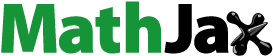
ABSTRACT
Urbanization improves the living environment of billions of people but negatively impacts the local carbon cycle as it encroaches on ecosystems. We analyzed this dual effect of urbanization by quantifying linkages between several indicators — urbanization-induced terrestrial carbon storage loss (uTCSL), per capita urban area (PCUA), and urban land use efficiency (LCRPGR) — within the Hohhot-Baotou-Ordos (HBO) region in 1990–2100. Results show that the growth of 4.45 million urban residents and 420.70 km2 urban area led to a uTCSL of 815.74 GgC in the HBO region in 1990–2020. The uTCSL contributed to improving the urban crowding indicated by PCUA but was subject to LCRPGR. The PCUA decreased by −33.75 m2/person under high land use efficiency (LCRPGR<1) in 1990–2010. Although the PCUA increased by 23.59 m2/person from 2010 to 2020, it resulted in a 2.08-fold increase in uTCSL compared to 1990–2010, with each additional 1 m2 increase in PCUA being associated with carbon storage loss of 24.50 GgC. Our projections indicate that regulating LCRPGR twenty years before the urban population reaches its peak could benefit both uTCSL and PCUA. This study provides valuable insights for urban planning aligned with the UN 2030 Agenda, especially for arid and semi-arid cities.
1. Introduction
Urbanization improves human well-being and socio-economic development, advancing many aspects of the Sustainable Development Goals (SDGs), such as Sustainable Cities and Communities (SDG 11) and No Poverty (SDG 1) (Sun et al. Citation2020; Zhou et al. Citation2023). One of the benefits of urbanization is the provision of sufficiently ample living space for a growing number of urban residents (Klein, Dekker, and van Zanden Citation2017; Xu et al. Citation2011). However, the intensification of urban expansion, especially in the last two decades, exceeded the growth in the number of residents by 1.3∼2 folds (Angel et al. Citation2011; UN Citation2018). Such observed land use patterns have an irreversible impact on ecosystem services and environmental sustainability at multiple scales (Chatterjee et al. Citation2022; Das and Das Citation2019; Sun and Li Citation2017), such as the loss of original ecosystems and their carbon storage in biomass and soils (Feng et al. Citation2017; He et al. Citation2016; Li et al. Citation2020; Liu et al. Citation2019; Seto, Guneralp, and Hutyra Citation2012), ultimately damaging the natural support systems essential for human well-being. By 2030, the projected urbanization-induced terrestrial carbon storage loss (uTCSL) may reach approximately 1.38 PgC (Seto, Guneralp, and Hutyra Citation2012), posing a significant challenge in achieving the SDGs and the ambitious 1.5°C goal set by the Paris Agreement. Therefore, addressing the urban living environment and mitigating uTCSL is an urgent topic for policymakers tasked with planning future urban development (Holobâcă et al. Citation2022; Koroso, Lengoiboni, and Zevenbergen Citation2021).
Because of its large impact on natural ecosystem services, uTCSL has been widely reported in previous studies. Assessing the uTCSL mainly includes field surveys (Hutyra et al. Citation2011; Ren et al. Citation2011) and the integration of remote sensing data with model simulations (Basu et al. Citation2023; He et al. Citation2016; Liu et al. Citation2019; Seto, Guneralp, and Hutyra Citation2012; Yan et al. Citation2018). Integrating remote sensing with model simulations is becoming more common because it can be used to predict and simulate the effects of urban expansion on carbon storage at various scales, allowing to conduct large-scale research. Thus, several studies have made predictions at global (Seto, Guneralp, and Hutyra Citation2012), national (Chuai et al. Citation2015; Jiang et al. Citation2023; Liu et al. Citation2019), and single-city scales (He et al. Citation2016; Li et al. Citation2020; Yan et al. Citation2018). Urban sprawl is widespread across the globe, especially in developing countries, where massive urban expansion has resulted in huge uTCSL. For example, it has been estimated that from 1990 to 2018, uTCSL in China amounted to approximately 1.35 PgC (Jiang et al. Citation2023). However, existing research does not comprehensively evaluate the cost of carbon storage losses in the context of improving urban settlements.
Over the period 2000–2020, the per capita urban area (PCUA) in major global cities increased from 84.56 m2 to 122.59 m2, improving living conditions, public service availability, and city prosperity (Wang, Shi, and Kuang Citation2021). Considering that population growth is the main driver of urban expansion, it can be inferred that uTCSL is a necessary cost contributing to the growth in PCUA. However, there is little research on such a people-centered coupling of the urban living environment versus ecosystem functioning, hindering approaches to address cross-cutting issues related to SDGs focused on urban and environmental sustainability.
The LCRPGR indicator, recommended by SDG 11, is available for quantifying the nexus between people and land-use change in urban areas (UN Citation2018; UN-Habitat Citation2018). Since the twenty-first century, the global rate of urban expansion has greatly surpassed urban population growth, leading to inefficient urban land use (Cai et al. Citation2020; Holobâcă et al. Citation2022; Mithun et al. Citation2022; Wang et al. Citation2020). However, the main use of the LCRPGR focuses on assessing the correlation between urban population growth and urban expansion, limiting its potential to understand the dynamics of people-land-environment systems. A potential breakthrough could lie in harnessing the LCRPGR to explain the nexus between people and land in urban areas, and its connections with uTCSL. This requires a comprehensive research framework that could provide a critical reference for SDG-oriented urban planning. This approach aims to synergize urban living conditions and environmental sustainability, considering that a projected 70% of the global population will be urbanized in the near future (Bren et al. Citation2017; Chen et al. Citation2020; IPCC Citation2022; Seto, Guneralp, and Hutyra Citation2012; UN Citation2018; Xu et al. Citation2022).
The Hohhot-Baotou-Ordos (HBO) region, located in the drylands of northern China, is the political, economic, and cultural center of the Inner Mongolia Autonomous Region (Miao and Zhou Citation2023; Qi et al. Citation2023). The urbanization rate of the HBO region increased rapidly after 2000, from 55.96% to 80.99% in 2020 (Qi et al. Citation2023). Although the urban living conditions in the region have been improving, its delicate ecological system is in danger. To ensure the sustainable development of the urban and ecological environment of the HBO region, it is imperative to examine connections between uTCSL and the urban living environment while making future development strategies. In this study, we built a comprehensive framework by integrating ecosystem service and land use models, using various datasets, including land use/cover data, statistical data, carbon density data, and shared socioeconomic pathways (SSPs). The aim was to harness the explanatory power of LCRPGR to (1) reveal the nexus between people and urban space and its connections with uTCSL in the HBO region from 1990 to 2020, and (2) forecast the optimal pathway for urban expansion that enhances the urban living environment while mitigating the uTCSL by 2100. Our findings unravel the cross-cutting issues among different SDGs focused on urban and environmental sustainability. This study is of major importance for the coordinated development of the urban living environment and ecosystem services within the HBO region.
2. Data and methods
2.1. Study area
The HBO region includes the three cities of Hohhot, Baotou, and Ordos, and is located in the Inner Mongolia Autonomous Region (). The HBO region has a semi-arid climate and a fragile ecosystem, with an area of 13.20×104 km2, an average annual precipitation of 300 mm, and an annual average temperature of 8 °C (Zhou et al. Citation2023). The HBO region occupies 28.09% of the urban area, 41.64% of the urban population, and 52.72% of the Gross Domestic Product (GDP) but only 11.16% of the total extent in the Inner Mongolia Autonomous Region. In this regard, as the economic core of the Inner Mongolia Autonomous Region and an important part of the Yellow River Basin, the HBO region is required by the central government to build a high-quality development area (that is, attach importance to environmental protection and sustainability in the process of social and economic development). However, the urban area of the HBO region increased by 136.04 km2 in 1990–2010 and 286.66 km2 in 2010–2020, with the rate of the last decade being more than two-fold that of the earlier two decades. Urban expansion mainly encroached farmland (35.29%) and grassland (41.13%). With its recent rapid growth, the HBO region can be used as a natural experimental site for people-land-environment systems in an arid and semi-arid region.
2.2. Data and pre-processing
We used the China Land Use/cover Dataset (CLUD), SSPs dataset, demographics and GDP statistical dataset, driving factors dataset, terrestrial carbon density data, and auxiliary data (). The CLUD provides China’s land use/cover from 1990 to 2020, with a spatial resolution of 30 m and an overall accuracy for 26 land use/cover types greater than 80% (Kuang et al. Citation2022; Liu et al. Citation2014).
Table 1. Research data sources.
Driving factors included the relief degree of the land surface, GDP and population raster data, roads (distance to national roads, distance to provincial roads, distance to railways, distance to expressways), and government location (distance to capital city government location, distance to prefecture-level city government location, distance to county level city government location). Distance of roads and government locations was calculated using the Euclidian distance in ArcGIS. Future urban population and GDP data for each decade from 2030 to 2100 were derived from the SSPs dataset (Jiang et al. Citation2020). Demographics and GDP statistics of each city were from the provincial and municipal Bureau of Statistics and the China Statistical Yearbook. Carbon density data were collected from published literature (Liu et al. Citation2019; Wang et al. Citation2022; Yao et al. Citation2022). The spatial resolution of all gridded data has been set to 100 m.
2.3. Methodology
The framework used to explore the relationship between the urban living environment and uTCSL around the explanatory power of LCRPGR is shown in . First, we collected and pre-processed the research dataset for the period 1990–2100. Second, we quantitatively analyzed the spatiotemporal patterns of urban area, population, PCUA, uTCSL, and LCRPGR from 1990–2020 and explored the relationship between urban living environment and uTCSL. Third, we predicted the development trends of urban areas, uTCSL, and PCUA from 2020 to 2100 and defined a future development strategy. As the main goal of this analysis, we aimed to characterize the urban living environment and uTCSL around the explanatory power of LCRPGR, further simulate future development trends, and propose a development strategy. To achieve these goals, we used indicators capturing the following aspects: changes in urban population and urban expansion dynamics; spatial-temporal changes of uTCSL and the urban living environment; and dynamic changes in LCRPGR. These indicators were further used to derive the relationship between the urban living environment and uTCSL over the entire period from 1990 to 2100.
Figure 2. Flowchart of research framework. uTCSL: urbanization-induced terrestrial carbon storage loss; PCUA: per capita urban area. GDP: Gross Domestic Product. POP: urban population; UA: urban area.

2.3.1. Assessment of urban land use efficiency and urban living environment
LCRPGR is a performance indicator employed by the United Nations to assess land intensification in sustainable cities and communities (SDG 11). LCRPGR is calculated as the ratio of land consumption rate (LCR) to population growth rate (PGR):
(1)
(1)
(2)
(2)
(3)
(3) where UAt1 and UAt2 are the urban areas (km2) at times t1 to t2, respectively; UPt1 and UPt2 are the urban population (million people) at times t1 and t2, respectively.
The urban living environment is mainly composed of housing, transportation, and public spaces, which play a significant role in determining the quality of the urban living environment (Xu et al. Citation2023; Yuan and Zhang Citation2020). One measure of urban development standards and resident welfare is the assessment of the urban living environment. The indicator PCUA is a tool for tracking the evolution of the urban population and urban area, as well as a key indicator for evaluating the urban living environment (Feng et al. Citation2017; Klein, Dekker, and van Zanden Citation2017; Xu et al. Citation2011). Thus, PCUA is viewed as a typical indicator for changing the urban living environment and its effects on ecosystems in this study. The PCUA is defined as the ratio of urban area to urban population:
(4)
(4) where PCUA is the per capita urban area (m2/person); UA is the urban area (m2); UP is the urban population (person).
2.3.2. Estimation of terrestrial carbon storage
Terrestrial carbon storage (TCS) was calculated by integrating land use/cover types and their carbon densities () based on the Carbon module in the InVEST model version 3.12.1 (Nelson et al. Citation2009). The carbon storage of regional terrestrial ecosystems was calculated by the collected carbon density of aboveground biomass, belowground biomass, soil, and dead organic matter in arid and semi-arid regions:
(5)
(5) where Ct, Ca, Cb, Cs and Cd are total carbon storage, aboveground biomass, belowground biomass, soil, and dead organic matter, respectively.
Table 2. Carbon density of different land use types in arid and semi-arid regions (mg/hm2).
Based off this, we examined the TCS changes caused by urban expansion from 1990 to 2100 across the study area and analysed the share of uTCSL caused by the conversion of farmland, forest, and grassland to new urban land.
2.3.3. Scenario setting and simulation process
The urban area of the HBO region was predicted for 2020–2100 using five SSP scenarios from the IPCC (SSP1, SSP2, SSP3, SSP4, and SSP5) (). Numerous previous studies have demonstrated a strong correlation between urban population increase and urban expansion (Zhang et al. Citation2020; Zhou et al. Citation2020). Subsequently, GDP—an indicator of economic growth—became another reliable measure of the expansion of urban areas (Chen et al. Citation2020). Thus, we established an urban area simulation equation based on historical GDP and urban population at the regional level in the SPSS software (Chen et al. Citation2020). On this basis, the future urban area was obtained based on the GDP and urban population of the future SSP scenarios:
(6)
(6) where ΔUAi,t is the urban area in scenario i and period t; ΔGDPi,t and ΔPOPi,t are the GDP and urban population in scenario i and period t, respectively; θ, ai,t and bi,t are constants.
Table 3. Description of each SSPs development path.
The patch-generating land use simulation (PLUS) model was used to predict the spatial distribution of urban areas. The PLUS model consists of the land expansion analysis strategy (LEAS), cellular automata (CA) based on multiple random patch seeds (CARS), validation module, etc (Liang et al. Citation2021). Firstly, the historical CLUD dataset and the driving factors dataset were employed in the LEAS to attain the probability of development for urban areas in the future. Secondly, the conversion matrix for land use and neighborhood weight of the CARS was utilized to accomplish the spatial allocation of urban areas with accuracy verification, thereby obtaining a simulated spatial distribution of future urban areas. Finally, these were integrated with the InVEST model to simulate future TCS.
The Kappa coefficient, Producer Accuracy (PA), User Accuracy (UA), and Overall Accuracy (OA) were used to comprehensively evaluate the simulated urban spatial distribution (). The Kappa coefficient of urban expansion remained above 0.76 in the HBO region, with an average value of 0.83±0.07. PA, UA, and OA were 85.03±4.00%, 86.67±4.56% and 87.61±1.67%, respectively. Therefore, it is reliable to evaluate TCS based on urban expansion simulations in the HBO region.
3. Results
3.1. TCS loss and uTCSL in 1990–2020
The total loss of TCS in the HBO region was 35,912.41 GgC in 1990–2020 (a-b). The total loss of TCS decreased over the last three periods (in 1990–2000, 2000–2010, and 2010–2020) at differing magnitudes. Specifically, 2000–2010 witnessed the largest loss in total TCS with 24,219.62 GgC (67.44%). The total TCS for 2010–2020 dropped to 6,754.35 GgC (18.81%).
Figure 4. Carbon storage change and urbanization-induced carbon storage loss for different land use/cover types in the HBO region from 1990 to 2020. uTCSL: urbanization-induced terrestrial carbon storage loss.

Contrary to the decreasing trend of total TCS loss, the uTCSL experienced an increasing trend in the HBO region from 1990 to 2020. There was a total of 420.70 km2 of land use/cover changes due to urban expansion in 1990–2020, with an uTCSL of 815.74 GgC. The uTCSL increased from 264.80 GgC in 1990–2010 to 550.94 GgC in 2010–2020, which was a 2.08-fold increase compared to 1990–2010. Furthermore, the annual rate of uTCSL in 2010–2020 was 29.18-fold and 2.24-fold that of the periods of 1990–2000 and 2000–2010, respectively. Moreover, the spatial changes of uTCSL in the HBO region were significant but differed in magnitude from 1990 to 2020 (d). Specifically, Hohhot witnessed the highest value in uTCSL, with a total of 328.29 GgC in 1990–2020, followed by Baotou with 288.49 GgC, and Ordos with 194.96 GgC.
Urban expansion, which mostly impacted farmland, forest, and grassland ecosystems in the HBO region, was one of the main factors contributing to the loss of TCS. From 1990 to 2020, urbanization of the HBO region converted farmland (148.47 km2), forest (10.89 km2), and grassland ecosystems (173.07 km2) (c). Specifically, the urban expansion in Hohhot and Baotou had the greatest impact on the carbon storage of both farmland and grassland ecosystems, while in Ordos it had the greatest impact on the carbon storage of grassland ecosystems. The uTCSL of farmland and grassland ecosystems in Hohhot during the period 1990–2020 was 137.54 and 145.10 GgC, respectively. The uTCSL of Baotou was 195.71 GgC from grassland ecosystems and 179.62 GgC from farmland ecosystems. On the other hand, Ordos witnessed 130.83 GgC of uTCSL in its grassland ecosystems. Moreover, the proportion of farmland and forest ecosystems trended downward, while the occupation of grassland ecosystems increased rapidly. The proportion of grassland ecosystems occupied by urban expansion increased from 33.68% in 1990–2000 to 43.07% in 2010–2020.
3.2. Impacts of people and space linkages on uTCSL during 1990–2020
The HBO region experienced a 4.45 million urban population increase between 1990 and 2020 (). From 1990 to 2010, the HBO region had an effective land use efficiency (LCRPGR<1), but the PCUA decreased by −33.75 m2/person. During this period, the urban environment was congested and offered poor living conditions. Also, the PCUA was only 83.62 m2/person in 2010. Urban expansion in the HBO region witnessed the largest shifts between 2010 and 2020, with a growth of 296.11 km2 (70.39%); at the same time, the PCUA improved significantly, changing from 23.59 m2/person to 107.21 m2/person in 2020. However, it came at the cost of a 2.08-fold increase in uTCSL compared to 1990–2010 and had an inefficient LCRPGR (LCRPGR = 1.98). This indicates that there has been an imbalance between the uTCSL and the enhancement of the urban living environment in the HBO region between 1990 and 2020.
Figure 5. Dynamic linkages between urban population, urban area, PCUA, and uTCSL in the HBO region from 1990 to 2020. uTCSL: urbanization-induced terrestrial carbon storage loss; PCUA: per capita urban area.

From 1990 to 2020, the HBO region showed a significant spatio-temporal variation of PCUA, with great regional differences in the TCS required for the improvement of the urban living environment (). Analysing the relationship between the change of the PCUA and uTCSL helps determine the loss of TCS required for the improvement of the urban living environment. The carbon storage consumed by each increment of 1 m2 PCUA was 24.50 GgC in the HBO region between 2010 and 2020. The TCS required for the improvement of the urban living environment in the Hohhot was the largest, with the carbon storage consumed by each increment of 1 m2 PCUA being 13.70 GgC. The carbon storage consumed by each increment of 1 m2 PCUA was 6.50 and 4.20 GgC in the Baotou and Ordos, respectively.
3.3. Costs of improving the urban living environment on uTCSL from 2020 to 2100
Simulations for the period from 2020 to 2100 show that the urban population in the HBO region is projected to first increase and then decrease from 2020 to 2100. Urban population in the SSP scenarios (SSP1, SSP2, SSP3, SSP4, and SSP5), on average, is projected to increase to 7.50±0.40 million people in 2050, then decrease to 5.23±0.61 million people in 2100 (a). By contrast, the urban area and the PCUA are expected to continue to increase under the SSP scenarios, at an average of 2387.71±784.52 km2 and 435.97±149.94 m2/person in 2100, respectively (b and d). At the end of this century (2100), the uTCSL is expected to reach 1770.96±340.25 GgC in the SSP scenarios (c).
Figure 7. Projected changes in urban population, urban area, PCUA, and uTCSL under different scenarios in the HBO region from 2020 to 2100. uTCSL: urbanization-induced terrestrial carbon storage loss; PCUA: per capita urban area.

Among the different scenarios, the SSP5 scenario is projected to have the largest changes in the HBO region in terms of urban area, urban population, PCUA, and uTCSL, whereas each increment of 1 m2 PCUA is expected to need 10.53 GgC of uTCSL (). However, under the SSP1 (sustainable development) and SSP4 (regional development) scenarios, the urban population, the urban area, and the PCUA are projected to gradually increase, and the uTCSL is expected to be modest. Under the SSP1 and SSP4 scenarios, each increment of 1 m2 PCUA will need 8.29 and 5.65 GgC of uTCSL, respectively. Increasing outward urban development in the HBO region may endanger local ecosystems and raise the cost of carbon storage for the improvement of the urban living environment. The effect of urban growth on ecological development is lessened in the event of intensive urban development, which is more appropriate for the arid and semi-arid ecosystems of the HBO region. The following two stages are particularly important in the future urban expansion process of the HBO region.
Figure 8. Projected change of uTCSL for each increment of 1 m2 of PCUA in the HBO region from 2020 to 2100. uTCSL: urbanization-induced terrestrial carbon storage loss; PCUA: per capita urban area.

From 2020 to 2030, the urban living environment in the HBO region is expected to significantly improve and essentially reach 150 m2 one person. Additionally, it has been simulated that the LCRPGR in the HBO region is projected to be inefficient under the SSP scenarios in this study (), despite SDG11's aim to achieve efficiency in urban land use by 2030. From 2030 to 2060, the urban living environments is projected to continue to improve but the uTCSL is expected to moderate. The PCUA is simulated to increase from 145.68±7.93 m2/person in 2030 to 203.37±22.36 m2/person in 2060. However, compared to the period between 2020 and 2030, uTCSL is expected to grow more slowly. In addition, LCRPGR is projected to present a decreasing trend, possibly reaching an efficient development state in 2030–2050. Thus, controlling urban expansion twenty years before the urban population reaches its peak is expected to effectively improve the urban living environment and mitigate the uTCSL.
Table 4. LCRPGR in the HBO region from 2020 to 2100.
4. Discussion
4.1. A hidden trade-off between uTCSL and enhancing the urban living environment
The urbanization of the HBO region resulted in a significant impact on TCS with 815.74 GgC during the period 1990–2020 (). This had a detrimental effect on ecosystem services. The uTCSL in the HBO region agreed with previous studies carried out at multiple scales, such as for all Chinese cities (Jiang et al. Citation2023; Liu et al. Citation2019), Beijing (He et al. Citation2016), and Wuhan (Li et al. Citation2020). The amount of uTCSL in the HBO region was relatively small, with uTCSL in Beijing being 6.81 TgC in 1990–2011 and in Wuhan being 3.05 TgC in 2000–2015 (He et al. Citation2016; Li et al. Citation2020). This may be related to the fact that the HBO region is located in an arid and semi-arid area. We also showed that urbanization in the HBO region accelerated between 2010 and 2020, resulting in a 29.18-fold and 2.24-fold rise in the annual rate of uTCSL compared to 1990–2000 and 2000–2010, respectively. However, the total carbon storage loss in the HBO region witnessed the largest change in 2000–2010 (67.44%) but showed a decreasing trend during 2010–2020 (18.81%). This might be related to the Chinese government's efforts to restore the environment, build an ecological barrier in northern China, and return farmlands to forests and grasslands. Therefore, the impact of urbanization on the TCS loss in the HBO region (also arid and semi-arid) necessitates attention and should not be disregarded.
We also found a relationship between improving the urban living environment and uTCSL ( and ). The imbalanced growth of urban population and land space, as well as the significant loss of TCS, are major problems with the urbanization process in the HBO region. In the past 30 years, land use efficiency in the HBO region has experienced a change from an effective to ineffective LCRPGR. The results of this study are consistent with the results of nationwide studies in China (Wang et al. Citation2020). Before 2010, land use efficiency was effective (LCRPGR<1), and the rate of urbanization of the urban population was higher than that of the land. However, the PCUA was just 82.44 m2 in 2010, which was smaller than China's PCUA in 2000 (Wang, Shi, and Kuang Citation2021). Furthermore, there was a continuous uTCSL increase. With the implementation of national and regional development policies (e.g. China's Western Development), social and economic development has been significantly improved in the HBO region between 2010 and 2020 (Miao and Zhou Citation2023), thus satisfying the people's requirements for a better life has been the primary task of urbanization development. During 2010–2020, the HBO region experienced a substantial enhancement in urban development and in the quality of urban living environments (the PCUA reached 107.21 m2 in 2020). Simultaneously, the uTCSL exhibited a remarkable 2.08-fold increase compared to 1990–2010 and land use efficiency became inefficient (LCRPGR>1). Therefore, the TCS cost of the urban living environment enhancement in the HBO region increased. Therefore, we provide a new framework in which linkages between people and space accelerate TCS loss in the HBO region, as well as a trade-off relationship between the uTCSL and enhancing the urban living environment.
Although urban land use efficiency has decreased and carbon storage loss has increased from 1990 to 2020, the PCUA in the HBO region is still lower than the global (122.59 m2/person) and Chinese (124.69 m2/person) average (Wang, Shi, and Kuang Citation2021). Therefore, a key concern for the HBO region's future urban development is to increase the quality of the urban living environment, improve urban land use efficiency, and mitigate the uTCSL. Urban expansion should be arranged and controlled in advance, with particular emphasis placed on the deterioration of ecological services and the devastation of the environment in ecologically vulnerable arid and semi-arid regions.
4.2. The P-OUD strategy is expected to synergistically improve the urban living environment and mitigate the uTCSL
With the ongoing rise in urban population and land space by the middle of this century, rapid urbanization will exert a substantial influence on carbon storage within terrestrial ecosystems (Basu et al. Citation2023; Chen et al. Citation2020; Das and Das Citation2019; Gao and O'Neill Citation2020; Seto, Guneralp, and Hutyra Citation2012). We also simulated urban population, urban expansion, uTCSL, urban living environment (PCUA), and land use efficiency (LCRPGR) following the IPCC shared socioeconomic development pathway in the HBO region of northern China from 2020 to 2100 (). According to the simulation's estimates, the urban area and PCUA are expected to continue to grow in this region (b and d), while the urban population is expected to increase at first and then decrease (a). These predictions are consistent with those of previous studies (Chen et al. Citation2020; Gao and O'Neill Citation2020). However, the uTCSL is projected to experience an increase-decrease-increase trend in the future (c). Moreover, we also found that the HBO region is expected to fail to achieve effective land use efficiency in 2030 under the SSPs, although it aims to achieve the effective development goals of urban population and urban land defined by 2030 SDG 11. Hence, an effective urban planning and administration regime should be established to rationally control urban expansion. In these situations, investigating an effective urban development strategy that integrates a high-quality urban living environment with environmental protection is one of the United Nations’ SDGs.
Therefore, we propose the Population-Oriented Urban Development (P-OUD) strategy. This strategy is mainly based on the change in urban population to effectively enhance the urban living environment and mitigate uTCSL by LCRPGR change in the future (). We offer two development scenarios. First, when the urban population peaks (Ppeak, t1), urban growth is controlled. Second, urban expansion is regulated when the urban growth line and the decline in the urban population intersect (t2). Thus, using the average of the SSP scenarios as a reference (UA1, PCUA1, LP1, and uTCSL1), we simulated the two P-OUD scenarios, including the line of UA2 (PCUA2, LP2, and uTCSL2) and line of UA3 (PCUA3, LP3, and uTCSL3).
Figure 9. Proposed Population-Oriented Urban Development for regulating future urban expansion. UA: urban area; POP: urban population; PCUA: per capita urban area; uTCSL: urbanization-induced terrestrial carbon storage loss.

Urban population is simulated to reach its peak in 2050 (t1). If urban expansion is kept in control by 2050 (UA3), the PCUA is projected to gradually increase from 2050 to 2100 (PCUA3), which is expected to greatly enhance the urban living environment and mitigate the uTCSL (uTCSL3). Furthermore, our simulation shows that the LCRPGR is projected to have an inverted-V shape until the urban population reaches its peak between 2030 and 2050. Therefore, adjusting urban expansion when LCRPGR declines in the HBO region is expected to have a substantial scientific value for the synergetic development of enhancing the urban living environment and mitigating uTCSL. The urban population decline and urban expansion is simulated to intersect in 2080 (t2). Controlling urban expansion from 2080 is expected to also improve the urban living environment and mitigate uTCSL. Hence, controlling urban expansion twenty years before the Ppeak can help both mitigate uTCSL and optimize the PCUA.
Overall, the integration of urban living environment improvements and mitigation of uTCSL is expected to provide a combined framework to synergistically promote urban development and ecological services in urban planning. Taking the HBO region as an example, the local government should initiate a series of plans to coordinate natural ecosystems and urban development, such as paying more attention to future urban population changes and establishing people-land-environment urban planning schemes. Moreover, the proposed strategy in this study can be extended to examine other urban areas in arid and semi-arid regions and developing countries.
4.3. Limitations and perspectives
In this study, we simulated the urban area in the HBO region using urban population and GDP as parameters. There is a robust correlation between the growth of GDP and the expansion of urban areas in many cities (Chen et al. Citation2020). However, a number of cities are presently working on reducing the correlation between urban expansion and GDP. Therefore, it is best to choose as many sustainable urban development indicators as possible for estimating urban area, including per capita GDP, urban water use, amount of urban green space, etc.
Numerous previous studies have presented empirical proof of the function, planning, and allocation ratio of urban green space (Dong et al. Citation2022; Chi et al. Citation2020). Various functions of urban green space, including aesthetic, cleaning, cooling, and humidifying effects on the environment, thus, significantly enhance the quality of life for urban residents (Dong et al. Citation2022; Eggimann Citation2022; Haaland and van den Bosch Citation2015). Within the context of the United Nations’ sustainable urban development and resilient city construction initiatives, national and regional policies have been vigorously promoting urban greening, as exemplified by the implementation of projects such as the ‘300 meters to glimpse at greenery, 500 meters to access a park’ initiative and the establishment of pocket parks (World's News Now Citation2023). Therefore, the contribution of urban green space and the offset of urban green space should be considered in the study of TCS loss caused by urbanization.
This study proposed P-OUD using the HBO region as an example. The inclusion of urban expansion under the P-OUD in future urban development planning is expected to effectively enhance the urban living environments and mitigate the uTCSL, which is projected to have important scientific significance for the development of SDGs. This approach needs to be further validated and applied to other urban areas in developing countries or arid and semi-arid regions.
5. Conclusions
Based on high-resolution land use/cover, demographics, GDP, carbon density, and SSPs datasets of the HBO region, we utilized spatio-temporal statistical methodologies and model simulations to investigate the relationship between uTCSL and the urban living environment. We proposed a strategy that aims to build synergy among the mitigation of uTCSL and enhancement of the urban living environment based on the powerful tool of LCRPGR. Overall, it was found that the uTCSL increased unevenly in the HBO region between 1990 and 2020. Urbanization was intensive and led to inadequate urban living environment conditions during the period 1990–2010, while the urban living environment improved and uTCSL increased during the period 2010–2020. However, TCS is a costly ecosystem change that has not been fully translated into resident welfare, showing that certain challenges to sustainable development in the HBO region still exist. Considering future urban population growth in the HBO region, controlling urban expansion in advance from 2030 to 2060 is key to steadily enhancing the urban living environment and mitigating uTCSL. More specifically, controlling urban expansion twenty years in advance of the Ppeak can make LCRPGR follow an inverted-V shape, which is expected to help to achieve a mutually beneficial outcome of uTCSL reduction and PCUA growth. We comprehensively analyzed the trade-off relationship between enhancing the urban living environment and mitigating uTCSL, considering future population changes, and highlighting the importance of planning and controlling urban expansion. We used the HBO region as an example to explore the relationship between the urban living environment and uTCSL through the lens of LCRPGR to connect people and space in arid and semi-arid areas. The research will provide an important scientific basis for identifying the optimal development path between urban expansion and ecosystem carbon storage loss in arid and semi-arid areas. The strategies and findings of this study can be extended to similar regions globally to accelerate land use efficiency, improve the living environment, mitigate uTCSL, and align with multiple SDG goals.
Acknowledgements
We are grateful to the editors and reviewers for reading this research and providing valuable comments and suggestions.
Disclosure statement
No potential conflict of interest was reported by the author(s).
Data availability statement
The data that support the findings of this study are available from the corresponding author, upon reasonable request.
Additional information
Funding
References
- Angel, Shlomo, Jason Parent, Daniel L. Civco, Alexander Blei, and David Potere. 2011. “The Dimensions of Global Urban Expansion: Estimates and Projections for All Countries, 2000–2050.” Progress in Planning 75: 53–107. https://doi.org/10.1016/j.progress.2011.04.001.
- Basu, Tirthankar, Arijit Das, Ketan Das, and Paulo Pereira. 2023. “Urban Expansion Induced Loss of Natural Vegetation Cover and Ecosystem Service Values: A Scenario-Based Study in the Siliguri Municipal Corporation (Gateway of North-East India).” Land Use Policy 123: 106838. https://doi.org/10.1016/j.landusepol.2023.106838.
- Bren, d'Amour Christopher, Femke Reitsma, Giovanni Baiocchi, Stephan Barthel, Burak Güneralp, Karl-Heinz Erb, Helmut Haberl, Felix Creutzig, and Karen C. Seto. 2017. “Future Urban Land Expansion and Implications for Global Croplands.” Proceedings of the National Academy of Sciencesof the United States of America 114 (34): 8939–8944. https://doi.org/10.1073/pnas.1606036114.
- Cai, Guoyin, Jinxi Zhang, Mingyi Du, Chaopeng Li, and Shu Peng. 2020. “Identification of Urban Land Use Efficiency by Indicator-SDG 11.3.1.” PLoS One 15 (12): e0244318. https://doi.org/10.1371/journal.pone.0244318.
- Chatterjee, Soumen, Shyamal Dutta, Ipsita Dutta, and Arijit Das. 2022. “Ecosystem Services Change in Response to Land Use Land Cover Dynamics in Paschim Bardhaman District of West Bengal, India.” Remote Sensing Applications: Society and Environment 27: 100793. https://doi.org/10.1016/j.rsase.2022.100793.
- Chen, Guangzhao, Xia Li, Xiaoping Liu, Yimin Chen, Xun Liang, Jiye Leng, Xiaocong Xu, et al. 2020. “Global Projections of Future Urban Land Expansion Under Shared Socioeconomic Pathways.” Nature Communications 11: 537. https://doi.org/10.1038/s41467-020-14386-x.
- Chuai, Xiaowei, Xianjin Huang, Qinli Lu, Mei Zhang, Rongqin Zhao, and Junyu Lu. 2015. “Spatiotemporal Changes of Built-Up Land Expansion and Carbon Emissions Caused by the Chinese Construction Industry.” Environmental Science & Technology 49 (21): 13021–13030. https://doi.org/10.1021/acs.est.5b01732.
- Das, Manob, and Arijit Das. 2019. “Dynamics o Urbanization and its Impact on Urban Ecosystem Services (Uess): A Study of a Medium Size Town of West Bengal, Eastern India.” Journal of Urban Management 8: 420–434. https://doi.org/10.1016/j.jum.2019.03.002.
- Dong, Yulin, Zhibin Ren, Yao Fu, Nanlin Hu, Yujie Guo, Guangliang Jia, and Xingyuan He. 2022. “Decrease in the Residents’ Accessibility of Summer Cooling Services Due to Green Space Loss in Chinese Cities.” Environment International 158: 107002. https://doi.org/10.1016/j.envint.2021.107002.
- Eggimann, Sven. 2022. “Expanding Urban Green Space with Superblocks.” Land Use Policy 117: 106111. https://doi.org/10.1016/j.landusepol.2022.106111.
- Feng, Yunyun, Dengsheng Lu, Qi Chen, Michael Keller, Emilio Moran, Maiza Nara dos-Santos, Edson Luis Bolfe, and Mateus Batistella. 2017. “Examining Effective Use of Data Sources and Modeling Algorithms for Improving Biomass Estimation in a Moist Tropical Forest of the Brazilian Amazon.” International Journal of Digital Earth 10 (10): 996–1016. https://doi.org/10.1080/17538947.2017.1301581.
- Gao, Jing, and Brian C. O'Neill. 2020. “Mapping Global Urban Land for the 21st Century with Data-Driven Simulations and Shared Socioeconomic Pathways.” Nature Communications 11: 2302. https://doi.org/10.1038/s41467-020-15788-7.
- Haaland, Christine, and Cecil Konijnendijk van den Bosch. 2015. “Challenges and Strategies for Urban Green-Space Planning in Cities Undergoing Densification: A Review.” Urban Forestry & Urban Greening 14: 760–771. https://doi.org/10.1016/j.ufug.2015.07.009.
- He, Chunyang, Da Zhang, Qingxu Huang, and Yuanyuan Zhao. 2016. “Assessing the Potential Impacts of Urban Expansion on Regional Carbon Storage by Linking the LUSD-Urban and Invest Models.” Environmental Modelling & Software 75: 44–58. https://doi.org/10.1016/j.envsoft.2015.09.015.
- Holobâcă, Iulian-Horia, József Benedek, Cosmina-Daniela Ursu, Mircea Alexe, and Kinga Temerdek-Ivan. 2022. “Ratio of Land Consumption Rate to Population Growth Rate in the Major Metropolitan Areas of Romania.” Remote Sensing 14: 6016. https://doi.org/10.3390/rs14236016.
- Hutyra, R. Lucy, Byungman Yoon, Jeffrey Hepinstall-Cymerman, and Marina Alberti. 2011. “Carbon Consequences of Land Cover Change and Expansion of Urban Lands: A Case Study in the Seattle Metropolitan Region.” Landscape and Urban Planning 103 (1): 83–93. https://doi.org/10.1016/j.landurbplan.2011.06.004.
- IPCC. 2022. Climate Change 2022: Impacts, Adaptation and Vulnerability. https://www.ipcc.ch/report/ar6/wg2/.
- Jiang, Tong, Su Bu-da, Yanjun Wang, Guojie Wang, Yong Luo, Jianqing Zhai, Jinlong Huang, et al. 2020. “Gridded Datasets for Population and Economy Under Shared Socioeconomic Pathways for 2020–2100.” Climate Change Research 18: 381–383. https://doi.org/10.12006/j.issn.1673-1719.2022.106. In Chinese.
- Jiang, Huiping, Huadong Guo, Zhongchang Sun, Xiongfeng Yan, Jinlin Zha, Haili Zhang, and Sijia Li. 2023. “Urban-Rural Disparities of Carbon Storage Dynamics in China's Human Settlements Driven by Population and Economic Growth.” Science of the Total Environment 871: 162092. https://doi.org/10.1016/j.scitotenv.2023.162092.
- Klein, Goldewijk Kees, Stefan C. Dekker, and Jan Luiten van Zanden. 2017. “Per-capita Estimations of Long-Term Historical Land Use and the Consequences for Global Change Research.” Journal of Land Use Science 12 (5): 313–337. https://doi.org/10.1080/1747423X.2017.1354938.
- Koroso, H. Nesru, Monica Lengoiboni, and Jaap A. Zevenbergen. 2021. “Urbanization and Urban Land Use Efficiency: Evidence from Regional and Addis Ababa Satellite Cities, Ethiopia.” Habitat International 117: 102437. https://doi.org/10.1016/j.habitatint.2021.102437.
- Kuang, Wenhui, Shuwen Zhang, Guoming Du, Changzhen Yan, Shixin Wu, Rendong Li, Dengsheng Lu, et al. 2022. “Monitoring Periodically National Land Use Changes and Analyzing their Spatiotemporal Patterns in China during 2015–2020.” Journal of Geographical Sciences 32 (9): 1705–1723. https://doi.org/10.1007/s11442-022-2019-0.
- Li, Lu, Yan Song, Wei Xuhua, and Jie Dong. 2020. “Exploring the Impacts of Urban Growth on Carbon Storage Under Integrated Spatial Regulation: A Case Study of Wuhan, China.” Ecological Indicators 111: 106064. https://doi.org/10.1016/j.ecolind.2020.106064.
- Liang, Xun, Qingfeng Guan, Keith C. Clarke, Shishi Liu, Bingyu Wang, and Yao Yao. 2021. “Understanding the Drivers of Sustainable Land Expansion Using a Patch-Generating Land Use Simulation (PLUS) Model: A Case Study in Wuhan, China.” Computers, Environment and Urban Systems 85: 101569. https://doi.org/10.1016/j.compenvurbsys.2020.101569.
- Liu, Jiyuan, Wenhui Kuang, Zengxiang Zhang, Xinliang Xu, Yuanwei Qin, Jia Ning, Wancun Zhou, et al. 2014. “Spatiotemporal Characteristics, Patterns, and Causes of Land-Use Changes in China Since the Late 1980s.” Journal of Geographical Sciences 24 (2): 195–210. https://doi.org/10.1007/s11442-014-1082-6.
- Liu, Xiaoping, Shaojian Wang, Peijun Wu, Kuishuang Feng, Klaus Hubacek, Xia Li, and Laixiang Sun. 2019. “Impacts of Urban Expansion on Terrestrial Carbon Storage in China.” Environmental Science & Technology 53 (12): 6834–6844. https://doi.org/10.1021/acs.est.9b00103.
- Miao, Hongliang, and Hui Zhou. 2023. “Evaluation of County-Level Economic Efficiency and its Spatiotemporal Differentiation in Hohhot-Baotou-Ordos-Yulin Urban Agglomeration in China.” Humanities and Social Sciences Communications 10: 99. https://doi.org/10.1057/s41599-023-01568-3.
- Mithun, Sk, Mehebub Sahana, Subrata Chattopadhyay, Soumendu Chatterjee, Jaidul Islam, and Romulus Costache. 2022. “Comparative Framework for Spatially Explicit Urban Growth Modeling for Monitoring Urban Land-Use Efficiency and Sustainable Urban Development (SDG 11.3.1): A Study on Kolkata Metropolitan Area, India.” Geocarto International 37 (27): 17933–17970. https://doi.org/10.1080/10106049.2022.2136259.
- Nelson, Erik, Guillermo Mendoza, James Regetz, Stephen Polasky, Heather Tallis, DRichard Cameron, Kai MA Chan, et al. 2009. “Modeling Multiple Ecosystem Services, Biodiversity Conservation, Commodity Production, and Tradeoffs at Landscape Scales.” Frontiers in Ecology and the Environment 7 (1): 4–11. https://doi.org/10.1890/080023.
- Qi, Tao, Qiang Ren, Da Zhang, Wenlu Lu, and Chunyang He. 2023. “Impacts of Urban Expansion on Vegetation in Drylands: A Multiscale Analysis Based on the Vegetation Disturbance Index.” Ecological Indicators 147: 109984. https://doi.org/10.1016/j.ecolind.2023.109984.
- Ren, Yin, Xing Wei, Xiaohua Wei, Junzhong Pan, Pingping Xie, Xiaodong Song, Dan Peng, and Jingzhu Zhao. 2011. “Relationship Between Vegetation Carbon Storage and Urbanization: A Case Study of Xiamen, China.” Forest Ecology and Management 261 (7): 1214–e1223. https://doi.org/10.1016/j.foreco.2010.12.038.
- Seto, C. Karen, Burak Guneralp, and Lucy R. Hutyra. 2012. “Global Forecasts of Urban Expansion to 2030 and Direct Impacts on Biodiversity and Carbon Pools.” Proceedings of the National Academy of Sciencesof the United States of America 109: 16083–16088. https://doi.org/10.1073/pnas.1211658109.
- Sun, Xiao, and Feng Li. 2017. “Spatiotemporal Assessment and Trade-Offs of Multiple Ecosystem Services Based on Land Use Changes in Zengcheng, China.” Science of the Total Environment 609: 1569–1581. https://doi.org/10.1016/j.scitotenv.2017.07.221.
- Sun, Zhongchang, Sisi Yu, Huadong Guo, Cuizhen Wang, Zengxiang Zhang, and Ru Xu. 2020. “Assessing 40 Years of Spatial Dynamics and Patterns in Megacities Along the Belt And Road Region Using Satellite Imagery.” International Journal of Digital Earth 14 (1): 71–87. https://doi.org/10.1080/17538947.2020.1747560.
- UN. 2018. The Sustainable Development Goals Report 2018. https://www.un.org/en/desa/sustainable-development-goals-report-2018.
- UN-Habitat. 2018. SDG Indicator 11.3.1 Training Module: Land Use Efficiency. https://unhabitat.org/sites/default/files/.
- Wang, Chaoyue, Xianhua Guo, Li Guo, Baili Fang, Lilin Xia, Chunbo Wang, and Tingzhen Li. 2022. “Land Use Change and its Impact on Carbon Storage in Northwest China Based on FLUS-Invest: A Case Study of Hu-Bao-Er-Yu Urban Agglomeration.” Ecology and Environmental Sciences 31 (8): 1667–1679. https://doi.org/10.16258/j.cnki.1674-5906.2022.08.019. In Chinese.
- Wang, Yunchen, Chunlin Huang, Yaya Feng, Minyan Zhao, and Juan Gu. 2020. “Using Earth Observation for Monitoring SDG 11.3.1-Ratio of Land Consumption Rate to Population Growth Rate in Mainland China.” Remote Sensing 12 (3): 357. https://doi.org/10.3390/rs12030357.
- Wang, Qi'an, Jiancheng Shi, and Wenhui Kuang. 2021. “Global Ecosystems and Environment Observation Analysis Report Cooperation (2020): Global Urban Land Composite and Expansion in 2000–2020.” http://old.chinageoss.cn/geoarc/2020/index.html.
- Wenfeng, Chi , Jing Jia, Tao Pan, Liang Jin, and Xiulian Bai. 2020. “Multi-Scale Analysis of Green Space for Human Settlement Sustainability in Urban Areas of the Inner Mongolia Plateau, China.” Sustainability 12 (17): 6783. https://doi.org/10.3390/su12176783.
- World's News Now. 2023. Tianjin: In 2025, 500 Meters in the Urban Area will See Greenery in the Core Area of 300 Meters. https://worldsnewsnow.com/tianjin-in-2025-500-meters-in-the-urban-area-will-see-greenery-in-the-core-area-of-300-meters/.
- Xu, Jiayuan, Nana Liu, Elli Polemiti, Liliana Garcia-Mondragon, Jie Tang, Xiaoxuan Liu, Tristram Lett, et al. 2023. “Effects of Urban Living Environments on Mental Health in Adults.” Nature Medicine 29: 1456–1467. https://doi.org/10.1038/s41591-023-02365-w.
- Xu, Yong, Qing Tang, Jie Fan, Sean J. Bennett, and Yang Li. 2011. “Assessing Construction Land Potential and its Spatial Pattern in China.” Landscape and Urban Planning 103 (2): 207–216. https://doi.org/10.1016/j.landurbplan.2011.07.013.
- Xu, Dehe, Ke Zhang, Lianhai Cao, Xiangrong Guan, and Hengbin Zhang. 2022. “Driving Forces and Prediction of Urban Land Use Change Based on the Geodetector and CA-Markov Model: A Case Study of Zhengzhou, China.” International Journal of Digital Earth 15 (1): 2246–2267. https://doi.org/10.1080/17538947.2022.2147229.
- Yan, Yuchao, Xiaoping Liu, Feiyue Wang, Xia Li, Jinpei Ou, Youyue Wen, and Xun Liang. 2018. “Assessing the Impacts of Urban Sprawl on Net Primary Productivity Using Fusion of Landsat and MODIS Data.” Science of the Total Environment 613–614: 1417–1429. https://doi.org/10.1016/j.scitotenv.2017.09.139.
- Yao, Nan, Guangquan Liu, Shunbo Yao, Yuanjie Deng, Mengyang Hou, and Xiao Zhang. 2022. “Evaluating on Effect of Conversion from Farmland to Forest and Grassland Porject on Ecosystem Carbon Storage in Loess Hilly-Gully Region Based on Invest Model.” Bulletin of Soil and Water Conservation 42 (5): 329–336. https://doi.org/10.13961/j.cnki.stbctb.2022.05.040. In Chinese.
- Yuan, Kefeng, and Xiaoxia Zhang. 2020. “Analysis on the Influence of Urban Living Environment on Healthy Human Capital Based on Health Production Function.” Management Science Informatization and Economic Innovation Development Conference (MSIEID), 142–149. https://doi.org/10.1109/MSIEID52046.2020.00033.
- Zhang, Yihan, Xiaoping Liu, Guangliang Chen, and Guohua Hu. 2020. “Simulation of Urban Expansion Based on Cellular Automata and Maximum Entropy Model.” Science China Earth Sciences 63 (5): 701–712. https://doi.org/10.1007/s11430-019-9530-8.
- Zhou, Liang, Xuewei Dang, Qinke Suna, and Shaohua Wang. 2020. “Multi-Scenario Simulation of Urban Land Change in Shanghai by Random Forest and CA-Markov Model.” Sustainable Cities and Society 55: 102045. https://doi.org/10.1016/j.scs.2020.102045.
- Zhou, Siyang, Wei Li, Zhonggui Lu, and Rongwu Yue. 2023. “An Analysis of Multiple Ecosystem Services in A Large-Scale Urbanized Area of Northern China Based on the Food-Energy-Water Integrative Framework.” Environmental Impact Assessment Review 98: 106913. https://doi.org/10.1016/j.eiar.2022.106913.