this document. The temporal delay (m and n) that exhibits the highest correlation coefficient is deemed the optimal time delay for the reaction of the NDVI to precipitation and temperature.
For the SPEI, the correlation coefficients of the monthly NDVI and accumulated SPEI within the 1-month to 12-month timeframe are regarded as potential indicators for assessing the cumulative effect of drought. The duration of the month displaying the highest coefficients is regarded as the cumulative impact of the drought.
3.5 Distinguishing the contributions of meteorological conditions and human interventions through a random forest model
The hyperparameters of the random forest model include the number of decision trees (n-estimators), the maximum depth of the decision tree (max_depth), the minimum number of separated samples (i.e. the minimum number of samples required to split decision tree nodes) min_samples_split, and the minimum number of leaf node samples (i.e. the minimum number of samples required to include a leaf node) min_samples_leaf. First, give a range of hyperparameters, and then use the RandomizedSearchCV function to randomly match each combination of hyperparameters and output the optimal combination.
In this paper, the vegetation of forests and grasslands is partitioned into two segments. A segment comprises the unaltered region (including unchanged forest, unchanged shrub, unchanged grassland, and unchanged bare land), and the other part is the changed area (including farmland transformed into forest and grassland, grassland transformed into forest and shrubland, and bare land transformed into forest and grassland). We believe that the unchanged areas are natural restoration areas, while the changed areas are man-made restoration areas. Moreover, we posit that the unaffected natural plant life is influenced solely by climate alterations, whereas plant life in the altered zones experienced the repercussions of both meteorological changes and human interventions (Shi et al. Citation2021).
The construction and simulation process of a random forest is carried out using the Python 3.7 scikit learn module. Taking the period 2000–2020 as an example, first, by utilizing land use data from 2000 and 2020, we acquired the unchanged and changed regions spanning from 2000 to 2020. Second, the yearly mean values of precipitation, temperature, and SPEI within the two zones, as well as six predictive factors of their slope trends, are calculated. A random forest model of the unchanged areas is trained, and a relationship model between the interannual fluctuation tendency (target variable) of the NDVI within the unchanged farmland, unchanged grassland, and unchanged bare land areas. Six predictive factors (predictive variables) were established. Finally, the three random forest models for the unchanged regions were applied to the three changed regions to obtain the proportionate impact of climate alterations in the changed regions on the variation trend of the NDVI. The actual NDVI trend is subtracted from the NDVI trend predicted by the trained model to ascertain the proportionate impact of human interventions.
4 Results
4.1 Temporal–spatial variations in the NDVI
Figure indicates that from 1982 to 1999 (y = 0.0013x − 2.30, R2 = 0.44, P < 0.05) and from 2000 to 2020 (y = 0.0051x − 10.00, R2 = 0.94, P < 0.01), the NDVI exhibited an increasing trend and significantly increased after 2000. In approximately 2000, the region manifested an increasing NDVI trend on the Loess Plateau increasing from 89.98% to 96.48%. The monthly average NDVI slope for each month during the two time periods is greater than 0, and the monthly average NDVI slope for the 12 months after 2000 is higher than before 2000, indicating that vegetation changes are more significant after 2000 ().
Figure 3. Evaluations of NDVI patterns on the Loess Plateau from 1982 to 2020. (A) Chronological progression of NDVI. The figures represent the rates of NDVI alteration, * denotes noteworthy alterations at the significance threshold of P < 0.05, and ** denotes noteworthy alterations at the significance threshold of P < 0.01. (B) Probability density distribution of the NDVI tendencies throughout the two intervals. The bars illustrate the ratios of heightened (gray) and diminished (black) reactions. (C) Average monthly NDVI variations between the two durations. The shaded region in (A) and the error bars in (C) signify ±1 SD.

The Theil-Sen median trend analysis and Mann-Kendall trend tests were implemented on all vegetation grids across the Loess Plateau to investigate spatial changes in yearly NDVI. The linear change rate of the yearly NDVI during the study period 1982–1999 ranged from −0.06 to 0.11/10a, predominantly falling between −0.02/10a and 0.04/10a. The linear alteration rate of the yearly NDVI from 2000 to 2020 from −0.4 to 0.37/10 years, mainly residing between 0 and 0.12/10 years. The percentages of the vegetation regions with an upward increase on the Loess Plateau throughout the periods 1982–1999 and 2000–2020 were 89.98% and 96.48%, respectively. The significance of the NDVI tendencies significantly increased from 43.4% to 89.5% in approximately ().
Figure 4. (a) Linear slope of the annual NDVI, (b) significance threshold of the annual NDVI, and (c) NDVI trend in the unchanged and variable areas from 1982 to 1999 on the Loess Plateau. (d) Linear slope of the annual NDVI, (e) significance threshold of the annual NDVI, and (f) NDVI trend in unchanged and variable areas from 2000 to 2020 on the Loess Plateau. SUT, significant upwards trend; NUT, non-significant upwards trend; SDT, significant downwards trend; and NDT, non-significant downwards trend.

By analysing the NDVI trends for distinct land cover categories, the following conclusions were drawn: the gradient of NDVI alterations was highest in regions where grassland transformed into forest and shrubland prior to 2000, whereas the gradient was most pronounced in areas where farmland transitioned into forest and grassland after 2000. After 2000, the slope of the NDVI changed in all of the unchanged and variable regions increased.
4.2 Factors influencing the spatial differentiation of the NDVI
illustrates the weights and interactions of factors influencing spatial variation in the NDVI during the periods of 1982–1999 and 2000–2020. The results indicated was the most significant contributors to the spatial variation in the NDVI between 1982 and 1999 were precipitation, slope, and soil type, respectively, with no significant differences among these factors. The secondary driving factors of the NDVI were sediment content, sand content, and topographic index. The q-values for temperature and clay content were 0.183 and 0.115, respectively, indicating that they had smaller impacts on the NDVI changes. The impacts of aspect and elevation on the alterations in the NDVI were not significant, with q-values less than 0.1. From 2000 to 2020, precipitation, slope, and soil type remained the primary variables influencing geographical diversity in NDVI, boasting q-values of 0.490, 0.488, and 0.356, respectively, and there were no significant differences among these factors. The secondary driving factors of the NDVI included sediment content, sand content, and topographic index. The q-values of the temperature, clay content, and population density were 0.222, 0.112, and 0.103, respectively, indicating that they had smaller impacts on the NDVI changes. The q-values of the aspect, elevation, and light data were all less than 0.1.
Figure 5. (a) Changes in the explanatory powers (q-values) during the two periods. (b, c) The explanatory powers (q-values) after interactions during the two periods. X1–X12 represent precipitation, temperature, aspect, slope, elevation, clay content, sand content, silt content, soil type, terrain index, population density, and nighttime light data, respectively.

The interplay among the influencing elements during the intervals 1982–1999 and 2000–2020 exhibited dual-factor reinforcement or nonlinear amplification characteristics. This implies that the alterations in vegetation on the Loess Plateau stemmed from the collective impact of diverse elements. During the period 1982–1999, the interaction explanatory power was strongest for precipitation and sand content, registering a q-value of 0.642, succeeded by the interplay between precipitation and slope, attaining a q-value of 0.633. The individual q-values of the temperature, aspect, elevation, and clay content were 0.183, 0.028, 0.076, and 0.115, respectively, i.e. all less than 0.2. However, their impacts were significantly enhanced when they were combined with other factors. Their maximum interaction explanatory powers after overlaying reached 0.626, 0.500, 0.589, and 0.594, respectively. During the period 2000–2020, the explanatory power of interactions was strongest for precipitation and soil type. A q-value of 0.627, succeeded by the interaction between precipitation and temperature, attaining a q-value of 0.626. Individual q-values of aspect, elevation, and clay content, population density, and lighting data were 0.039, 0.062, 0.112, 0.103, and 0.008, respectively, i.e. all less than 0.2. However, their impacts were significantly enhanced when combined with other factors. Their maximum interaction explanatory powers after overlaying reached 0.498, 0.590, 0.538, 0.536, and 0.497.
4.3 Time-lag and accumulation effects of meteorological indicators on the NDVI variation
illustrates geographical patterns of lagged and accumulated months of the meteorological elements impacting the NDVI on the Loess Plateau. The lag and accumulation times of rainfall during 1982–1999 are 1.14 ± 0.73 and 2.07 ± 0.78 respectively. The cumulative area at 2 months constituted the most significant share, which was 39.52%; this was followed by a cumulative three-month period of 31.55%. The lag and accumulation times of rainfall during 2000–2020 were 0.89 ± 0.80 and 1.96 ± 0.84, respectively. The cumulative area at 2 months constituted the most significant share, which was 35.84%; this was followed by a cumulative one-month period of 30.68%. The lag and accumulation times of temperature during 1982–1999 are 0.99 ± 0.56 and 1.64 ± 0.85, respectively. The cumulative area after 1 month constituted the most significant share, which was 41.52%, followed by a cumulative two-month period of 33.15%. The lag and accumulation time of temperature during 2000–2020 were 1.20 ± 0.55 and 2.05 ± 0.79, respectively. The cumulative area of 2 months constituted the most significant share, which was 38.33%; this was followed by a cumulative one-month period of 24.70%. The SPEI exhibited accumulations of 8.11 ± 2.27 and 3.96 ± 1.65 during the periods 1982–1999 and 2000–2020, respectively. The largest area proportions before and after 2000 were accumulated for 8 months (32.92%) and 4 months (55.46%), respectively ().
Figure 6. Spatial distributions of the lag and accumulation durations of meteorological elements influencing the NDVI on the Loess Plateau.

Figure 7. Area ratios of the lag and accumulation times of the three factors on the Loess Plateau. (a) Rainfall and temperature, two of the same colors represent the two periods (left: 1982-1999, right: 2000-2020), (b) SPEI cumulative time area proportion of the two periods.

The correlations between different climatic factors and the NDVI varied significantly on different time scales. For rainfall, the 3-month accumulation dominates the western region and the 2-month accumulation dominates the eastern region. For temperature, before 2000, the two-month accumulation dominated the northern region, and the one-month accumulation dominated the southern region. After 2000, the dominant vegetation increased significantly in the 3-month cumulative area (Huang qiu area), and the dominant sand area in the 2-month cumulative area. The association between the estimated SPEI and NDVI underwent substantial variations over distinct temporal spans. In approximately 2000, the optimal accumulation time interval shifted from 8–10 months to 3–4 months. A longer accumulation time indicates greater drought resistance.
4.4 Impacts of human interventions and climatic shifts on the dynamics of vegetation
Based on independently developed surface cover products on the Loess Plateau, geographical distribution maps of unchanged and changed categories were obtained from 1990 to 2000 and from 2000 to 2020. The percentage of unaltered categories decreased from 94.28% to 74.84%, and the area of changed types increased from 2.83% to 19.82% ().
Figure 8. Spatial arrangement of unchanged (a) and variable classes (b) from 1990 to 2000; distribution of unchanged (c) and variable classes (d) from 2000 to 2020.

A random forest model was applied to gauge the contributions of the meteorological factors (including Pre, Temp, and SPEI) and human activities to the NDVI in the artificially restored areas of the Loess Plateau throughout the intervals of 1982–1999 and 2000–2020. The model considered the climate change lag and accumulation effects. The ecological restoration areas included transformation examples such as the conversion of cropland to forest/veldt pastureland, grassland to forest/shrubland, and barren terrain to forest/grassland.
During the period 1982–1999, in the artificially restored areas (farmland converted to forests and grassland, grassland converted to forests and shrubs, and bare land converted to forests and grassland), the total contributions of the meteorological factors (Pre, Temp, and SPEI) to the NDVI changes were 0.0089, 0.0116, and 0.0060, accounting for 78.76%, 74.84%, and 75.00%, respectively, of the total NDVI changes. The total contributions of anthropogenic actions on the NDVI changes in the artificially restored areas were 0.0024, 0.0039, and 0.0020, accounting for 21.24%, 25.16%, and 25.00% of the total NDVI changes(Figure ).
During the period 2000–2020, in the artificially restored areas (farmland converted to forests and grassland, grassland converted to forests and shrubs, and bare land converted to forests and grassland), the total contributions of the meteorological factors (Pre, Temp, and SPEI) to the NDVI changes were 0.0029, 0.0032, and 0.0013, accounted for 35.27%, 36.49%, and 35.76%, respectively, of the total NDVI changes. The overall impacts of anthropogenic actions on the changes in the NDVI restored areas were 0.0053, 0.0055, and 0.0023, accounting for 64.73%, 63.51%, and 64.24% of the total NDVI changes, respectively (; ).
Figure 9. Contributions of climate change (CC) and human activities (HA) to the NDVI of the forest and grassland vegetation from 1982 to 2020 on the Loess Plateau. The regions studied in (a) and (c) are unchanged forest, unchanged shrubland unchanged grassland, unchanged bare land, farmland transformed into forest and grassland, grassland transformed into forest and shrubland, and bare land transformed into forest and grassland, and the regions studied in (b) and (d) are farmland transformed into forest and grassland, grassland transformed into forest and shrubland, and bare land transformed into forest and grassland areas.

Table 2. Contributions of climate change (CC) and human activities (HA) to the NDVI at the different vegetation regions.
Throughout the woodland and meadow vegetation on the Loess Plateau, climate variation contributed to 98.88% of the impact before 2000, with human activities contributing 1.12%. The percentage of climate alteration post-2000 was 86.77%, while the engagement of human actions accounted for 13.23%. It is apparent that post-2000, the involvement of human actions notably escalated. Furthermore, the regions where human actions triggered an increase in the NDVI. Hence, the favourable influence of human actions on plant development notably intensified, and these regions were predominantly dispersed within the central region of the Loess Plateau.
5 Discussion
Measuring the proportional influences of human interventions and meteorological factors on alterations in vegetation is crucial for addressing the challenges posed by climate alteration. However, in previous studies, some issues remained unresolved. Initially, in estimating the effect of climate alteration on the fluctuations in vegetation, the neglect of the delayed and cumulative impacts of climatic factors on plant life has been disregarded. Second, multivariate linear regression or regular ordinary least squares modelling was frequently utilized to ascertain linear connections between vegetation indicators and climatic parameters, overlooking the problem of multicollinearity among climatic factors. We included the delayed and accumulated consequences of climatic factors when measuring the effects of climatic alteration and human actions on alterations in NDVI. We utilized a random forest model to formulate a model depicting the connection between the NDVI and climatic elements.
5.1 Climate-driven impacts on the NDVI
The Loess Plateau is located in the transitional zone from semi-humid to arid regions in China, with special terrain, geology, soil, etc., which may affect the spatial differentiation of NDVI. This article concludes that precipitation, soil type, and slope are the dominant factors. Precipitation is undoubtedly the most important driving factor affecting vegetation growth (Wang et al. Citation2020). Soil conditions are also one of the important factors affecting the spatial differentiation of NDVI. Leaching soil is suitable for vegetation growth, while desert and arid soil severely restrict vegetation growth. The slope affects the redistribution of rainfall and solar radiation, such as water evaporates faster on sunny slopes, while water evaporates slower on shady slopes. The change in slope leads to changes in water and heat conditions, which in turn affects the spatial distribution of vegetation cover (Trujillo et al. Citation2012; Sundqvist et al. Citation2014).
Table 3. The lag and accumulation (Accu) times of precipitation (Pre), temperature (Temp) and SPEI in the invariant and variable regions.
The temporal impacts of climatic elements exhibit strong spatial heterogeneity, with different regions having distinct primary drivers of vegetation growth changes, and their time effects also vary (). The precipitation gradient on the Loess Plateau ascends from northwest to southeast, so the diverse reactions to precipitation could be linked to the varied distribution pattern of rainfall (Davenport and Nicholson Citation1993). Hua et al. (Citation2017) validated the presence of a strong correlation between plant activity in the growing period and the incidence of drought in semiarid regions of China. The findings of this investigation suggested that the accumulated water deficit affects the development of vegetation in the majority of forests and grasslands, with cumulative time frames of 3–4 months and 8–10 months (). The effectiveness of moisture over longer periods of time scales may exert a notable influence on plant activity, a proposition also put forth in prior research (Zhan et al. Citation2022; Zhao et al. Citation2020b). Moreover, variations exist in the accumulated repercussions of drought on vegetation across diverse vegetation categories on the Loess Plateau. The drought tolerance of different flora categories is different, from grassland to cropland, to forest. This tendency could be associated with the root traits and water utilization behaviors of different flora categories (Peng et al. Citation2019).
In this research, we additionally discovered that, for the majority of vegetation categories, the influence of time-accumulation effects on the growth of plants exceeded that of time-lag effects. These influences are intricate and exhibit substantial variations among diverse climatic elements, vegetation types, and geographical areas. Analysing the time-delay and cumulative consequences provides a more precise comprehension of the connection between vegetation and climate.
5.2 Effects of climate change and human activities on NDVI change
Human actions have consistently been a pivotal element influencing alterations in vegetation, particularly since the complete execution of the Grain for Green Initiative in 1999 (Zheng et al. Citation2019). This research exclusively concentrates on the vegetation of forests and grasslands on the Loess Plateau. We compute the consequences of climatic elements and human actions on alterations in the NDVI in various vegetation zones. As depicted in , prior to 2000, the impacts of climatic elements were typically greater substantially in different regions and surpassed the impacts of human actions. The favourable influence of human actions notably rose post-2000, with expansive ecological restoration policies playing a pivotal role in fostering the greening of dry flora regions of northern China (Hu et al. Citation2021).
Figure 10. (a) Proportion of the unchanged and variable areas in the two periods; contributions of (b) meteorological elements and (c) human endeavors to NDVI in unchanged and variable areas on the Loess Plateau from 1982 to 2020. In the box plots, the two boxes of the same color represent the two periods.

Based on the research results, the involvement of human actions in the NDVI of forests and grassland vegetation increased from 23.8% before 2000 to 64.16% after 2000, signifying a notable enhancement in the environment through human activities. Before 2000, climatic elements exerted the most substantial influence on alterations in vegetation within the unaltered shrub region, while human endeavors exerted the most substantial influence on alterations in vegetation within the region where grassland transformed into forest and shrubland. After 2000, climatic factors exerted the most substantial influence on alterations in vegetation within the region where grassland transformed into forest and shrubs, while human endeavors exerted the most substantial influence on alterations in vegetation within the region where farmland transformed into forest and grassland.
However, vegetation recovery induced by human activities is often immediate and is not subject to natural constraints (Shi et al. Citation2021). A considerable body of research indicates that excessive vegetation recovery can lead to diminished runoff and soil desiccation (Li et al. Citation2021a). Several earlier investigations proposed the following reforestation has resulted in an increase in evapotranspiration and a decrease in water accessibility, intensifying water scarcity challenges in northern China (Feng et al. Citation2016; Liang et al. Citation2022; Liu et al. Citation2016). Therefore, a vegetation restoration plan that meets regional climatic circumstances suitable for the restoration of ecosystems essentially, aims to maintain water balance without destabilizing the ecology.
5.3 Limitations and uncertainty
Our investigation encompassed some uncertainties and constraints. A basis for the hypothesis of this study is based on prior knowledge such as the implementation policy of the 1999 Loess Plateau Grain for Green Project. Prior to implementation, most of the land was farmland and bare land, and the classification of land cover products was based on surface cover change detection algorithms (Wang et al. Citation2018). Therefore, it is believed that the 'grassland forest' type in this area can represent a forest and grassland vegetation enclosure area with less human activity impact. It should be pointed out that we are considering the general situation in most areas of the Loess Plateau, and there may be some exceptions, but individual exceptions will not affect the attribution analysis and results of the entire Loess Plateau region. First, the source of the data may introduce uncertainty. In this study, we used two NDVI datasets, AVHRR NDVI and MODIS NDVI (Li et al. Citation2021b). Notably, the results of vegetation change may be different due to differences in the resolution and quality of different NDVI products. Furthermore, remote sensing datasets are prone to contamination from clouds and haze (Zhang et al. Citation2023). Second, when the random forest model was established to model the predicted value of the NDVI and the input factors of the model were only the rainfall, temperature and SPEI, but there were other factors in the actual vegetation growth site environment that contributed to the NDVI. Finally, Human interventions are one of the important elements influencing plant life, but the influence of human interventions on enhancing vegetation resistance to meteorological conditions. In total, drought events, such as farmland irrigation and reservoir regulation, were not analysed. In addition, only the linear relationships between the NDVI and meteorological variables at the monthly level were considered, without eliminating seasonal variations. Despite the uncertainties and limitations mentioned above, our study still provides meaningful insights into the roles of vegetation change and its influencing factors.
6 Conclusions
In this investigation, we primarily scrutinized the spatiotemporal attributes of NDVI on the Loess Plateau during the periods 1982–1999 and 2000–2020, as well as the quantitative influence of climatic variations and human interventions on the NDVI fluctuations in the forest and grassland vegetation. The primary findings can be summarized as follows. (1) After 2000 the annual NDVI trend increased by 74.51%, and monthly NDVI trends in the growing season also improved significantly. (2) Precipitation, terrain slope, and soil type strongly influence the spatial distribution of the NDVI. Furthermore, the interaction of the two variables can better explain the alteration of the NDVI. (3) Precipitation in the current month and the cumulative temperature of the previous 1–2 months steadily affect vegetation growth significantly, and the optimal accumulation time interval for SPEI shifted from predominantly 8–10 months before 2000 to 3–4 months after 2000. (4) After 2000, the ratio of areas where NDVI was driven only by climate change decreased from 94.28% to 74.84%, whereas the percentage of areas where the NDVI was affected by a combination of climate change and human activities increased from 2.83% to 19.82%. The increase in average NDVI in the forest area and grassland vegetation on the Loess Plateau was primarily driven by climate change before 2000, accounting for 76.2%, whereas after 2000, it was dominantly driven by human activities, accounting for 64.16%. It is crucial to recognize immediately that the implementation of extensive afforestation and reforestation for ecological restoration strategy may not be conducted in every climatic region. Reasonable afforestation methods should consider local water conditions, the environment, and population density. In addition, the findings of this investigation, such as those of human interventions dominated NDVI changes within the woodland and grassland vegetation on the Loess Plateau, can inform the development of viable flora rehabilitation strategies for the Loess Plateau.
Author contributions
Acquisition of financial support for the project leading to this publication, Z.W.; Application of statistical, mathematical, computational, or other formal techniques to analyze or synthesize study data, M.C. and Z.W.; Y.Z. helped with some data processing; Preparation, creation, and/or presentation of the published work by those from the original research group, specifically critical review, commentary, or revision, including pre- or post-publication stages, S.W., Z.W., X.L. and W.J.
Acknowledgments
The authors thank Haolin Huang, Shuaifeng Peng and Yi Zhang for assistance in writing the code. The authors also thank LetPub (www.letpub.com) for its linguistic assistance during the preparation of this manuscript.
Disclosure statement
No potential conflict of interest was reported by the authors.
Data availability statement
The code used in this study is available upon contacting the corresponding author.
Additional information
Funding
References
- Ahmed, S. 2014. “Assessment of Irrigation System Sustainability Using the Theil-Sen Estimator of Slope of Time Series.” Sustainability Science 9: 293–302. https://doi.org/10.1007/s11625-013-0237-1.
- Cao, R., W. Jiang, L. Yuan, W. Wang, Z. Lv, and C. Zheng. 2014. “Inter-Annual Variations in Vegetation and Their Response to Climatic Factors in the Upper Catchments of the Yellow River from 2000 to 2010.” Journal of Geographical Sciences 24 (6): 963–979. https://doi.org/10.1007/s11442-014-1131-1.
- Chen, T., R. De Jeu, Y. Liu, G. Van der Werf, and A. Dolman. 2014. “Using Satellite Based Soil Moisture to Quantify the Water Driven Variability in NDVI: A Case Study Over Mainland Australia.” Remote Sensing of Environment 140: 330–338. https://doi.org/10.1016/j.rse.2013.08.022.
- Chu, H., S. Venevsky, C. Wu, and M. Wang. 2019. “NDVI-Based Vegetation Dynamics and Its Response to Climate Changes at Amur-Heilongjiang River Basin from 1982 to 2015.” Science of the Total Environment 650: 2051–2062. https://doi.org/10.1016/j.scitotenv.2018.09.115.
- Davenport, M., and S. Nicholson. 1993. “On the Relation Between Rainfall and the Normalized Difference Vegetation Index for Diverse Vegetation Types in East Africa.” International Journal of Remote Sensing 14 (12): 2369–2389. https://doi.org/10.1080/01431169308954042.
- Deng, C., B. Zhang, L. Cheng, L. Hu, and F. Chen. 2019. “Vegetation Dynamics and Their Effects on Surface Water-Energy Balance Over the Three-North Region of China.” Agricultural and Forest Meteorology 275: 79–90. https://doi.org/10.1016/j.agrformet.2019.05.012.
- Ding, Y., Z. Li, and S. Peng. 2020. “Global Analysis of Time-Lag and -Accumulation Effects of Climate on Vegetation Growth.” International Journal of Applied Earth Observation and Geoinformation 92: 102179. https://doi.org/10.1016/j.jag.2020.102179.
- Feng, X., B. Fu, S. Piao, S. Wang, P. Ciais, Z. Zeng, Y. Lv, Y. Zeng, Y. Li, X. Jiang, et al. 2016. “Revegetation in China’s Loess Plateau Is Approaching Sustainable Water Resource Limits.” Nature Climate Change 6 (11): 1019–1022. https://doi.org/10.1038/nclimate3092.
- Holben, B. 1986. “Characteristics of Maximum-Value Composite Images from Temporal AVHRR Data.” International Journal of Remote Sensing 7 (11): 1417–1434. https://doi.org/10.1080/01431168608948945.
- Hu, Y., H. Li, D. Wu, W. Chen, X. Zhao, M. Hou, A. Li, and Y. Zhu. 2021. “LAI-Indicated Vegetation Dynamic in Ecologically Fragile Region: A Case Study in the Three-North Shelter Forest Program Region of China.” Ecological Indicators 120: 106932. https://doi.org/10.1016/j.ecolind.2020.106932.
- Hua, T., X. Wang, C. Zhang, L. Lang, and H. Li. 2017. “Responses of Vegetation Activity to Drought in Northern China.” Land Degradation & Development 28 (7): 1913–1921. https://doi.org/10.1002/ldr.2709.
- Li, T., J. Xia, L. Zhang, D. She, G. Wang, and L. Cheng. 2021b. “An Improved Complementary Relationship for Estimating Evapotranspiration Attributed to Climate Change and Revegetation in the Loess Plateau, China.” Journal of Hydrology 592: 125516. https://doi.org/10.1016/j.jhydrol.2020.125516.
- Li, S., L. Xu, Y. Jing, H. Yin, X. Li, and X. Guan. 2021a. “High-Quality Vegetation Index Product Generation: A Review of NDVI Time Series Reconstruction Techniques.” International Journal of Applied Earth Observation and Geoinformation 105: 102640. https://doi.org/10.1016/j.jag.2021.102640.
- Liang, H., Y. Xue, Z. Li, G. Gao, and G. Liu. 2022. “Afforestation May Accelerate the Depletion of Deep Soil Moisture on the Loess Plateau: Evidence from a Meta-Analysis.” Land Degradation & Development 33 (18): 3829–3840. https://doi.org/10.1002/ldr.4426.
- Liu, Y., Y. Li, S. Li, and S. Motesharrei. 2015. “Spatial and Temporal Patterns of Global NDVI Trends: Correlations with Climate and Human Factors.” Remote Sensing 7 (10): 13233–13250. https://doi.org/10.3390/rs71013233.
- Liu, Y., J. Xiao, W. Ju, K. Xu, Y. Zhou, and Y. Zhao. 2016. “Recent Trends in Vegetation Greenness in China Significantly Altered Annual Evapotranspiration and Water Yield.” Environmental Research Letters 11 (9): 94010. https://doi.org/10.1088/1748-9326/11/9/094010.
- Ma, Y., Q. Guan, Y. Sun, J. Zhang, L. Yang, E. Yang, H. Li, and Q. Du. 2022. “Three-Dimensional Dynamic Characteristics of Vegetation and Its Response to Climatic Factors in the Qilian Mountains.” Catena 208: 105694. https://doi.org/10.1016/j.catena.2021.105694.
- Peng, J., C. Wu, X. Zhang, X. Wang, and A. Gonsamo. 2019. “Satellite Detection of Cumulative and Lagged Effects of Drought on Autumn Leaf Senescence Over the Northern Hemisphere.” Global Change Biology 25 (6): 2174–2188. https://doi.org/10.1111/gcb.14627.
- Qi, X., J. Jia, H. Liu, and Z. Lin. 2019. “Relative Importance of Climate Change and Human Activities for Vegetation Changes on China’s Silk Road Economic Belt Over Multiple Timescales.” Catena 180: 224–237. https://doi.org/10.1016/j.catena.2019.04.027.
- Qiao, Y., Y. Jiang, and C. Zhang. 2021. “Contribution of Karst Ecological Restoration Engineering to Vegetation Greening in Southwest China During Recent Decade.” Ecological Indicators 121: 107081. https://doi.org/10.1016/j.ecolind.2020.107081.
- Shi, S., J. Yu, F. Wang, P. Wang, Y. Zhang, and K. Jin. 2021. “Quantitative Contributions of Climate Change and Human Activities to Vegetation Changes Over Multiple Time Scales on the Loess Plateau.” Science of the Total Environment 755: 142419. https://doi.org/10.1016/j.scitotenv.2020.142419.
- Stow, D., A. Hope, D. Mcguire, D. Verbyla, J. Gamon, F. Huemmrich, S. Houston, C. Racine, M. Sturm, K. Tape, et al. 2004. “Remote Sensing of Vegetation and Land-Cover Change in Arctic Tundra Ecosystems.” Remote Sensing of Environment 89 (3): 281–308. https://doi.org/10.1016/j.rse.2003.10.018.
- Su, W., D. Yu, Z. Sun, J. Zhan, X. Liu, and Q. Luo. 2016. “Vegetation Changes in the Agricultural-Pastoral Areas of Northern China from 2001 to 2013.” Journal of Integrative Agriculture 15 (5): 1145–1156. https://doi.org/10.1016/S2095-3119(15)61159-5.
- Sun, Z., Z. Mao, L. Yang, Z. Liu, J. Han, H. Wanag, and W. He. 2021. “Impacts of Climate Change and Afforestation on Vegetation Dynamic in the Mu Us Desert.” Ecological Indicators 129: 108020. https://doi.org/10.1016/j.ecolind.2021.108020.
- Sundqvist, M. K., Z. Liu, R. Giesler, and D. A. Wardle. 2014. “Plant and Microbial Responses to Nitrogen and Phosphorus Addition Across An Elevational Gradient in Subarctic Tundra.” Ecology 95 (7): 1819–1835.
- Tong, X., K. Wang, Y. Yue, M. Brandt, B. Liu, C. Zhang, C. Liao, and R. Fensholt. 2017. “Quantifying the Effectiveness of Ecological Restoration Projects on Long-Term Vegetation Dynamics in the Karst Regions of Southwest China.” International Journal of Applied Earth Observation and Geoinformation 54: 105–113. https://doi.org/10.1016/j.jag.2016.09.013.
- Trujillo, E., N. Molotch, M. Goulden, A. Kelly, and R. Bales. 2012. “Elevation-Dependent Influence of Snow Accumulation on Forest Greening.” Nature Geoscience 5: 705–709.
- Vicente-Serrano, S., S. Beguería, and J. Lopez-Moreno. 2010. “A Multiscalar Drought Index Sensitive to Global Warming: The Standardized Precipitation Evapotranspiration Index.” Journal of Climate 23: 1696–1718. https://doi.org/10.1175/2009JCLI2909.1.
- Vicente-Serrano, S., C. Gouveia, J. Camarero, S. Beguería, R. Trigo, J. López-Moreno, C. Azorín-Molina, E. Pasho, J. Lorenzo-Lacruz, J. Revuelto, et al. 2013. “Response of Vegetation to Drought Time-Scales Across Global Land Biomes.” Proceedings of the National Academy of Sciences 110: 52–57. https://doi.org/10.1073/pnas.1207068110.
- Wang, Y., T. Chen, Q. Wang, and L. Peng. 2023. “Time-Lagged and Cumulative Effects of Drought and Anthropogenic Activities on China’s Vegetation Greening from 1990 to 2018.” International Journal of Digital Earth 16 (1): 2233–2258. https://doi.org/10.1080/17538947.2023.2224086.
- Wang, Z., M. Cheng, Y. Zhang, S. Peng, and H. Huang. 2023. “A 30 m Annual Land Cover Dataset of Chinese Loess Plateau from 1990 to 2020.” Zenodo. https://doi.org/10.5281/zenodo.10225564.
- Wang, J., X. Li, G. Christakos, Y. Liao, T. Zhang, and X. Gu. 2010. “Geographical Detectors-Based Health Risk Assessment and Its Application in the Neural Tube Defects Study of the Heshun Region, China.” International Journal of Geographical Information Science 24 (1): 107–127. https://doi.org/10.1080/13658810802443457.
- Wang, F., X. Pan, G. Cynthia, X. Cao, S. Wang, L. Gu, D. Wang, and Q. Lu. 2020. “Vegetation Restoration in Northern China: A Contrasted Picture.” Land Degradation & Development 31 (6): 1–8.
- Wang, Z., W. Yao, Q. Tang, L. Liu, P. Xiao, X. Kong, P. Zhang, F. Shi, and Y. Wang. 2018. “Continuous Change Detection of Forest/Grassland and Cropland in the Loess Plateau of China Using All Available Landsat Data.” Remote Sensing 10 (11): 1775.
- Wei, Y., S. Sun, D. Liang, and Z. Jia. 2022. “Spatial–Temporal Variations of NDVI and Its Response to Climate in China from 2001 to 2020.” International Journal of Digital Earth 15 (1): 1463–1484. https://doi.org/10.1080/17538947.2022.2116118.
- Wu, D., X. Zhao, S. Liang, T. Zhou, K. Huang, B. Tang, and W. Zhao. 2015. “Time-Lag Effects of Global Vegetation Responses to Climate Change.” Global Change Biology 21 (9): 3520–3531. https://doi.org/10.1111/gcb.12945.
- Zhan, C., C. Liang, L. Zhao, S. Jiang, K. Niu, and Y. Zhang. 2022. “Drought-Related Cumulative and Time-Lag Effects on Vegetation Dynamics Across the Yellow River Basin, China.” Ecological Indicators 143: 109409. https://doi.org/10.1016/j.ecolind.2022.109409.
- Zhang, J., Y. Zhang, N. Cong, T. Li, Z. Guang, Z. Zheng, G. Jie, Y. Zhu, and Z. Yu. 2023. “Coarse Spatial Resolution Remote Sensing Data with AVHRR and MODIS Miss the Greening Area Compared with the Landsat Data in Chinese Drylands.” Frontiers in Plant Science 14: 1129665. https://doi.org/10.3389/fpls.2023.1129665.
- Zhao, J., S. Huang, Q. Huang, H. Wang, G. Leng, and W. Fang. 2020b. “Time-Lagged Response of Vegetation Dynamics to Climatic and Teleconnection Factors.” Catena 189: 104474. https://doi.org/10.1016/j.catena.2020.104474.
- Zhao, A., Q. Yu, L. Feng, A. Zhang, and T. Pei. 2020a. “Evaluating the Cumulative and Time-Lag Effects of Drought on Grassland Vegetation: A Case Study in the Chinese Loess Plateau.” Journal of Environmental Management 261: 110214. https://doi.org/10.1016/j.jenvman.2020.110214.
- Zheng, K., J. Wei, J. Pei, H. Cheng, X. Zhang, F. Huang, and F. Li. 2019. “Impacts of Climate Change and Human Activities on Grassland Vegetation Variation in the Chinese Loess Plateau.” Science of the Total Environment 660: 236–244. https://doi.org/10.1016/j.scitotenv.2019.01.022.